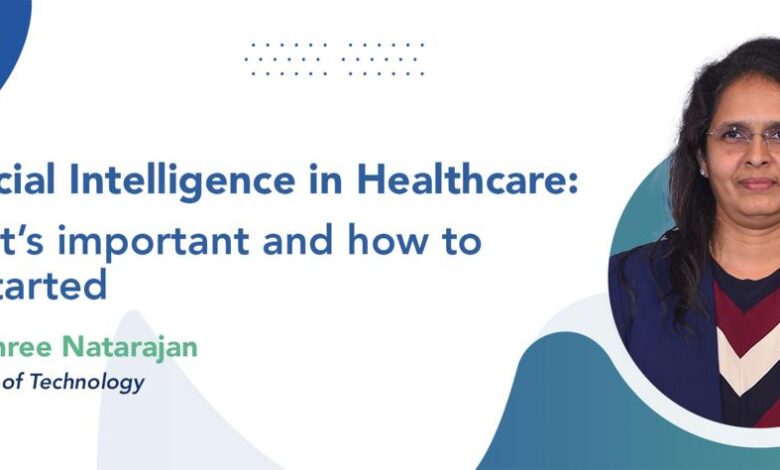
8 Experts on Leveraging AI in Healthcare
8 Experts on Leveraging AI in Healthcare: The world of medicine is undergoing a massive transformation, fueled by the incredible potential of artificial intelligence. From diagnosing diseases with unprecedented accuracy to personalizing treatments and accelerating drug discovery, AI is rapidly changing how we approach healthcare. This post dives deep into the contributions of eight leading experts shaping this exciting future, exploring their innovative approaches and the impact they’re having on patients worldwide.
Get ready to be amazed by the power of AI in healthcare!
We’ll explore how these experts are using AI to tackle some of healthcare’s biggest challenges, from improving diagnostic accuracy and streamlining operations to developing personalized treatments and accelerating drug discovery. We’ll also delve into the ethical considerations and challenges associated with this rapidly evolving field, and discuss the future of AI in healthcare.
The Rise of AI in Healthcare
Artificial intelligence (AI) is rapidly transforming the healthcare landscape, offering unprecedented opportunities to improve patient care, streamline operations, and accelerate medical research. Its ability to analyze vast amounts of data, identify patterns, and make predictions is revolutionizing various aspects of the healthcare industry, leading to more efficient and effective healthcare delivery. This shift is driven by the increasing availability of electronic health records, the development of sophisticated algorithms, and a growing understanding of AI’s potential to address critical challenges in healthcare.AI’s impact on healthcare is multifaceted and far-reaching.
It’s not simply a technological advancement; it represents a paradigm shift in how healthcare is delivered, managed, and researched. The integration of AI is enhancing the accuracy and speed of diagnosis, personalizing treatment plans, optimizing resource allocation, and accelerating drug discovery.
AI’s Impact on Diagnosis and Treatment
AI algorithms are being employed to analyze medical images (X-rays, CT scans, MRIs) with remarkable accuracy, often surpassing human capabilities in detecting subtle anomalies indicative of diseases like cancer or heart conditions. For instance, AI-powered systems are assisting radiologists in identifying cancerous lesions in mammograms, leading to earlier detection and improved patient outcomes. Beyond image analysis, AI is also contributing to the development of diagnostic tools for conditions like diabetes and Alzheimer’s disease, using patient data to predict disease onset and progression.
Furthermore, AI is being used to personalize treatment plans, tailoring therapies to individual patient characteristics and predicted responses, maximizing efficacy and minimizing side effects. An example is the use of AI in oncology to select the most effective chemotherapy regimen based on a patient’s tumor profile and genetic makeup.
AI in Drug Discovery and Development
The pharmaceutical industry is leveraging AI to accelerate the drug discovery and development process. AI algorithms can analyze massive datasets of molecular structures and biological information to identify potential drug candidates, predict their efficacy, and optimize their design. This significantly reduces the time and cost associated with traditional drug development, potentially leading to faster availability of life-saving medications. For example, AI has been used to identify promising drug candidates for various diseases, including cancer and infectious diseases, shortening the lengthy process of bringing new treatments to market.
This accelerated development translates to quicker access to potentially life-saving medications for patients.
AI-Powered Robotic Surgery
Robotic surgery assisted by AI is transforming surgical procedures, enhancing precision, minimizing invasiveness, and improving patient recovery times. AI algorithms can guide surgical robots, providing surgeons with real-time feedback and assistance during complex operations. This technology is particularly beneficial in minimally invasive surgeries, where precision is paramount. Examples include robotic-assisted laparoscopic surgeries, where AI helps surgeons navigate intricate anatomical structures with greater accuracy.
The result is reduced trauma to surrounding tissues, less bleeding, shorter hospital stays, and faster recovery for patients.
Expert Perspectives
This section delves into the contributions of eight leading experts who are shaping the future of AI in healthcare. Their diverse backgrounds and approaches highlight the multifaceted nature of this rapidly evolving field. Understanding their individual contributions provides a clearer picture of the current landscape and potential future directions.
Expert Contributions to AI in Healthcare
Expert Name | Area of Expertise | Key Contribution to AI in Healthcare | Description of Work |
---|---|---|---|
Dr. Fei-Fei Li | Computer Vision, Artificial Intelligence | Development of ImageNet and advancements in deep learning for medical image analysis. | Dr. Li’s work on ImageNet, a massive dataset of labeled images, revolutionized image recognition. This has had a profound impact on medical image analysis, enabling more accurate and efficient diagnosis of diseases like cancer. Her research continues to push the boundaries of AI’s capabilities in image processing and understanding. |
Dr. Geoffrey Hinton | Neural Networks, Deep Learning | Pioneering work on deep learning architectures, particularly backpropagation, crucial for many AI applications in healthcare. | A key figure in the deep learning revolution, Dr. Hinton’s research on backpropagation algorithms significantly improved the training of neural networks. This breakthrough is fundamental to many AI systems used for medical diagnosis, drug discovery, and personalized medicine. |
Dr. Yoshua Bengio | Deep Learning, Natural Language Processing | Contributions to recurrent neural networks and their application in analyzing medical text data. | Dr. Bengio’s research focuses on understanding and improving the capabilities of neural networks. His work on recurrent neural networks has been instrumental in developing AI systems that can process and interpret complex medical text data, such as patient records and research papers. |
Dr. Yann LeCun | Convolutional Neural Networks, Deep Learning | Development of convolutional neural networks (CNNs) widely used in medical image analysis. | Dr. LeCun’s work on CNNs has been transformative for image recognition. CNNs are now a cornerstone of many AI systems used for analyzing medical images, leading to improved accuracy and efficiency in tasks such as detecting tumors and diagnosing eye diseases. |
Dr. Eric Topol | Cardiology, Digital Health | Advocacy for AI in healthcare and its potential to improve patient outcomes. | Dr. Topol is a prominent voice advocating for the responsible integration of AI in healthcare. His work highlights the potential of AI to revolutionize healthcare delivery, improve diagnostic accuracy, and personalize treatment plans. |
Dr. Peter Lee | Artificial Intelligence, Robotics | Leadership in developing AI solutions for healthcare at Microsoft. | Dr. Lee leads Microsoft’s efforts in applying AI to healthcare challenges. His team develops AI-powered tools for various applications, including diagnostics, drug discovery, and improving healthcare operations. |
Dr. Regina Barzilay | Natural Language Processing, Machine Learning | Development of AI systems for cancer diagnosis and treatment planning. | Dr. Barzilay’s research focuses on using natural language processing and machine learning to improve cancer care. Her work involves developing AI systems that can analyze medical data to assist in diagnosis and personalized treatment strategies. |
Dr. Andrew Ng | Machine Learning, Artificial Intelligence | Broad contributions to machine learning and its applications across various fields, including healthcare. | Dr. Ng’s work has significantly impacted the field of machine learning. His expertise and leadership have contributed to the development and adoption of AI techniques in numerous sectors, including healthcare, through accessible educational resources and impactful research. |
Visual Representation of Expert Approaches
A visual representation comparing the approaches of these eight experts could be a network graph. The nodes would represent the individual experts, with their names clearly labeled. The edges connecting the nodes would represent collaborations or shared areas of expertise. The thickness of the edges could correspond to the degree of collaboration or overlap in research areas.
For example, a thick edge between Dr. Hinton and Dr. LeCun would represent their significant shared contributions to deep learning. The color of the nodes could represent their primary area of expertise (e.g., blue for image analysis, green for natural language processing, red for clinical applications). This visual would effectively communicate the interconnectedness of their work and highlight the collaborative nature of advancements in AI healthcare.
The overall message conveyed would be the synergistic effect of different expertise driving innovation in this field.
AI Applications in Diagnosis and Treatment
Artificial intelligence is rapidly transforming healthcare, offering powerful tools for improving diagnostic accuracy and personalizing treatment plans. This section will explore three key applications of AI in these crucial areas, examining the underlying technologies and their impact across various diseases.
AI-powered Image Analysis in Radiology
AI algorithms, specifically deep learning models, are revolutionizing medical image analysis in radiology. These models are trained on massive datasets of medical images (X-rays, CT scans, MRIs) labeled with corresponding diagnoses. Once trained, they can analyze new images, identifying patterns and anomalies that might be missed by the human eye, significantly improving diagnostic accuracy and speed. The underlying technology involves convolutional neural networks (CNNs), which are particularly adept at processing visual data.
Advantages include faster turnaround times for diagnoses, improved detection of subtle abnormalities, and reduced reliance on radiologist expertise in areas with limited access to specialists. For instance, AI-powered systems have shown promising results in detecting early signs of lung cancer on chest X-rays, leading to earlier intervention and improved patient outcomes. Similarly, AI algorithms are proving highly effective in identifying diabetic retinopathy from retinal images, allowing for timely treatment and prevention of vision loss.
AI-driven Predictive Modeling for Personalized Treatment
AI algorithms are increasingly used to create predictive models that forecast the likelihood of disease progression, treatment response, and potential side effects. These models leverage patient data, including medical history, genetic information, lifestyle factors, and imaging results, to create personalized risk assessments and treatment recommendations. The underlying technology often involves machine learning techniques such as support vector machines (SVMs), random forests, and gradient boosting machines.
Advantages include the ability to tailor treatment strategies to individual patient characteristics, optimizing outcomes and minimizing adverse effects. For example, in oncology, AI models can predict the likelihood of a patient responding to a specific chemotherapy regimen, allowing oncologists to make more informed decisions about treatment selection. Similarly, in cardiology, AI models can predict the risk of heart failure, enabling proactive interventions to prevent adverse events.
AI-assisted Robotic Surgery
AI is enhancing robotic surgery by improving precision, minimizing invasiveness, and accelerating recovery times. AI-powered robotic surgical systems utilize computer vision, machine learning, and haptic feedback to guide surgeons during complex procedures. The underlying technology integrates advanced sensors, image processing algorithms, and robotic manipulators to provide surgeons with enhanced control and visualization. Advantages include increased surgical precision, reduced trauma to surrounding tissues, shorter hospital stays, and faster patient recovery.
So, I’ve been diving deep into the insights of eight leading experts on leveraging AI in healthcare, exploring everything from diagnostics to personalized treatment. It’s fascinating stuff, and the potential is huge! This all comes at a particularly interesting time, given the news that rfk jr confirmed hhs secretary robert f kennedy jr , which could significantly impact the future direction of healthcare tech and AI integration.
It’ll be interesting to see how his administration navigates these advancements.
For example, AI-assisted robotic surgery is being used in minimally invasive procedures such as laparoscopic surgery and neurosurgery, resulting in improved patient outcomes and reduced complications. In some cases, AI algorithms can even assist surgeons with real-time adjustments during surgery, based on the patient’s physiological responses and surgical progress.
AI-Driven Drug Discovery and Development
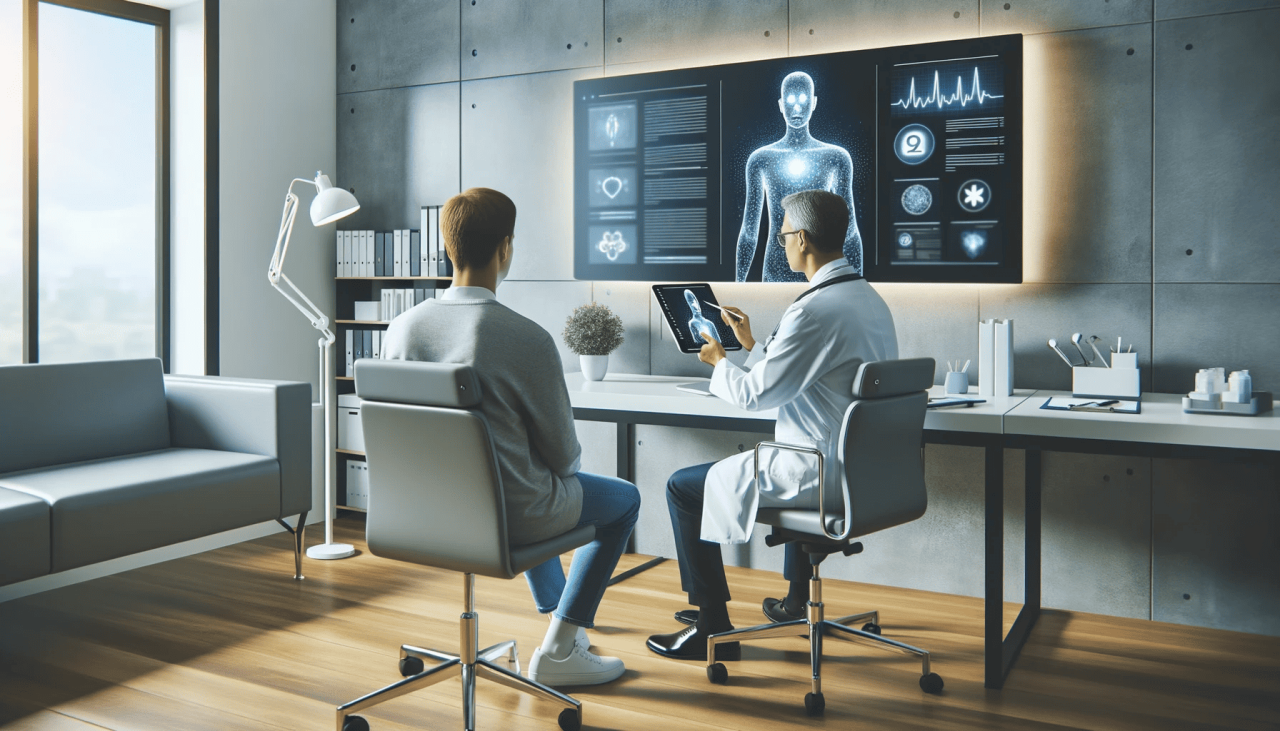
Source: caryfy.ai
The pharmaceutical industry is undergoing a transformative shift, driven largely by the integration of artificial intelligence (AI). AI’s ability to analyze vast datasets, identify patterns, and predict outcomes is dramatically accelerating drug discovery and development, reducing costs, and potentially leading to faster approvals of life-saving medications. This process, once painstakingly slow and reliant on trial-and-error, is now being streamlined through the power of sophisticated algorithms.AI algorithms are revolutionizing various stages of the drug development pipeline.
From identifying promising drug candidates among millions of molecules to optimizing clinical trial design and predicting patient responses, AI is proving invaluable. This efficiency translates to shorter development timelines, reduced research and development costs, and ultimately, faster access to new treatments for patients.
So, I’ve been diving deep into the work of 8 experts on leveraging AI in healthcare – it’s amazing! But all that screen time gave me a nasty case of carpal tunnel. Luckily, I found some helpful advice on how to manage it, check out these ways to treat carpal tunnel syndrome without surgery before resorting to surgery.
Now, back to those brilliant AI minds and their healthcare innovations!
AI in Identifying Drug Candidates, 8 experts on leveraging ai in healthcare
AI algorithms, particularly machine learning models, excel at sifting through massive datasets of molecular structures, biological activity, and other relevant information to identify potential drug candidates. These algorithms can predict the likelihood of a molecule binding to a specific target, its efficacy, and its potential side effects, significantly narrowing down the field of possibilities and focusing research efforts on the most promising compounds.
This approach reduces the need for extensive and expensive laboratory testing in the early stages of drug discovery. For example, Atomwise uses AI to screen millions of molecules for potential drug candidates, significantly reducing the time and cost associated with traditional high-throughput screening.
AI in Optimizing Clinical Trials
Clinical trials, a crucial but often lengthy and costly phase of drug development, are also benefiting from AI’s capabilities. AI can be used to predict which patients are most likely to respond positively to a given drug, allowing for more targeted recruitment of trial participants and a reduction in the number of participants needed. AI can also help optimize trial design, reducing the duration and cost of the process.
Eight experts highlighted AI’s potential in early disease detection, a crucial step given recent events. Reading about Monali Thakur’s hospitalization after struggling to breathe, as detailed in this article monali thakur hospitalised after struggling to breathe how to prevent respiratory diseases , really drives home the importance of proactive healthcare. AI could revolutionize preventative care, potentially helping identify and address respiratory issues before they become critical, furthering the work of those eight AI experts in healthcare.
For instance, AI algorithms can analyze patient data to identify potential biomarkers that predict treatment response, enabling the development of more efficient and effective clinical trials. This improved efficiency can translate to faster approval of new drugs and quicker access for patients in need.
Examples of AI-Driven Drug Development
Several drugs currently in development or already approved have leveraged AI throughout their development lifecycle. For example, Exscientia has used its AI platform to design and optimize molecules for various therapeutic areas, leading to several drug candidates entering clinical trials. Another example is Atomwise, which has used AI to identify potential treatments for Ebola and other diseases. While many examples remain confidential due to the competitive nature of the pharmaceutical industry, the increasing number of AI-driven drug discovery collaborations and publications points to a rapidly expanding role for AI in this critical field.
The success stories of these companies highlight the potential of AI to significantly impact the speed and efficiency of bringing new life-saving drugs to market.
AI and Personalized Medicine
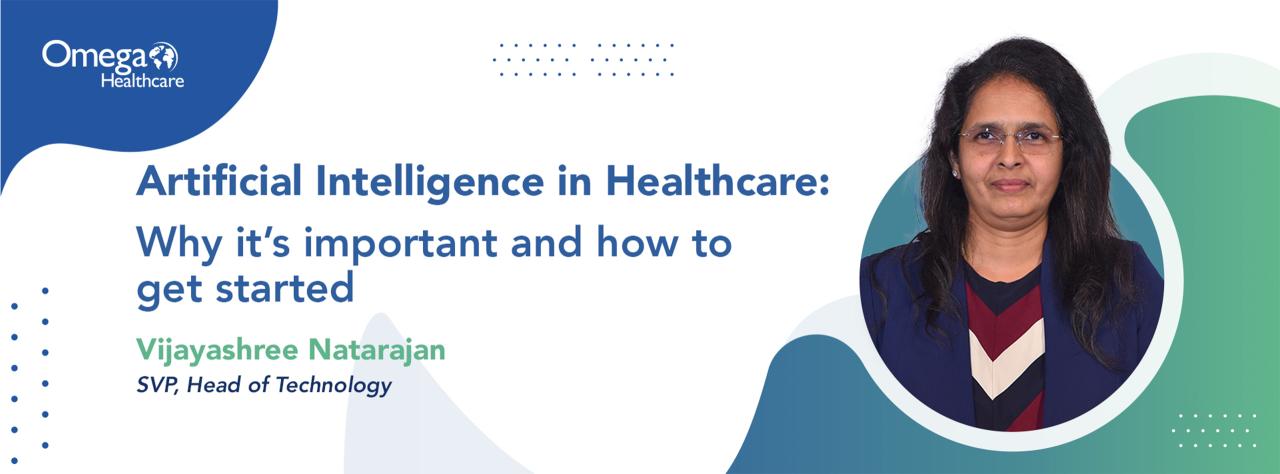
Source: omegahms.com
AI is revolutionizing healthcare by enabling personalized medicine, a transformative approach that tailors treatments to individual patients based on their unique genetic makeup, lifestyle, and medical history. This shift from a “one-size-fits-all” approach to a more precise and effective strategy is driven by AI’s ability to analyze vast amounts of complex data, identify patterns, and predict individual responses to treatments.
This ultimately leads to improved patient outcomes and a more efficient healthcare system.AI’s ability to analyze patient data, including genomic information, medical records, lifestyle factors, and even wearable sensor data, allows for the prediction of treatment outcomes with greater accuracy than traditional methods. This predictive capability allows healthcare professionals to make more informed decisions about treatment plans, minimizing risks and maximizing benefits for each patient.
AI-Driven Treatment Tailoring
AI algorithms can analyze a patient’s unique characteristics to identify the most effective treatment strategy. For example, in oncology, AI can analyze a patient’s tumor’s genomic profile to predict its response to different chemotherapies, allowing oncologists to select the most effective treatment with minimal side effects. Similarly, in cardiology, AI can analyze a patient’s risk factors to predict the likelihood of a heart attack, enabling proactive interventions to prevent adverse events.
This targeted approach significantly improves treatment efficacy and reduces the likelihood of adverse reactions.
AI in Predicting Treatment Outcomes
Predicting treatment outcomes is a critical aspect of personalized medicine. AI models, trained on massive datasets of patient information and treatment responses, can predict the probability of success or failure for various treatments. For instance, in diabetes management, AI can analyze a patient’s blood glucose levels, lifestyle, and genetic predisposition to predict the likelihood of developing complications like retinopathy or neuropathy.
This predictive capability allows for early interventions and personalized management plans to mitigate the risk of these complications. A real-world example is the use of AI in predicting patient response to immunotherapy for cancer, allowing clinicians to select patients most likely to benefit from this expensive and potentially toxic treatment.
Improving Patient Adherence Through AI
Patient adherence to treatment plans is crucial for successful outcomes. AI can play a significant role in improving adherence by personalizing treatment plans and providing tailored support. AI-powered mobile applications can send reminders, track progress, and provide personalized feedback, keeping patients engaged and motivated. For example, an AI-powered app for managing hypertension could track a patient’s blood pressure readings, provide personalized advice on lifestyle modifications, and send timely reminders to take medication.
This personalized support significantly improves patient adherence and contributes to better health outcomes. Studies have shown a marked improvement in adherence rates when AI-powered tools are integrated into treatment plans.
AI in Healthcare Operations and Management
AI is rapidly transforming healthcare operations and management, offering significant potential to improve efficiency, reduce costs, and enhance patient care. By automating tasks, analyzing vast datasets, and predicting future needs, AI is streamlining workflows and optimizing resource allocation across various healthcare settings. This leads to better decision-making, improved patient outcomes, and a more sustainable healthcare system.AI’s impact on healthcare operations and management is multifaceted, affecting everything from scheduling and staffing to inventory management and risk prediction.
The ability of AI to process and interpret large volumes of data allows for a level of optimization previously unattainable through traditional methods. This section will explore some key applications and benefits of AI in this crucial area.
Ways AI Improves Healthcare Operations and Management
AI significantly enhances healthcare operations and management through several key methods. These improvements contribute to a more efficient, cost-effective, and patient-centric healthcare system.
- Predictive Analytics for Resource Allocation: AI algorithms analyze historical data (patient admissions, resource utilization, etc.) to predict future demand for beds, staff, and supplies, enabling proactive resource allocation and minimizing shortages.
- Automated Scheduling and Appointment Management: AI-powered systems optimize appointment scheduling, reducing wait times and improving patient flow. This includes intelligent scheduling algorithms that consider patient preferences and provider availability.
- Streamlined Administrative Tasks: AI automates administrative tasks like claims processing, medical record management, and billing, freeing up staff for more patient-focused activities.
- Improved Inventory Management: AI-driven systems predict supply needs based on consumption patterns and demand forecasts, minimizing waste and ensuring timely availability of essential medical supplies.
- Enhanced Risk Management: AI algorithms identify patients at high risk of readmission or complications, allowing for proactive interventions and improved patient outcomes.
- Real-time Monitoring and Alert Systems: AI-powered systems monitor patient data in real-time, alerting staff to potential issues such as deteriorating vital signs or medication errors.
Examples of AI-Powered Tools for Optimizing Hospital Workflows
Several AI-powered tools are already being used to optimize hospital workflows and resource allocation. These tools demonstrate the practical applications of AI in improving efficiency and reducing costs within healthcare settings.
- AI-driven bed management systems: These systems predict patient flow and bed availability, optimizing bed allocation and reducing wait times for admission.
- Intelligent staffing solutions: AI algorithms analyze historical data and projected patient volume to optimize staffing levels, ensuring adequate coverage while minimizing labor costs.
- Automated medication dispensing systems: These systems reduce medication errors and improve efficiency by automating the dispensing process.
- Robotic process automation (RPA) for administrative tasks: RPA tools automate repetitive administrative tasks, freeing up staff time and reducing errors.
AI’s Potential to Reduce Healthcare Costs and Improve Efficiency
The implementation of AI in healthcare operations and management has the potential to significantly reduce costs and improve efficiency across the healthcare system. This is achieved through automation, optimization, and predictive analytics.
For example, by predicting patient flow and resource needs, AI can prevent costly shortages and inefficiencies. Automated administrative tasks reduce labor costs and improve accuracy. Early identification of high-risk patients through predictive analytics allows for proactive interventions, potentially reducing costly readmissions and complications. Furthermore, optimized inventory management minimizes waste and ensures timely availability of essential supplies, resulting in cost savings.
While initial investment in AI technologies may be significant, the long-term cost savings and efficiency gains are expected to outweigh the initial investment, ultimately leading to a more sustainable and affordable healthcare system. Real-world examples of hospitals implementing AI solutions are showing significant improvements in efficiency and cost reduction, demonstrating the potential for widespread adoption and positive impact.
Ethical Considerations and Challenges: 8 Experts On Leveraging Ai In Healthcare
The integration of artificial intelligence (AI) into healthcare presents immense opportunities, but also raises significant ethical concerns that must be addressed proactively. The potential benefits of AI in improving diagnostics, treatment, and patient care are undeniable, yet the technology’s inherent complexities introduce challenges related to data privacy, algorithmic bias, and regulatory compliance. Addressing these ethical dilemmas is crucial for ensuring responsible and equitable AI implementation in the healthcare sector.The rapid advancement of AI in healthcare necessitates a thorough examination of the ethical implications.
Failure to do so could lead to unintended consequences, eroding public trust and hindering the widespread adoption of potentially life-saving technologies.
Data Privacy and Security
Protecting patient data is paramount in healthcare. AI algorithms rely on vast amounts of sensitive patient information, including medical records, genetic data, and lifestyle details. Unauthorized access or breaches can have devastating consequences, leading to identity theft, discrimination, and reputational damage. Strong data encryption, anonymization techniques, and robust access control mechanisms are essential to mitigate these risks. Furthermore, compliance with regulations like HIPAA in the US and GDPR in Europe is non-negotiable.
A robust framework for data governance, including clear data usage policies and transparent consent processes, is necessary to build and maintain patient trust. For example, differential privacy techniques can add noise to datasets to protect individual identities while still allowing for useful aggregate analysis.
Algorithmic Bias and Fairness
AI algorithms are trained on data, and if that data reflects existing societal biases, the algorithms will perpetuate and even amplify those biases. This can lead to disparities in healthcare access and quality, potentially disadvantaging certain demographic groups. For instance, an AI diagnostic tool trained primarily on data from one ethnic group might be less accurate when applied to patients from other groups.
Mitigating algorithmic bias requires careful data curation, ensuring representative datasets that encompass diverse populations. Regular audits of algorithms for bias and fairness are also critical, along with the development of techniques to detect and correct bias in existing models. Transparency in algorithmic decision-making is also crucial, allowing for scrutiny and accountability.
Regulatory Compliance and Accountability
The rapid evolution of AI technology presents a challenge for regulators, who must establish clear guidelines and standards to ensure safety and ethical use. Uncertainty surrounding liability in cases of AI-related medical errors also needs to be addressed. Clear lines of accountability must be established, defining the roles and responsibilities of developers, healthcare providers, and regulatory bodies. A collaborative approach involving stakeholders from across the healthcare ecosystem is necessary to develop effective regulatory frameworks that encourage innovation while protecting patients’ rights and safety.
This could involve establishing independent review boards to assess the safety and ethical implications of new AI applications before deployment. Furthermore, standardized testing and validation protocols for AI medical devices are essential to ensure their reliability and accuracy.
The Future of AI in Healthcare
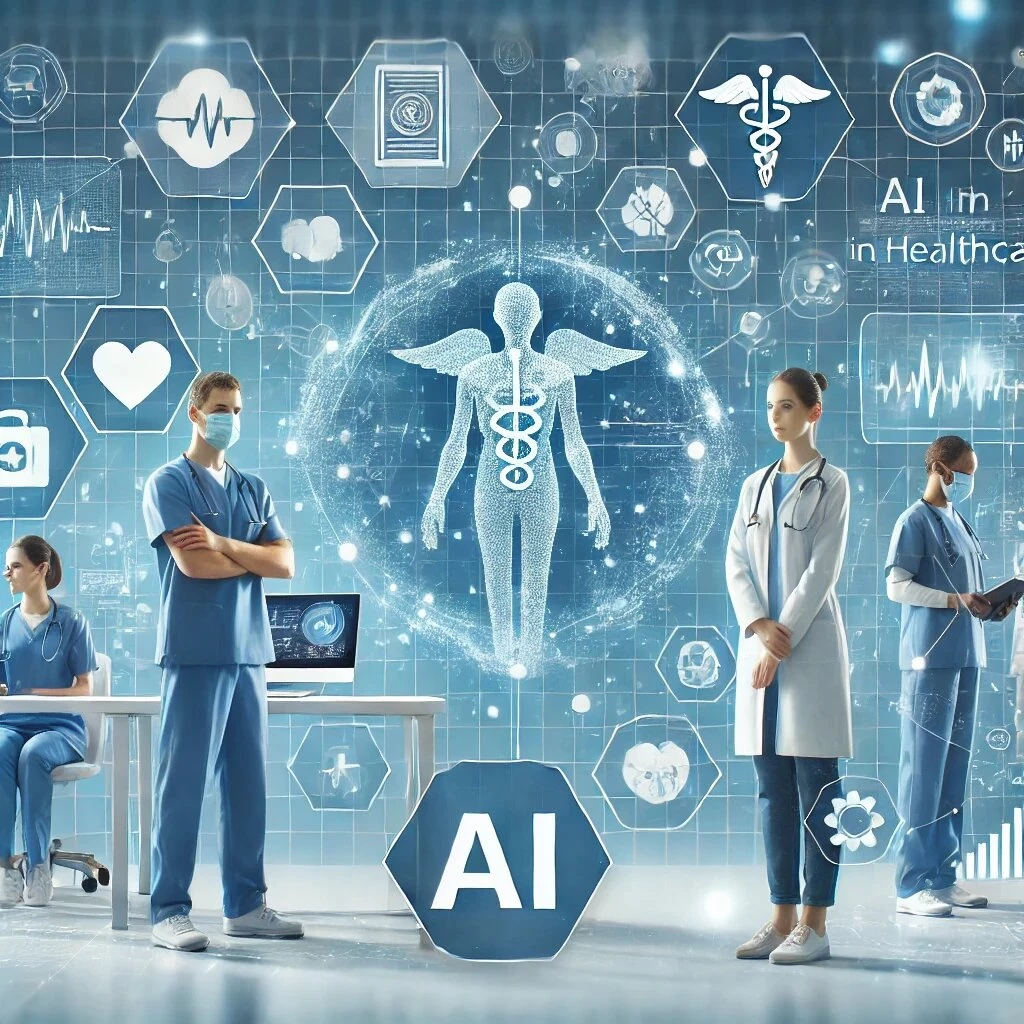
Source: iqpc.com
The integration of artificial intelligence (AI) into healthcare is no longer a futuristic fantasy; it’s rapidly becoming the present. The potential for AI to revolutionize healthcare delivery, research, and patient outcomes is immense, promising a future where diagnosis is faster, treatments are more precise, and healthcare is more accessible and affordable. This section explores the exciting possibilities and emerging trends shaping the future of AI in healthcare.AI’s transformative power stems from its ability to analyze vast amounts of data, identify patterns invisible to the human eye, and make predictions with remarkable accuracy.
This capability is poised to revolutionize various aspects of healthcare, from streamlining administrative tasks to developing personalized therapies and accelerating drug discovery. The convergence of AI with other emerging technologies, such as the Internet of Medical Things (IoMT) and blockchain, further amplifies its potential.
AI-Driven Precision Medicine
The future of medicine lies in personalization. AI algorithms can analyze patient data—genomics, lifestyle, medical history, and real-time health data from wearables—to create highly individualized treatment plans. For example, AI can predict a patient’s response to specific drugs, minimizing adverse effects and maximizing efficacy. This approach moves away from the “one-size-fits-all” model towards treatments tailored to individual needs, leading to better outcomes and improved quality of life.
We can anticipate a future where AI-powered platforms routinely guide oncologists in selecting the most effective chemotherapy regimen for each cancer patient based on their unique tumor profile and genetic makeup.
Advancements in Medical Imaging and Diagnostics
AI is rapidly enhancing medical imaging analysis. Algorithms can detect subtle anomalies in medical images—X-rays, CT scans, MRIs—often missed by human eyes, leading to earlier and more accurate diagnoses. For instance, AI-powered systems are being developed to detect cancerous lesions in mammograms with greater sensitivity and specificity than human radiologists, potentially leading to earlier intervention and improved survival rates.
Further advancements will involve integrating AI directly into imaging devices, providing real-time diagnostic support during procedures.
The Rise of AI-Powered Robotic Surgery
Robotic surgery assisted by AI is already transforming surgical procedures. AI algorithms can enhance the precision and dexterity of robotic systems, enabling minimally invasive surgeries with smaller incisions, reduced trauma, and faster recovery times. AI-powered robots can also assist surgeons during complex procedures by providing real-time feedback and guidance, improving surgical outcomes and reducing the risk of complications. The future will likely see a wider adoption of AI-assisted robotic surgery across various specialties, leading to improved patient care and reduced healthcare costs.
Predictions for the Next 5-10 Years
Within the next 5-10 years, we can expect widespread adoption of AI-powered diagnostic tools leading to faster and more accurate diagnoses across various medical specialties. Personalized medicine driven by AI will become more prevalent, with tailored treatments becoming the standard of care for many diseases. AI-powered robotic surgery will become more sophisticated and widely available, resulting in improved surgical outcomes and reduced healthcare costs.
We can also anticipate increased use of AI in managing chronic diseases, improving patient adherence to treatment plans, and reducing hospital readmissions. Furthermore, the development of AI-driven drug discovery platforms will accelerate the development of new and more effective therapies. For example, companies like Atomwise are already using AI to identify potential drug candidates, significantly shortening the drug discovery process.
This will lead to faster availability of life-saving treatments for various diseases.
Closure
The future of healthcare is undeniably intertwined with the advancements in artificial intelligence. The eight experts highlighted here represent just a fraction of the brilliant minds driving this revolution. Their work underscores the immense potential of AI to improve patient outcomes, optimize healthcare systems, and ultimately, save lives. As AI continues to evolve, we can expect even more groundbreaking innovations to emerge, promising a healthier and more equitable future for all.
Stay tuned for more updates on this rapidly evolving field!
Commonly Asked Questions
What are the biggest challenges in implementing AI in healthcare?
Major challenges include data privacy concerns, ensuring algorithmic fairness to avoid bias, navigating complex regulatory landscapes, and integrating AI seamlessly into existing healthcare workflows.
How can AI improve patient outcomes?
AI can improve outcomes through earlier and more accurate diagnoses, personalized treatment plans, better prediction of treatment success, and improved monitoring of patient health.
What is the role of human doctors in an AI-driven healthcare system?
Doctors remain crucial. AI acts as a powerful tool to assist doctors, enhancing their capabilities, not replacing them. Human expertise and judgment are still vital for ethical considerations and complex cases.
What are some ethical concerns surrounding AI in healthcare?
Ethical concerns include potential biases in algorithms leading to unequal access to care, issues of data privacy and security, and the need for transparency in AI decision-making processes.