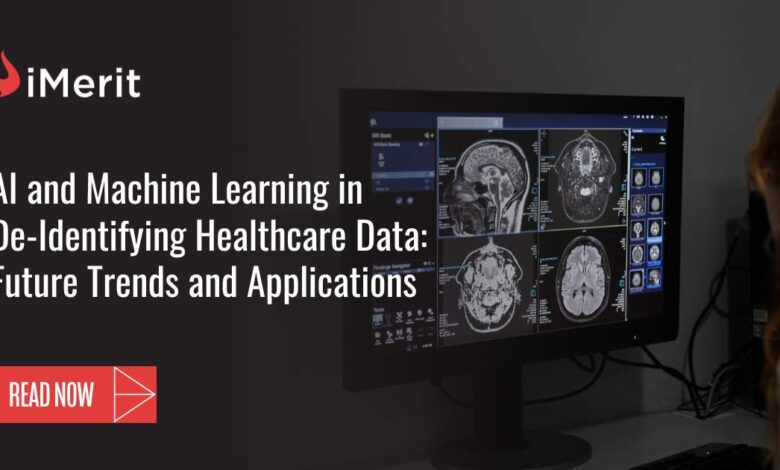
AI Machine Learning Hot Topics in Health IT & Stoltenberg Consulting
Ai machine learning hot topics health it stoltenberg consulting – AI Machine Learning: Hot Topics in Health IT & Stoltenberg Consulting – that’s a mouthful, right? But it’s also the future of healthcare. We’re diving deep into the exciting (and sometimes scary!) world of artificial intelligence and its impact on the medical field. From groundbreaking applications already transforming patient care to the ethical dilemmas we’ll need to navigate, this post explores the cutting edge of healthcare innovation, and how Stoltenberg Consulting is playing a key role.
We’ll cover everything from the latest machine learning algorithms to the critical issues of data security and the potential to revolutionize healthcare access and affordability.
This isn’t just another tech blog post; it’s a look at how AI is poised to reshape how we approach health and wellness. We’ll explore real-world examples, discuss potential pitfalls, and examine the crucial role of expert consulting firms like Stoltenberg in guiding this transformative technology.
AI in Healthcare
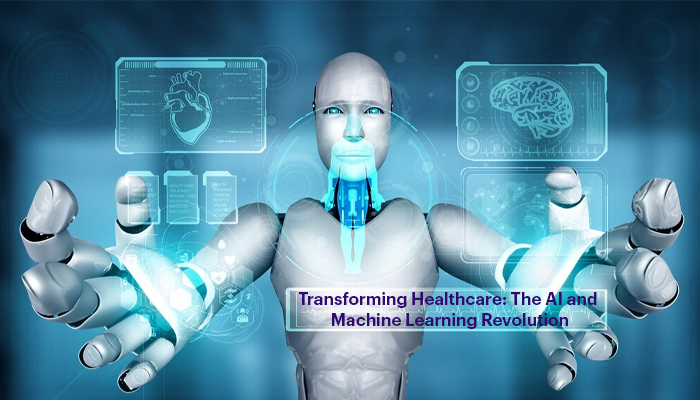
Source: healthcarebloggers.com
Artificial intelligence is rapidly transforming the healthcare landscape, offering unprecedented opportunities to improve patient care, streamline operations, and accelerate medical research. From diagnosis and treatment to drug discovery and administrative tasks, AI’s influence is becoming increasingly pervasive, promising a future where healthcare is more efficient, effective, and personalized.
Current Applications of AI in Healthcare
AI is already making a tangible impact across various healthcare sectors. Its applications are diverse and rapidly evolving, driven by the increasing availability of large datasets and advancements in machine learning algorithms. For example, AI-powered diagnostic tools are assisting radiologists in detecting cancerous tumors with greater accuracy and speed than ever before. Similarly, AI algorithms are being used to analyze patient data to predict potential health risks and personalize treatment plans.
AI-Driven Healthcare Advancements: 2024-2029
Looking ahead to the next 5-10 years, the potential for AI in healthcare is truly transformative. We can expect significant advancements in areas such as personalized medicine, drug discovery, and robotic surgery. The integration of AI with wearable sensors and other connected devices will lead to more proactive and preventative healthcare strategies. Improved diagnostic accuracy, reduced medical errors, and accelerated drug development are just some of the anticipated benefits.
Application | Current Status | Future Potential | Stoltenberg Consulting Relevance |
---|---|---|---|
Medical Imaging Analysis | Assisting radiologists in detecting anomalies; limited autonomous diagnosis. | Fully autonomous diagnosis; real-time image analysis during procedures; predictive modeling of disease progression. | Consulting on AI implementation strategies, risk mitigation, and regulatory compliance. |
Disease Prediction and Prevention | Risk stratification based on existing data; limited personalized prevention strategies. | Predictive modeling for personalized preventative care; early detection of diseases through wearable sensor integration; proactive interventions. | Assisting healthcare providers in adopting predictive analytics for improved patient outcomes. |
Drug Discovery and Development | Accelerating drug screening and identification of potential drug candidates. | AI-driven design of novel drugs; personalized medicine based on individual genetic profiles; significantly reduced drug development timelines. | Supporting pharmaceutical companies in leveraging AI for faster and more efficient drug discovery. |
Robotic Surgery | Assisting surgeons with precision and minimally invasive procedures. | Autonomous surgical robots performing complex procedures with increased precision and safety; remote surgery capabilities. | Advising on the integration of robotic surgery systems and the associated technological and regulatory challenges. |
Ethical Considerations in AI Healthcare
The widespread adoption of AI in healthcare necessitates careful consideration of ethical implications. Concerns include data privacy and security, algorithmic bias leading to disparities in care, and the potential displacement of healthcare professionals. Ensuring transparency, accountability, and fairness in the development and deployment of AI systems is crucial to maintain public trust and ensure equitable access to healthcare.
For instance, algorithms trained on biased data may perpetuate existing health inequalities, leading to misdiagnosis or inappropriate treatment for certain patient populations. Robust regulatory frameworks and ethical guidelines are essential to mitigate these risks.
Machine Learning Algorithms in Health IT
The application of machine learning (ML) is rapidly transforming healthcare IT, improving both patient care and operational efficiency. Several algorithms are particularly well-suited to the complexities of medical data, offering unique strengths and weaknesses depending on the specific application. Understanding these algorithms is crucial for leveraging their potential to revolutionize healthcare.
Comparison of Three Machine Learning Algorithms in Health IT
Three commonly used machine learning algorithms in health IT are linear regression, support vector machines (SVMs), and neural networks. Each offers distinct advantages and disadvantages when applied to healthcare data analysis and prediction.
Algorithm | Strengths | Weaknesses | Healthcare Applications |
---|---|---|---|
Linear Regression | Simple to understand and implement; provides interpretable results; computationally efficient. | Assumes a linear relationship between variables; sensitive to outliers; may not capture complex relationships. | Predicting patient length of stay based on demographics and medical history; forecasting hospital resource needs; analyzing the relationship between risk factors and disease prevalence. |
Support Vector Machines (SVMs) | Effective in high-dimensional spaces; relatively memory efficient; versatile with different kernel functions. | Can be computationally expensive for very large datasets; the choice of kernel function can significantly impact performance; less interpretable than linear regression. | Classifying medical images (e.g., X-rays, CT scans) to detect diseases; predicting patient risk of readmission; identifying patients likely to benefit from specific treatments. |
Neural Networks (Deep Learning) | Can model highly complex relationships; excellent performance on large datasets; adaptable to various data types (images, text, etc.). | Computationally intensive; requires large amounts of data for training; can be difficult to interpret and debug (“black box” problem); prone to overfitting if not carefully tuned. | Image analysis for disease detection (e.g., skin cancer, diabetic retinopathy); natural language processing for analyzing patient records; predicting patient response to treatment; drug discovery and development. |
Examples of Algorithm Applications Improving Patient Outcomes and Operational Efficiency
These algorithms are not used in isolation; rather, they contribute to a broader ecosystem of improvements within health IT systems.Linear regression models, for instance, can predict the likelihood of a patient developing a specific complication post-surgery, allowing for proactive intervention and improved patient outcomes. Similarly, SVMs can help prioritize patients for urgent care based on the severity of their condition as assessed from electronic health records.
Deep learning models can analyze medical images to detect early signs of diseases like cancer, leading to earlier diagnosis and treatment, significantly improving patient survival rates. From an operational perspective, these algorithms can optimize staffing levels, predict equipment needs, and improve resource allocation, leading to cost savings and increased efficiency.
Hypothetical Scenario: Predicting Hospital Readmissions using SVMs
Imagine a large hospital system struggling with high rates of readmissions for patients with congestive heart failure (CHF). An SVM model could be trained on a dataset containing patient demographics, medical history (including previous hospitalizations, medication history, and diagnostic test results), and lifestyle factors. The model would learn to identify patterns and risk factors associated with readmission within 30 days of discharge.
This predictive model could then be used to flag patients at high risk of readmission, allowing healthcare providers to proactively intervene with additional support, such as home healthcare visits, medication adherence programs, and closer monitoring. This targeted intervention could significantly reduce readmission rates, improving patient outcomes and reducing healthcare costs. The SVM’s ability to handle high-dimensional data and identify complex relationships between variables makes it a suitable choice for this task.
The model’s performance would be evaluated using metrics like accuracy, precision, and recall, ensuring its reliability and effectiveness in guiding clinical decision-making.
The Role of Stoltenberg Consulting in AI and Healthcare
Stoltenberg Consulting plays a significant role in the burgeoning field of AI in healthcare, acting as a bridge between cutting-edge technology and the complex regulatory landscape of the medical industry. Their expertise lies in helping healthcare organizations effectively integrate AI solutions, ensuring both technological efficacy and regulatory compliance. This involves a multifaceted approach encompassing strategic planning, technological implementation, and navigating the intricate web of healthcare regulations.Stoltenberg Consulting’s contributions extend beyond simple implementation; they focus on creating sustainable, long-term AI strategies for their clients.
This involves understanding the unique needs of each healthcare provider and tailoring AI solutions to meet specific challenges and opportunities.
Stoltenberg Consulting’s AI Projects in Healthcare
Stoltenberg Consulting’s involvement in AI-related healthcare projects is extensive and varied. While specific client details are often confidential due to non-disclosure agreements, publicly available information suggests a focus on projects involving predictive analytics for patient risk stratification. For example, they may have assisted hospitals in developing AI models that predict which patients are at high risk of readmission following discharge, allowing for proactive interventions and improved patient outcomes.
Another potential area of involvement is the application of AI in streamlining administrative tasks, such as automating appointment scheduling or processing insurance claims, leading to increased efficiency and reduced operational costs. These projects often involve collaboration with leading AI technology providers and software developers.
Stoltenberg Consulting’s Expertise in Healthcare AI Regulation
Navigating the regulatory landscape of AI in healthcare is a critical aspect of successful implementation. Stoltenberg Consulting possesses deep expertise in this area, understanding the intricacies of regulations such as HIPAA (Health Insurance Portability and Accountability Act) in the United States and GDPR (General Data Protection Regulation) in Europe, along with other relevant country-specific laws. Their consultants guide clients through the complex processes of data privacy, security, and compliance, ensuring that AI solutions are developed and deployed ethically and legally.
This often involves conducting thorough risk assessments, developing robust data governance frameworks, and preparing for audits to maintain compliance. This proactive approach minimizes potential legal and financial risks associated with non-compliance.
Potential Areas of Future Collaboration
Stoltenberg Consulting’s future collaborations in AI healthcare could focus on several key areas. One promising avenue is the development and implementation of AI-driven diagnostic tools. Partnering with medical device manufacturers and research institutions, Stoltenberg Consulting could help bring innovative diagnostic technologies to market, ensuring they meet both clinical efficacy and regulatory standards. Another potential area is the expansion of AI’s role in personalized medicine.
By collaborating with pharmaceutical companies and genomic research centers, they can facilitate the development of AI-powered tools for tailoring treatments to individual patients based on their genetic makeup and medical history. Finally, enhancing the security and privacy of AI systems in healthcare will remain a critical focus, requiring collaboration with cybersecurity firms and data protection specialists to develop robust and resilient systems.
Hot Topics in AI and Machine Learning
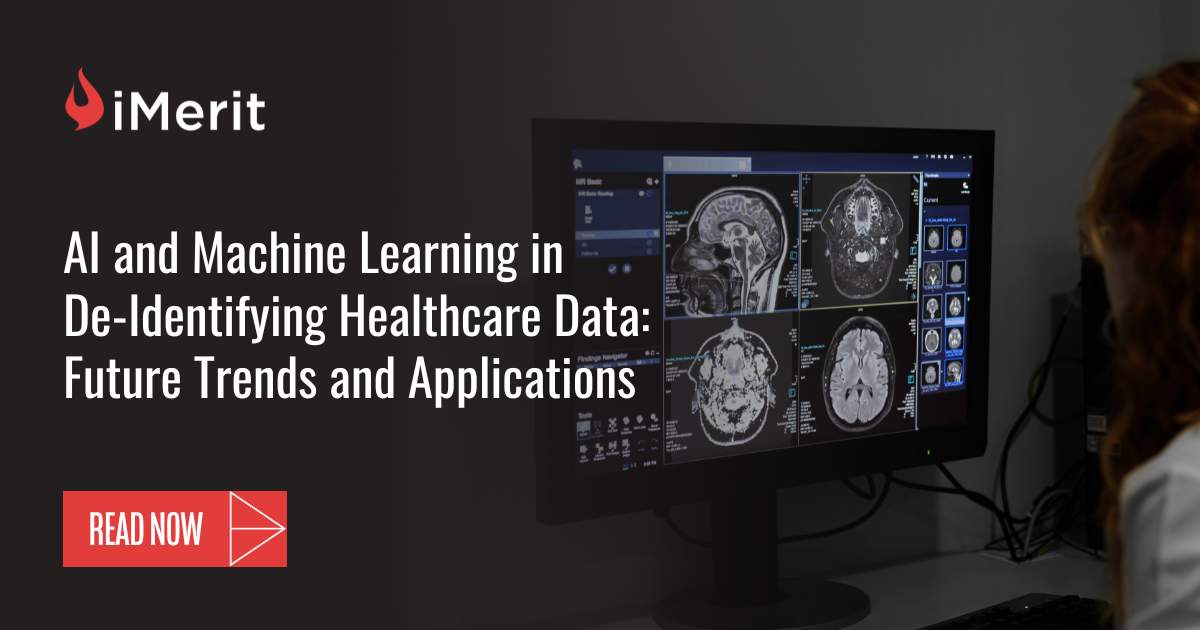
Source: imerit.net
The intersection of artificial intelligence (AI), machine learning (ML), and healthcare is rapidly evolving, sparking intense debate and innovation. Several key areas are dominating discussions, shaping the future of patient care and healthcare delivery. Understanding these hot topics is crucial for anyone involved in or interested in the future of medicine.The following sections delve into three of the most hotly debated topics within the healthcare AI/ML landscape, examining their potential impacts and associated risks.
AI Bias and Fairness in Healthcare
AI algorithms are trained on data, and if that data reflects existing societal biases (e.g., racial, socioeconomic), the resulting AI system will likely perpetuate and even amplify those biases. This is particularly problematic in healthcare, where biased algorithms could lead to misdiagnosis, unequal access to care, and discriminatory treatment. For example, an algorithm trained primarily on data from one demographic group might perform poorly when applied to patients from a different group, leading to inaccurate predictions or recommendations.
This is not just a technical issue; it’s a matter of ethical responsibility and ensuring equitable healthcare access for all.The potential impact of biased AI on healthcare delivery is significant. It can lead to widening health disparities, erode trust in AI-powered systems, and create legal and regulatory challenges. The lack of fairness and transparency in AI algorithms could hinder the adoption of beneficial AI tools, undermining their potential to improve healthcare outcomes.
- Lack of diverse and representative datasets used for training AI models.
- Difficulty in identifying and mitigating bias in complex algorithms.
- Potential for legal challenges and regulatory scrutiny due to discriminatory outcomes.
- Erosion of patient trust in AI-driven healthcare solutions.
- Exacerbation of existing health disparities.
Data Privacy and Security in AI-driven Healthcare
The use of AI in healthcare necessitates the collection and analysis of vast amounts of sensitive patient data. This raises serious concerns about data privacy and security. Breaches can have devastating consequences, leading to identity theft, financial loss, and reputational damage for healthcare providers. Furthermore, the use of patient data for AI development must adhere to strict ethical guidelines and regulations like HIPAA in the United States and GDPR in Europe.
AI machine learning is revolutionizing healthcare, a hot topic in IT consulting, especially with Stoltenberg’s recent work. One fascinating example of this convergence is the expansion of convenient healthcare access, like the partnership described in this article about humana centerwell primary care centers walmart , which showcases how AI-powered tools could improve patient outcomes and streamline operations within these centers.
This highlights the practical applications of AI within the broader healthcare IT landscape.
Balancing the need for data to train and improve AI systems with the imperative to protect patient privacy is a critical challenge.The impact on healthcare delivery is substantial. Data breaches can disrupt services, erode patient trust, and result in significant financial penalties for healthcare organizations. Stringent data protection measures are essential to foster public confidence in AI-powered healthcare and ensure the responsible use of sensitive patient information.
- Risk of data breaches and unauthorized access to sensitive patient information.
- Challenges in ensuring compliance with data privacy regulations (e.g., HIPAA, GDPR).
- Potential for misuse of patient data for purposes beyond healthcare.
- Difficulty in anonymizing and de-identifying patient data effectively.
- Lack of standardized security protocols for AI systems in healthcare.
Explainability and Transparency of AI Algorithms in Healthcare
Many AI algorithms, particularly deep learning models, are often referred to as “black boxes” due to their complexity and opacity. Understandingwhy* an algorithm makes a specific prediction or recommendation can be challenging. This lack of transparency poses significant challenges in healthcare, where clinicians need to understand the reasoning behind AI-driven diagnoses or treatment plans to make informed decisions and maintain patient trust.
The need for explainable AI (XAI) is paramount to ensure accountability, build confidence, and facilitate the responsible integration of AI into clinical workflows.The impact on the future of healthcare is profound. Without explainability, clinicians may be hesitant to adopt AI tools, hindering the potential benefits. Furthermore, a lack of transparency can lead to difficulties in identifying and correcting errors in AI systems, potentially compromising patient safety.
Developing and implementing XAI methods is crucial for building trust and ensuring the safe and effective use of AI in healthcare.
AI, machine learning, and their applications in healthcare are definitely hot topics right now, especially with the rise of Stoltenberg Consulting’s innovative work. The implications for policy are huge, and this makes the news about Robert F. Kennedy Jr. clearing a key hurdle on his path to becoming HHS Secretary, as reported in this article , incredibly relevant.
His views on these technologies could significantly shape the future of healthcare IT and AI development. It will be fascinating to see how this plays out for the future of AI in health.
- Difficulty in interpreting the decision-making processes of complex AI algorithms.
- Lack of trust in AI-driven recommendations due to lack of transparency.
- Challenges in identifying and correcting errors in opaque AI systems.
- Potential for misinterpretations of AI-generated insights by clinicians.
- Increased liability risks for healthcare providers using unexplainable AI systems.
Data Security and Privacy in AI-Driven Healthcare
The integration of artificial intelligence (AI) into healthcare promises revolutionary advancements in diagnostics, treatment, and patient care. However, this progress comes with significant challenges, particularly concerning the security and privacy of sensitive patient data. AI algorithms require vast amounts of data to function effectively, raising concerns about the potential for data breaches, unauthorized access, and misuse of personal health information.
Addressing these concerns is paramount to ensuring the ethical and responsible implementation of AI in healthcare.The use of AI in healthcare presents unique vulnerabilities. Traditional security measures may be insufficient to protect against sophisticated attacks targeting large datasets. Furthermore, the interconnected nature of healthcare systems, involving various devices, networks, and data repositories, creates a complex landscape for security management.
The potential consequences of a data breach in this context are severe, ranging from financial losses and reputational damage to legal penalties and erosion of patient trust.
Data Breach Prevention Strategies
Effective data breach prevention requires a multi-layered approach encompassing technological, procedural, and human elements. Robust security measures must be implemented at every stage of the data lifecycle, from data collection and storage to processing and analysis. This includes employing strong encryption techniques to protect data both in transit and at rest, implementing access control measures to restrict access to authorized personnel only, and regularly conducting security audits and penetration testing to identify and address vulnerabilities.
Employee training on data security best practices is also crucial to prevent human error, a common cause of data breaches.
Data Anonymization and De-identification Techniques
Protecting patient privacy is a critical ethical and legal requirement. Data anonymization and de-identification techniques aim to remove or obscure identifying information from datasets used for AI development and analysis. This involves techniques such as data masking, generalization, and suppression, carefully removing or replacing personally identifiable information (PII) while preserving the data’s utility for AI algorithms. However, it’s important to note that perfect anonymization is challenging, and advanced techniques like re-identification can potentially reveal hidden identities.
Therefore, a thorough risk assessment and careful selection of anonymization techniques are essential.
Secure Data Management and Access Control
A comprehensive framework for secure data management and access control is crucial in AI-driven healthcare. This includes establishing clear data governance policies, defining roles and responsibilities for data access and management, and implementing robust authentication and authorization mechanisms. Data should be stored in secure environments, preferably using cloud-based solutions with robust security features. Regular monitoring and logging of data access activities are also necessary to detect and respond to potential security incidents promptly.
Furthermore, implementing a data loss prevention (DLP) system can help prevent sensitive data from leaving the organization’s control.
Flowchart: Securing and Managing Sensitive Patient Data in an AI-Driven Healthcare Environment
Imagine a flowchart depicting the following steps:
1. Data Collection
Data is collected from various sources (e.g., EHRs, wearables) following strict protocols and consent procedures.
2. Data Anonymization/De-identification
PII is removed or masked using appropriate techniques.
3. Data Storage
Anonymized/de-identified data is stored in a secure, encrypted environment (e.g., cloud storage with access controls).
4. Data Access Control
Access to data is granted only to authorized personnel with specific roles and permissions.
5. Data Processing and Analysis
AI algorithms process the data, adhering to security and privacy protocols.
6. Data Output and Reporting
AI and machine learning are exploding in healthcare IT, and Stoltenberg Consulting is right in the thick of it. We’re seeing huge potential for these technologies to improve efficiency and outcomes, especially with the recent news that the CMS launched a primary care Medicare model ACO, as detailed in this article: cms launches primary care medicare model aco.
This new model could significantly impact how AI is implemented in primary care, creating new opportunities for innovation within the healthcare IT sector and driving further work at Stoltenberg Consulting.
Results are generated and shared securely, respecting patient privacy.
7. Monitoring and Auditing
Regular monitoring and auditing of data access and processing activities are performed to detect anomalies and potential breaches.
8. Incident Response
In case of a security incident, a well-defined incident response plan is activated to mitigate the impact and restore data integrity.
The Impact of AI on Healthcare Costs and Accessibility: Ai Machine Learning Hot Topics Health It Stoltenberg Consulting
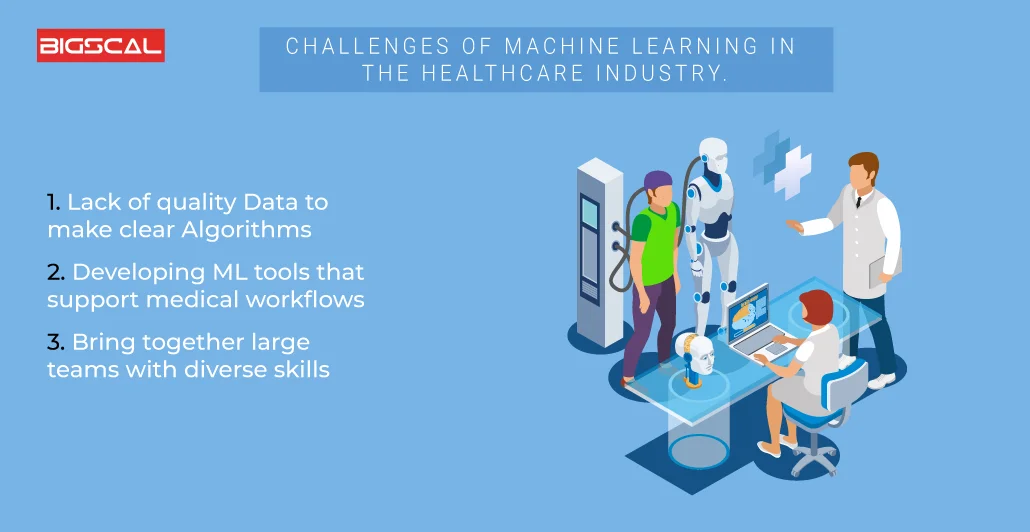
Source: bigscal.com
The integration of artificial intelligence (AI) into healthcare promises a transformative shift, impacting both the cost of care and its accessibility. While the potential benefits are substantial, a nuanced understanding of both the positive and negative consequences is crucial for responsible implementation. AI’s influence on healthcare economics is complex, with the potential to simultaneously reduce expenses and introduce new ones.
Similarly, its ability to expand access to care is not without its challenges.AI’s potential to reduce healthcare costs is significant. This is achieved through increased efficiency, improved diagnostics, and more effective treatment strategies. However, the initial investment in AI technologies, the need for skilled professionals to manage and interpret AI outputs, and potential unforeseen challenges could lead to increased costs in the short term.
AI-Driven Cost Reduction Strategies
AI can streamline administrative tasks, automating processes like appointment scheduling, billing, and insurance claims processing, leading to significant cost savings for healthcare providers. AI-powered diagnostic tools can improve accuracy and speed, reducing the need for expensive and time-consuming tests. Predictive analytics can identify patients at high risk of developing certain conditions, allowing for proactive interventions and preventing costly hospitalizations.
For example, an AI system analyzing patient data might predict a patient’s likelihood of developing heart failure, allowing for early lifestyle changes or medication adjustments, thereby preventing costly emergency room visits and hospital stays. This proactive approach is demonstrably more cost-effective than reactive care.
Expanding Healthcare Access with AI, Ai machine learning hot topics health it stoltenberg consulting
AI has the potential to dramatically improve access to healthcare, particularly in underserved communities. Telemedicine platforms powered by AI can connect patients in remote areas with specialists, overcoming geographical barriers. AI-powered diagnostic tools can be deployed in resource-limited settings, providing accurate diagnoses even where skilled medical professionals are scarce. AI chatbots can provide basic medical advice and triage patients, directing them to appropriate resources based on their needs.
Consider a rural community with limited access to specialists. An AI-powered telemedicine platform could allow patients to consult with cardiologists remotely, receive timely diagnoses, and access appropriate treatment plans, significantly improving their healthcare outcomes and reducing the need for expensive travel to urban centers.
Hypothetical Scenario: AI Optimizing Healthcare Delivery
Imagine a hypothetical scenario in a rural region with limited access to specialists and high healthcare costs. An AI-powered system is implemented, integrating several key features: First, an AI-driven telemedicine platform connects patients with specialists remotely. Second, an AI diagnostic tool helps local healthcare providers make accurate diagnoses using readily available data, minimizing the need for expensive specialist referrals.
Third, an AI-powered system analyzes patient data to predict and manage chronic conditions proactively, preventing costly hospitalizations. This integrated system reduces the financial burden on patients and the healthcare system while simultaneously expanding access to high-quality care. The system’s predictive capabilities allow for proactive interventions, reducing the need for expensive emergency care and hospitalizations. The reduction in unnecessary tests and specialist referrals contributes significantly to cost savings, while the improved access to care ensures better health outcomes for the community.
This scenario demonstrates how AI can be a powerful tool for optimizing healthcare delivery, leading to both cost reduction and improved access.
End of Discussion
The integration of AI and machine learning in healthcare is undeniably transformative, promising incredible advancements in diagnosis, treatment, and accessibility. However, the journey is not without its challenges. Navigating ethical considerations, ensuring data security, and understanding the potential impact on costs and access are all crucial aspects of responsible AI implementation. The future of healthcare is intertwined with AI, and the insights and expertise of firms like Stoltenberg Consulting will be instrumental in shaping a future where technology enhances, rather than hinders, the delivery of quality care for all.
User Queries
What are the biggest risks associated with AI in healthcare?
Bias in algorithms, data breaches leading to privacy violations, and the potential for job displacement are major concerns.
How can Stoltenberg Consulting help my organization implement AI solutions?
They offer expertise in strategy, implementation, regulatory compliance, and risk mitigation related to AI in healthcare.
What is the difference between AI and machine learning in this context?
AI is the broader concept of machines mimicking human intelligence. Machine learning is a subset of AI focusing on systems learning from data without explicit programming.
What role does data privacy play in AI-driven healthcare?
It’s paramount. Robust security measures and adherence to regulations like HIPAA are crucial to protect sensitive patient information.