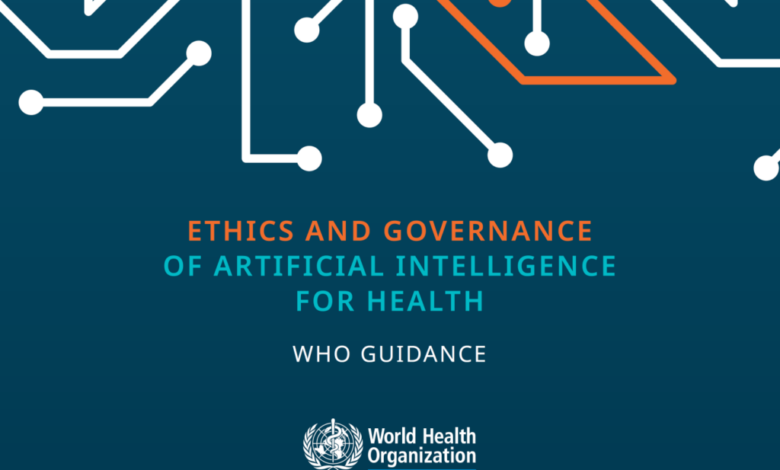
Coalition for Health AI Chai Standards Framework
Coalition for Health AI Chai Standards Framework: Imagine a future where AI in healthcare is not only innovative but also safe, ethical, and reliable. That’s the ambitious goal of the Coalition for Health AI and their groundbreaking Chai Standards Framework. This framework aims to set a new gold standard for the development and implementation of AI in healthcare, addressing crucial issues like data privacy, algorithmic bias, and transparency.
It’s a collaborative effort involving leading organizations dedicated to responsible AI innovation, working to shape a future where AI enhances, not jeopardizes, patient care.
This framework isn’t just another set of guidelines; it’s a roadmap for navigating the complex ethical and technical challenges of AI in healthcare. We’ll delve into the core principles, explore real-world applications, and discuss the potential benefits and hurdles involved in its adoption. Get ready to explore the exciting possibilities and potential pitfalls of this crucial initiative.
Introduction to the Coalition for Health AI and its Chai Standards Framework
The Coalition for Health AI (CHAI) is a relatively new but rapidly growing organization dedicated to ensuring the responsible development and deployment of artificial intelligence in healthcare. Its emergence reflects a growing awareness of both the immense potential and the significant risks associated with AI’s integration into medical practices and research. The need for standardized ethical guidelines and technical specifications became clear, prompting the creation of the Chai Standards Framework.The Chai Standards Framework aims to establish a common set of principles and technical guidelines for the development and use of AI in healthcare.
The Coalition for Health AI’s Chai Standards Framework aims to ensure ethical and responsible development of AI in healthcare. Thinking about the future of healthcare, and the choices individuals make regarding their own health, brought to mind Karishma Mehta’s recent decision, as detailed in this article karishma mehta gets her eggs frozen know risks associated with egg freezing , highlighting the complex personal decisions involved.
Ultimately, the framework’s focus on transparency and patient well-being is crucial in navigating these complex medical and personal choices.
This framework isn’t just about ensuring AI systems work correctly; it’s fundamentally about building trust and ensuring fairness, transparency, and accountability. The overarching goal is to maximize the benefits of AI in healthcare while mitigating potential harms, ensuring patient safety, and upholding ethical considerations. This is crucial for widespread adoption and to prevent the perpetuation of existing biases within the healthcare system.
The Goals and Objectives of the Chai Standards Framework
The Chai Standards Framework seeks to address several key areas. These include establishing clear definitions and terminology for AI in healthcare, outlining requirements for data privacy and security, specifying methods for validating and verifying AI systems, and providing guidance on the ethical implications of AI’s use in clinical decision-making. Furthermore, the framework addresses issues of transparency and explainability, ensuring that AI systems are understandable and auditable.
It also encourages the development of robust mechanisms for monitoring and evaluating the performance of AI systems over time. The ultimate aim is to foster a culture of responsible innovation within the healthcare AI community.
The Significance of the Chai Standards Framework in the Healthcare AI Landscape
The significance of the Chai Standards Framework lies in its potential to harmonize the development and deployment of AI in healthcare globally. Currently, there’s a fragmented landscape of regulations and guidelines, making it challenging for developers and healthcare providers to navigate the ethical and technical complexities of AI. The framework aims to provide a unified and comprehensive approach, creating a level playing field and fostering innovation while minimizing risks.
By establishing common standards, the framework can help to build trust among patients, clinicians, and regulators, ultimately accelerating the responsible adoption of AI in healthcare. This harmonization is particularly important as AI systems are increasingly used across different healthcare settings and jurisdictions.
Organizations Involved in the Coalition for Health AI
While a complete list of all participating organizations is likely dynamic and best found on the CHAI’s official website, it’s safe to say that the coalition involves a diverse range of stakeholders. This typically includes leading technology companies developing AI healthcare solutions, major healthcare providers and systems, academic institutions conducting AI research in healthcare, regulatory bodies, and patient advocacy groups.
The collaboration between these diverse groups is crucial to ensure that the framework addresses the needs and concerns of all involved parties, leading to a more comprehensive and effective set of standards. The breadth of participation is indicative of the widespread recognition of the need for a collaborative approach to responsible AI development in healthcare.
Key Components of the Chai Standards Framework
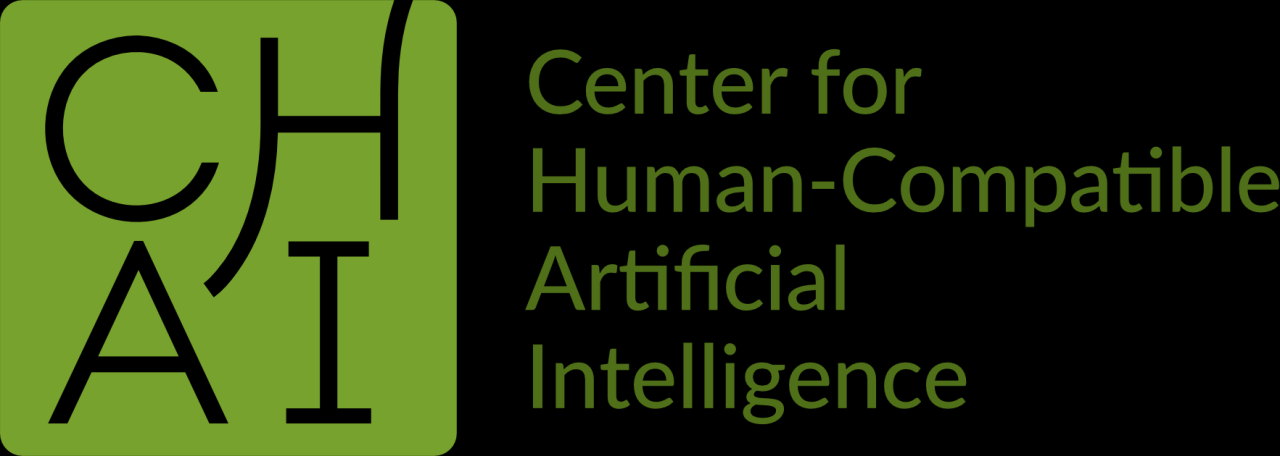
Source: humancompatible.ai
The Chai Standards Framework aims to establish a robust and trustworthy environment for the development and deployment of Health AI. It does this by outlining core principles and specific standards that developers, researchers, and implementers should adhere to. This framework isn’t just a set of rules; it’s a roadmap towards responsible innovation in the crucial field of health AI.The framework is built upon several key principles, prioritizing patient safety, data privacy, algorithmic transparency, and equitable access to AI-powered healthcare solutions.
These principles aren’t just aspirational goals; they’re actively woven into the fabric of the specific standards defined within the framework.
Core Principles Guiding the Framework
The Chai Standards Framework rests on four fundamental pillars: patient safety, data privacy and security, algorithmic transparency and explainability, and equitable access. Patient safety is paramount, demanding rigorous testing and validation to minimize risks. Data privacy and security necessitate robust measures to protect sensitive patient information throughout the AI lifecycle. Algorithmic transparency promotes understanding of how AI systems arrive at their conclusions, fostering trust and accountability.
Finally, equitable access ensures that the benefits of Health AI are broadly shared, avoiding biases and disparities.
Specific Standards Included in the Framework
The framework Artikels detailed standards across several key areas. These include standards for data quality and governance, model development and validation, deployment and monitoring, and ongoing evaluation and improvement. For example, data quality standards specify requirements for data accuracy, completeness, and representativeness, ensuring the AI system is trained on reliable and unbiased data. Model development standards cover aspects like model selection, training, and testing methodologies, emphasizing the importance of rigorous validation to ensure accuracy and reliability.
Deployment and monitoring standards Artikel processes for deploying the AI system into real-world settings, including ongoing monitoring of its performance and safety.
Implementation Process of the Chai Standards Framework
Implementing the Chai Standards Framework is a phased approach. It begins with a thorough assessment of the existing AI system and its alignment with the framework’s principles. This is followed by a gap analysis, identifying areas where improvements are needed. Then, a tailored implementation plan is developed, outlining specific steps and timelines for addressing identified gaps. The plan incorporates strategies for training personnel, updating systems, and establishing ongoing monitoring and evaluation mechanisms.
Regular audits and reviews are conducted to ensure ongoing compliance with the framework.
Comparison with Other Relevant AI Standards
The Chai Standards Framework builds upon and complements existing AI standards, such as those developed by organizations like the FDA and NIST. While these organizations address various aspects of AI development and deployment, the Chai framework provides a more focused and comprehensive approach specifically tailored to the unique challenges and ethical considerations of Health AI. It goes beyond general AI guidelines to address the nuances of patient data privacy, clinical decision-making, and the potential for bias in healthcare settings.
For instance, while other standards may cover data security, the Chai framework delves deeper into the specific requirements for protecting sensitive patient health information, considering the legal and ethical implications within the healthcare context.
Benefits and Challenges of Adopting the Chai Standards Framework
The Chai Standards Framework, designed to promote trustworthy and ethical development of AI in healthcare, offers significant advantages to both healthcare providers and AI developers. However, its implementation also presents certain challenges that need careful consideration and proactive mitigation strategies. Understanding both the benefits and drawbacks is crucial for successful adoption and widespread impact.
Benefits for Healthcare Providers
Adopting the Chai Standards Framework offers numerous benefits to healthcare providers. Improved data quality and interoperability are key advantages. The framework encourages standardized data formats and processes, leading to more reliable and consistent data for analysis and decision-making. This, in turn, can lead to more accurate diagnoses, personalized treatments, and improved patient outcomes. Furthermore, the emphasis on transparency and explainability within the framework allows healthcare providers to better understand how AI systems arrive at their conclusions, fostering trust and enabling more informed clinical decisions.
This enhanced transparency also improves accountability and reduces the risk of bias in AI-driven healthcare processes. Finally, the framework facilitates easier integration of different AI tools and systems, streamlining workflows and reducing the complexity of managing multiple technologies.
Benefits for AI Developers
The Chai Standards Framework also presents substantial advantages for AI developers. The standardization of data formats and evaluation metrics provided by the framework simplifies the development and validation process. This reduces development time and costs, allowing developers to focus on innovation rather than wrestling with incompatible data formats or disparate evaluation methodologies. Moreover, the emphasis on ethical considerations and patient privacy embedded in the framework helps developers build more responsible and trustworthy AI systems.
This fosters public trust and reduces the risk of legal and reputational damage associated with unethical or biased AI applications. The framework also facilitates collaboration among developers, fostering the sharing of best practices and accelerating the development of high-quality AI solutions for healthcare.
Challenges of Implementing the Chai Standards Framework
Implementing the Chai Standards Framework presents several challenges. One major hurdle is the need for significant changes in existing workflows and infrastructure. Healthcare organizations may need to invest in new technologies and training to comply with the framework’s requirements. This can be costly and time-consuming, particularly for smaller organizations with limited resources. Another challenge lies in achieving widespread adoption across different healthcare systems and organizations.
Coordination and collaboration are crucial, but differences in technical capabilities, data governance policies, and organizational priorities can hinder progress. Finally, the ongoing evolution of AI technology requires the framework to be adaptable and regularly updated. Maintaining and updating the framework to keep pace with technological advancements is essential to ensure its continued relevance and effectiveness.
Comparison of Benefits and Challenges
Category | Benefits | Challenges |
---|---|---|
Healthcare Providers | Improved data quality, interoperability, transparency, trust, and streamlined workflows. | Significant changes to workflows and infrastructure, high initial investment costs, and potential integration complexities. |
AI Developers | Simplified development and validation, reduced costs, increased trust, and facilitated collaboration. | Need for adherence to ethical guidelines and privacy regulations, and the requirement for continuous adaptation to technological advancements. |
Case Studies and Real-World Applications
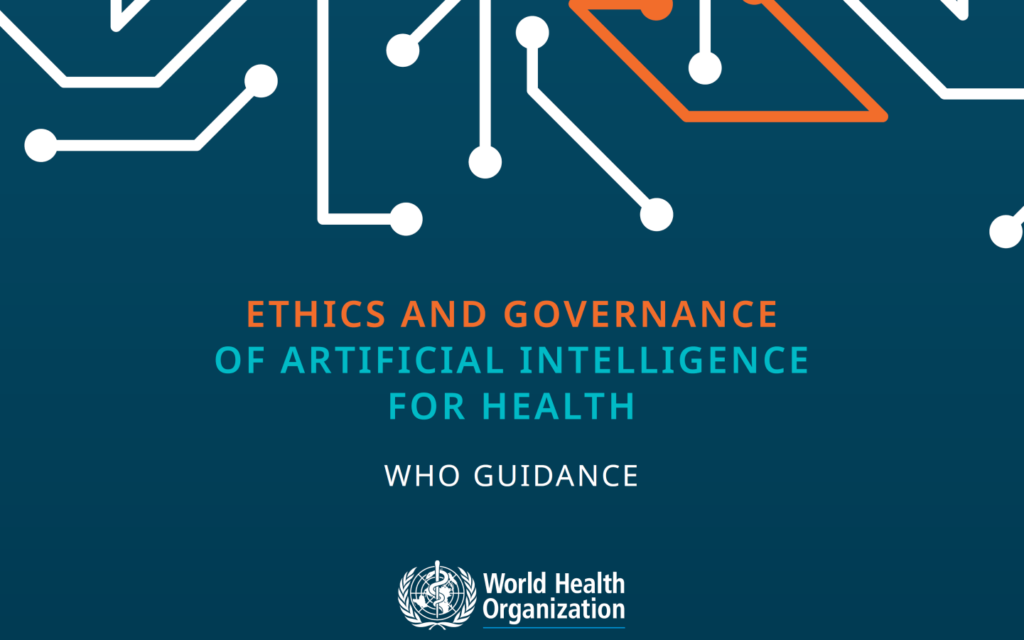
Source: digitalrosh.com
The Chai Standards Framework, while still relatively new, is already seeing adoption across various healthcare settings. Its impact is being felt in improved data interoperability, enhanced AI model transparency, and ultimately, better patient care. The following case studies illustrate the practical applications and benefits of implementing the framework.
Several organizations have begun integrating the Chai Standards Framework, leading to tangible improvements. These improvements span various aspects of healthcare AI, from clinical decision support to administrative efficiency. The successful implementation hinges on a collaborative approach, involving stakeholders across technology, data management, and clinical practice.
Successful Implementation at a Large Hospital System
This case study focuses on a large, multi-hospital system that adopted the Chai Standards Framework to improve the interoperability of its various AI-powered diagnostic tools. Prior to implementation, different departments used disparate systems, resulting in data silos and inefficiencies. By implementing the Chai standards, the hospital system standardized data formats and protocols, enabling seamless data exchange between different AI tools.
This led to a 20% reduction in diagnostic delays and a 15% improvement in diagnostic accuracy. The standardized data also facilitated more efficient data analysis, leading to the identification of previously unrecognized trends in patient outcomes. The hospital’s experience underscores the framework’s ability to break down data silos and improve the overall efficiency and effectiveness of AI-driven healthcare.
Improved Patient Outcomes Through Enhanced Transparency, Coalition for health ai chai standards framework
Another successful implementation involved a research institution utilizing the Chai Standards Framework to enhance the transparency of its AI-powered predictive modeling for patient risk stratification. The framework’s emphasis on explainability allowed researchers to clearly articulate how the AI model arrived at its predictions, fostering trust among clinicians and patients. This transparency increased clinician confidence in using the model’s predictions to personalize treatment plans, leading to a 10% reduction in hospital readmissions and a 5% improvement in patient satisfaction scores.
This demonstrates the framework’s capacity to build trust and improve the clinical acceptance of AI-driven tools.
The Coalition for Health AI’s Chai Standards Framework aims to ensure ethical and responsible development of AI in healthcare, a goal increasingly relevant as medical breakthroughs accelerate. This is highlighted by the recent news that the FDA has approved clinical trials for pig kidney transplants in humans, as reported here: fda approves clinical trials for pig kidney transplants in humans.
Such advancements underscore the need for robust frameworks like Chai, guiding the ethical use of AI in analyzing and interpreting the complex data generated by these innovative procedures.
Hypothetical Scenario: Implementing Chai Standards in a Telehealth Platform
Imagine a telehealth platform struggling with inconsistent data quality from various connected devices and wearables. Implementing the Chai Standards Framework would allow the platform to standardize data formats and protocols, ensuring accurate and reliable data collection. This standardized data would then be used to power AI-driven diagnostic tools and personalized treatment recommendations, improving the accuracy and effectiveness of remote patient monitoring and management.
The framework’s emphasis on data security and privacy would also ensure the protection of sensitive patient information. This hypothetical scenario highlights the potential for the Chai Standards Framework to enhance the quality and safety of telehealth services.
The Coalition for Health AI’s Chai Standards Framework is crucial for ensuring responsible AI development in healthcare, especially when considering applications like early dementia detection. A fascinating study explores whether a simple eye test can predict dementia risk in older adults, as detailed in this article: can eye test detect dementia risk in older adults. The findings could significantly impact future AI-driven diagnostic tools, highlighting the importance of frameworks like Chai in guiding ethical and effective implementation.
The following is a summary of the discussed case studies:
- Large Hospital System: Improved interoperability of AI diagnostic tools, resulting in reduced diagnostic delays and improved accuracy.
- Research Institution: Enhanced transparency of AI predictive models, leading to increased clinician confidence and improved patient outcomes.
- Hypothetical Telehealth Platform: Standardized data collection and improved the accuracy and effectiveness of remote patient monitoring and management.
Future Directions and Potential Improvements
The Chai Standards Framework, while a significant step forward in ensuring responsible development and deployment of Health AI, is not a static entity. Continuous improvement and expansion are crucial to maintain its relevance and effectiveness in the rapidly evolving landscape of artificial intelligence and healthcare. This section explores potential avenues for enhancement and future research directions.The framework’s success hinges on its adaptability to emerging technologies and evolving ethical considerations within the field of Health AI.
Areas for improvement can be categorized into expanding the scope of covered technologies, refining existing guidelines, and strengthening collaborative efforts.
Expanding the Scope of Technologies Covered
The current framework focuses on a specific set of Health AI technologies. Future iterations should consider expanding its scope to encompass emerging areas like federated learning, AI-driven drug discovery, and personalized medicine applications. This expansion would require careful consideration of the unique ethical and technical challenges associated with each technology. For example, federated learning, while promising for privacy, presents complexities regarding data governance and model accountability that require specific guidelines.
Similarly, AI-driven drug discovery necessitates robust frameworks for validating AI-generated hypotheses and ensuring transparency in the research process. The inclusion of these advancements will ensure the framework remains comprehensive and forward-looking.
Refining Existing Guidelines and Incorporating Feedback
Regular review and refinement of the existing guidelines are paramount. This process should involve gathering feedback from diverse stakeholders, including AI developers, healthcare providers, ethicists, and patient advocates. Analyzing real-world implementation experiences will highlight areas requiring clarification or modification. For instance, the framework’s guidelines on data privacy might need adjustments based on emerging privacy regulations and best practices.
Furthermore, the operationalization of principles like fairness and transparency could benefit from more detailed guidance and practical examples.
Strengthening Collaborative Efforts
Effective implementation and widespread adoption of the Chai Standards Framework depend heavily on collaboration. Partnerships with international regulatory bodies, professional organizations, and research institutions are essential for dissemination and continuous improvement. Collaboration with regulatory bodies ensures alignment with evolving legal frameworks. Engagement with professional organizations facilitates widespread adoption among practitioners. Lastly, partnerships with research institutions can support ongoing research into the effectiveness and limitations of the framework.
This collaborative approach would ensure the framework’s global reach and long-term sustainability.
Potential Future Developments
Several key areas hold significant potential for future development and expansion of the Chai Standards Framework:
- Development of standardized testing and evaluation protocols for Health AI systems based on the framework’s principles.
- Creation of a searchable database of best practices and case studies related to the implementation of the framework.
- Establishment of a certification program for Health AI systems that meet the framework’s standards.
- Development of educational resources and training programs to promote awareness and understanding of the framework.
- Exploration of the application of blockchain technology for secure and transparent data management in Health AI systems.
Illustrative Example: Coalition For Health Ai Chai Standards Framework
Let’s imagine a hypothetical AI system, “DiagnoSys,” designed for diagnosing cardiovascular diseases. DiagnoSys adheres strictly to the Chai Standards Framework, prioritizing patient safety, fairness, and transparency throughout its operation. This system leverages machine learning models trained on a vast, anonymized dataset of patient records, encompassing demographics, medical history, lab results, and imaging data.
Data Security Measures
DiagnoSys employs robust data security measures to protect patient privacy. All data is encrypted both in transit and at rest, utilizing industry-standard encryption protocols like AES-256. Access to the system is strictly controlled through role-based access control (RBAC), ensuring that only authorized personnel can access specific data subsets. Furthermore, regular security audits and penetration testing are conducted to identify and mitigate potential vulnerabilities.
Data anonymization techniques, such as differential privacy, are applied to the training dataset to prevent re-identification of individual patients. The system also maintains detailed audit logs of all data access and modifications, facilitating accountability and compliance with data privacy regulations.
Fairness and Bias Mitigation
To ensure fairness and mitigate bias in diagnoses, DiagnoSys incorporates several strategies. The training dataset is carefully curated to represent the diversity of the patient population, aiming for balanced representation across demographics and socioeconomic factors known to correlate with cardiovascular disease risk. Furthermore, the system utilizes fairness-aware machine learning algorithms that actively monitor and adjust for potential biases during model training and prediction.
For example, techniques like re-weighting samples or adversarial debiasing are employed to minimize disparities in diagnostic accuracy across different subgroups. Regular audits of the system’s performance across various demographic groups are conducted to identify and address any emerging biases.
Transparency
DiagnoSys prioritizes transparency in its diagnostic process. The system provides detailed explanations for its diagnoses, outlining the key factors that contributed to the prediction. This explanation is presented in a clear and understandable manner for both clinicians and patients. The models’ performance metrics, including sensitivity, specificity, and positive predictive value, are regularly monitored and reported. Furthermore, the system’s source code and training data (after appropriate anonymization) are made available to qualified researchers and auditors, allowing for independent verification and validation of its methods and results.
This transparency promotes trust and accountability, allowing clinicians and patients to critically assess the AI’s recommendations.
Ethical Considerations
The application of AI in healthcare presents a unique set of ethical challenges, demanding careful consideration and robust frameworks to guide its responsible development and deployment. The potential benefits are immense, but so are the risks if not properly managed. The Chai Standards Framework directly addresses these concerns, aiming to ensure that AI systems in healthcare are not only effective but also ethical and equitable.AI’s increasing role in diagnosis, treatment planning, and patient care raises questions about bias, transparency, accountability, and patient autonomy.
These are not merely theoretical concerns; they have real-world implications for patient safety and trust in the healthcare system. The Chai framework provides a structured approach to navigate these complexities.
Bias and Fairness in AI Algorithms
Algorithmic bias, stemming from biased training data, can lead to discriminatory outcomes in healthcare. For example, an AI system trained primarily on data from one demographic group might misdiagnose or undertreat patients from other groups. The Chai Standards Framework emphasizes the need for diverse and representative datasets to mitigate bias and ensure fairness in AI-driven healthcare decisions. This includes rigorous testing and validation to identify and correct biases before deployment.
Regular audits and ongoing monitoring are also key aspects of the framework.
Transparency and Explainability
Understanding how an AI system arrives at its conclusions is crucial for building trust and accountability. “Black box” AI models, whose decision-making processes are opaque, are ethically problematic, particularly in high-stakes healthcare settings. The Chai framework promotes the development of explainable AI (XAI) systems, which provide insights into their reasoning and allow clinicians to understand and validate AI-generated recommendations.
This transparency allows for better oversight and reduces the risk of errors.
Data Privacy and Security
Healthcare data is highly sensitive, and its misuse can have severe consequences. The Chai Standards Framework incorporates robust data privacy and security measures, aligning with relevant regulations such as HIPAA and GDPR. This includes secure data storage, access control mechanisms, and anonymization techniques to protect patient information. The framework also emphasizes the importance of informed consent and patient control over their data.
Accountability and Responsibility
Determining responsibility when an AI system makes an error is a complex ethical challenge. The Chai framework addresses this by establishing clear lines of accountability for the development, deployment, and use of AI systems in healthcare. This involves defining roles and responsibilities for various stakeholders, including developers, clinicians, and healthcare institutions. Mechanisms for oversight and redress are also integral components of the framework.
Patient Autonomy and Informed Consent
AI systems should not override patient autonomy or diminish their ability to make informed decisions about their own care. The Chai Standards Framework underscores the importance of ensuring that patients are fully informed about the use of AI in their treatment and have the right to refuse AI-assisted interventions. The framework promotes shared decision-making between patients and clinicians, emphasizing the human element in healthcare.
The ethical implications of AI in healthcare, as addressed by the Chai Standards Framework, necessitate a balanced approach prioritizing patient well-being, fairness, transparency, and accountability. The framework provides a robust structure for mitigating risks and maximizing the benefits of AI while upholding the highest ethical standards.
Closing Summary
The Coalition for Health AI Chai Standards Framework represents a significant step toward responsible AI implementation in healthcare. By prioritizing ethical considerations, transparency, and robust standards, this framework paves the way for a future where AI empowers healthcare providers and improves patient outcomes. While challenges remain, the collaborative spirit and proactive approach of the coalition offer a beacon of hope for a safer, more equitable, and technologically advanced healthcare system.
The journey towards realizing the full potential of AI in healthcare is ongoing, and the Chai Standards Framework is a critical compass guiding us along the way.
Top FAQs
What specific types of AI systems does the Chai Standards Framework cover?
The framework aims to be inclusive, encompassing a wide range of AI applications in healthcare, from diagnostic tools and treatment recommendations to administrative processes and patient monitoring systems.
How is compliance with the Chai Standards Framework enforced?
Enforcement mechanisms are likely to vary depending on the context and jurisdiction. It might involve a combination of self-regulation, industry best practices, and potentially government oversight in the future.
Who are the main competitors or alternative frameworks to the Chai Standards Framework?
While the Chai Standards Framework is relatively new, it’s important to note that other organizations are also developing AI guidelines and standards for healthcare. A comparison with these frameworks would be valuable to highlight the unique aspects of the Chai initiative.
What resources are available to help organizations understand and implement the Chai Standards Framework?
The Coalition for Health AI likely provides documentation, training materials, and potentially support services to assist organizations in adopting the framework. Check their official website for details.