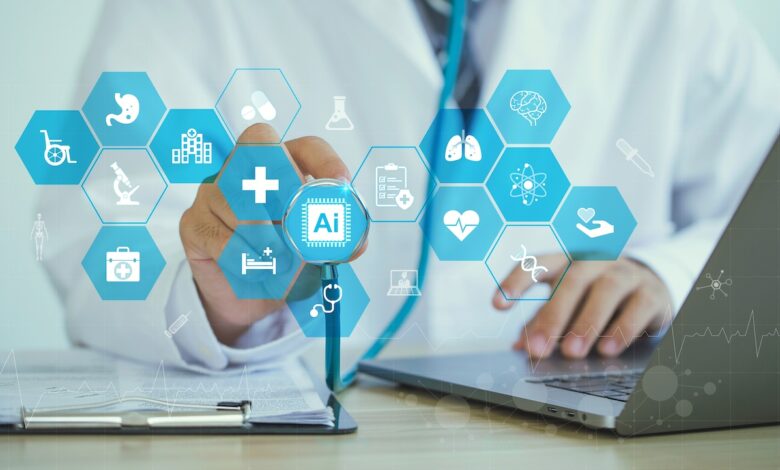
Coalition Health AI Model Card Registry
Coalition Health AI Model Card Registry: Imagine a world where every AI model used in healthcare is transparent, accountable, and readily understood. That’s the promise of a coalition-built registry for AI model cards. This isn’t just about technical specifications; it’s about building trust, ensuring fairness, and ultimately, improving patient care. This registry aims to be the central hub for understanding the strengths, weaknesses, and potential biases of AI models used in diagnosis, treatment, and research.
The key is collaboration. A successful registry needs buy-in from researchers, clinicians, regulators, and tech developers. It requires standardized data formats, robust security measures to protect sensitive patient information, and a commitment to ongoing evaluation and improvement. This post delves into the exciting potential – and the considerable challenges – of creating a truly impactful coalition health AI model card registry.
Defining “Coalition Health AI Model Card Registry”
The development and deployment of AI models in healthcare is rapidly accelerating, presenting both immense opportunities and significant challenges. A key aspect of responsible AI development is transparency and accountability. This is where the concept of a Coalition Health AI Model Card Registry comes into play, offering a structured approach to documenting and sharing information about these vital tools.A coalition, in this context, represents a collaborative effort between diverse stakeholders in the healthcare AI ecosystem.
This might include hospitals, research institutions, technology companies, regulatory bodies, and patient advocacy groups. The shared goal is to foster trust, improve model quality, and promote responsible innovation. By working together, these entities can leverage their collective expertise and resources to address the complexities of developing and deploying AI in healthcare more effectively.
The Purpose of a Health AI Model Card Registry
The primary purpose of a registry specifically designed for health AI models is to provide a central repository of standardized information about these models. This information, presented in the form of model cards, allows for a transparent and easily accessible overview of a model’s capabilities, limitations, and potential biases. This enhanced transparency is crucial for building trust amongst clinicians, patients, and regulators.
The registry acts as a vital tool for facilitating informed decision-making regarding the selection and deployment of AI models in various healthcare settings. It supports responsible innovation by promoting critical evaluation and informed usage.
Key Features and Functionalities of a Coalition Health AI Model Card Registry
A successful registry requires several key features. Firstly, it must adhere to a standardized model card format, ensuring consistency and comparability across different models. This standardized format should include details such as model description, intended use case, training data, performance metrics, limitations, and ethical considerations. Secondly, the registry needs a robust search and filtering functionality, enabling users to easily locate models relevant to their specific needs.
This might involve searching by disease area, model type, or performance metrics. Thirdly, version control is essential, allowing users to track changes and updates to individual models over time. Finally, access control mechanisms are crucial, balancing the need for open access with the protection of sensitive data. A well-designed registry might offer different levels of access based on user roles and affiliations.
Benefits and Challenges of a Coalition Health AI Model Card Registry
Benefit | Challenge |
---|---|
Increased transparency and accountability in AI model development | Ensuring consistent and high-quality model card submissions |
Improved model comparability and selection | Maintaining the registry and updating it regularly |
Facilitated collaboration and knowledge sharing among stakeholders | Balancing open access with the need for data privacy and security |
Enhanced trust and confidence in AI-driven healthcare | Addressing potential biases and ensuring fairness in AI models |
Data Governance and Standards
Building a robust and trustworthy Coalition Health AI Model Card Registry requires a strong foundation in data governance and standardized practices. This ensures the registry’s utility, promotes interoperability between different AI models, and safeguards sensitive patient data. The key lies in defining essential data elements, implementing standardized metadata, and establishing robust data privacy and security protocols.
Essential Data Elements for Model Cards
Model cards within the registry must contain comprehensive information to facilitate effective evaluation and comparison of AI models. This goes beyond simple performance metrics and delves into the model’s development, deployment, and potential biases. A minimum viable set of data elements should include: model name and version, intended use case, training data description (including size, source, and preprocessing steps), model architecture, performance metrics (with clear definitions and context), limitations and potential biases, intended user group, validation and testing procedures, and contact information for the model developers.
Additionally, details about the model’s deployment environment and any ongoing monitoring activities should be documented. This comprehensive approach ensures transparency and accountability.
The Coalition Health AI Model Card Registry is a fantastic resource for understanding the biases and limitations of different AI models. But sometimes, even the best AI can’t help with physical problems like carpal tunnel syndrome, which is why I found this article on ways to treat carpal tunnel syndrome without surgery so helpful. Understanding these non-surgical options is important, especially when considering the potential impact of repetitive tasks on data scientists working with the registry itself.
Model Evaluation and Transparency
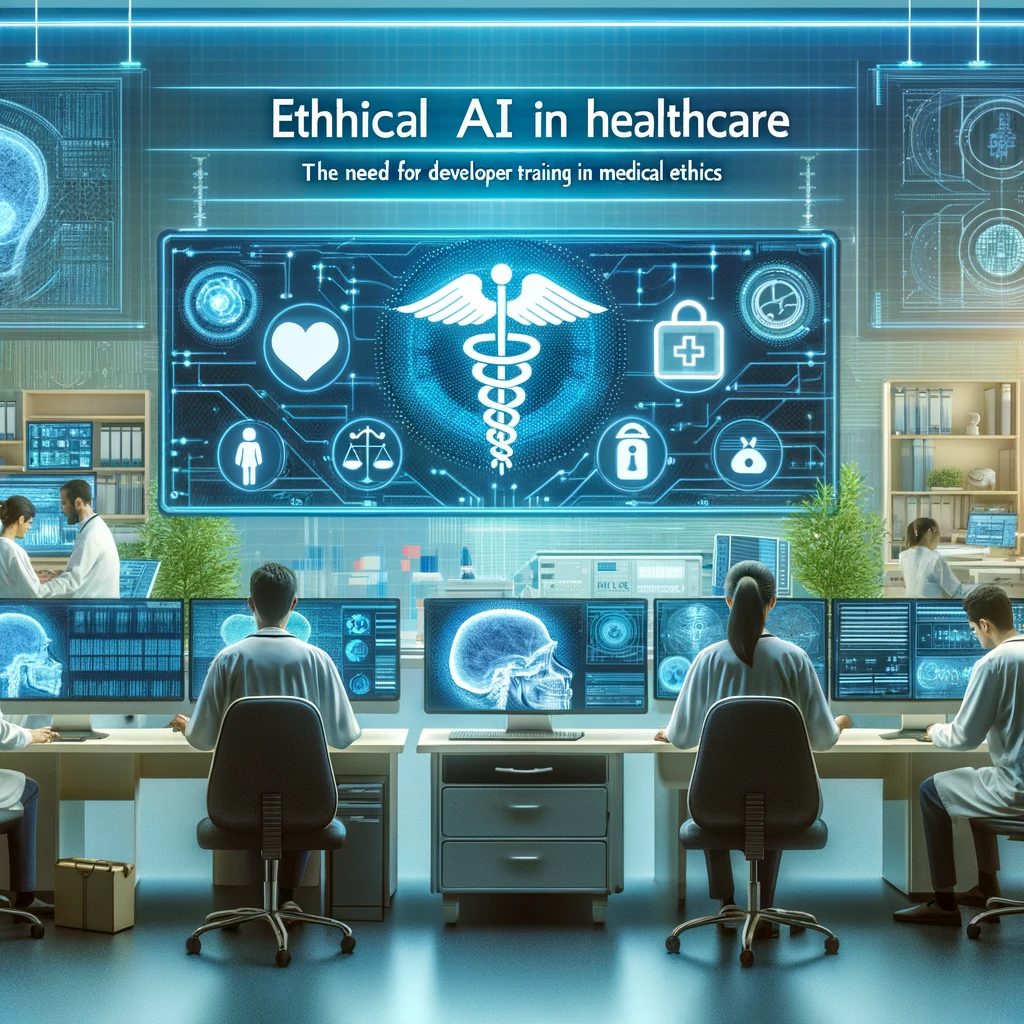
Source: medium.com
The evaluation and transparent reporting of health AI models are paramount for ensuring their responsible development and deployment. This involves not only rigorous performance assessment but also clear documentation of the entire model lifecycle, from data collection to final deployment. This transparency fosters trust, facilitates scrutiny, and ultimately protects patient safety.The performance of a health AI model is judged against several key metrics and evaluation methods, chosen based on the specific task and intended use case.
The process needs to be documented meticulously to ensure reproducibility and allow others to validate the findings.
Metrics and Methods for Evaluating Health AI Model Performance
Model performance in healthcare often involves assessing several aspects, going beyond simple accuracy. For example, in a diagnostic setting, sensitivity (true positive rate), specificity (true negative rate), and the positive predictive value (PPV) are critical. These metrics quantify the model’s ability to correctly identify positive and negative cases, and the likelihood that a positive prediction is actually correct.
Furthermore, the area under the receiver operating characteristic curve (AUC-ROC) provides a comprehensive measure of the model’s ability to discriminate between different classes. For models predicting continuous outcomes, metrics like mean absolute error (MAE), root mean squared error (RMSE), and R-squared are relevant. The choice of metric depends heavily on the specific clinical task. For example, in a cancer detection model, high sensitivity might be prioritized to minimize false negatives, even if it means accepting a higher rate of false positives.
Best Practices for Ensuring Transparency and Reproducibility
Reproducibility is crucial for validating the results of AI model development. This necessitates meticulous documentation of the entire process, including data preprocessing steps, feature engineering techniques, model training parameters, and hyperparameter tuning strategies. Version control systems (like Git) should be used to track changes in code and data. Detailed model architectures should be documented, and all code should be well-commented and publicly available (where appropriate and ethically permissible).
Furthermore, standardized data formats and metadata should be employed to ensure data interoperability and facilitate reproducibility across different research groups. The use of standardized evaluation protocols also enhances reproducibility and comparability of results. A clear description of the evaluation dataset, including its size, characteristics, and any potential biases, is vital.
The Role of Model Cards in Promoting Responsible AI Development and Deployment
Model cards are concise summaries of a model’s capabilities, limitations, and intended use. They are crucial tools for promoting transparency and facilitating responsible AI development and deployment in healthcare. A well-structured model card should clearly describe the model’s intended use, the data used for training and evaluation, the model’s performance metrics, and any known limitations or biases. It should also specify the intended user group and any ethical considerations related to the model’s use.
Model cards empower clinicians and other stakeholders to make informed decisions about the suitability and trustworthiness of a specific AI model for their particular needs. They also promote accountability and facilitate ongoing monitoring and evaluation of the model’s performance. By standardizing the format and content of model cards, we can improve the clarity and comparability of information about different health AI models.
Potential Biases in Health AI Models
Addressing bias is critical for ensuring fairness and equity in health AI. Biases can arise at various stages of the model development lifecycle, from data collection and preprocessing to model training and evaluation. These biases can lead to inaccurate or discriminatory outcomes, potentially exacerbating existing health disparities.
- Data Bias: Imbalance in the representation of different demographic groups (e.g., race, gender, socioeconomic status) in the training data can lead to models that perform poorly for underrepresented groups.
- Algorithmic Bias: The algorithms themselves can introduce biases, either through the design choices or through unintended consequences of the learning process.
- Measurement Bias: Differences in the quality or availability of data across different populations can lead to systematic errors in model predictions.
- Label Bias: Inaccuracies or inconsistencies in the labeling of data can introduce biases into the model’s learning process.
- Selection Bias: Bias in the selection of patients for inclusion in the dataset can lead to models that do not generalize well to the broader population.
Collaboration and Community Building
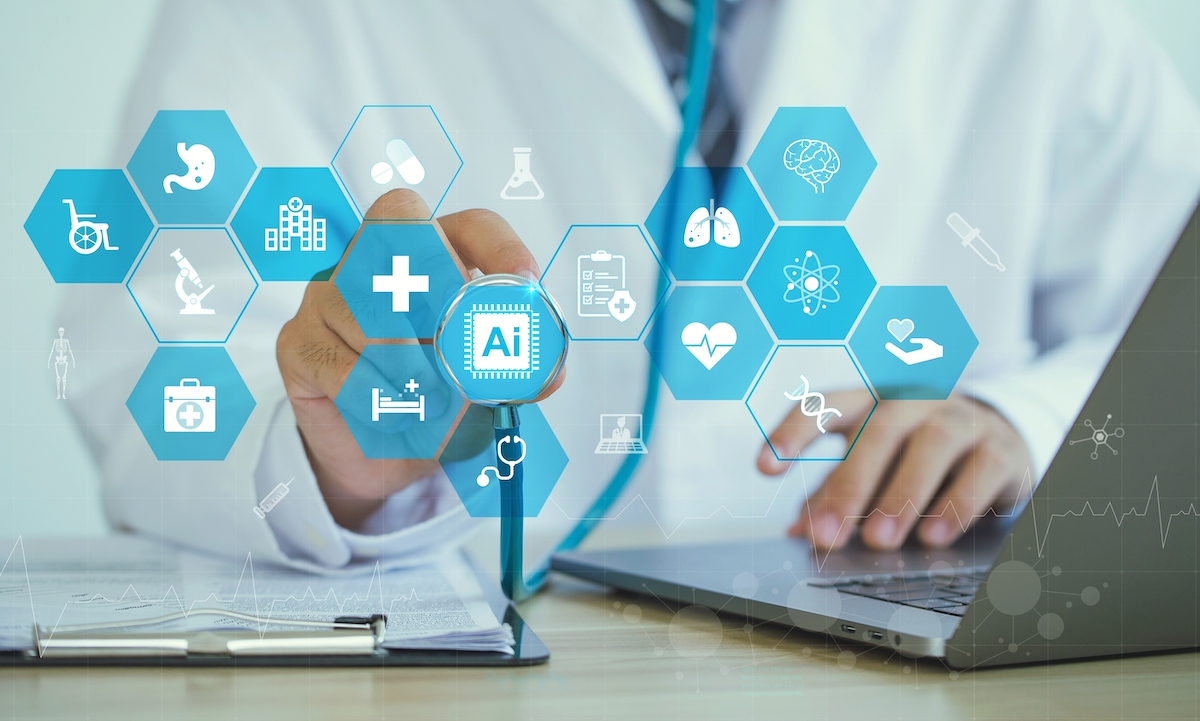
Source: pymnts.com
Building a successful Coalition Health AI Model Card Registry requires a robust collaborative framework and a thriving community of engaged stakeholders. This involves carefully considering governance models, developing effective engagement strategies, and implementing efficient communication channels. The ultimate goal is to foster widespread adoption and utilization of the registry, ensuring its value as a central resource for healthcare AI.
Effective collaboration hinges on selecting the right governance model and engaging key stakeholders. Different models offer varying levels of control and participation, each with its own strengths and weaknesses. A successful engagement strategy must consider the unique needs and perspectives of researchers, clinicians, and regulators, fostering trust and transparency throughout the process.
Governance Models for the Registry
Several models can govern the registry, each with implications for decision-making, resource allocation, and overall success. A centralized model, where a single organization manages all aspects of the registry, offers strong control and consistency. However, it might limit participation and responsiveness to diverse needs. A decentralized model, distributing governance among multiple organizations, fosters greater inclusivity and adaptability but could lead to inconsistencies and coordination challenges.
A hybrid approach, combining elements of both, might strike the best balance, leveraging the strengths of each while mitigating their weaknesses. For example, a central coordinating body could oversee standards and data governance, while individual organizations manage their contributions and participation. This hybrid model could also incorporate a tiered system, giving different stakeholders varying degrees of influence based on their expertise and contribution.
For instance, a clinical advisory board could guide clinical aspects, while a technical working group addresses technical issues.
Stakeholder Engagement Strategies
Engaging researchers, clinicians, and regulators is crucial for the registry’s success. Researchers need to be incentivized to contribute their model cards, potentially through recognition, publication opportunities, or access to collaborative tools. Clinicians require assurance of the registry’s reliability and clinical relevance, necessitating clear communication of the registry’s purpose, rigorous evaluation standards, and potential clinical benefits. Regulators need transparency and assurance of compliance with relevant regulations, which can be achieved through open communication, clear documentation of governance processes, and adherence to established data privacy and security standards.
Regular workshops, conferences, and online forums can facilitate interaction and feedback, fostering a sense of shared ownership and commitment.
Registry Adoption and Use Promotion Plan, Coalition health ai model card registry
Promoting adoption requires a multi-pronged approach. This includes targeted outreach to key healthcare organizations, highlighting the registry’s benefits through publications, presentations at relevant conferences, and online resources. Creating user-friendly documentation, tutorials, and FAQs is essential to reduce the barrier to entry. Incentivizing participation, such as offering data sharing agreements or collaborative research opportunities, can further encourage adoption. Regular updates and improvements to the registry, based on user feedback, will maintain its relevance and value.
Success stories and case studies demonstrating the practical benefits of using the registry can be powerful tools for promotion.
Facilitating Communication and Knowledge Sharing
Effective communication is paramount. A dedicated online platform, possibly incorporating a forum or discussion board, can facilitate information sharing and collaboration among users. Regular newsletters, updates, and announcements will keep users informed about registry developments and best practices. Workshops and training sessions can provide hands-on experience with the registry and its features. The platform could also include tools for identifying and connecting users with similar interests or expertise, fostering collaboration on specific projects or research initiatives.
Establishing a clear feedback mechanism allows for continuous improvement and responsiveness to user needs. The use of a standardized model card template, along with clear guidelines and documentation, will also promote efficient communication and reduce ambiguity.
Future Directions and Scalability: Coalition Health Ai Model Card Registry
The Coalition Health AI Model Card Registry, while already a significant resource, possesses immense potential for growth and improvement. Its future success hinges on strategic enhancements, robust scalability, and proactive mitigation of potential challenges. This section explores these critical aspects, outlining a path towards a more comprehensive and sustainable registry.The registry’s scalability must address both the increasing number of AI models submitted and the growing user base seeking access to this valuable information.
Future enhancements will focus on improving the user experience, expanding the types of models included, and strengthening the data governance framework.
The Coalition Health AI Model Card Registry is a crucial resource for understanding the potential biases and limitations of AI in healthcare. It’s vital to ensure these models are used responsibly, especially when considering conditions like respiratory illnesses. News reports, like this one about Monali Thakur’s hospitalization after struggling to breathe monali thakur hospitalised after struggling to breathe how to prevent respiratory diseases , highlight the urgent need for accurate and reliable AI tools.
Ultimately, the registry’s goal is to promote the development of safe and effective AI solutions for improving patient care.
Registry Architecture and Workflow
A visual representation of the registry would depict a modular architecture. At its core is a central database storing model card information, meticulously organized and indexed for efficient retrieval. This database interacts with a user interface (UI) allowing users to search, filter, and view model cards. A separate module manages user accounts and access permissions, ensuring data security and privacy.
The workflow begins with model submitters uploading their model cards through a standardized submission process. These cards are then reviewed and validated by curators before being added to the database. Users can then access the database through the UI, performing searches based on various criteria. A feedback mechanism allows users to report issues or suggest improvements, feeding back into the curation and improvement process.
This entire system is built upon a robust infrastructure capable of handling increasing data volume and user traffic. For example, a microservices architecture could be employed to allow for independent scaling of different components of the system, ensuring responsiveness even under high load.
Enhanced Search and Filtering Capabilities
Current search functionalities will be expanded to include advanced filtering options, allowing users to refine their searches based on specific model characteristics, such as intended application, data used for training, performance metrics, and even specific ethical considerations. This will enable more targeted searches and quicker identification of relevant models. For instance, a researcher seeking a model for diagnostic purposes in a specific medical field could filter by application, dataset, and performance metrics relevant to their specific needs.
This granular level of search functionality will be crucial for the growing volume of models within the registry.
Improved Model Card Standardization and Validation
To maintain data quality and consistency, the registry will continue to refine its model card standardization process. This includes implementing automated validation checks during the submission process to flag inconsistencies or missing information. This will reduce manual review time and ensure all model cards adhere to the highest standards of transparency and completeness. For example, the system could automatically check for required fields, data type consistency, and adherence to pre-defined formats.
This automated validation will significantly enhance the efficiency of the curation process.
The Coalition Health AI Model Card Registry is a crucial resource for understanding the biases and limitations of AI in healthcare. This is especially important when considering personalized medicine, which needs to account for factors like individual nutritional needs; check out this interesting article on how those needs differ between genders: are women and men receptive of different types of food and game changing superfoods for women.
Ultimately, the registry’s goal is to promote responsible AI development, ensuring fairness and efficacy across all patient populations, including those with unique dietary requirements.
Addressing Scalability Challenges
Scaling the registry to accommodate a growing number of models and users requires a multi-pronged approach. This includes adopting a scalable database technology capable of handling large datasets and high query volumes. Load balancing and caching mechanisms will be implemented to ensure responsiveness under peak demand. Furthermore, the system architecture will be designed to be modular and easily extensible, allowing for the addition of new features and functionalities without disrupting existing operations.
For example, migrating to a cloud-based infrastructure will allow for on-demand scaling of resources, adapting to fluctuating user demand and model submissions. Regular performance testing and optimization will be crucial to maintain system stability and responsiveness.
Long-Term Sustainability and Maintenance
The long-term sustainability of the registry requires a clear governance structure, ongoing funding, and a dedicated team for maintenance and updates. A robust funding model, potentially involving partnerships with research institutions, industry stakeholders, and government agencies, is essential. Furthermore, a clear roadmap for future development and a community engagement strategy will ensure the registry remains relevant and valuable in the evolving landscape of AI in healthcare.
For example, establishing a community advisory board composed of experts from various fields can provide valuable insights and guidance on the future direction of the registry. Regular audits and security assessments will be conducted to maintain data integrity and user privacy.
Closing Notes
The creation of a coalition health AI model card registry represents a significant step towards responsible and ethical AI in healthcare. While challenges exist regarding data governance, standardization, and scalability, the potential benefits – increased transparency, improved model evaluation, and ultimately, better patient outcomes – are immense. This collaborative effort demands continued dialogue, innovation, and a shared commitment to leveraging AI’s power for good.
The future of healthcare AI hinges on initiatives like this, fostering trust and ensuring that this powerful technology serves humanity’s best interests.
Questions and Answers
What are the legal implications of using a model from this registry?
The legal implications vary depending on jurisdiction and specific model use. The registry itself doesn’t provide legal advice; users are responsible for understanding and complying with all relevant laws and regulations.
How often will the model cards be updated?
The update frequency will depend on the model and any changes in its performance, underlying data, or intended use. A version control system within the registry will track updates and their rationale.
Who is responsible for ensuring the accuracy of information in the registry?
Responsibility for accuracy rests primarily with the model developers and contributing organizations. The registry may employ mechanisms for validation and community review to ensure data quality.
What happens if a model listed in the registry is found to be biased or inaccurate?
A clear process for reporting and addressing issues, including retractions or updates to model cards, will be crucial. This process will likely involve a review board and transparent communication to users.