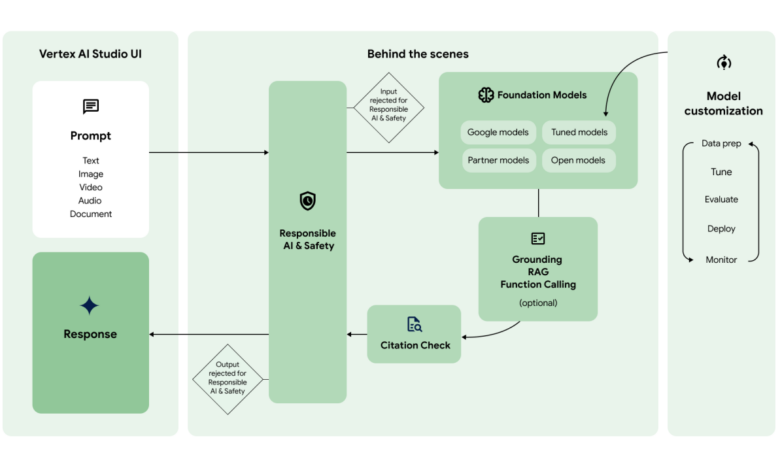
Community Health Systems Google Cloud Generative AI
Community Health Systems Google Cloud Generative AI: Imagine a future where predicting patient outcomes, personalizing treatment plans, and streamlining administrative tasks are all handled with the power of AI, seamlessly integrated within the robust infrastructure of Google Cloud. This isn’t science fiction; it’s the rapidly evolving reality for community health systems, poised to revolutionize healthcare delivery and patient experiences.
This exploration delves into how generative AI, coupled with Google Cloud’s capabilities, is transforming data management, predictive analytics, operational efficiency, and patient engagement within these vital systems.
The challenges faced by community health systems in managing vast amounts of patient data are significant. Google Cloud offers solutions like data warehousing and data lakes to overcome these hurdles, enabling efficient storage, analysis, and utilization of this crucial information. Generative AI, further enhancing these capabilities, allows for predictive modeling to identify at-risk populations, personalize treatment plans, and automate various administrative processes.
This ultimately leads to improved operational efficiency, enhanced patient engagement through AI-powered communication tools, and a more proactive, data-driven approach to healthcare.
Community Health Systems and Data Management: Community Health Systems Google Cloud Generative AI
Community health systems (CHS) play a vital role in providing healthcare to diverse populations, often facing unique challenges. Managing the ever-increasing volume and complexity of patient data is a significant hurdle for these systems, impacting operational efficiency, care quality, and financial sustainability. The sheer scale of data, coupled with disparate systems and legacy technologies, creates a complex landscape demanding innovative solutions.The challenges CHSs face in managing patient data are multifaceted.
Community health systems are exploring Google Cloud’s generative AI for improving patient care, and this includes identifying ways to personalize treatment plans. For example, understanding the specific needs of children with neurological conditions is crucial, which is why learning about effective strategies to manage Tourette syndrome in children is so valuable. This knowledge can then inform the development of AI-driven tools within community health systems to provide better support and resources for families dealing with these challenges.
They often grapple with data silos, where information is fragmented across different departments and systems, hindering seamless access and analysis. Data security and compliance with regulations like HIPAA are paramount, requiring robust security measures and stringent data governance policies. Furthermore, the need for real-time data access for informed decision-making necessitates efficient data processing and analytics capabilities. Finally, budget constraints and limited IT expertise can hinder the adoption and implementation of advanced data management solutions.
Google Cloud’s Infrastructure Solutions for CHS Data Management
Google Cloud Platform (GCP) offers a comprehensive suite of tools and services designed to address these data management challenges. Its scalable and secure infrastructure provides the foundation for building a robust and efficient data management system. GCP’s cloud-based data warehouse solutions, such as BigQuery, offer unparalleled scalability and speed for processing and analyzing large datasets, enabling CHSs to gain valuable insights from their patient data.
Furthermore, GCP’s data lake solutions, such as Dataproc and Dataplex, allow for the storage and processing of diverse data types in their raw format, providing flexibility and agility in data analysis. The robust security features within GCP, including encryption, access control, and auditing, ensure compliance with HIPAA and other relevant regulations. Finally, GCP’s managed services reduce the burden on CHS IT teams, allowing them to focus on strategic initiatives rather than infrastructure management.
Data Warehousing and Data Lakes Improving Healthcare Operations
Implementing a data warehouse on GCP can streamline reporting and analytics. For example, a CHS can consolidate patient data from various sources – electronic health records (EHRs), billing systems, and public health databases – into a centralized data warehouse. This enables the generation of comprehensive reports on patient demographics, disease prevalence, and treatment outcomes, supporting better resource allocation and improved public health initiatives.
A data lake, on the other hand, offers a more flexible approach, allowing the CHS to store both structured and unstructured data, such as medical images, clinical notes, and sensor data. This enables advanced analytics using machine learning, for example, predicting patient readmissions or identifying at-risk populations for proactive intervention. By combining data warehouse and data lake capabilities, CHSs can gain a 360-degree view of their patients, leading to improved care coordination and better population health management.
Migrating Existing Patient Data to Google Cloud
Migrating existing patient data to Google Cloud requires a well-defined strategy that prioritizes data security and compliance. A phased approach, starting with a pilot project involving a smaller dataset, is recommended. This allows for testing and refinement of the migration process before scaling to the entire dataset. Data encryption both in transit and at rest is crucial. Access control mechanisms should be implemented to restrict access to authorized personnel only.
Regular data backups and disaster recovery planning are essential to ensure business continuity. Throughout the migration process, rigorous auditing and monitoring are necessary to maintain data integrity and compliance with HIPAA regulations. Working with a Google Cloud certified partner can provide expertise and support in navigating the complexities of data migration and ensuring a smooth transition. For example, a CHS might choose to use Google Cloud’s Data Transfer Service for efficient and secure data movement, and leverage their professional services for guidance on compliance and best practices.
Leveraging Generative AI for Predictive Analytics
Generative AI offers a powerful new tool for community health systems, promising significant advancements in predictive analytics and personalized care. By analyzing vast amounts of historical patient data, these AI models can identify patterns and relationships invisible to the human eye, leading to more accurate predictions and proactive interventions. This capability translates to improved patient outcomes, more efficient resource allocation, and a stronger focus on preventative care.Predicting Patient Health Outcomes Based on Historical DataGenerative AI models, trained on extensive datasets encompassing patient demographics, medical history, lifestyle factors, and genetic information, can predict the likelihood of future health events.
For example, a model could predict the probability of a patient developing diabetes based on their current health metrics and family history. This predictive power allows for timely interventions, such as lifestyle modifications or medication adjustments, to mitigate the risk. The accuracy of these predictions improves as the model is trained on more data, allowing for increasingly refined risk assessments.
Identifying At-Risk Populations
Generative AI can effectively identify at-risk populations within a community by analyzing aggregated and anonymized patient data. By identifying clusters of individuals with similar risk profiles, health systems can target preventative care programs and allocate resources more efficiently. For instance, a model might identify a specific geographic area with a high prevalence of heart disease risk factors, enabling targeted public health initiatives focused on improving diet, exercise, and access to healthcare within that community.
This proactive approach reduces healthcare costs and improves overall community health.
Personalized Medicine and Treatment Recommendations
Generative AI can personalize medicine by tailoring treatment plans to individual patients. By considering a patient’s unique genetic makeup, medical history, and lifestyle, the AI can generate personalized treatment recommendations, potentially including medication choices, dosages, and therapy options. For example, a model might suggest a specific cancer treatment regimen based on a patient’s tumor characteristics and genetic profile, maximizing treatment effectiveness and minimizing side effects.
This personalized approach moves beyond one-size-fits-all treatments, leading to improved patient outcomes.
Integrating Generative AI Predictions into Clinical Decision-Making
Integrating generative AI predictions into existing clinical workflows requires a carefully designed process. First, the AI model’s output should be presented to clinicians in a clear and understandable format, avoiding technical jargon. Second, the system should allow clinicians to review the AI’s reasoning and potentially override its recommendations based on their clinical judgment. Third, a robust system for monitoring the AI’s performance and accuracy is crucial, ensuring that the model’s predictions are reliable and up-to-date.
A successful integration involves a collaborative approach, empowering clinicians with AI-driven insights while maintaining human oversight. For instance, a system might flag patients at high risk for readmission, allowing clinicians to proactively contact them for follow-up care and reduce hospital readmission rates.
Improving Operational Efficiency with Generative AI
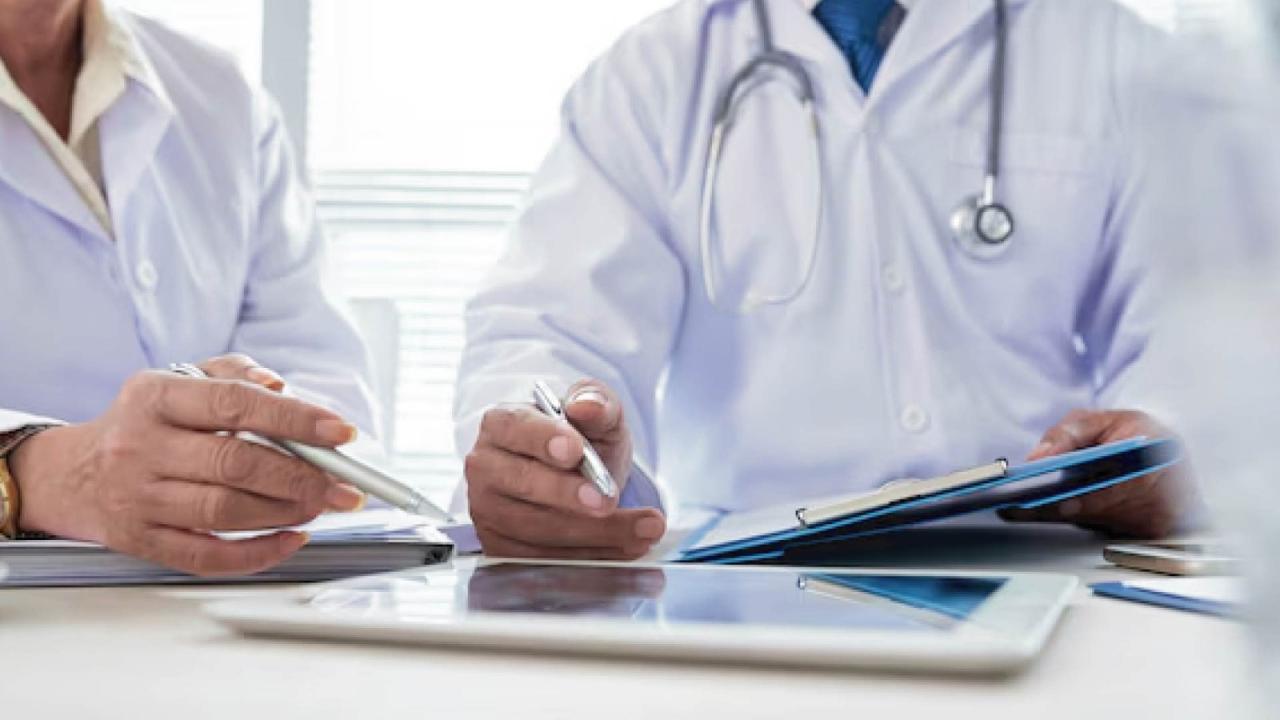
Source: ai-techpark.com
Generative AI offers community health systems a powerful toolset for streamlining operations and enhancing efficiency. By automating repetitive tasks, improving communication, and optimizing resource allocation, these systems can free up valuable staff time, reduce costs, and ultimately improve patient care. This section explores how generative AI can be leveraged to achieve significant operational improvements.
Areas for Generative AI Automation in Community Health Systems, Community health systems google cloud generative AI
Generative AI can automate numerous tasks within a community health system, leading to significant efficiency gains. For instance, AI-powered chatbots can handle routine patient inquiries, freeing up administrative staff to focus on more complex issues. Medical transcription can be significantly sped up and improved through AI-driven tools, reducing delays in report generation and improving accuracy. Furthermore, AI can assist in preliminary diagnosis support by analyzing patient data, flagging potential issues for physician review, and suggesting relevant tests.
Finally, the automated generation of standard reports and documents can reduce the time spent on administrative paperwork.
Cost-Effectiveness of Generative AI vs. Manual Processes
While the initial investment in generative AI technology may seem significant, the long-term cost savings can be substantial. Manual processes often involve higher labor costs, increased risk of human error, and slower turnaround times. For example, a study by [Insert reputable source and study here with specific data comparing costs] showed that implementing AI-powered appointment scheduling reduced administrative staff time by X% and decreased no-show rates by Y%, resulting in a Z% reduction in overall costs.
The cost-effectiveness of generative AI will vary depending on the specific application and the size of the health system, but the potential for ROI is considerable.
Comparison of Generative AI Tools for Community Health Systems
Tool Name | Strengths | Weaknesses | Suitable Use Cases |
---|---|---|---|
Example AI Tool 1 (e.g., a specific chatbot platform) | Easy integration, scalable, handles high volume of inquiries | May require customization for specific medical terminology, potential for inaccurate responses | Patient appointment scheduling, answering frequently asked questions |
Example AI Tool 2 (e.g., a specific medical transcription service) | High accuracy, fast transcription speeds, reduced human error | High initial investment cost, potential dependence on reliable internet connection | Medical record transcription, generating clinical reports |
Example AI Tool 3 (e.g., a specific predictive analytics platform) | Predictive capabilities for resource allocation, identifies potential bottlenecks | Requires significant data input for accurate predictions, complex implementation | Optimizing staffing levels, predicting patient flow |
Example AI Tool 4 (e.g., a specific document generation tool) | Automates creation of standard documents, reduces manual paperwork | Limited flexibility for highly customized documents, potential for errors in complex cases | Generating discharge summaries, creating patient letters |
Streamlining Appointment Scheduling, Patient Communication, and Resource Allocation
Generative AI can significantly streamline various operational aspects of a community health system. AI-powered scheduling systems can optimize appointment slots, reduce wait times, and minimize no-shows through intelligent reminders and personalized communication. AI-driven chatbots can handle routine patient communication, such as appointment confirmations, medication reminders, and answering basic health questions, freeing up staff time. Finally, generative AI can analyze patient data and predict resource needs, allowing for proactive adjustments in staffing levels and resource allocation to ensure optimal efficiency and avoid bottlenecks.
For example, by analyzing historical data on patient volume and staff availability, an AI system can predict peak demand periods and automatically adjust scheduling to meet the anticipated need.
Enhancing Patient Engagement and Experience
Generative AI offers a powerful toolkit for revolutionizing patient engagement and improving the overall healthcare experience. By automating tasks, personalizing communications, and providing readily available support, AI can significantly enhance patient satisfaction and improve health outcomes. This goes beyond simple efficiency gains; it’s about building stronger, more trusting relationships between patients and healthcare providers.
Generative AI’s ability to process and understand vast amounts of data allows for highly personalized patient communication and education. This personalized approach fosters better understanding and compliance with treatment plans, ultimately leading to better health outcomes. For example, instead of generic pamphlets, AI can generate tailored educational materials that address a patient’s specific condition, literacy level, and cultural background.
This ensures the information is easily understood and relevant, increasing the likelihood of engagement.
Personalized Patient Communication and Education Materials
Generative AI can analyze a patient’s medical history, demographics, and preferences to create customized communications. Imagine receiving an email explaining your upcoming surgery in clear, simple language, tailored to your specific understanding. Or consider a post-operative care plan that’s not just a list of instructions, but a step-by-step guide with personalized reminders and motivational messages. This level of personalization ensures patients feel understood and supported throughout their healthcare journey.
The system could even adapt the communication style to match the patient’s preferred method, whether that’s email, text message, or even a phone call.
AI-Powered Chatbots for Patient Support
AI-powered chatbots can provide 24/7 access to information and support. These chatbots can answer frequently asked questions about appointments, medications, billing, and other administrative tasks, freeing up human staff to focus on more complex issues. For instance, a patient could use a chatbot to reschedule an appointment, request a prescription refill, or access their test results, all without having to wait on hold or make a phone call.
Beyond simple information retrieval, advanced chatbots can even offer preliminary symptom assessment and triage, guiding patients to the appropriate level of care. Consider a chatbot that can assess a patient’s symptoms and determine if an urgent care visit is necessary, potentially preventing unnecessary trips to the emergency room.
Community health systems are exploring Google Cloud’s generative AI for improving patient care and operational efficiency. This comes at a critical time, as the recent Supreme Court decision, scotus overturns chevron doctrine healthcare , could significantly reshape healthcare regulations and funding. Understanding these legal shifts is crucial as we integrate AI, ensuring compliance and responsible innovation within community health systems.
Ethical Considerations of Patient-Facing AI
The use of generative AI in patient care necessitates careful consideration of ethical implications. Maintaining patient privacy and data security is paramount. Robust security measures, including encryption and access controls, are crucial to protect sensitive health information. Transparency is also essential; patients should be informed about the use of AI in their care and have the option to opt out.
Furthermore, the potential for bias in AI algorithms needs to be addressed. Algorithms trained on biased data may perpetuate inequalities in healthcare access and quality. Regular audits and ongoing monitoring are essential to ensure fairness and equity. Clear guidelines and protocols should be established to manage situations where the AI system provides inaccurate or inappropriate information.
Implementing a Patient-Facing AI System
A successful implementation of a patient-facing AI system requires a phased approach that prioritizes user experience and accessibility. The first step involves identifying key areas where AI can provide the most value, such as appointment scheduling or medication reminders. A pilot program with a small group of patients can help identify areas for improvement and gather feedback. The system should be designed to be intuitive and easy to use, regardless of the patient’s technical skills or level of digital literacy.
Community health systems could revolutionize patient care using Google Cloud’s generative AI, analyzing vast datasets to predict health risks and optimize resource allocation. This opens doors to breakthroughs like the incredible news that the FDA has approved clinical trials for pig kidney transplants in humans, as reported by this article. Imagine how generative AI could further enhance the success of such trials by modeling optimal transplant strategies and improving post-operative care within community health systems.
Multilingual support and accessibility features for patients with disabilities are essential to ensure inclusivity. Ongoing monitoring and evaluation are crucial to ensure the system continues to meet the needs of patients and evolves with advancements in AI technology. Regular user feedback should be incorporated into the system’s development to maintain relevance and usability.
Security and Privacy Considerations
Implementing generative AI in a community health system offers significant advantages, but it also introduces considerable security and privacy challenges. Protecting sensitive patient data is paramount, requiring a robust and multifaceted approach that goes beyond standard data security practices. Failure to adequately address these concerns can lead to severe legal repercussions, reputational damage, and erosion of patient trust.
Data Encryption and Access Control
Protecting patient data requires employing strong encryption methods both in transit and at rest. This means all data, including patient records, AI models, and training data, should be encrypted using industry-standard algorithms. Access control mechanisms, such as role-based access control (RBAC), should be implemented to restrict access to sensitive data based on individual roles and responsibilities. Only authorized personnel should have access to patient data, and their access should be regularly audited and reviewed.
For example, a radiologist might have access to radiology images, but not to patient billing information. Multi-factor authentication should be mandatory for all users accessing the system.
HIPAA Compliance and Data Governance
Compliance with the Health Insurance Portability and Accountability Act (HIPAA) is non-negotiable. This includes implementing appropriate administrative, physical, and technical safeguards to protect the privacy and security of protected health information (PHI). A comprehensive data governance framework should be established, outlining policies and procedures for data handling, access control, data retention, and disposal. Regular audits and risk assessments should be conducted to ensure ongoing compliance.
This framework should explicitly address the use of generative AI, outlining specific procedures for data anonymization, de-identification, and data minimization. For instance, the system should be designed to prevent the accidental or intentional exposure of PHI during the training or deployment of AI models.
Risk Mitigation Strategies
Generative AI systems, while powerful, present unique risks. One key risk is the potential for data breaches resulting from vulnerabilities in the AI models or the underlying infrastructure. Robust security testing and penetration testing should be regularly performed to identify and address potential vulnerabilities. Regular software updates and patching are crucial to mitigate known vulnerabilities. Another risk is the potential for bias in the AI models, leading to unfair or discriminatory outcomes.
Mitigating this requires careful selection of training data, rigorous model evaluation, and ongoing monitoring for bias. Finally, the potential for adversarial attacks, where malicious actors attempt to manipulate the AI model, needs to be considered. Employing techniques like adversarial training and input validation can help mitigate this risk.
Best Practices Checklist for Secure AI in Google Cloud
Prior to implementing any AI solution within a Google Cloud environment, a comprehensive checklist should be followed. This checklist should include, but is not limited to:
- Conduct a thorough risk assessment to identify potential security and privacy risks.
- Implement strong access control mechanisms, including RBAC and multi-factor authentication.
- Encrypt all data both in transit and at rest using industry-standard encryption algorithms.
- Regularly monitor and audit system logs for suspicious activity.
- Implement data loss prevention (DLP) measures to prevent sensitive data from leaving the system.
- Regularly update and patch all software and hardware components.
- Develop and implement incident response plans to handle security breaches.
- Ensure compliance with all relevant healthcare regulations, including HIPAA.
- Conduct regular security testing and penetration testing.
- Establish a data governance framework to manage the lifecycle of patient data.
Future Applications and Innovations
Generative AI’s potential within community health systems extends far beyond its current applications. We’re only scratching the surface of what’s possible as the technology matures and datasets grow larger and more sophisticated. The coming years will likely witness a dramatic shift in how healthcare is delivered, thanks to the power of generative AI.The rapid advancement of generative AI presents a wave of exciting opportunities for revolutionizing healthcare delivery within community health systems.
Its ability to analyze complex datasets, identify patterns, and generate insightful predictions offers a powerful tool for enhancing various aspects of patient care and operational efficiency. This section explores emerging trends and potential future applications, along with the challenges and opportunities inherent in integrating this technology into the broader healthcare landscape.
Emerging Trends in Generative AI for Community Health Systems
Several key trends are shaping the future of generative AI in community health. Firstly, we’re seeing a move towards more personalized medicine, where AI models can tailor treatment plans based on individual patient characteristics and genetic predispositions. Secondly, federated learning is gaining traction, allowing multiple healthcare organizations to collaboratively train AI models on their combined data without sharing sensitive patient information directly.
This addresses crucial privacy concerns. Finally, explainable AI (XAI) is becoming increasingly important, as the need to understand the reasoning behind AI’s predictions grows alongside the complexity of the models. This ensures trust and accountability.
Potential Future Applications in Drug Discovery, Disease Prevention, and Public Health
Generative AI holds immense promise for accelerating drug discovery. By analyzing vast datasets of molecular structures and biological activity, AI models can predict the effectiveness of potential drug candidates, significantly reducing the time and cost associated with traditional drug development. For example, generative models could predict which compounds are most likely to be effective against specific disease targets, such as novel antibiotic resistant bacteria.
In disease prevention, AI could analyze population health data to identify individuals at high risk of developing certain conditions, allowing for proactive interventions and personalized preventative strategies. Imagine AI identifying individuals with a high genetic predisposition to heart disease and suggesting lifestyle changes or early screening. Finally, in public health, generative AI can model disease outbreaks, predict their spread, and optimize resource allocation to control epidemics.
For instance, an AI model could predict the spread of influenza based on mobility data and weather patterns, helping public health officials allocate vaccines and resources more effectively.
Challenges and Opportunities for Integrating Generative AI into the Healthcare Ecosystem
Integrating generative AI into the healthcare ecosystem presents both challenges and opportunities. A major challenge is ensuring data security and patient privacy. Robust data governance frameworks and advanced encryption techniques are essential to protect sensitive patient information. Another challenge is the need for robust validation and regulatory approval of AI-driven diagnostic and treatment tools. Rigorous clinical trials and regulatory oversight are necessary to ensure the safety and efficacy of these technologies.
However, the opportunities are immense. Generative AI has the potential to improve the efficiency and effectiveness of healthcare delivery, leading to better patient outcomes and reduced costs. The ability to analyze large datasets, identify patterns, and generate personalized insights can revolutionize healthcare as we know it. Successful integration will require collaboration between healthcare providers, technology developers, and policymakers.
Visual Representation of Generative AI’s Impact on Community Health Systems
Imagine a vibrant, interconnected network representing a community health system. Nodes represent individual patients, clinics, hospitals, and research institutions. Lines connecting the nodes represent data flows – patient records, clinical data, research findings, and public health information. Generative AI is depicted as a central hub, receiving and processing this data. From the hub, radiating outwards are streams of information representing personalized treatment plans, predictive risk assessments, optimized resource allocation, and accelerated drug discovery.
The network is dynamic, with the lines thickening and changing color to reflect the flow of information and the impact of AI insights. The overall visual emphasizes the transformative power of generative AI in connecting and optimizing the entire healthcare ecosystem, ultimately improving patient care and population health. The brighter and more vibrant the network, the more effectively the system is operating thanks to AI.
Dimmer areas represent areas needing more attention and data integration.
Final Wrap-Up
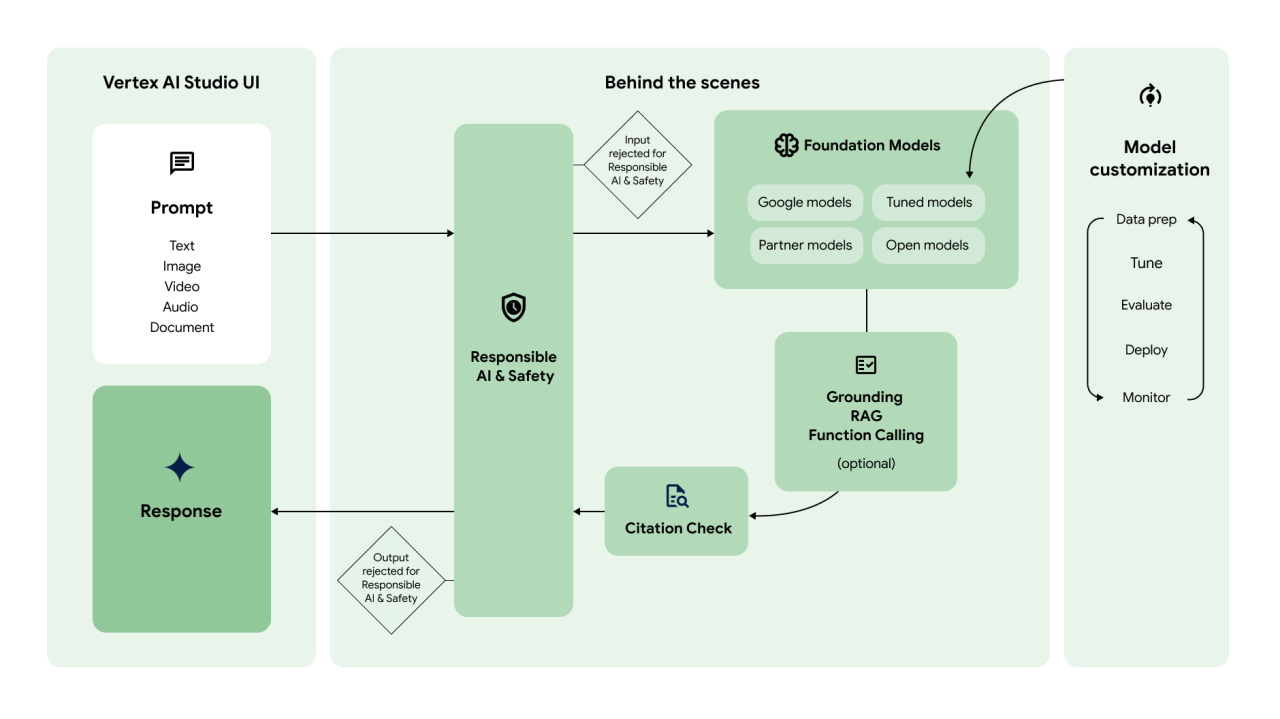
Source: google.com
The integration of Google Cloud and generative AI presents an unprecedented opportunity for community health systems to enhance patient care, optimize operations, and navigate the complexities of modern healthcare. While security and ethical considerations are paramount, the potential benefits – from predictive analytics and personalized medicine to streamlined workflows and improved patient engagement – are transformative. The future of community health systems is undeniably intertwined with the responsible and strategic implementation of these powerful technologies, promising a more efficient, effective, and patient-centric healthcare landscape.
FAQ Overview
What are the biggest security risks associated with using generative AI in healthcare?
Data breaches, unauthorized access to sensitive patient information, and the potential for AI bias leading to unfair or inaccurate diagnoses are major concerns. Robust security measures and adherence to regulations like HIPAA are crucial.
How can community health systems ensure data privacy when using generative AI?
Implementing strong data encryption, access control measures, and regular security audits are essential. Data anonymization techniques and compliance with relevant regulations are also vital.
What is the return on investment (ROI) for implementing generative AI in a community health system?
ROI varies greatly depending on implementation and specific use cases. Potential benefits include reduced administrative costs, improved patient outcomes leading to reduced readmissions, and enhanced operational efficiency. A thorough cost-benefit analysis is necessary before implementation.
What training is needed for staff to effectively use generative AI tools?
Training needs will vary based on the specific tools implemented. However, staff should receive training on data interpretation, ethical considerations, AI limitations, and the secure handling of patient data.