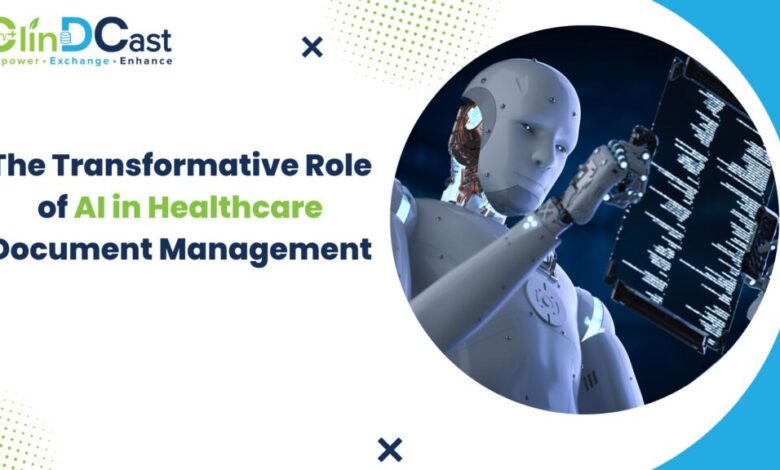
Could AI Increase Clinician Burden?
Could artificial intelligence increase clinician burden? It’s a question sparking heated debate in healthcare circles. While AI promises to revolutionize medicine with automated tasks and faster diagnoses, a crucial counterpoint emerges: will these advancements truly lighten the load on already overworked clinicians, or will they inadvertently add to their existing responsibilities? This post delves into the potential benefits and drawbacks of AI integration in healthcare, exploring whether AI is a helping hand or an extra burden for those on the front lines.
The integration of AI in healthcare is a double-edged sword. On one hand, AI-powered tools can automate administrative tasks, streamline data entry, and even assist with diagnosis and treatment planning, potentially freeing up valuable clinician time. On the other hand, the need to learn new technologies, manage AI-generated data, and address potential ethical concerns could lead to increased workload and stress.
The key lies in responsible implementation and a careful consideration of the potential impact on clinicians’ workflows and well-being.
AI-Driven Automation & Clinician Workflow
The increasing administrative burden on clinicians is a significant concern in healthcare. AI offers a powerful solution by automating many time-consuming tasks, allowing clinicians to focus more on patient care. This section explores how AI can revolutionize clinician workflows, improving efficiency and job satisfaction.
AI Automation of Administrative Tasks
AI-powered tools can significantly reduce the time clinicians spend on administrative tasks. This includes everything from scheduling appointments and managing patient records to generating reports and billing. By automating these processes, clinicians can dedicate more time to direct patient interaction and complex medical decision-making. The following table illustrates the potential time savings:
Task | Current Time (minutes) | AI-Assisted Time (minutes) | Time Saved (minutes) |
---|---|---|---|
Charting a patient visit | 30 | 10 | 20 |
Scheduling appointments for a week | 60 | 15 | 45 |
Generating patient reports | 45 | 5 | 40 |
Processing insurance claims for one patient | 20 | 2 | 18 |
AI Streamlining Data Entry and Record-Keeping
Manual data entry and record-keeping are notoriously time-consuming and prone to errors. AI can streamline this process through several applications. Natural Language Processing (NLP) can automatically transcribe dictated notes and convert unstructured data into structured formats suitable for electronic health records (EHRs). Machine learning algorithms can identify missing data points and flag potential inconsistencies, improving data quality and reducing the risk of errors.
For example, an AI system could automatically extract vital signs from a patient’s chart and populate the EHR, eliminating the need for manual data entry. Another example would be an AI system that flags potential medication interactions based on a patient’s medical history and current prescriptions.
AI Impact on Appointment Scheduling and Patient Communication
AI can optimize appointment scheduling by analyzing clinician availability, patient preferences, and appointment durations. This leads to better utilization of clinician time and reduces patient wait times. AI-powered chatbots can handle routine patient inquiries, such as appointment reminders, prescription refills, and basic medical advice, freeing up clinicians to address more complex issues. For instance, an AI chatbot could automatically send appointment reminders to patients via text message or email, reducing the number of missed appointments and improving clinic efficiency.
Furthermore, AI can personalize patient communication by tailoring messages to individual needs and preferences, improving patient engagement and satisfaction.
AI-Assisted Diagnosis & Treatment Planning: Could Artificial Intelligence Increase Clinician Burden
The integration of artificial intelligence (AI) into healthcare promises to revolutionize how clinicians approach diagnosis and treatment planning. By automating tedious tasks and providing data-driven insights, AI has the potential to significantly reduce clinician workload, improve diagnostic accuracy, and ultimately enhance patient care. This section explores the specific ways AI can assist in these crucial aspects of medical practice.AI’s role in diagnosis and treatment planning is not about replacing clinicians, but rather augmenting their capabilities.
It acts as a powerful tool, providing clinicians with the information they need to make faster, more informed decisions. This ultimately frees up their time to focus on patient interaction and complex cases requiring human expertise.
So, could AI actually increase clinician burden? It’s a complex question. While AI could automate tasks, the implementation and oversight often require significant clinician time. Consider this: Kaiser Permanente’s decision to nix a $500M Seattle bed tower, as reported in this article , highlights resource constraints. If hospitals are cutting back on beds, the pressure on existing clinicians to manage increased patient loads might make AI integration a frustrating, rather than helpful, addition.
Improved Diagnostic Accuracy and Speed
AI algorithms can analyze medical images (X-rays, CT scans, MRIs) and patient data far more quickly than a human clinician, potentially identifying subtle anomalies that might be missed. This speed and accuracy translate directly into reduced clinician workload.
- Faster review of test results: AI can automatically flag critical findings in lab results, imaging scans, and other diagnostic tests, prioritizing those requiring immediate attention and allowing clinicians to focus on the most urgent cases.
- Increased diagnostic accuracy: AI algorithms, trained on vast datasets of medical images and patient records, can identify patterns and subtle indicators of disease that might be missed by the human eye, leading to earlier and more accurate diagnoses.
- Reduced diagnostic errors: By providing a second opinion and highlighting potential diagnostic pitfalls, AI can help clinicians avoid errors and improve the overall accuracy of diagnoses. For example, in radiology, AI can assist in identifying subtle fractures or tumors that might be easily overlooked by a human radiologist.
AI Assistance in Treatment Planning
Beyond diagnosis, AI can significantly contribute to treatment planning by providing evidence-based recommendations and personalized treatment options. This reduces the time clinicians spend on literature reviews and allows for more tailored approaches to patient care.AI systems can access and process vast amounts of clinical trial data, research papers, and patient records to identify the most effective treatment strategies for specific conditions and patient profiles.
This personalized approach ensures that patients receive the most appropriate and effective treatment, minimizing the risk of adverse effects and maximizing the chances of a successful outcome. For instance, in oncology, AI can analyze a patient’s tumor characteristics and genetic profile to recommend the most effective chemotherapy regimen, minimizing trial-and-error approaches.
Differential Diagnosis and Clinician Decision-Making
AI’s application in differential diagnosis – the process of identifying the most likely diagnosis from a list of possibilities – offers both advantages and drawbacks.
- Benefits: AI can analyze patient symptoms, medical history, and test results to generate a ranked list of potential diagnoses, significantly narrowing down the possibilities and guiding clinician decision-making. This reduces the time spent considering various diagnostic possibilities and improves diagnostic efficiency.
- Drawbacks: AI algorithms are only as good as the data they are trained on. Biases in the training data can lead to inaccurate or biased diagnoses. Furthermore, AI cannot replace the clinician’s judgment and clinical experience, especially in complex or unusual cases. The clinician retains ultimate responsibility for the final diagnosis and treatment plan.
AI’s Role in Patient Monitoring & Management
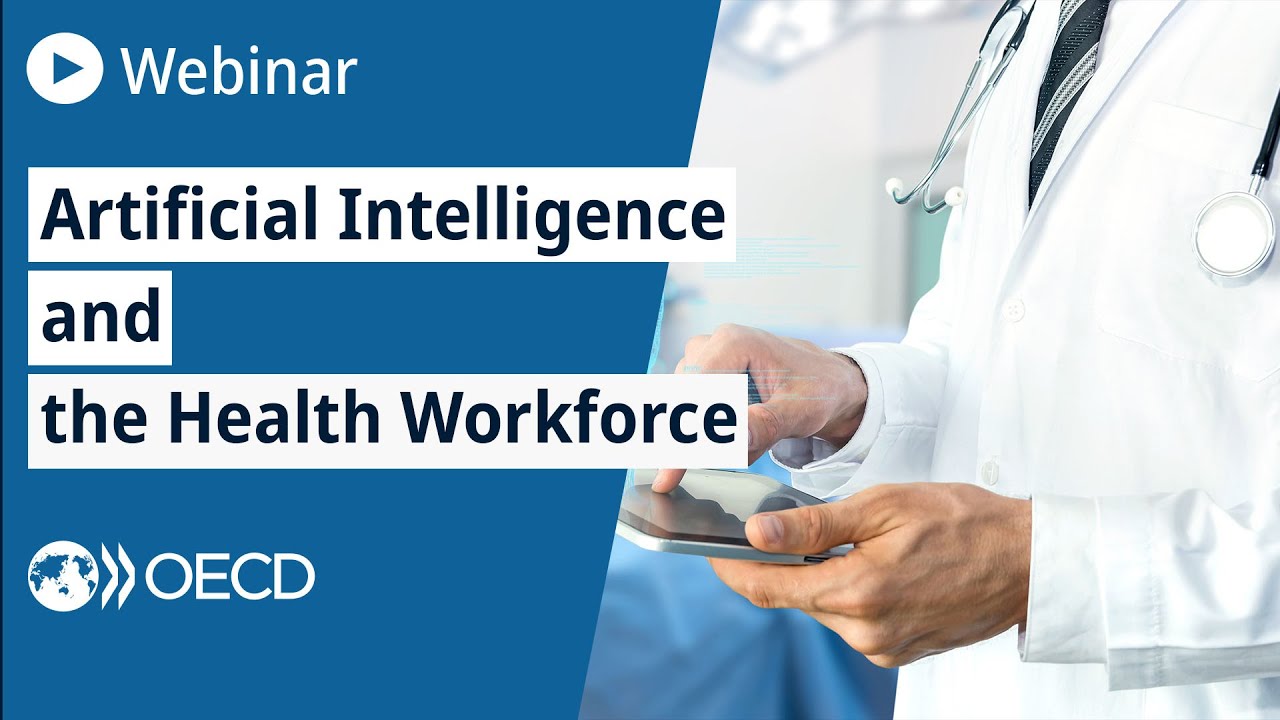
Source: ytimg.com
AI is rapidly transforming healthcare, and its impact on patient monitoring and management is particularly profound. By automating tasks, providing real-time insights, and enabling proactive interventions, AI has the potential to significantly reduce clinician burden and improve patient outcomes. This shift towards AI-powered patient care promises a more efficient and effective healthcare system.AI’s application in remote patient monitoring offers several avenues for reducing the need for in-person consultations.
This technology empowers patients to actively participate in their care, leading to improved adherence to treatment plans and better overall health management. Simultaneously, it frees up clinicians’ time, allowing them to focus on more complex cases.
Remote Patient Monitoring Applications
The use of wearable sensors and connected devices allows for continuous monitoring of vital signs such as heart rate, blood pressure, and blood glucose levels. This data is then transmitted to a secure platform where AI algorithms analyze it, identifying potential issues before they escalate. For example, an AI system could detect an irregular heartbeat pattern in a patient with a history of heart disease, prompting an alert to the patient’s physician.
This proactive approach can prevent serious complications and reduce the need for emergency room visits. Further, remote monitoring via telehealth platforms allows for virtual check-ins with patients, addressing minor concerns and reducing the need for unnecessary office visits. This can be especially beneficial for patients with chronic conditions who require frequent monitoring. Imagine a scenario where a patient with diabetes can track their blood glucose levels at home, and the AI system automatically alerts their doctor if levels fall outside of a safe range.
This avoids a potentially urgent in-person visit, while still allowing for timely intervention.
AI-Powered Proactive Risk Identification
AI algorithms can analyze large datasets of patient information – including medical history, lab results, and lifestyle factors – to identify individuals at high risk of deterioration or hospital readmission. For instance, an AI system could analyze a patient’s post-discharge data to predict their likelihood of readmission within 30 days. This allows clinicians to proactively intervene with support services, medication adjustments, or follow-up appointments, preventing unnecessary hospitalizations and reducing the strain on hospital resources.
The Mayo Clinic, for example, has implemented AI-powered tools that predict the risk of sepsis, enabling early intervention and improving patient outcomes. These tools significantly improve efficiency by flagging high-risk patients, enabling clinicians to prioritize their attention where it is most needed.
AI Support for Chronic Disease Management
Consider a hypothetical scenario involving a patient with hypertension. Currently, managing hypertension often requires regular in-person visits to the doctor for blood pressure checks and medication adjustments. However, with AI-powered remote monitoring, the patient could wear a device that continuously monitors their blood pressure. The AI system could analyze this data, automatically adjusting medication dosages as needed within pre-defined parameters.
The system would only alert the physician in case of significant deviations or if the medication adjustments reach pre-set limits, requiring a clinician’s review and potential intervention. This reduces the frequency of necessary in-person visits, freeing up both the patient’s and the clinician’s time. This approach not only improves the patient’s quality of life but also significantly reduces the clinician’s workload associated with routine hypertension management.
AI’s Impact on Clinician Training & Education
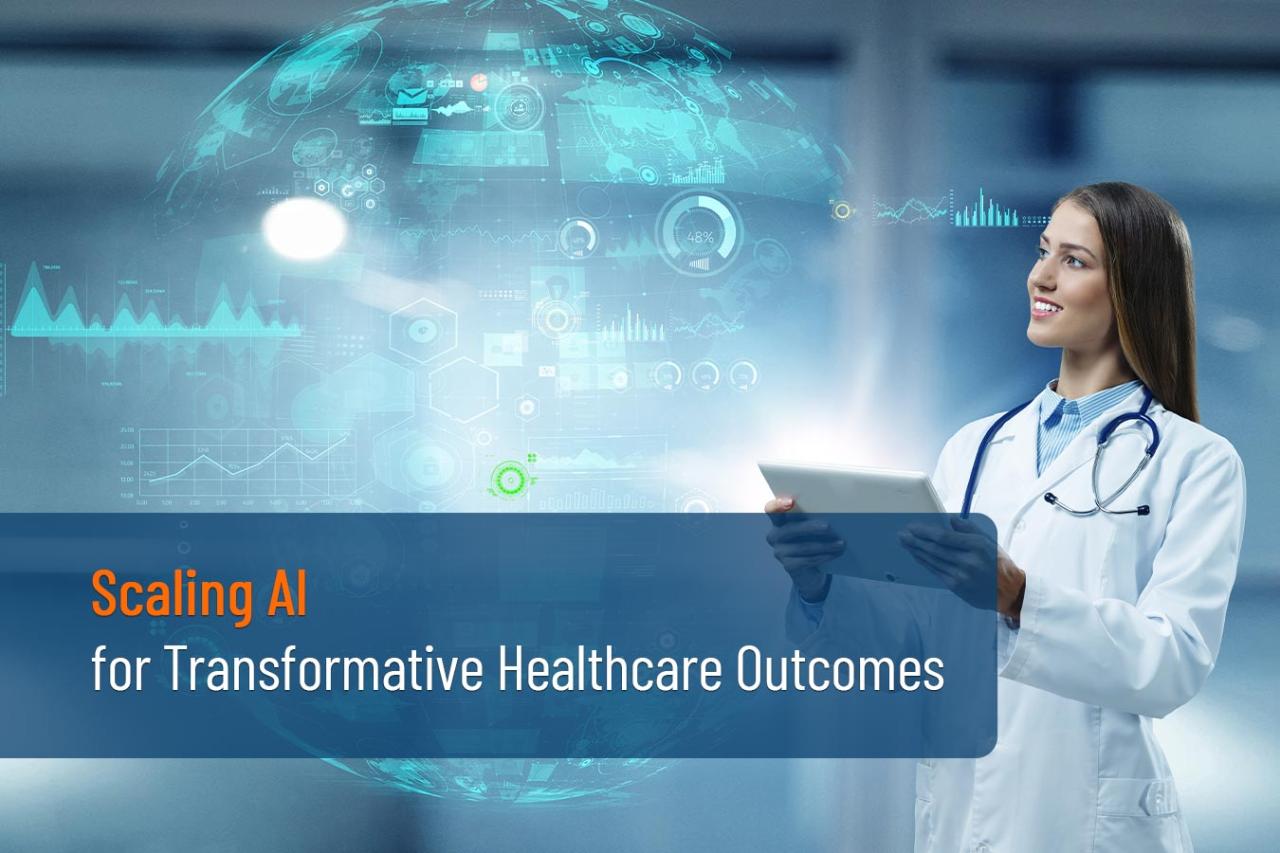
Source: evoluteiq.com
The integration of artificial intelligence (AI) into healthcare is rapidly transforming not only patient care but also the very way clinicians are trained and educated. AI-powered tools offer the potential to revolutionize medical education, leading to more efficient learning, improved knowledge retention, and ultimately, better patient outcomes. This shift promises a more personalized and effective approach to clinician development, addressing the evolving needs of a complex and rapidly advancing medical landscape.AI-powered educational tools can significantly enhance clinician training and improve knowledge retention by offering several key advantages.
I’ve been thinking a lot lately about how AI could actually increase clinician burden, ironically, by creating more administrative tasks and data analysis needs. The news that Robert F. Kennedy Jr. cleared a key hurdle on his path to becoming HHS Secretary, as reported in this article , makes me wonder even more about the future of healthcare tech.
Will his policies prioritize effective AI integration or just add to the already overflowing plate of healthcare professionals? Ultimately, careful implementation is key to avoiding an AI-induced administrative nightmare for clinicians.
These tools can provide interactive simulations of complex medical scenarios, allowing trainees to practice diagnosis and treatment in a risk-free environment. They can also offer personalized feedback, highlighting areas where improvement is needed and providing targeted guidance. Furthermore, AI can curate vast amounts of medical literature and research, making it readily accessible to clinicians at all stages of their training.
This curated information, delivered in a digestible format, can significantly boost learning efficiency and ensure clinicians are up-to-date with the latest advancements in their field.
AI-Enhanced Personalization of Clinician Learning
AI algorithms can analyze a clinician’s learning style, strengths, and weaknesses to personalize their training experience. This personalized approach ensures that learning materials are tailored to individual needs, maximizing knowledge acquisition and minimizing wasted effort. For instance, an AI system could identify a trainee who struggles with interpreting complex diagnostic imaging and provide them with additional practice exercises and targeted tutorials focusing on that specific skill.
Conversely, a trainee demonstrating proficiency in a particular area could be presented with more challenging material to further develop their expertise. This adaptive learning approach ensures that each clinician receives the optimal level of support and challenge, fostering a more effective and engaging learning journey. This contrasts sharply with traditional methods which often deliver a one-size-fits-all approach, potentially leaving some trainees behind or others unchallenged.
Comparison of Traditional and AI-Enhanced Clinician Training Methods
The following table compares traditional methods of clinician training with AI-enhanced methods, highlighting differences in efficiency, effectiveness, and cost.
Method | Time Commitment | Effectiveness | Cost |
---|---|---|---|
Traditional Lectures & Case Studies | High; often requires significant time in classrooms and practical sessions. | Moderate; effectiveness varies depending on individual learning styles and instructor quality. | Moderate to High; includes costs associated with faculty, facilities, and materials. |
AI-Powered Simulations & Interactive Learning Platforms | Can be flexible; allows for self-paced learning and focused practice. | High; personalized feedback and adaptive learning pathways enhance knowledge retention and skill development. | Moderate; initial investment in software and technology may be required, but long-term cost savings can be achieved through increased efficiency. |
Traditional Textbook Learning | High; requires significant independent study and self-directed learning. | Moderate; relies heavily on self-motivation and effective study habits. | Moderate; costs associated with purchasing textbooks and other learning materials. |
AI-Curated Medical Literature & Knowledge Bases | Moderate; allows for efficient access to relevant information and reduces time spent searching for resources. | High; ensures clinicians have access to the most up-to-date and relevant information. | Low to Moderate; subscription costs for AI-powered knowledge bases may be incurred. |
Ethical and Practical Challenges of AI Implementation
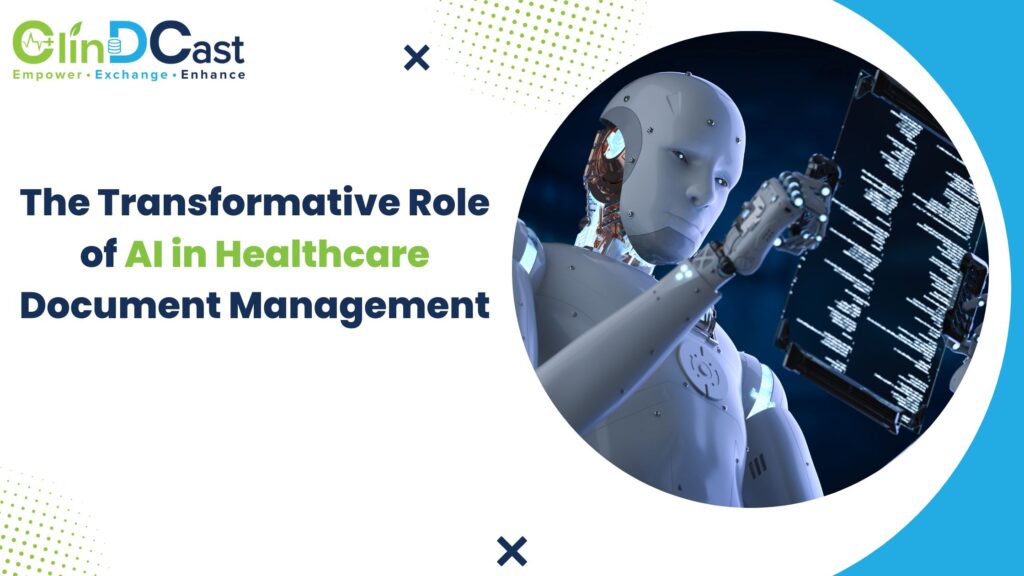
Source: clindcast.com
The integration of artificial intelligence (AI) into healthcare holds immense promise, but its implementation is not without significant ethical and practical hurdles. Successfully navigating these challenges is crucial to ensure AI benefits all patients and doesn’t worsen existing inequalities within the healthcare system. Addressing these concerns proactively is paramount to realizing AI’s full potential in improving healthcare delivery.
I’ve been thinking a lot lately about how AI could actually increase clinician burden, ironically. While it promises efficiency, the implementation and oversight required are substantial. This is further complicated by the recent Supreme Court decision, as reported in this article: scotus overturns chevron doctrine healthcare , which could significantly alter regulatory pathways for AI in healthcare.
The potential for increased administrative tasks related to compliance adds another layer to the already complex question of AI’s impact on clinician workload.
AI and Health Disparities
The potential for AI to exacerbate existing health disparities is a serious concern. Bias in algorithms, stemming from biased training data, can lead to inaccurate or discriminatory outcomes for certain patient populations. For example, an AI system trained primarily on data from one demographic group might perform poorly when applied to patients from a different background. Mitigating these risks requires a multi-pronged approach.
- Diverse and Representative Datasets: Ensuring AI training datasets accurately reflect the diversity of the patient population is critical. This involves actively collecting data from underrepresented groups and carefully auditing datasets for bias.
- Algorithmic Transparency and Explainability: Understanding how an AI system arrives at its conclusions is vital for identifying and correcting biases. “Explainable AI” (XAI) techniques are being developed to make AI decision-making more transparent.
- Continuous Monitoring and Evaluation: Regularly assessing the performance of AI systems across different demographic groups is crucial to detect and address any emerging disparities. This includes tracking metrics like accuracy, sensitivity, and specificity for various subgroups.
- Equitable Access to AI-Powered Tools: Ensuring that all patients, regardless of socioeconomic status or geographic location, have equal access to AI-powered healthcare tools is essential to prevent the creation of a two-tiered system.
Data Privacy and Security in AI Healthcare, Could artificial intelligence increase clinician burden
The use of AI in healthcare necessitates the collection and analysis of vast amounts of sensitive patient data. Protecting this data from unauthorized access and misuse is paramount. Breaches can have devastating consequences, both for individuals and for the trust placed in healthcare systems.
- Robust Data Encryption and Anonymization: Employing strong encryption methods to protect data at rest and in transit is crucial. Anonymization techniques can help to remove or mask identifying information while preserving the utility of the data for AI training and analysis.
- Strict Access Control and Authentication: Implementing rigorous access control measures, including multi-factor authentication, ensures that only authorized personnel can access sensitive patient data.
- Compliance with Data Privacy Regulations: Adhering to relevant data privacy regulations, such as HIPAA in the United States and GDPR in Europe, is essential. This includes obtaining informed consent from patients before collecting and using their data.
- Regular Security Audits and Penetration Testing: Conducting regular security audits and penetration testing helps identify vulnerabilities in data security systems and allows for proactive mitigation of potential threats.
Integrating AI into Clinical Workflows
Successfully integrating AI tools into existing clinical workflows requires careful planning and consideration. AI systems should be designed to complement, not replace, clinicians, and the implementation process should be tailored to the specific needs and capabilities of the healthcare organization.
- User-Friendly Interfaces: AI tools should be designed with intuitive interfaces that are easy for clinicians to use and understand, minimizing disruption to their existing workflows.
- Effective Training and Support: Providing clinicians with adequate training and ongoing support is crucial to ensure successful adoption of AI tools. This includes hands-on training, troubleshooting assistance, and access to expert consultation.
- Phased Implementation: Implementing AI tools in a phased approach, starting with a pilot program in a limited setting, allows for iterative improvement and reduces the risk of widespread disruption.
- Workflow Optimization: Careful consideration should be given to how AI tools can be integrated into existing workflows to maximize efficiency and minimize disruption. This might involve redesigning certain aspects of the workflow to accommodate the new technology.
Concluding Remarks
Ultimately, the question of whether AI will increase clinician burden isn’t a simple yes or no. The answer hinges on thoughtful implementation, robust training programs, and a proactive approach to addressing ethical and practical challenges. While AI offers incredible potential to improve healthcare efficiency and patient outcomes, its success depends on prioritizing the needs and well-being of the clinicians who are essential to its effective use.
Let’s strive to harness AI’s power to truly support, not overwhelm, our healthcare professionals.
Query Resolution
What are the biggest concerns about AI data privacy in healthcare?
Maintaining patient confidentiality and complying with regulations like HIPAA are paramount. AI systems must be designed with robust security measures to prevent data breaches and unauthorized access.
How can hospitals effectively train clinicians on new AI tools?
Effective training requires a multi-faceted approach, including hands-on workshops, online modules, mentorship programs, and ongoing support from IT staff. Tailoring training to individual needs and learning styles is crucial.
Will AI replace clinicians entirely?
No. AI is designed to augment, not replace, clinicians. It’s a tool to improve efficiency and accuracy, allowing clinicians to focus on patient interaction and complex decision-making.