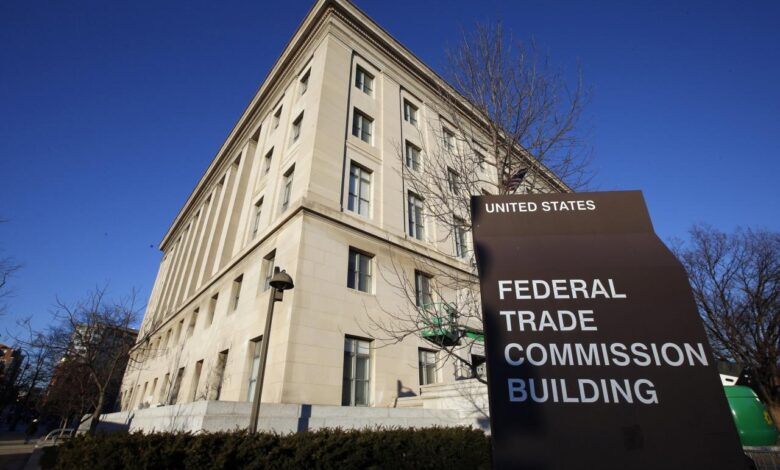
Texas Pharmacy Benefit Manager Pharma Company Lawsuit Insulin Prices
Texas pharmacy benefit manager pharma company lawsuit insulin prices: The soaring cost of insulin has become a crisis for many Texans, and this massive lawsuit aims to address the alleged price-gouging by pharmaceutical companies and pharmacy benefit managers (PBMs). This case could reshape the healthcare landscape in Texas and potentially nationwide, impacting millions of people living with diabetes.
We’ll delve into the specifics of the lawsuit, the impact on patients, and what the future might hold for insulin affordability.
This blog post will explore the complex web of players involved – from the PBMs negotiating drug prices to the pharmaceutical companies setting them, and the devastating consequences for Texans struggling to afford life-saving medication. We’ll examine the legal arguments, the potential outcomes, and explore what policy changes might offer a path towards more affordable insulin.
Texas Pharmacy Benefit Managers (PBMs)
Pharmacy Benefit Managers (PBMs) play a significant role in the Texas healthcare system, acting as intermediaries between pharmaceutical companies, insurance providers, and patients. They manage prescription drug benefits for insurance plans, negotiating drug prices, processing claims, and overseeing the distribution of medications. Their influence on the cost of prescription drugs, particularly insulin, is substantial and has become a focal point of recent debate.PBMs in Texas utilize various business models, often a combination of approaches.
Some operate as independent entities negotiating directly with pharmacies and manufacturers, while others are subsidiaries of large insurance companies or health systems, integrating their PBM operations vertically. These different structures can influence their negotiation strategies and impact on drug pricing.
PBM Negotiation Strategies and Drug Pricing
PBMs employ various tactics to negotiate lower drug prices from pharmaceutical companies. These include leveraging their large purchasing volume to secure rebates and discounts, utilizing formularies (lists of approved drugs) to incentivize manufacturers to offer lower prices for preferred drugs, and employing strategies like “spread pricing,” where they collect rebates from manufacturers but only partially pass those savings onto the plan sponsor or patient.
For example, a PBM might negotiate a rebate of $10 per insulin vial from a manufacturer, but only pass on a $2 discount to the patient, pocketing the remaining $8. This practice, while legal, has been heavily criticized for its opacity and potential for profit maximization at the expense of patients.
Impact of PBM Practices on Insulin Pricing in Texas
The impact of PBM practices on insulin pricing in Texas is complex and multifaceted. While PBMs claim to negotiate lower prices, their complex rebate and pricing structures often obscure the actual cost savings passed on to patients. The high cost of insulin remains a significant burden for many Texans, and the role of PBMs in this issue is a subject of ongoing scrutiny.
For instance, while a PBM may negotiate a lower wholesale price for insulin, their administrative fees and other charges might offset these savings, resulting in little to no reduction in the patient’s out-of-pocket expenses. Furthermore, the use of formularies can restrict patient access to specific insulin products, potentially limiting their choices and increasing their costs if their preferred insulin isn’t on the formulary.
The lack of transparency in PBM pricing practices makes it difficult to definitively assess their overall impact on insulin affordability for Texans.
The Lawsuit
The Texas lawsuit against pharmaceutical companies and Pharmacy Benefit Managers (PBMs) regarding insulin pricing is a complex legal battle with significant implications for patients and the healthcare system. This case alleges a coordinated effort to artificially inflate insulin prices, leaving many diabetics struggling to afford this life-saving medication. The lawsuit aims to hold accountable those responsible for this alleged price gouging and to seek redress for the harm inflicted on consumers.
Plaintiffs and Defendants
The plaintiffs in this lawsuit are a group of Texas residents diagnosed with diabetes who have directly experienced the financial burden of high insulin costs. They represent a larger class of individuals facing similar challenges. The defendants include several major pharmaceutical companies that manufacture insulin and several prominent Pharmacy Benefit Managers (PBMs) that manage prescription drug benefits for many insurance plans.
Specific company names would need to be included here based on the actual lawsuit filings, but for illustrative purposes, we can imagine companies like Eli Lilly, Novo Nordisk, Sanofi, and major PBMs like CVS Caremark, Express Scripts, and OptumRx being involved. The exact lineup of plaintiffs and defendants will vary depending on the specific case.
Allegations of Price Manipulation
The core allegation of the lawsuit centers on the claim that pharmaceutical companies and PBMs have engaged in anti-competitive practices to artificially inflate insulin prices. This involves several potential mechanisms. The lawsuit likely alleges that the pharmaceutical companies have engaged in practices to limit generic competition, such as patent extensions or other strategies to maintain market control. Simultaneously, the lawsuit may claim that PBMs, while ostensibly negotiating lower drug prices on behalf of insurers, have engaged in “rebate” systems that ultimately benefit the manufacturers, resulting in higher prices for consumers despite the appearance of negotiation.
The argument is that this system allows for the appearance of price competition while actually suppressing true cost reductions.
Specific Claims Regarding Insulin Prices
The lawsuit likely details specific examples of how insulin prices have increased dramatically over the years, far exceeding the rate of inflation and the cost of manufacturing. It will likely present data comparing the prices of insulin in the United States with those in other developed countries, highlighting the disproportionately high cost in the US. Furthermore, it will probably include evidence of internal company communications, financial documents, and expert testimony to support the claim of intentional price manipulation.
For instance, the lawsuit could point to specific instances where a PBM received a rebate from a manufacturer that was greater than the apparent price reduction passed on to the consumer, demonstrating a lack of genuine price competition.
Legal Arguments Presented
The plaintiffs’ legal arguments will center on violations of antitrust laws, alleging that the defendants engaged in price-fixing and other anti-competitive practices. They will argue that the defendants’ actions have resulted in an unreasonable increase in insulin prices, causing significant harm to consumers. The defendants, on the other hand, will likely argue that their pricing practices are legitimate business decisions driven by research and development costs, marketing expenses, and the complexities of the pharmaceutical market.
They may contest the plaintiffs’ claims of anti-competitive behavior, arguing that market forces and innovation justify the pricing strategies employed. The legal battle will likely revolve around the interpretation of antitrust laws and the evidence presented regarding the defendants’ intent and the impact of their actions on insulin pricing.
Impact of Insulin Pricing on Texas Residents
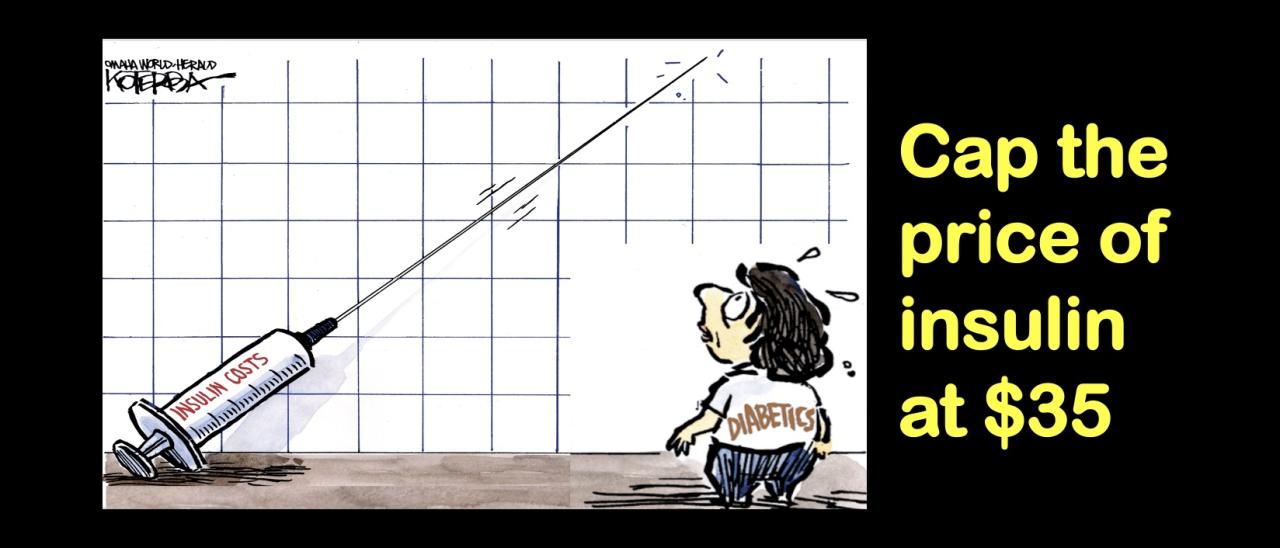
Source: thedemlabs.org
The exorbitant cost of insulin has created a critical health crisis in Texas, disproportionately affecting vulnerable populations and placing an undue burden on families already struggling to make ends meet. The lack of price regulation and the complex interplay of pharmaceutical companies, pharmacy benefit managers (PBMs), and insurance providers contribute to this ongoing problem. Understanding the scope of this issue requires examining its impact on Texans’ health and finances.The high cost of insulin in Texas directly impacts a significant portion of the population.
According to the Centers for Disease Control and Prevention (CDC), millions of Texans live with diabetes, a substantial number of whom require insulin for survival. While precise figures on insulin-dependent diabetics in Texas are difficult to obtain due to data limitations and variations in reporting, the number is undeniably large and represents a considerable portion of the state’s population.
This translates to a massive number of individuals and families directly affected by the price hikes.
The Texas pharmacy benefit manager lawsuit targeting inflated insulin prices got me thinking about market control. It’s all about who holds the power, and this reminds me of the FTC’s recent lawsuit against the Novant Health and Community Health Systems merger, which you can read about here: federal trade commission sues block novant health community health systems hospital acquisition.
Both cases highlight how unchecked consolidation can lead to higher prices for consumers, whether it’s life-saving insulin or healthcare services.
Financial Burden of High Insulin Costs on Texas Families
The financial burden imposed by high insulin prices is staggering for many Texas families. The cost of insulin can range from hundreds to thousands of dollars per month, depending on the type and dosage required. For individuals without comprehensive insurance coverage or those with high deductibles and co-pays, the cost of insulin can quickly become insurmountable, forcing difficult choices between paying for medication and meeting other essential needs like food and housing.
This often leads to individuals skipping doses or rationing their insulin, resulting in severe health consequences. Stories abound of Texans selling possessions, taking on debt, or going without necessary insulin to make ends meet.
Health Consequences of Insulin Rationing
Rationing insulin due to cost has severe and potentially life-threatening consequences. Insulin deficiency can lead to hyperglycemia (high blood sugar), causing a cascade of complications including diabetic ketoacidosis (DKA), a potentially fatal condition. Long-term consequences of poorly managed diabetes due to insulin rationing include kidney disease, blindness, nerve damage, heart disease, and stroke. The impact extends beyond the individual, affecting families and the healthcare system as a whole through increased hospitalizations and long-term care costs.
The human cost of this crisis is immeasurable.
Comparison of Insulin Costs Across States
It’s important to understand how insulin prices in Texas compare to other states. Gathering precise, up-to-the-minute pricing data across all states is challenging due to variations in insurance plans, formularies, and drug pricing strategies. However, numerous reports and studies have consistently shown significant price discrepancies between states. The following table offers a generalized comparison, acknowledging that actual costs vary widely:
State | Average Monthly Cost (Estimate) | Notes | Data Source (Illustrative) |
---|---|---|---|
Texas | $300 – $500 | Wide range due to varying insurance plans and drug formularies. | Various news reports and patient testimonials |
California | $250 – $400 | State regulations may contribute to lower costs in some instances. | State-level health data publications (Illustrative) |
Florida | $350 – $600 | High cost due to a combination of factors including high demand and limited price regulation. | Patient advocacy group reports (Illustrative) |
New York | $200 – $450 | State-level initiatives may influence pricing. | News articles and policy analyses (Illustrative) |
*Note: The cost figures in this table are estimates based on publicly available information and should be considered illustrative rather than definitive. Actual costs vary significantly based on several factors.*
The Texas pharmacy benefit manager lawsuit targeting inflated insulin prices highlights a larger healthcare crisis. Accurate and timely medical billing is crucial for fair pricing, and the current system is strained by a shortage of medical coders. Addressing this bottleneck is vital, which is why I was so interested in learning about the ai powered solution to the medical coding worker shortage ; hopefully, solutions like this can help keep healthcare costs down and ensure everyone has access to affordable medication, including insulin, especially given the ongoing legal battles.
Regulatory Oversight and Policy Implications
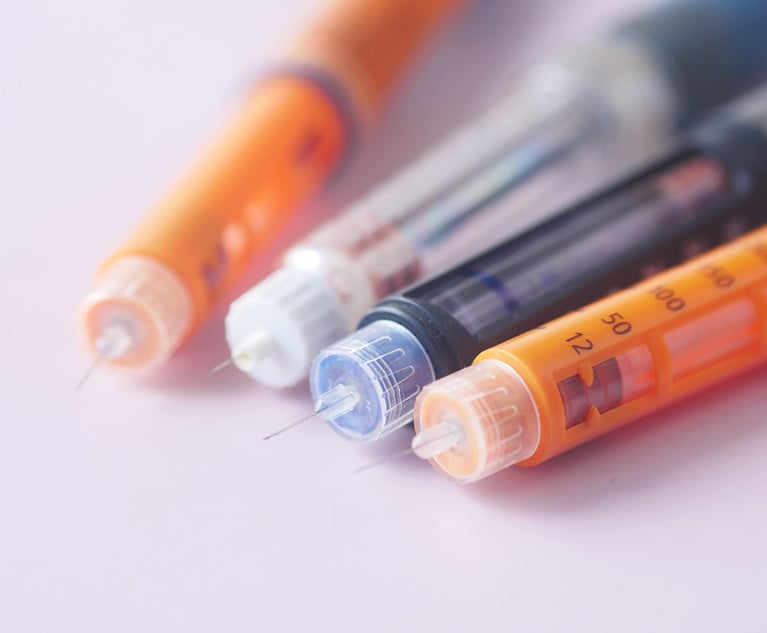
Source: law.com
The Texas lawsuit against pharmacy benefit managers (PBMs) regarding insulin pricing has significant implications for regulatory oversight and policy. The current regulatory landscape in Texas, while evolving, is insufficient to fully address the complexities of PBM practices and their impact on drug costs, particularly for essential medications like insulin. The outcome of this lawsuit could be a catalyst for stronger regulations and policy changes at both the state and federal levels.
Current PBM Regulations in Texas
Texas currently has some regulations governing PBM activities, primarily focusing on transparency and reporting requirements. These regulations often mandate PBMs to disclose certain information to pharmacies and the state, such as reimbursement rates and pharmacy network participation criteria. However, these regulations are often criticized for being insufficiently robust to prevent anti-competitive practices or address issues such as spread pricing (the difference between what a PBM pays a pharmacy and what it charges an insurer) and the use of rebates that may not fully benefit consumers.
The specifics of these regulations are constantly evolving and are subject to interpretation and enforcement challenges. For instance, while Texas may mandate disclosure of certain data, the format or level of detail may not always be sufficient for effective analysis or comparison.
Potential Impact of the Lawsuit on Future Regulations
The lawsuit’s outcome could significantly influence future regulations in Texas. A successful plaintiff’s case might lead to more stringent regulations on PBM practices, potentially including stricter controls on spread pricing, greater transparency regarding rebate negotiations, and enhanced oversight of pharmacy network adequacy. Conversely, a ruling in favor of the PBMs could embolden their current practices and potentially stifle future efforts to regulate their activities.
The precedent set by this case will likely impact other states considering similar legislative or regulatory actions. For example, if the lawsuit leads to a finding of anti-competitive behavior, it could encourage other states to strengthen their own anti-trust regulations regarding PBMs.
Policy Changes to Lower Insulin Prices
Several policy changes could help lower insulin prices in Texas and nationally. These include:
- Direct Negotiation: Allowing Medicare and Medicaid to directly negotiate drug prices with pharmaceutical manufacturers, as is currently done in many other developed countries, could significantly reduce costs. This approach bypasses the complex web of intermediaries, including PBMs, that currently influence pricing.
- Price Caps: Implementing price caps or other affordability measures specifically for insulin, similar to those adopted in some other states, could directly address the high cost of this life-saving medication. This would require careful consideration of the potential impact on pharmaceutical innovation and drug availability.
- Increased Transparency: Enhancing transparency throughout the drug pricing system, including requiring PBMs to disclose all fees and rebates, would allow for better scrutiny of pricing practices and potentially reduce the influence of opaque financial arrangements. This transparency would empower consumers and policymakers to make informed decisions.
State Approaches to Insulin Pricing Regulation
Different states have adopted varying approaches to regulate insulin pricing. Some states, such as Colorado and Illinois, have implemented price caps or affordability programs specifically targeting insulin. Other states have focused on increasing transparency or strengthening anti-trust enforcement related to PBM practices. The effectiveness of these different approaches varies, and there’s ongoing debate about the best regulatory strategy to control insulin costs.
For example, some price cap initiatives have faced legal challenges from pharmaceutical companies, highlighting the complexities and potential unintended consequences of such interventions. The ongoing experimentation with different regulatory models across states provides valuable data to inform future policy decisions.
Pharmaceutical Company Practices and Insulin Pricing
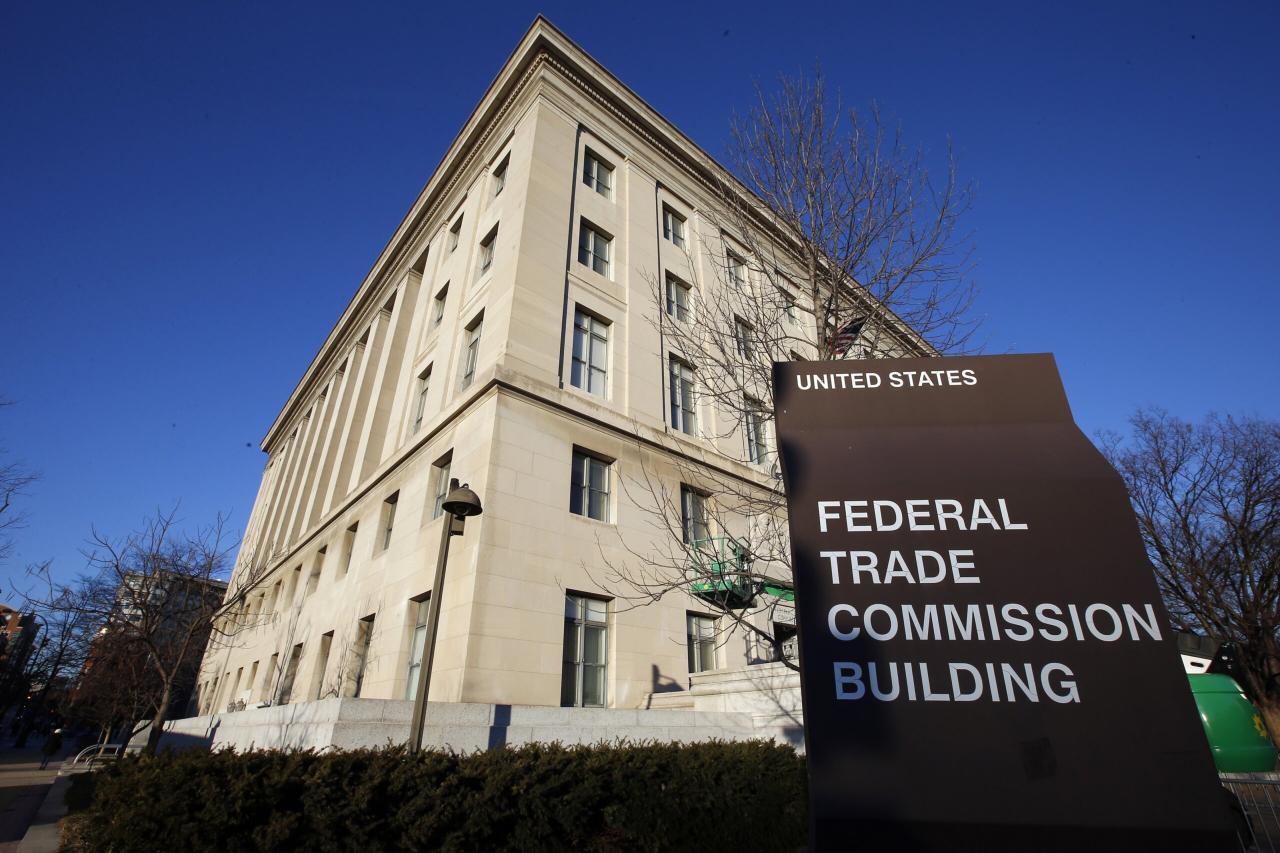
Source: wtop.com
The high cost of insulin is a complex issue stemming from a confluence of factors, including the pricing strategies of major insulin manufacturers, the role of patent protection, and the substantial research and development investments involved. Understanding these elements is crucial to addressing the affordability crisis faced by many insulin-dependent individuals.The pricing strategies employed by major insulin manufacturers are multifaceted and often opaque.
While they cite high R&D costs and the need for profit to fund future innovation, critics argue that these companies leverage their market dominance to maximize profits, particularly given the inelastic demand for a life-saving medication. Strategies include setting high list prices, negotiating rebates with pharmacy benefit managers (PBMs), and employing tiered pricing structures that often leave patients with significant out-of-pocket expenses.
This system, critics argue, creates an environment where the actual price paid by consumers bears little relation to the true cost of production.
Patent Protection and Insulin Pricing
Patent protection plays a significant role in shaping insulin pricing. Exclusive patent rights granted to pharmaceutical companies provide a period of market exclusivity, allowing them to set prices without direct competition from generic alternatives. This period, which can extend for years, allows manufacturers to recoup R&D investments and generate substantial profits. However, the length and scope of patent protection have been criticized for contributing to artificially inflated prices, especially considering that the core technology behind insulin production has been known for decades.
The extension of patents through minor modifications or formulation changes is another point of contention, further limiting competition and prolonging high prices.
Research and Development Costs in Insulin Production
The research and development costs associated with insulin production are substantial, involving complex processes of genetic engineering, manufacturing, and rigorous testing to ensure safety and efficacy. However, the actual costs are often difficult to ascertain due to the lack of transparency in the pharmaceutical industry’s accounting practices. While companies cite these high R&D costs as justification for high prices, critics argue that the actual cost of producing insulin itself is relatively low, with a significant portion of the price attributed to marketing, distribution, and profit margins.
Furthermore, the argument that new insulin analogs require substantial new R&D is contested, as many are incremental improvements on existing formulations.
Pricing Structures of Different Insulin Products
The following table illustrates the significant price variations among different insulin products. These prices are approximate and can vary based on factors like dosage, formulation, and payer contracts. It’s important to note that these prices represent list prices, and the actual cost to patients will depend on their insurance coverage and co-pay structure. The vast price differences highlight the lack of transparency and the significant impact on patients’ out-of-pocket costs.
Insulin Product | Approximate List Price (per vial) |
---|---|
Humalog (lispro) | $300 – $400 |
NovoLog (aspart) | $300 – $400 |
Lantus (glargine) | $300 – $400 |
Levemir (detemir) | $300 – $400 |
Generic Insulin (NPH) | $100 – $200 (but often not covered by insurance) |
Potential Outcomes of the Lawsuit
The Texas lawsuit against pharmacy benefit managers (PBMs) and pharmaceutical companies regarding insulin pricing holds significant implications for various stakeholders. The outcome could drastically reshape the insulin market in Texas and potentially serve as a precedent for similar legal challenges nationwide. The potential ramifications are far-reaching, affecting not only the financial standing of the involved corporations but also the accessibility and affordability of insulin for millions of Texans.The financial implications for the involved parties are substantial and multifaceted.
The Texas pharmacy benefit manager lawsuit against pharma companies over insulin prices got me thinking about the complexities of healthcare costs. It’s frustrating to see such vital medications priced so high, especially when considering the challenges faced by families managing conditions like Tourette Syndrome. Learning about effective strategies to manage Tourette syndrome in children highlighted the need for affordable healthcare access across the board, making the insulin price lawsuit even more urgent.
We need solutions that ensure everyone can access the medications they need, regardless of cost.
A ruling in favor of Texas could result in massive financial penalties for the defendant PBMs and pharmaceutical companies, potentially involving billions of dollars in fines and restitution. This could significantly impact their stock prices, profitability, and future investment strategies. Conversely, a loss for Texas could lead to substantial legal fees and a continued struggle to control insulin pricing within the state.
The financial burden of litigation itself is a considerable factor, regardless of the final verdict.
Financial Implications for Involved Parties
A favorable ruling for Texas could trigger a wave of similar lawsuits across the country, creating a domino effect of legal battles and potentially leading to significant industry-wide restructuring. Conversely, a dismissal of the case could embolden PBMs and pharmaceutical companies to maintain their current pricing strategies, potentially leading to further price increases and continued struggles for patients. The uncertainty surrounding the outcome makes it difficult to predict the precise financial impact, but the stakes are undeniably high for all parties involved.
For example, a significant fine levied against a major pharmaceutical company could force them to re-evaluate their pricing models, potentially leading to lower prices for other medications as well. Conversely, a victory for the defense could embolden other companies to continue current practices.
Impact on Future Insulin Pricing Practices
The lawsuit’s outcome will significantly influence future insulin pricing practices, potentially setting a new standard for transparency and regulation. A victory for Texas could lead to increased regulatory scrutiny of PBM practices, potentially resulting in greater price transparency and stricter regulations on rebates and discounts. This could translate to lower prices for consumers, although the extent of the price reduction remains uncertain.
Conversely, a loss for Texas could perpetuate the status quo, allowing PBMs and pharmaceutical companies to continue their existing pricing strategies, potentially leading to further price increases. The precedent set by this case could significantly shape future negotiations between PBMs, pharmaceutical companies, and state governments. For instance, a successful lawsuit could lead to state-level legislation mandating price caps or other regulatory measures aimed at controlling insulin costs.
Potential Legal Precedents
This lawsuit has the potential to establish significant legal precedents regarding the regulation of pharmaceutical pricing and PBM practices. A ruling in favor of Texas could establish a legal framework for holding PBMs accountable for their role in setting insulin prices, potentially opening the door for similar lawsuits in other states. This could lead to increased federal oversight of the pharmaceutical industry and greater transparency in pricing negotiations.
Conversely, a ruling against Texas could solidify the current legal landscape, potentially making it more difficult to challenge PBM practices in the future. The case’s outcome will influence future legal challenges and policy debates concerning drug pricing and healthcare access. The specific legal precedent set could significantly affect the future of healthcare legislation and regulation.
Impact on Healthcare Access in Texas
The lawsuit’s outcome will directly affect healthcare access for Texans with diabetes. A favorable ruling could lead to lower insulin prices, making the life-saving medication more affordable and accessible to a larger population. This could improve health outcomes and reduce the financial burden on individuals and families. Conversely, a loss for Texas could exacerbate existing problems, potentially leading to increased rates of diabetes-related complications due to reduced access to affordable insulin.
This scenario could disproportionately affect low-income individuals and families who already struggle to afford essential medications. For example, a significant price reduction could prevent individuals from rationing insulin, reducing the risk of severe health complications. Alternatively, a lack of change could lead to increased instances of rationing and subsequent health crises.
Illustrative Case Studies: Texas Pharmacy Benefit Manager Pharma Company Lawsuit Insulin Prices
This section delves into the lived experiences of Texans affected by the high cost of insulin, offering a glimpse into the human impact of the ongoing legal battle. We will examine the challenges faced by both patients and pharmacies navigating this complex landscape. We will also explore the internal pressures within PBMs that contribute to drug pricing decisions.
A Texan’s Struggle with Insulin Costs
Maria Rodriguez, a 58-year-old diabetic living in El Paso, Texas, has relied on insulin for over 20 years. Diagnosed with type 1 diabetes as a young adult, Maria has meticulously managed her condition, adhering to a strict diet and exercise regimen. However, the escalating cost of insulin has become an insurmountable burden. Her fixed income, supplemented by a part-time job, barely covers her rent and basic necessities.
The cost of her insulin has increased by over 50% in the last five years, forcing her to make difficult choices, such as skipping meals or forgoing necessary medications to afford her life-saving insulin. She has resorted to rationing her insulin, a dangerous practice that has resulted in several episodes of dangerously high blood sugar levels requiring emergency room visits, incurring further financial strain.
Her coping mechanisms include relying on support from a local diabetic support group and seeking assistance from charitable organizations, but these resources are often limited and insufficient to cover her ongoing insulin needs.
Challenges Faced by a Texas Pharmacy Dispensing Insulin, Texas pharmacy benefit manager pharma company lawsuit insulin prices
Garcia’s Pharmacy, a small, independent pharmacy in Austin, Texas, has witnessed firsthand the impact of rising insulin prices. The pharmacy owner, Ricardo Garcia, explains that the reimbursement rates from PBMs have not kept pace with the increasing cost of insulin from pharmaceutical companies. This disparity forces the pharmacy to absorb a significant portion of the cost, squeezing profit margins and threatening the pharmacy’s financial viability.
The pharmacy often faces difficult conversations with patients who cannot afford their insulin, sometimes resorting to offering discounts or working with patient assistance programs, which often have complex eligibility requirements and lengthy processing times. The constant pressure to maintain profitability while providing affordable access to essential medication creates immense stress for the pharmacy staff. They feel caught between the pharmaceutical companies setting high prices and the PBMs offering inadequate reimbursement.
Internal Pressures within a PBM Regarding Drug Pricing Negotiations
An anonymous employee working in the pricing department of a major Texas-based PBM describes the internal pressures surrounding drug pricing negotiations. The employee, who wishes to remain anonymous for fear of retaliation, explains that the primary goal is to negotiate the lowest possible price for drugs, including insulin, to maximize profits for the PBM. This often involves complex negotiations with pharmaceutical companies, balancing the need for cost-effective drugs with the potential for negative impacts on patient access.
The employee notes that internal pressures to meet financial targets can sometimes overshadow concerns about the affordability and accessibility of essential medications. The decision-making process is often influenced by complex algorithms and data analysis, which may not fully capture the human impact of drug pricing decisions. The employee expresses concern that the current system prioritizes profits over patient well-being, leading to ethically challenging situations.
Closure
The Texas pharmacy benefit manager pharma company lawsuit concerning insulin prices is far more than just a legal battle; it’s a fight for access to life-saving medication. The outcome will have profound implications for the affordability of insulin, not just in Texas, but potentially across the nation. While the legal process unfolds, it’s crucial to continue advocating for policies that prioritize patient access and affordability.
Stay tuned for updates as this critical case progresses.
Questions and Answers
What are Pharmacy Benefit Managers (PBMs)?
PBMs are third-party administrators that manage prescription drug benefits for insurance companies and employers. They negotiate drug prices with pharmaceutical companies and control which drugs are covered by insurance plans.
How does the lawsuit allege price manipulation?
The lawsuit alleges that PBMs and pharmaceutical companies colluded to artificially inflate insulin prices, limiting competition and exploiting patients’ dependence on this life-saving medication.
What are the potential penalties for the defendants if they lose?
Potential penalties could include substantial fines, changes to their business practices, and potentially even criminal charges depending on the specifics of the findings.
Are there any similar lawsuits in other states?
Yes, several other states have seen similar lawsuits alleging price-gouging in the pharmaceutical industry, particularly concerning insulin and other essential medications.