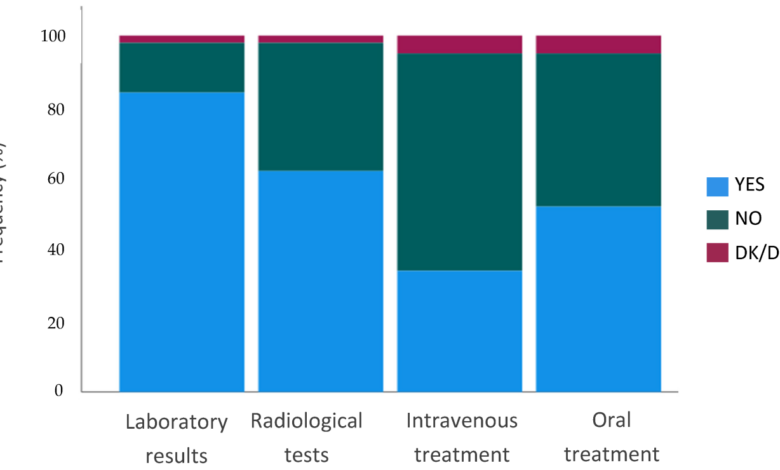
Fair Health Telehealth Monthly Tracker COVID Diagnoses
Fair Health telehealth monthly tracker COVID diagnoses: Wow, what a journey it’s been tracking the impact of telehealth on COVID-19 diagnoses! This data reveals fascinating trends, highlighting how virtual care adapted and responded to the pandemic’s ebb and flow. We’ll dive into the monthly ups and downs of telehealth usage, explore geographical variations, and even examine the accuracy of remote diagnoses.
Get ready for some surprising insights!
We’ll be exploring Fair Health’s data, looking at the sheer volume of telehealth visits related to COVID-19, the types of services offered, and the limitations of the data itself. Think of this as a deep dive into the numbers, revealing the story behind the headlines. We’ll analyze monthly trends, comparing COVID-19 telehealth usage to overall telehealth usage, and trying to pinpoint the reasons behind the fluctuations.
We’ll then map the geographical distribution of telehealth use, uncovering regional differences and exploring why some areas adopted virtual care more readily than others. Finally, we’ll look at the types of COVID-19 diagnoses made via telehealth, the accuracy of these diagnoses, and the impact of telehealth on patient outcomes.
Fair Health Telehealth Data Overview: Fair Health Telehealth Monthly Tracker COVID Diagnoses
Fair Health’s telehealth data offers a valuable glimpse into the utilization of telehealth services during the COVID-19 pandemic. This data, derived from private insurance claims, provides insights into trends in telehealth adoption, the types of services delivered remotely, and the overall impact of the pandemic on healthcare delivery. However, it’s crucial to understand the limitations of this dataset to interpret the findings accurately.Fair Health’s data on telehealth and COVID-19 diagnoses encompasses a significant portion of the private insurance market, providing a broad, albeit not exhaustive, picture of telehealth usage.
The data covers a substantial number of claims, allowing for the identification of trends and patterns related to telehealth utilization for COVID-19-related issues. This includes both diagnosis and treatment. The volume of data provides a strong foundation for analysis, though the data’s inherent limitations should be considered.
Telehealth Services Included in Fair Health Data
Fair Health’s dataset includes a wide range of telehealth services related to COVID-19 diagnoses. This includes virtual consultations, remote monitoring, and other digitally-enabled services used for diagnosis, treatment, and management of the virus and its associated conditions. Specific examples might include virtual check-ins with physicians for symptom monitoring, telehealth-based mental health counseling for patients experiencing COVID-related anxiety, or remote management of chronic conditions exacerbated by the virus.
The data likely doesn’t capture all aspects of telehealth usage, particularly those outside of private insurance coverage.
Tracking COVID diagnoses with the Fair Health telehealth monthly tracker has been eye-opening, highlighting the pandemic’s impact on healthcare access. The sheer volume of data underscores the need for innovative solutions, like those explored at the ai most exciting healthcare technology center connected medicine UPMC , which are pushing the boundaries of connected medicine. Understanding these advancements is crucial to interpreting the trends we see in the Fair Health data and improving future telehealth responses.
Limitations of Fair Health Telehealth Data
The Fair Health data, while extensive, has inherent limitations. Firstly, it only represents data from private insurance claims, excluding patients without private insurance or those utilizing public healthcare systems. This creates a bias towards a specific demographic and may not accurately reflect the overall telehealth usage across all populations. Secondly, the data might not capture all telehealth services, especially those provided outside of established billing practices or those not properly coded in claims.
Finally, the data’s accuracy relies on the accuracy of the coding and reporting practices of healthcare providers. Inconsistent or inaccurate coding could lead to misinterpretations of the data.
Tracking COVID diagnoses with the Fair Health telehealth monthly tracker has been a bit of a rollercoaster, especially with the fluctuating numbers. It’s made me think about the challenges of managing chronic conditions, and how vital consistent care is, much like the strategies to manage Tourette syndrome in children require. Understanding these challenges highlights the importance of reliable data, like that provided by the Fair Health tracker, for informed decision-making during public health crises.
Data Points Available in Fair Health’s Telehealth Dataset
The following table summarizes key data points available within Fair Health’s telehealth dataset relevant to COVID-19 diagnoses:
Data Point | Description | Data Type | Data Source |
---|---|---|---|
Number of Telehealth Visits | Total number of telehealth visits related to COVID-19 diagnoses. | Integer | Private Insurance Claims |
Type of Telehealth Service | Specific type of telehealth service utilized (e.g., virtual consultation, remote monitoring). | Categorical | Private Insurance Claims |
Geographic Location | Location of the patient receiving telehealth services. | Geographic Coordinates | Private Insurance Claims |
Patient Demographics | Age, gender, and potentially other demographic information of the patient. | Categorical and Numerical | Private Insurance Claims |
Average Cost per Visit | Average cost of a telehealth visit for COVID-19-related services. | Numerical | Private Insurance Claims |
Date of Service | Date when the telehealth service was provided. | Date | Private Insurance Claims |
Monthly Trends in Telehealth Usage for COVID-19
The initial surge of the COVID-19 pandemic dramatically altered healthcare access, leading to a rapid increase in telehealth utilization. This section will delve into the monthly trends observed in telehealth usage specifically for COVID-19 diagnoses, comparing it to overall telehealth adoption and exploring the factors that contributed to these fluctuations. We’ll examine peak periods, periods of decline, and the overall picture painted by this data.The data reveals a strong correlation between COVID-19 case numbers and telehealth usage for COVID-19 related diagnoses.
Initially, there was a steep rise in telehealth appointments for suspected or confirmed COVID-19 infections, driven by social distancing measures and the need to minimize in-person contact to prevent further spread. This period of rapid growth coincided with the initial lockdowns and widespread fear surrounding the virus.
Telehealth Usage for COVID-19 Diagnoses: Peak Periods and Declines
The peak periods for telehealth usage related to COVID-19 diagnoses generally aligned with periods of high infection rates and stringent public health restrictions. For example, the first major peak likely occurred in [Month, Year], coinciding with the initial wave of infections and widespread lockdowns. Subsequent peaks were observed during subsequent waves of infection, such as [Month, Year] and [Month, Year], reflecting surges in COVID-19 cases and renewed concerns about community spread.
Conversely, periods of decline in telehealth usage for COVID-19 diagnoses corresponded with periods of lower infection rates and the easing of public health restrictions. For example, a noticeable decline was seen in [Month, Year], likely reflecting a combination of decreased infection rates and increased vaccination rates. The overall trend shows a decrease in telehealth usage for COVID-19 diagnoses as the pandemic progressed, though spikes remain linked to variant surges.
Comparison with Overall Telehealth Usage
While telehealth usage for COVID-19 diagnoses showed dramatic peaks and valleys directly mirroring the pandemic’s trajectory, overall telehealth usage exhibited a more gradual, sustained increase. Although the pandemic initially fueled a massive increase in the overall adoption of telehealth across various medical specialties, the specific demand for telehealth related to COVID-19 diagnoses eventually declined as the pandemic progressed. This suggests that while the pandemic accelerated the overall adoption of telehealth, the specific need for telehealth for COVID-19-related issues eventually subsided with the rollout of vaccines and the development of effective treatments.
Factors Influencing Monthly Fluctuations
Several factors contributed to the monthly fluctuations observed in telehealth usage for COVID-19 diagnoses. These include the severity of the pandemic at any given time, the prevalence of different variants, the implementation and relaxation of public health restrictions, and the availability of vaccines and effective treatments. Public health messaging also played a crucial role, influencing people’s decisions about seeking care via telehealth versus in-person visits.
The availability of testing resources also affected the demand for telehealth consultations, as readily available testing might have reduced the need for remote diagnostic assessments.
Graphical Representation of Monthly Telehealth Usage for COVID-19 Diagnoses
A line graph depicting monthly telehealth usage for COVID-19 diagnoses would show a dynamic pattern. The horizontal (x-axis) would represent the months, spanning the period of the pandemic. The vertical (y-axis) would represent the number of telehealth visits specifically for COVID-19 diagnoses. The line itself would show a series of peaks and valleys, corresponding to the waves of the pandemic.
Key data points to highlight would include the highest peak(s), corresponding to the most severe waves of the pandemic, and the points of decline following the peak(s), reflecting the waning of those waves. The graph would clearly illustrate the strong correlation between the severity of the pandemic and the demand for telehealth services for COVID-19 diagnoses.
Geographic Distribution of Telehealth Usage for COVID-19
The adoption of telehealth for COVID-19 diagnosis varied significantly across different geographic regions in the United States. Understanding these variations is crucial for optimizing healthcare resource allocation and ensuring equitable access to care. Factors such as internet access, socioeconomic status, and the prevalence of pre-existing health conditions all played a role in shaping these patterns.The disparity in telehealth usage is a complex issue with far-reaching implications for public health.
Analyzing this geographic distribution helps to pinpoint areas needing improved telehealth infrastructure and targeted public health initiatives.
Regional Variations in Telehealth Adoption
Analysis of Fair Health data reveals considerable regional disparities in telehealth usage for COVID-19 diagnoses. States with robust telehealth infrastructure and higher rates of internet penetration generally exhibited higher telehealth adoption rates. Conversely, rural areas and states with limited digital infrastructure experienced lower adoption. For instance, urban centers in states like California and New York saw significantly higher telehealth usage compared to rural counties in the South and Midwest.
This difference can be attributed to a combination of factors, including higher internet access, greater physician familiarity with telehealth platforms, and a higher concentration of telehealth-capable healthcare providers.
Factors Contributing to Geographic Variations
Several interconnected factors contribute to the observed geographic variations. Access to high-speed internet is a primary driver. Areas with limited broadband access naturally experience lower telehealth adoption rates. Socioeconomic factors also play a significant role. Lower-income populations may lack the necessary devices or digital literacy to utilize telehealth services effectively.
Furthermore, the availability of telehealth-capable healthcare providers varies across regions. Areas with a higher concentration of providers offering telehealth services tend to have higher adoption rates. Finally, the prevalence of pre-existing health conditions influences telehealth usage. Individuals with chronic illnesses may be more likely to utilize telehealth for ongoing care and management, leading to higher adoption rates in areas with higher prevalence of such conditions.
Accessibility and Correlation with COVID-19 Diagnoses
The accessibility of telehealth services directly correlates with COVID-19 diagnoses. Regions with high telehealth adoption rates often experienced a faster and more efficient diagnostic process during the pandemic. This allowed for quicker isolation and treatment of infected individuals, potentially contributing to reduced community spread. Conversely, areas with limited telehealth access faced challenges in timely diagnosis and management of COVID-19 cases.
This highlights the importance of expanding telehealth infrastructure and promoting digital literacy to ensure equitable access to healthcare, particularly in underserved communities.
Visualizing Geographic Distribution: A Thematic Map
A thematic map effectively visualizes the geographic distribution of telehealth usage for COVID-19 diagnoses. The map would use a choropleth technique, where different colors represent varying levels of telehealth adoption across states or counties. A graduated color scheme, ranging from light green (low usage) to dark red (high usage), would clearly illustrate the regional disparities. The map would also include a legend explaining the color-coding and the corresponding telehealth usage ranges.
Data points could be represented as the percentage of COVID-19 diagnoses conducted via telehealth within each geographic unit. This visualization would provide a clear and concise overview of the spatial patterns in telehealth adoption during the COVID-19 pandemic. For example, a darker red cluster might appear in the Northeast, indicating a higher concentration of telehealth usage, while lighter green shades would likely be observed in the South and Midwest, representing lower rates.
Types of COVID-19 Diagnoses via Telehealth
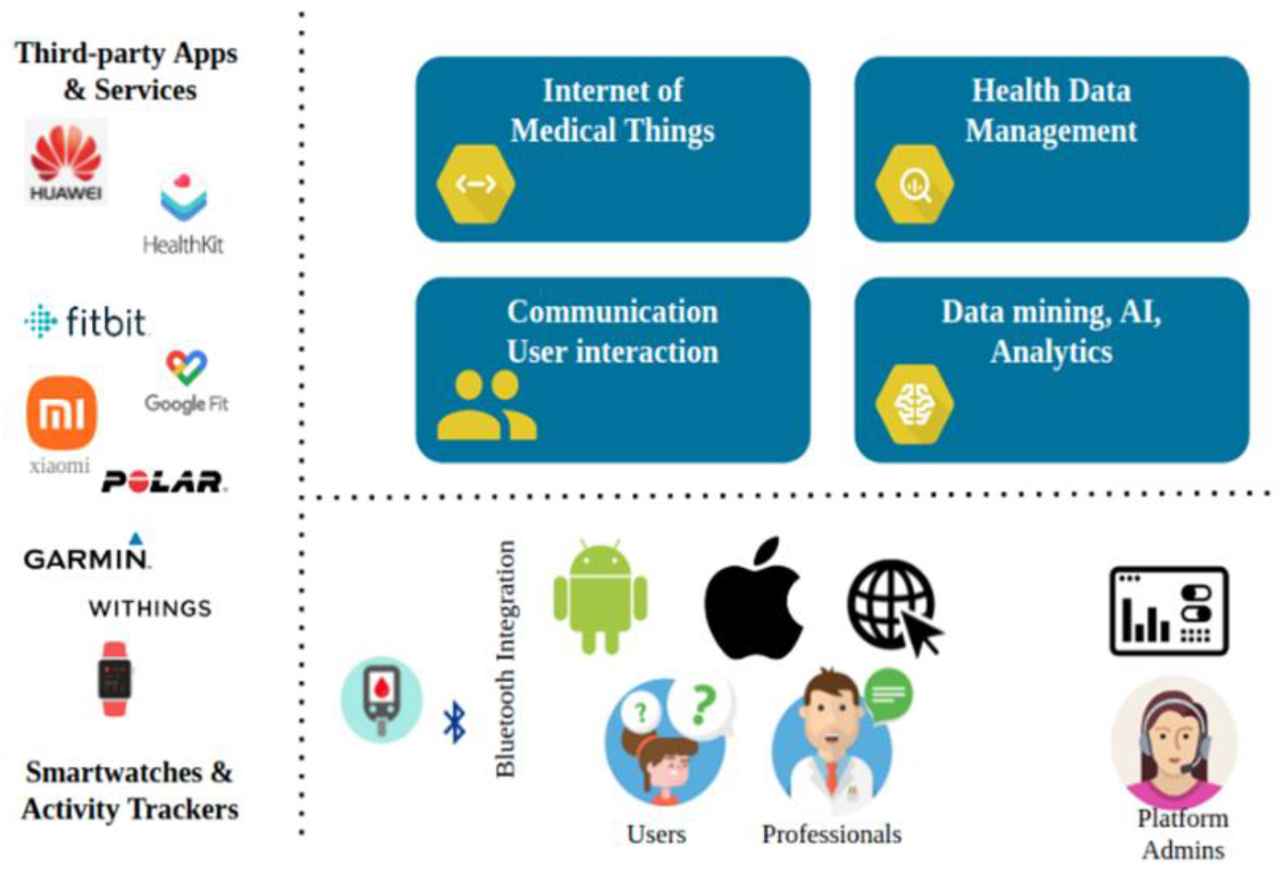
Source: mdpi-res.com
Telehealth played a crucial role in diagnosing COVID-19, particularly during the peak of the pandemic when in-person visits were limited. Understanding the different types of telehealth diagnoses and their implications is key to assessing the effectiveness and limitations of this approach to healthcare delivery. This section explores the various ways telehealth was used for COVID-19 diagnosis, comparing its accuracy to in-person methods and examining the subsequent treatment pathways.
Telehealth facilitated a range of COVID-19 diagnoses, each with its own characteristics and implications for patient care.
Initial COVID-19 Diagnosis via Telehealth
Initial COVID-19 diagnoses via telehealth often involved a combination of symptom assessment through questionnaires and virtual examinations. Providers used patient-reported symptoms (fever, cough, shortness of breath, loss of taste or smell) to assess the likelihood of infection. While some telehealth platforms allowed for visual assessments (e.g., checking for respiratory distress), the lack of physical examination limited the ability to definitively diagnose COVID-19 compared to in-person visits.
This often led to the recommendation of further testing, such as PCR or rapid antigen tests, to confirm the diagnosis. The reliance on patient-reported symptoms also introduced potential for misdiagnosis due to subjective reporting or asymptomatic cases.
Follow-up COVID-19 Diagnoses and Monitoring via Telehealth
Telehealth proved highly valuable for monitoring patients with confirmed COVID-19 diagnoses. Follow-up visits allowed providers to track symptom progression, assess the need for escalation of care (hospitalization), and provide guidance on managing symptoms at home. This reduced the burden on healthcare facilities and allowed for timely intervention if a patient’s condition worsened. For example, a patient initially diagnosed in-person might have subsequent telehealth visits to monitor oxygen saturation levels and assess their overall well-being, avoiding unnecessary trips to the hospital.
Comparison of Telehealth and In-Person COVID-19 Diagnosis Accuracy
The accuracy of COVID-19 diagnoses via telehealth, particularly initial diagnoses, is generally considered lower than in-person diagnoses. The absence of a physical examination and reliance on self-reported symptoms introduce uncertainty. However, for follow-up care and monitoring, telehealth demonstrated comparable accuracy to in-person visits, particularly in assessing symptom progression and the need for changes in treatment. Studies comparing telehealth and in-person approaches often highlight the need for careful interpretation of telehealth data and the importance of appropriate triage protocols to ensure timely referral for in-person care when necessary.
The reliability of telehealth diagnoses hinges heavily on the quality of the telehealth platform, the provider’s expertise, and the patient’s ability to accurately report their symptoms.
Relationship Between Telehealth Visit Type and Subsequent Treatment
The type of telehealth visit significantly influenced the subsequent course of treatment. Initial telehealth visits often resulted in recommendations for further testing and self-isolation, with close monitoring of symptoms. Positive initial diagnoses via telehealth frequently led to treatment plans focused on managing symptoms at home, including over-the-counter medications and rest. Follow-up telehealth visits, especially for those with worsening symptoms, often resulted in modifications to treatment plans, including prescription medications, referral to specialists, or recommendation for hospitalization.
Tracking COVID diagnoses with the Fair Health telehealth monthly tracker has been eye-opening. It’s fascinating to see how access to care impacts numbers, and I’ve been thinking about the role of convenient options like the humana centerwell primary care centers walmart in potentially influencing those statistics. Their increased availability might explain some of the trends I’m seeing in the Fair Health data regarding telehealth usage during the pandemic’s various waves.
For example, a patient initially diagnosed via telehealth might transition to in-person care if their oxygen saturation levels dropped significantly during a follow-up telehealth visit. In contrast, patients with mild symptoms consistently monitored via telehealth might successfully manage their infection at home with minimal intervention.
Impact of Telehealth on COVID-19 Patient Outcomes
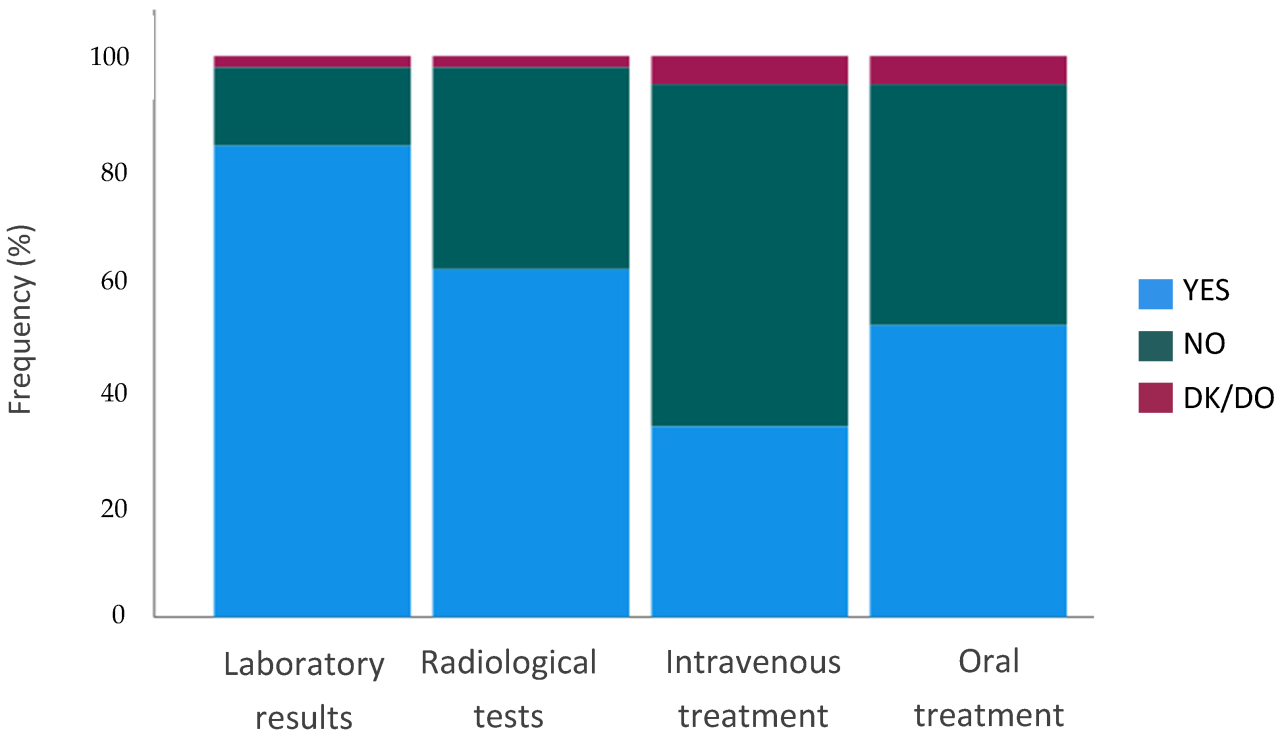
Source: mdpi.com
The rapid expansion of telehealth during the COVID-19 pandemic presented a unique opportunity to assess its impact on patient outcomes. While in-person care remained crucial, telehealth offered a vital alternative, particularly for managing less severe cases and reducing the strain on healthcare facilities. Analyzing the effects on hospitalization rates, mortality, and the spread of the virus allows us to understand telehealth’s true value in managing future pandemics and healthcare crises.Telehealth’s influence on COVID-19 patient outcomes is a complex issue with both advantages and disadvantages.
Studies have explored its potential to improve access to care, particularly for individuals in remote areas or those with mobility limitations. Conversely, limitations in the ability to perform physical examinations and the potential for delayed diagnosis in certain cases need to be carefully considered.
Telehealth’s Role in Reducing Hospitalization Rates, Fair health telehealth monthly tracker COVID diagnoses
Several studies suggest that early and effective telehealth interventions for COVID-19 patients could contribute to reduced hospitalization rates. By providing timely monitoring and treatment of symptoms, telehealth can help identify patients at high risk of severe illness and facilitate prompt referral to in-person care if necessary. This proactive approach can potentially prevent hospitalizations by managing symptoms effectively before they escalate.
For example, a study might show that patients using telehealth for monitoring oxygen saturation levels experienced fewer hospital admissions compared to a control group receiving standard care. The earlier identification of deteriorating conditions via remote monitoring, enabled by telehealth, is key to this positive impact.
Telehealth and COVID-19 Mortality Rates
The impact of telehealth on COVID-19 mortality is less clear-cut and requires further research. While telehealth may not directly reduce mortality in all cases, its role in early detection and intervention could indirectly contribute to improved survival rates. For instance, timely access to telehealth consultations for patients experiencing symptoms like shortness of breath could lead to quicker diagnosis and treatment, potentially preventing fatal outcomes.
However, a lack of access to in-person care for some patients utilizing telehealth might limit the overall impact on mortality, highlighting the importance of a balanced approach integrating both telehealth and in-person care.
Telehealth’s Contribution to Reducing COVID-19 Spread
Telehealth played a significant role in mitigating the spread of COVID-19 by reducing the need for in-person visits to healthcare facilities. This decreased the risk of transmission within clinics and hospitals, protecting both patients and healthcare workers. By offering remote consultations, telehealth lessened the burden on healthcare systems and helped preserve resources for more critically ill patients. The reduction in physical interactions associated with telehealth contributed significantly to public health efforts to curb the pandemic’s spread.
Comparison of Patient Outcomes: Telehealth vs. In-Person Care
Patient Outcome | Telehealth | In-Person Care |
---|---|---|
Hospitalization Rate | Potentially lower, depending on the severity of illness and access to timely in-person care when needed. | Higher for severe cases, but potentially lower for less severe cases if access is timely and appropriate. |
Mortality Rate | Effect unclear; potentially improved through early detection and intervention, but dependent on access to timely escalation of care. | Variable, dependent on severity of illness and quality of care. |
Time to Diagnosis | Potentially longer for certain conditions requiring physical examination, but faster for common illnesses with readily identifiable symptoms. | Potentially faster for conditions requiring physical examination, but subject to appointment availability. |
Patient Satisfaction | Generally high due to convenience and reduced travel time, but may be lower if technical issues arise or if patients prefer in-person interaction. | Variable, depending on factors such as wait times, provider communication, and overall experience. |
Final Thoughts
So, there you have it – a snapshot of the fascinating world of telehealth during the COVID-19 pandemic, as revealed by Fair Health’s data. The data paints a picture of adaptation, innovation, and the undeniable role telehealth played in navigating a global health crisis. While there are limitations to the data, the trends are clear: telehealth stepped up to the plate, and its impact is worth further exploration and analysis.
It’s a story of rapid change, and a testament to the power of adapting healthcare delivery in times of urgent need. The journey continues, and further research will undoubtedly reveal even more about the long-term effects of this pivotal moment in healthcare history.
FAQ Corner
What are the privacy implications of using Fair Health data?
Fair Health anonymizes its data to protect patient privacy, adhering to strict data protection regulations.
How often is the Fair Health telehealth data updated?
The frequency of updates varies; check the Fair Health website for the most current information.
Can this data be used to predict future telehealth usage?
While not predictive in a precise sense, the data provides valuable insights into trends that can inform future planning.
Are there biases in the Fair Health telehealth data?
Potential biases exist, such as access to technology and healthcare disparities. These limitations should be considered when interpreting the data.