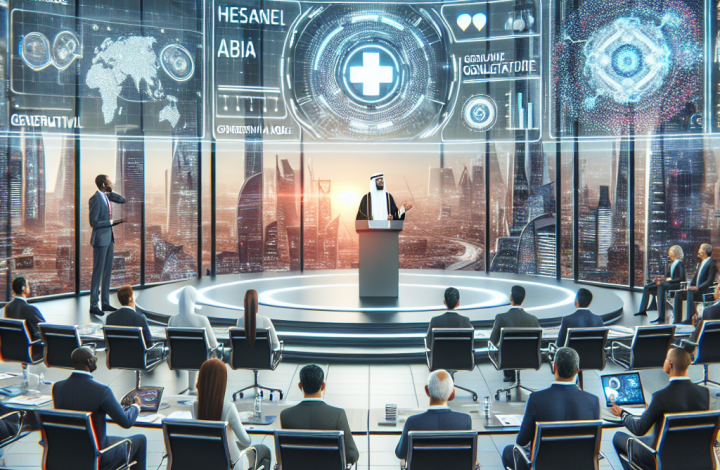
Google Clinical Search Images Vertex AI Healthcare
Google Clinical Search Images Vertex AI Healthcare: Imagine a future where doctors can instantly access the most relevant medical information and analyze complex medical images with unparalleled speed and accuracy. This is the promise of integrating Google’s powerful search capabilities with the advanced machine learning prowess of Vertex AI, revolutionizing healthcare as we know it. This powerful combination offers a glimpse into a future of faster diagnoses, more effective treatments, and ultimately, better patient outcomes.
This post delves into the exciting world of Google Clinical Search and Vertex AI, exploring their individual capabilities and the transformative potential of their integration. We’ll examine how this technology can streamline workflows, improve diagnostic accuracy, and address critical ethical considerations surrounding AI in healthcare. We’ll also look at real-world examples and future applications that are poised to reshape the landscape of medical practice.
Google Clinical Search Functionality
Google Clinical Search, built on Vertex AI, offers a powerful tool for healthcare professionals seeking quick and accurate access to medical information. It leverages Google’s advanced search technology to sift through vast amounts of clinical data, providing relevant results tailored to specific needs. This allows for more efficient workflows and improved decision-making in a time-sensitive environment.Google Clinical Search integrates seamlessly with other Google Cloud services, enhancing its capabilities and utility.
This integration allows for a streamlined workflow where data from various sources can be easily accessed and analyzed within a single platform. For instance, it can connect with Google Cloud Storage for storing and retrieving medical images, or with BigQuery for advanced data analytics on patient populations. This interconnectedness reduces the need for manual data transfers and improves overall data management.
Benefits for Healthcare Professionals
The benefits of using Google Clinical Search are substantial. It significantly reduces the time spent searching for relevant medical information, allowing healthcare professionals to focus more on patient care. The system’s accuracy and reliability minimize the risk of errors stemming from outdated or unreliable information. Furthermore, the ability to quickly access and analyze patient data, combined with relevant research, improves the quality of diagnosis and treatment planning.
The platform’s user-friendly interface ensures that even those less familiar with complex search engines can efficiently utilize its capabilities.
Integration with Google Cloud Services
Google Clinical Search’s integration with other Google Cloud services is a key strength. For example, integration with Google Cloud Healthcare API allows for secure access to and processing of protected health information (PHI). This ensures compliance with regulations such as HIPAA. The integration with other AI/ML services within Vertex AI enables the development of custom solutions for specific clinical needs, such as predictive modeling for disease risk or personalized treatment recommendations.
Google’s Clinical Search Images and Vertex AI Healthcare are amazing tools for improving medical diagnoses, but access to these technologies hinges on a robust healthcare infrastructure. The recent news about Steward Health Care’s Ohio hospital closures and the Pennsylvania facility at risk, as reported in this article steward ohio hospitals closures pennsylvania facility at risk , highlights how vital maintaining accessible healthcare is, impacting the potential reach and effectiveness of advanced AI tools like those offered by Google.
Ultimately, the future of AI in healthcare depends on a strong and equitable system.
This flexibility allows healthcare organizations to tailor the platform to their unique requirements and workflows.
Comparison with Other Medical Search Engines
While several medical search engines exist, Google Clinical Search distinguishes itself through its powerful search algorithms, extensive data sources, and seamless integration within the Google Cloud ecosystem. Other engines may lack the breadth and depth of Google’s data indexing, or they might not offer the same level of security and compliance features crucial in the healthcare sector. The integration with other Google Cloud services provides a significant advantage, offering a unified platform for data management, analysis, and clinical decision support.
Examples of Search Queries and Results
Consider a scenario where a physician needs to quickly research the latest treatment guidelines for a specific type of cancer. A search query like “NCCN guidelines metastatic melanoma” might yield a direct link to the relevant guidelines, alongside related research articles and clinical trials. Another example could be a radiologist searching for similar cases of a rare bone fracture.
A query like “differential diagnosis enchondroma radiographic findings” could return relevant medical images, case studies, and research papers supporting a differential diagnosis. The results are presented in a structured and easily digestible format, prioritizing the most relevant information based on the query.
Medical Image Analysis using Vertex AI
Vertex AI offers a powerful suite of tools for analyzing medical images, significantly accelerating diagnosis and treatment planning. Its capabilities leverage cutting-edge machine learning models to process and interpret various image types, providing clinicians with valuable insights that can improve patient outcomes. This goes beyond traditional methods by offering speed, scalability, and the potential for improved accuracy.
Types of Medical Images Handled by Vertex AI
Vertex AI can handle a wide range of medical image modalities, including X-rays, CT scans, MRI scans, ultrasound images, and even microscopy images. This versatility makes it a valuable tool across numerous medical specialties. The platform’s ability to process diverse image formats allows for a comprehensive approach to medical image analysis, regardless of the imaging technique used. The system is designed to be adaptable to new image types as technology evolves.
Machine Learning Models for Medical Image Analysis in Vertex AI
Vertex AI utilizes various machine learning models for medical image analysis, primarily deep learning architectures like convolutional neural networks (CNNs). These models are trained on massive datasets of medical images, allowing them to identify patterns and features that may be subtle or difficult for the human eye to detect. Specific models employed may vary depending on the task, but generally involve techniques such as image classification, object detection, and segmentation.
For instance, a CNN might be trained to identify cancerous lesions in mammograms or detect fractures in X-rays.
Accuracy and Efficiency Compared to Traditional Methods
Compared to traditional methods of medical image analysis, which often rely heavily on manual interpretation by radiologists, Vertex AI offers significant improvements in both accuracy and efficiency. While human expertise remains crucial, Vertex AI can assist by providing a second opinion, flagging potentially concerning areas for review, and reducing the time required for image interpretation. Studies have shown that AI-assisted analysis can increase diagnostic accuracy, especially in detecting subtle abnormalities.
The efficiency gains are significant, as AI can process images much faster than a human, allowing for quicker turnaround times and potentially earlier interventions. This improved speed and accuracy can lead to better patient outcomes and improved workflow efficiency in healthcare settings.
Examples of Vertex AI Assistance in Diagnosis and Treatment Planning
Vertex AI can assist in various aspects of diagnosis and treatment planning. For example, in oncology, it can help identify and classify tumors, assisting in staging and treatment planning. In cardiology, it can aid in the detection of heart abnormalities from echocardiograms or CT scans. In radiology, it can help detect fractures, internal bleeding, or other injuries from X-rays or CT scans.
A specific example could involve a radiologist using Vertex AI to analyze a chest X-ray. The AI might highlight a suspicious nodule, prompting the radiologist to investigate further, potentially leading to early detection of lung cancer. Another example might be in ophthalmology, where Vertex AI could assist in the detection of diabetic retinopathy from retinal images, facilitating timely intervention and preventing vision loss.
Comparison of Vertex AI Models for Medical Image Analysis
Model Name | Strengths | Weaknesses | Applications |
---|---|---|---|
Example Model A (Illustrative – Replace with actual model names) | High accuracy in identifying specific anomalies; fast processing speed. | Requires large training datasets; may struggle with unusual cases. | X-ray analysis, fracture detection. |
Example Model B (Illustrative – Replace with actual model names) | Robust performance across diverse image types; good generalizability. | Computationally intensive; may require specialized hardware. | MRI analysis, tumor detection. |
Example Model C (Illustrative – Replace with actual model names) | Excellent segmentation capabilities; detailed anatomical information. | Potential for overfitting; requires careful validation. | Ultrasound analysis, organ segmentation. |
Example Model D (Illustrative – Replace with actual model names) | Handles noisy images effectively; high sensitivity. | Lower specificity compared to other models; requires further refinement. | Microscopy image analysis, cell classification. |
Integration of Google Clinical Search and Vertex AI: Google Clinical Search Images Vertex Ai Healthcare
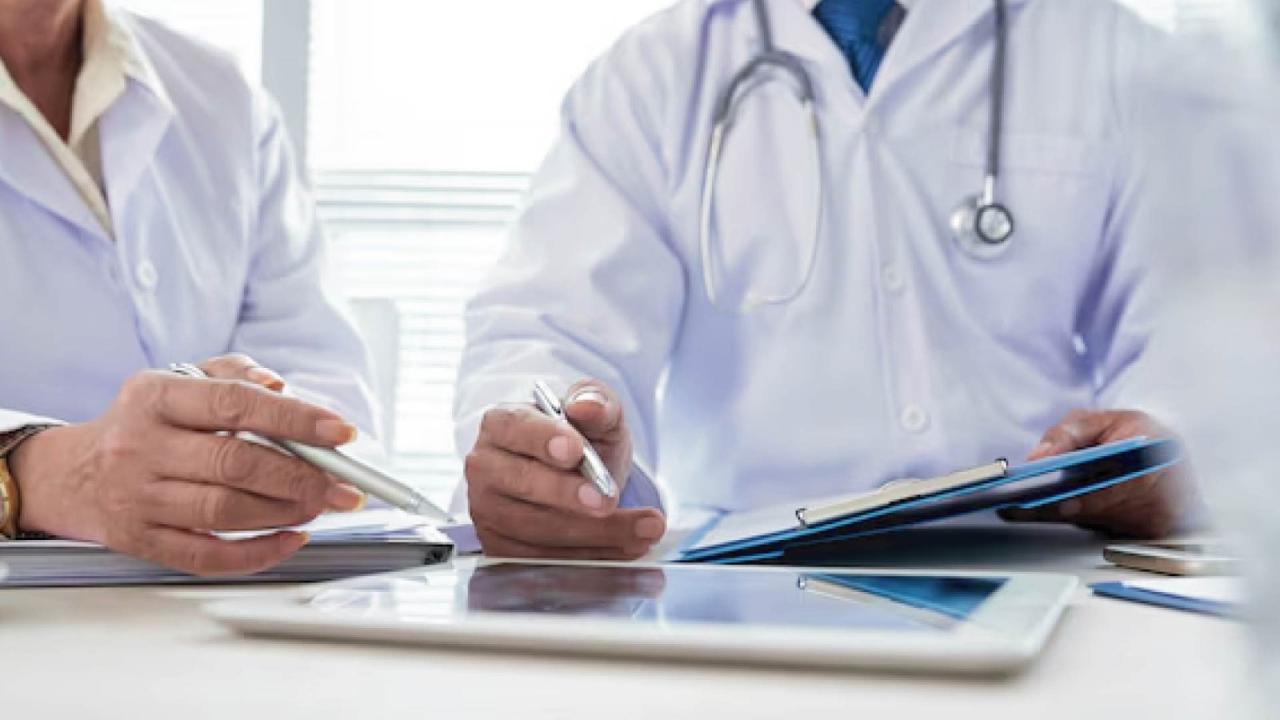
Source: ai-techpark.com
Harnessing the power of Google Clinical Search and Vertex AI together unlocks a new era of efficiency and accuracy in healthcare. By combining the vast knowledge base of Google Clinical Search with the advanced analytical capabilities of Vertex AI, we can create a system that significantly improves diagnostic accuracy, streamlines workflows, and ultimately, enhances patient care. This integration allows for a powerful synergy, leveraging the strengths of each platform to overcome individual limitations.A well-designed workflow integrates Google Clinical Search’s comprehensive medical literature database with Vertex AI’s machine learning prowess for a more effective and informed decision-making process.
Workflow Design for Integrated System
The workflow begins with a healthcare professional querying Google Clinical Search with a patient’s clinical data, including symptoms, medical history, and imaging results. The search engine returns relevant medical literature, research papers, and guidelines. This information is then fed into Vertex AI, which uses machine learning models to analyze the data, identify patterns, and generate insights that might not be readily apparent to a human reviewer.
For example, Vertex AI could identify subtle correlations between seemingly disparate pieces of information, suggesting a diagnosis that might otherwise be missed. The results, including the original search results and Vertex AI’s analysis, are then presented to the healthcare professional in a clear and concise format, aiding in diagnosis and treatment planning.
Data Flow Between Google Clinical Search and Vertex AI
The data flow is a crucial aspect of this integration. Clinical data from Electronic Health Records (EHRs) or other sources is first anonymized and securely transferred to Google Clinical Search. The search engine then retrieves relevant information, which is structured and pre-processed before being sent to Vertex AI. Vertex AI’s models analyze this data, potentially using techniques like natural language processing (NLP) to interpret text from research papers and image analysis algorithms to interpret medical images.
The processed results, including predictions and insights, are then sent back to the healthcare professional via a user-friendly interface. The entire process is designed with security and privacy as paramount concerns.
Challenges in System Integration
Integrating Google Clinical Search and Vertex AI presents several challenges. One significant hurdle is ensuring data interoperability. Different healthcare systems use various data formats and standards, requiring careful data mapping and transformation to ensure seamless flow between the two platforms. Another challenge is maintaining data accuracy and reliability. The quality of the insights generated by Vertex AI depends heavily on the quality of the input data.
Therefore, robust data validation and cleaning processes are crucial. Finally, ensuring the ethical and responsible use of AI in healthcare is paramount. Bias in algorithms and the potential for misinterpretation of results must be carefully addressed.
Data Privacy and Security Strategies
Data privacy and security are of utmost importance in this integrated system. Strict adherence to HIPAA and other relevant regulations is crucial. Data anonymization techniques, such as differential privacy and federated learning, can be employed to protect patient confidentiality. Robust security measures, including encryption and access control, must be implemented throughout the entire data flow. Regular security audits and penetration testing should be conducted to identify and mitigate vulnerabilities.
Transparency and user control over data access and usage are also critical aspects to ensure ethical and responsible data handling.
Potential Use Cases in Healthcare Settings
This integrated system holds immense potential across various healthcare settings. In radiology, it could assist in faster and more accurate diagnosis of medical images by combining image analysis with relevant research. In oncology, it could help predict treatment response and personalize cancer care based on patient-specific genetic and clinical data. In cardiology, it could aid in risk stratification and early detection of cardiovascular diseases.
Google Clinical Search, Images, and Vertex AI Healthcare offer incredible potential for diagnosing and managing complex conditions. Understanding neurological disorders like Tourette Syndrome is crucial, and finding effective strategies is key; check out this helpful resource on strategies to manage Tourette Syndrome in children to learn more. This knowledge, combined with AI-powered tools, could revolutionize pediatric neurology and improve patient outcomes using Google’s advanced healthcare technologies.
In general practice, it could help improve diagnostic accuracy and treatment decisions by providing healthcare professionals with comprehensive and relevant information. The possibilities are vast and continually expanding as AI technology advances.
Ethical Considerations and Data Privacy
The integration of artificial intelligence (AI) into healthcare, particularly with tools like Google Clinical Search and Vertex AI, presents incredible opportunities for improved diagnosis, treatment, and patient care. However, this progress comes with significant ethical responsibilities, primarily concerning data privacy and patient confidentiality. The potential benefits must be carefully weighed against the risks of misuse and the need to protect sensitive health information.The use of AI in healthcare necessitates a robust ethical framework to ensure responsible innovation and deployment.
This framework must prioritize patient well-being, transparency, and accountability. Failing to address these ethical considerations could erode patient trust and hinder the widespread adoption of beneficial AI technologies.
HIPAA Compliance and Data Security
Compliance with the Health Insurance Portability and Accountability Act (HIPAA) is paramount when handling Protected Health Information (PHI). This involves implementing stringent security measures to protect patient data from unauthorized access, use, or disclosure. This includes physical security of data centers, robust access control systems, encryption of data both in transit and at rest, and regular security audits.
Furthermore, organizations must establish comprehensive data breach response plans to mitigate the impact of any potential security incidents. Failure to comply with HIPAA can result in significant financial penalties and reputational damage. Examples of HIPAA-compliant practices include using de-identification techniques and employing robust encryption methods across all stages of data processing and storage.
Responsible Use of AI in Medical Decision-Making
AI-powered tools should be used as decision support systems, augmenting – not replacing – the clinical judgment of healthcare professionals. Over-reliance on AI without proper clinical oversight could lead to misdiagnosis, inappropriate treatment, and ultimately harm patients. Transparency in the AI’s decision-making process is crucial, allowing clinicians to understand the factors contributing to the AI’s recommendations and make informed judgments.
This includes clear documentation of the AI’s limitations and potential biases. Continuous monitoring of the AI’s performance and regular retraining to ensure accuracy and effectiveness are also essential components of responsible use. For example, a radiologist using an AI-powered tool for detecting lung nodules should always review the AI’s findings and use their clinical expertise to make the final diagnosis.
Data Anonymization and De-identification Best Practices
Protecting patient privacy in medical image analysis requires robust data anonymization and de-identification techniques. Simple removal of identifying information like names and dates of birth is insufficient. More sophisticated methods, such as data perturbation (adding noise to the data), differential privacy (adding carefully calibrated noise to protect individual data points), and synthetic data generation (creating artificial datasets that mimic the characteristics of real data without containing actual patient information), are necessary to ensure strong privacy protections.
The effectiveness of these methods should be regularly evaluated to ensure they adequately protect patient privacy while maintaining the utility of the data for research and development. For instance, before using medical images for AI training, techniques like blurring sensitive anatomical details or altering pixel intensities while preserving diagnostic information can be implemented.
Potential Biases in AI Algorithms and Mitigation Strategies, Google clinical search images vertex ai healthcare
AI algorithms used in medical image analysis can inherit and amplify existing biases present in the training data. This can lead to inaccurate or discriminatory outcomes.
- Bias due to underrepresentation of certain demographics: If the training dataset predominantly includes images from one demographic group (e.g., a specific age, gender, or ethnicity), the AI may perform poorly on images from underrepresented groups. Mitigation: Carefully curate training datasets to ensure they are representative of the diverse patient population. This may involve actively seeking out and including data from underrepresented groups.
- Bias due to image acquisition techniques: Differences in imaging equipment, protocols, or image quality can introduce biases. Mitigation: Standardize image acquisition protocols and apply image preprocessing techniques to minimize variations in image quality.
- Bias due to labeling errors: Inconsistent or inaccurate labeling of training data can lead to biased AI models. Mitigation: Employ multiple expert annotators to label the training data and implement quality control measures to identify and correct errors.
Addressing these biases requires a multi-faceted approach, including careful dataset curation, rigorous algorithm validation, and ongoing monitoring of the AI’s performance across different demographic groups. Regular audits and transparency are key to maintaining fairness and accountability.
Future Trends and Applications
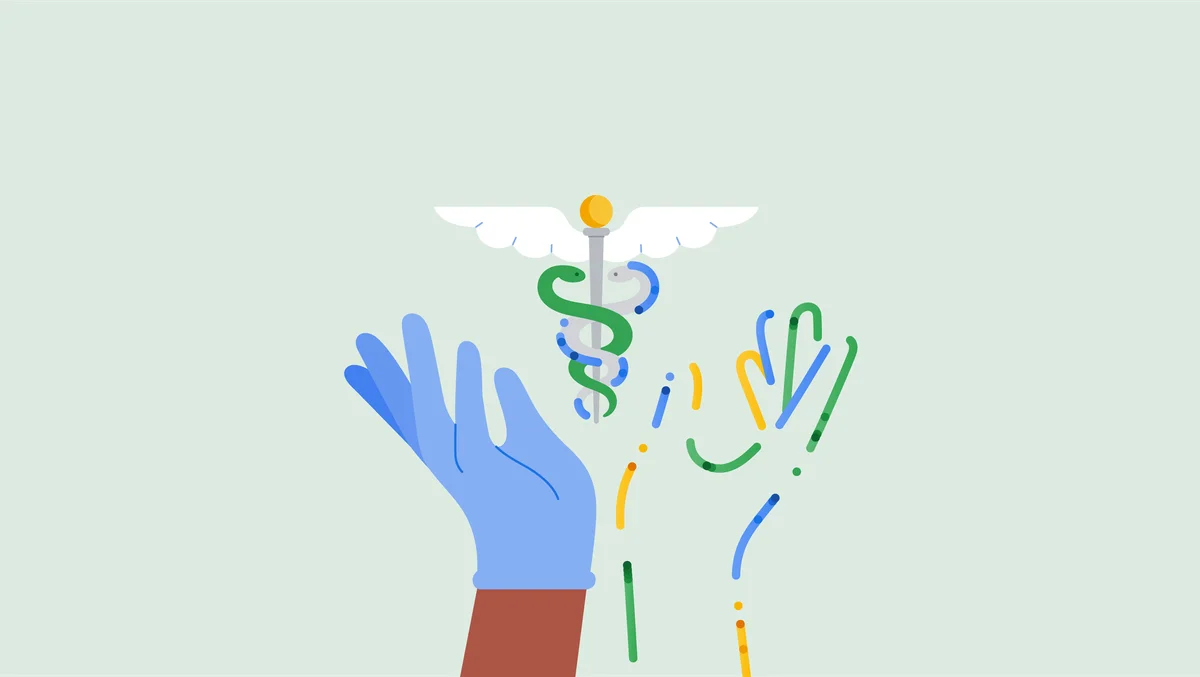
Source: googleapis.com
The integration of Google Clinical Search and Vertex AI represents a significant leap forward in healthcare, promising a future where diagnosis, treatment, and patient care are dramatically improved through the power of AI-driven insights and readily accessible medical information. The potential applications extend far beyond current capabilities, shaping a more personalized, efficient, and equitable healthcare system.The synergistic combination of Google Clinical Search’s vast medical knowledge base and Vertex AI’s powerful analytical capabilities will fuel innovation across multiple domains.
Google’s Clinical Search Images and Vertex AI Healthcare are game-changers for medical professionals, offering powerful tools for diagnosis and treatment planning. This improved access to information becomes even more critical given the recent CMS initiative, as detailed in this article on the cms launches primary care medicare model aco , which will likely increase the demand for efficient, accurate healthcare solutions.
Ultimately, technologies like Google’s AI tools will be crucial for navigating this evolving landscape and providing the best possible patient care.
This powerful duo will not only improve the speed and accuracy of diagnoses but also pave the way for predictive analytics and personalized treatment plans, ultimately transforming how healthcare is delivered and experienced.
Personalized Medicine and Precision Healthcare
The convergence of Google Clinical Search and Vertex AI offers unprecedented opportunities for personalized medicine. By analyzing a patient’s unique medical history, genetic information, lifestyle factors, and other relevant data available through Google Clinical Search, Vertex AI can identify patterns and predict potential health risks with greater accuracy. This allows for the creation of highly tailored treatment plans, optimizing therapies based on individual patient needs and reducing the likelihood of adverse reactions.
For example, a patient with a family history of heart disease could receive a personalized risk assessment, prompting earlier intervention and preventative measures, such as lifestyle changes or targeted medication. This proactive approach shifts the focus from reactive treatment to proactive health management.
Improved Hospital Workflow and Patient Care
Imagine a hospital where radiologists can instantly access relevant research papers and clinical trials while analyzing medical images using Vertex AI’s image analysis capabilities. This integrated system could flag potential anomalies in scans, providing second opinions and significantly reducing diagnostic errors. Furthermore, clinicians can access a comprehensive patient history in seconds, ensuring informed decision-making and minimizing delays in treatment.
For instance, a patient admitted with chest pain could have their scans analyzed by Vertex AI, instantly highlighting potential areas of concern and providing the attending physician with relevant research and treatment protocols within the Google Clinical Search interface. This integrated workflow streamlines the process, leading to faster diagnosis and treatment, ultimately improving patient outcomes.
Impact on Healthcare Costs and Accessibility
The widespread adoption of Google Clinical Search and Vertex AI has the potential to significantly reduce healthcare costs and improve accessibility. By automating tasks such as image analysis and preliminary diagnosis, these technologies can free up clinicians’ time, allowing them to focus on more complex cases and patient interaction. Furthermore, the improved accuracy of diagnoses can lead to a reduction in unnecessary tests and procedures, lowering overall healthcare expenditure.
The accessibility of medical information through Google Clinical Search can also empower patients and improve health literacy, promoting better self-management of chronic conditions. This increased efficiency and accessibility could lead to a more equitable distribution of healthcare resources, benefiting underserved communities and bridging the healthcare gap. For example, remote diagnostic capabilities using AI-powered image analysis could significantly improve access to specialized care in rural or underserved areas.
Summary
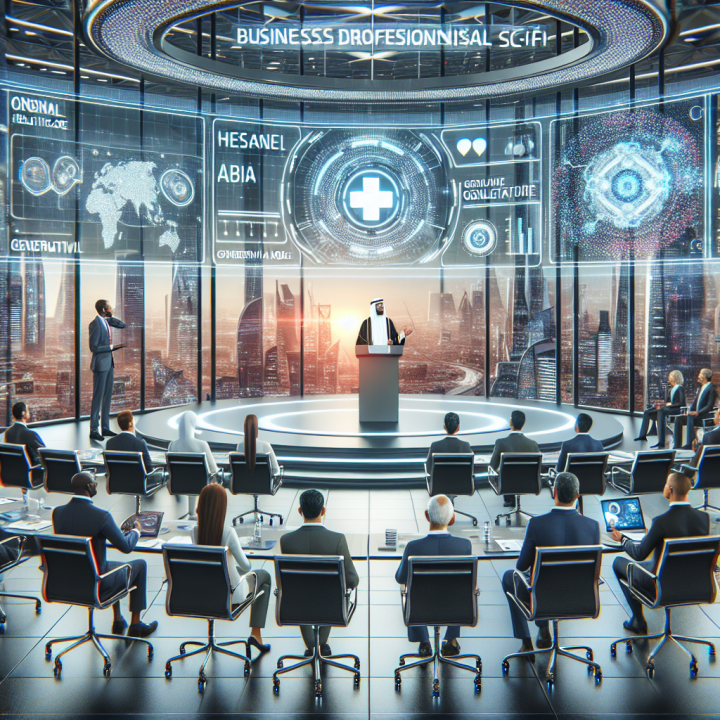
Source: watkinslabs.com
The convergence of Google Clinical Search and Vertex AI represents a significant leap forward in healthcare technology. By combining the power of comprehensive medical information retrieval with advanced AI-driven image analysis, this integrated system promises to enhance diagnostic accuracy, personalize treatment plans, and improve overall patient care. While challenges remain in data privacy and ethical considerations, the potential benefits are undeniable, paving the way for a more efficient, effective, and ultimately, more humane approach to healthcare delivery.
The future of medicine is here, and it’s powered by intelligent technology.
FAQs
What types of data can Google Clinical Search handle?
Google Clinical Search can handle a wide range of data types, including clinical notes, research papers, medical images (though image analysis requires Vertex AI), patient records (with appropriate privacy measures), and more.
How does Vertex AI ensure the privacy and security of patient data?
Vertex AI employs robust security measures, including encryption and access controls, to protect patient data. Compliance with regulations like HIPAA is paramount. Data anonymization and de-identification techniques are also crucial.
What are the limitations of using AI in medical image analysis?
AI models can be susceptible to biases in training data, leading to inaccurate or unfair results. The interpretability of AI’s decisions can also be a challenge, requiring careful consideration and human oversight.
What is the cost of using Google Clinical Search and Vertex AI?
The cost depends on usage and specific features employed. Google Cloud provides pricing details on their website. Factors like data storage, compute resources, and API calls all contribute to the overall expense.