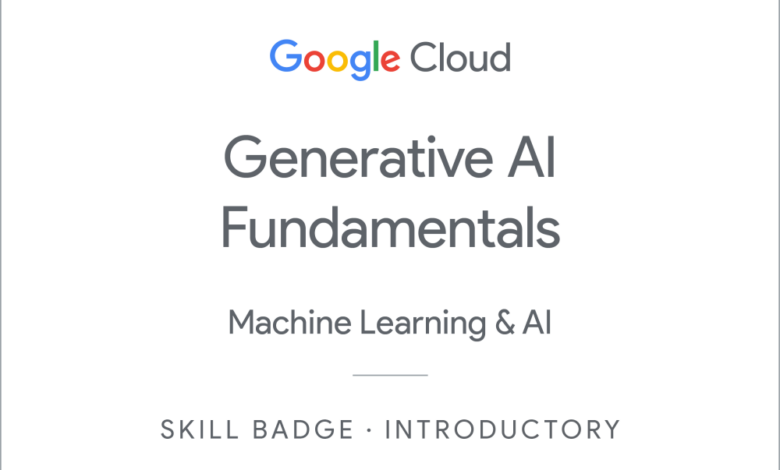
Google Cloud Documentation Tool Generative AI
Google cloud documentation tool generative ai – Google Cloud Documentation Tool: Generative AI – Imagine a future where Google Cloud’s documentation isn’t just helpful, but proactively anticipates your needs. That’s the promise of integrating generative AI into the documentation process. This isn’t just about making things easier to find; it’s about creating a truly personalized and intuitive learning experience. We’ll explore how AI could revolutionize how we access and understand Google Cloud services, from automating creation to personalizing the experience for every user.
This post delves into the exciting possibilities and potential challenges of using generative AI to enhance Google Cloud’s documentation. We’ll cover everything from the current state of Google Cloud’s documentation and a comparison to competitors, to the design of a hypothetical AI-powered tool and the ethical considerations involved. Get ready for a deep dive into the future of tech documentation!
Google Cloud Documentation’s Current State
Google Cloud Platform (GCP) boasts a vast and ever-expanding ecosystem of services, and its documentation aims to reflect this complexity. The current structure is largely hierarchical, organizing information by product and service category. Users navigate through a series of pages, often drilling down from broad overviews to specific API references or how-to guides. While generally well-organized, the sheer scale of the documentation can be overwhelming for newcomers.The current organization, while logical, sometimes lacks intuitive pathways for users seeking solutions to specific problems.
A user facing a particular challenge might struggle to find the relevant documentation quickly. Furthermore, the consistency of style and terminology across different services could be improved. In some areas, the documentation is exceptionally detailed, while in others, crucial information might be missing or buried deep within lengthy pages. Finally, the search functionality, while powerful, could benefit from more sophisticated algorithms that better understand natural language queries.
Documentation Clarity and Ease of Use
Improving clarity and ease of use necessitates several key changes. Firstly, a more user-centered approach is needed, focusing on common use cases and workflows. This involves creating more concise, task-oriented guides that walk users through specific problems, rather than relying solely on comprehensive but potentially overwhelming reference materials. Secondly, improved cross-linking between related documents is crucial. Users should be able to easily navigate between conceptual overviews, practical tutorials, and API references.
Finally, incorporating more visual aids, such as diagrams and screenshots, would significantly improve comprehension, especially for complex concepts. For instance, a visual representation of a data flow in a particular service would be far more effective than a lengthy textual description.
Comparison with AWS Documentation
A direct comparison with Amazon Web Services (AWS) documentation highlights both strengths and weaknesses of GCP’s approach. While both platforms offer extensive documentation, their styles and approaches differ significantly.
Feature | Google Cloud Platform | Amazon Web Services |
---|---|---|
Overall Structure | Hierarchical, product-focused; can feel overwhelming for beginners. | Similar hierarchical structure, but often employs more intuitive navigation and search. |
Search Functionality | Powerful but can struggle with nuanced queries; requires precise s. | Generally considered more robust and effective at handling natural language searches. |
Tutorial Quality | Mix of excellent and less comprehensive tutorials; consistency varies across services. | Widely praised for its many high-quality, practical tutorials and examples. |
API Documentation | Comprehensive and well-structured, but can lack contextual information. | Similarly comprehensive, but often includes more practical examples and usage guidance. |
Community Support | Active community forums and Stack Overflow presence, but can be less centralized than AWS. | Extremely active and well-established community, providing ample support resources. |
Generative AI’s Potential Applications in Documentation
Generative AI offers a transformative potential for Google Cloud documentation, promising significant improvements in efficiency, accuracy, and user experience. By automating tasks and personalizing content, it can revolutionize how we create, maintain, and interact with our technical documentation. This shift will allow our documentation team to focus on higher-level tasks, ensuring the continued high quality and relevance of our resources.Generative AI could automate the creation of documentation for new Google Cloud services by analyzing code, configuration files, and API specifications to generate initial drafts of documentation.
This automated process would significantly reduce the time and effort required to produce comprehensive documentation for new releases, allowing for faster time-to-market. Imagine a scenario where a new service is deployed, and a preliminary set of documentation – including API references, tutorials, and quickstarts – is automatically generated, requiring only minor human review and refinement.
Automating Documentation Creation for New Google Cloud Services
Generative AI can analyze the codebase of a new Google Cloud service, extracting key information such as function parameters, return values, and usage examples. This information can then be used to automatically generate API reference documentation, code samples, and tutorials. For instance, if a new service for managing virtual machines is released, the AI could analyze the code responsible for creating, starting, and stopping VMs, automatically generating documentation for these functions, including parameters, return types, and usage examples.
This automated generation of documentation reduces the manual effort required by engineers, allowing for faster release cycles and improved time-to-market.
Improving the Accuracy and Consistency of Existing Documentation
Generative AI can analyze existing documentation for inconsistencies, inaccuracies, and outdated information. By cross-referencing information across multiple documents and comparing it against the latest service specifications, the AI can identify areas needing improvement. For example, the AI could detect discrepancies between the described functionality of a service and its actual implementation in the code, flagging these inconsistencies for review and correction.
This ensures that the documentation always accurately reflects the current state of the services. Furthermore, generative AI can help maintain consistency in style and terminology across all Google Cloud documentation, improving the overall user experience.
Personalizing Documentation Based on User Roles and Experience Levels
Generative AI can personalize the documentation experience by tailoring content to specific user roles and experience levels. For example, a beginner might receive a simplified tutorial with basic explanations and clear visual aids, while an experienced user might access more advanced documentation with detailed technical specifications and code examples. The AI could analyze user behavior and preferences to dynamically adjust the content and complexity of the documentation presented, ensuring a more efficient and effective learning experience for all users.
This personalized approach will significantly improve user satisfaction and reduce the learning curve for new Google Cloud users. Imagine a scenario where a user, identified as a data scientist, is presented with documentation that focuses on machine learning APIs and relevant use cases, while a system administrator receives documentation geared towards managing infrastructure.
Challenges and Limitations of Using Generative AI for Documentation
Generative AI offers exciting possibilities for streamlining documentation creation, but it’s crucial to acknowledge the inherent challenges and limitations before fully embracing this technology. While AI can significantly accelerate the writing process and improve consistency, potential pitfalls related to bias, security, and accuracy must be carefully considered and mitigated.
Potential Biases in AI-Generated Documentation
AI models are trained on vast datasets, and if these datasets reflect existing societal biases, the generated documentation will likely inherit and amplify those biases. For example, an AI trained primarily on documentation written by a specific demographic might perpetuate gender or cultural stereotypes in its output. This could lead to inaccurate, insensitive, or even offensive content, undermining the credibility and inclusivity of the documentation.
Addressing this requires careful curation of training data to ensure diversity and representation, as well as rigorous review processes to identify and correct biased outputs. The development of bias detection tools specifically designed for text analysis will also be crucial.
Google Cloud’s generative AI documentation tools are a game-changer for tech writers, streamlining the process of creating comprehensive help files. It’s amazing how technology is evolving, even as we see major political shifts like Robert F. Kennedy Jr. clearing a key hurdle in his bid for HHS Secretary, as reported here: rfk jr clears key hurdle on path to hhs secretary.
This rapid pace of change underscores the need for efficient documentation tools like Google Cloud’s offering, ensuring users can quickly adapt to new technologies and processes.
Security Risks Associated with Sensitive Information
Using generative AI to create documentation involving sensitive information, such as proprietary algorithms, financial data, or customer details, presents significant security risks. The AI model itself could inadvertently leak information during the generation process, or malicious actors could attempt to extract sensitive data from the model’s training data or outputs. Furthermore, the use of cloud-based AI services introduces additional vulnerabilities related to data storage and transmission.
Robust security measures, including data encryption, access control, and regular security audits, are essential to mitigate these risks. Strict adherence to data privacy regulations is also paramount.
Ensuring Accuracy and Reliability of AI-Generated Documentation
While generative AI can produce fluent and grammatically correct text, ensuring the accuracy and reliability of the generated documentation remains a significant challenge. AI models can sometimes “hallucinate” facts or create plausible-sounding but ultimately incorrect information. This is especially problematic for technical documentation where precision is critical. Therefore, rigorous fact-checking and verification processes are essential. Human oversight and expert review are crucial steps in validating the accuracy and completeness of the information before it is released.
The development of AI-powered tools that can automatically detect inconsistencies and potential inaccuracies in generated text is also an area of active research.
Designing a Generative AI-Powered Documentation Tool
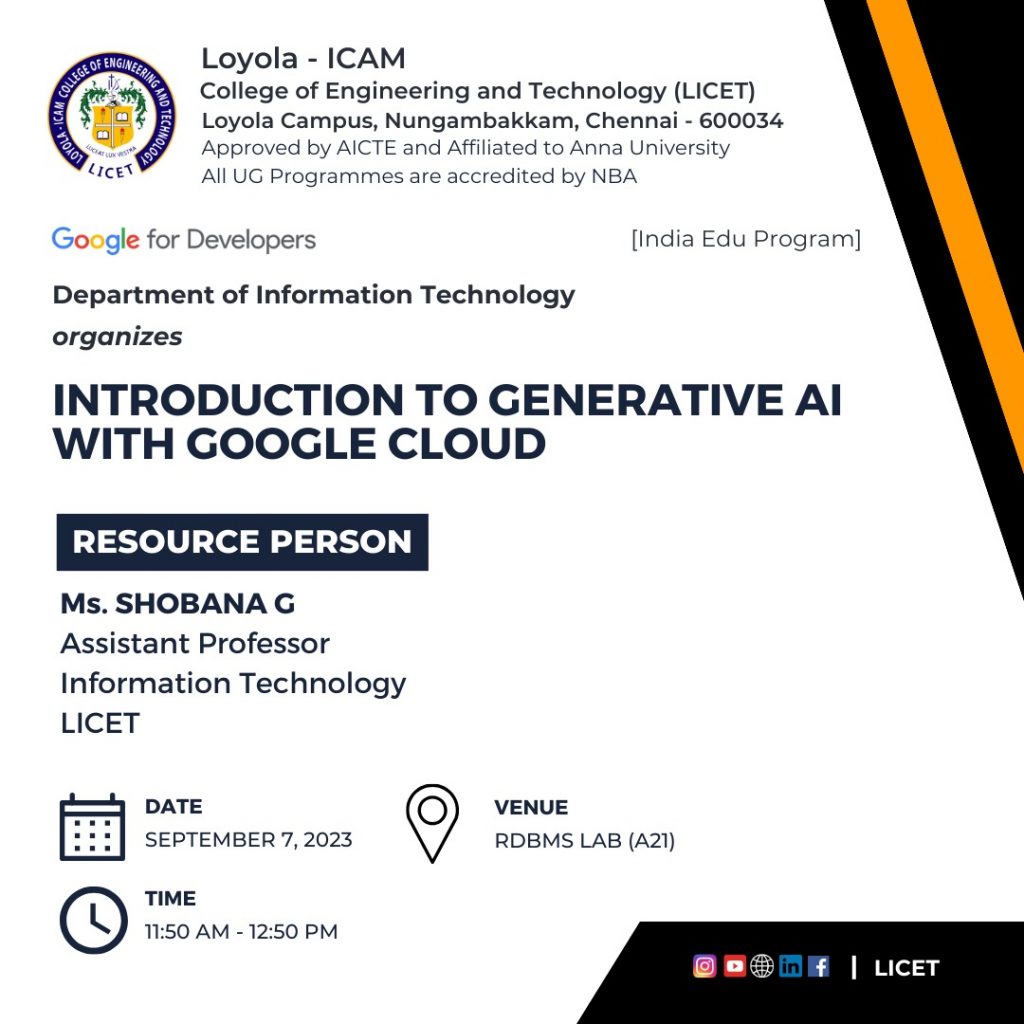
Source: ac.in
Building a generative AI-powered documentation tool for Google Cloud presents a unique opportunity to streamline the creation and maintenance of comprehensive, accurate, and up-to-date documentation. This tool would leverage the power of large language models to assist technical writers and engineers, significantly improving efficiency and reducing the burden of manual documentation processes.
User Interface Design
The user interface should be intuitive and user-friendly, even for those unfamiliar with generative AI. A mockup of the tool would feature a central text editor where users can input prompts or directly edit generated content. A sidebar would offer options for specifying document type (e.g., API reference, tutorial, conceptual guide), target audience (e.g., beginner, intermediate, expert), and desired style guide.
Additional features could include a preview pane displaying the rendered documentation in real-time, a version history tracker for easy rollback, and integration with Google Cloud’s existing documentation repository. The overall aesthetic should be clean and modern, aligning with Google’s existing design language. This would avoid overwhelming the user with excessive features, focusing instead on a smooth and efficient workflow.
Workflow for Documentation Creation
The workflow begins with the user providing input to the AI model. This could involve a simple prompt describing the topic to be documented, or it could involve providing more structured input, such as a skeleton Artikel or a series of bullet points. The AI model then generates the initial draft of the documentation. The user can then review and edit this draft, refining the content, style, and accuracy.
Google Cloud’s generative AI documentation tools are amazing; they’re making tech writing so much easier! I was just reading about how AI is revolutionizing healthcare, and came across this fascinating article on the ai powered solution to the medical coding worker shortage , which highlights how AI can address the critical staffing issues in medical coding. Thinking about how this kind of AI-driven efficiency could be documented using Google Cloud’s tools – definitely something to explore further!
The iterative process of generation, review, and refinement continues until the user is satisfied with the final output. The completed documentation can then be exported in various formats (e.g., Markdown, HTML) and integrated into Google Cloud’s documentation platform. This collaborative approach ensures high-quality documentation while maximizing efficiency.
Technical Specifications and Requirements
Building this tool requires a robust technical infrastructure. The core component is a powerful large language model (LLM), ideally fine-tuned on Google Cloud’s existing documentation and technical literature. This ensures the AI model understands the specific terminology, style, and context of Google Cloud documentation. The tool will also require a natural language processing (NLP) engine to process user input and generate coherent, accurate text.
A robust database is needed to store and manage the generated documentation, as well as the user’s input and editing history. The system must also integrate seamlessly with Google Cloud’s existing infrastructure and security protocols. Furthermore, the system should be scalable to handle a large volume of requests and ensure high availability. Finally, robust monitoring and logging are crucial for identifying and resolving any issues promptly.
Consideration should be given to using Google Cloud’s own services such as Cloud Natural Language API, Cloud Storage, and Cloud Functions for building this tool.
Impact on User Experience
Integrating generative AI into Google Cloud documentation has the potential to revolutionize how users interact with and understand complex technical information. By moving beyond simple searches and offering more conversational and intuitive interactions, we can significantly improve the overall user experience, leading to increased efficiency and reduced frustration. This enhanced experience will be particularly beneficial for users with varying levels of technical expertise, from seasoned developers to those new to cloud computing.The core benefit lies in the AI’s ability to understand natural language queries and provide relevant, concise answers.
Imagine a scenario where a user is struggling with a specific error message. Instead of sifting through lengthy manuals, they can simply type the error message into the AI-powered tool, receiving a clear explanation of the problem and potential solutions, possibly even with code snippets or configuration examples tailored to their specific context. This drastically reduces the time spent searching for information and increases the likelihood of successfully resolving the issue.
Google Cloud’s generative AI documentation tool is amazing for quickly finding info, but sometimes I get sidetracked! For example, I was researching its use in medical applications, and I stumbled upon a fascinating article about risk factors that make stroke more dangerous , which made me realize how crucial accurate and readily available information is in healthcare.
Getting back to Google Cloud’s AI, I’m now exploring how it can help improve access to vital health data.
Improved Search and Information Retrieval
Generative AI can transform the search experience by moving beyond simple matching. Instead of presenting a long list of potentially relevant documents, the AI can understand the user’s intent and provide the most pertinent information directly. This could involve summarizing relevant sections from multiple documents, presenting information in a more digestible format, or even generating entirely new content based on the user’s specific needs.
For example, a user searching for “deploying a Kubernetes cluster on Google Kubernetes Engine” might receive a concise, step-by-step guide tailored to their specific infrastructure setup, rather than a list of links to potentially irrelevant documentation pages.
Personalized and Contextualized Assistance
A generative AI-powered tool can personalize the documentation experience based on the user’s role, project, and previous interactions. This contextual awareness allows the AI to anticipate the user’s needs and provide proactively relevant information. For instance, a user working on a machine learning project might receive tailored recommendations for relevant APIs, libraries, and best practices, while a user managing databases might see different, more relevant information prioritized.
This personalized approach drastically reduces information overload and guides users more effectively through the complex landscape of Google Cloud services.
Benefits and Drawbacks of Generative AI Integration on User Experience, Google cloud documentation tool generative ai
The integration of generative AI offers significant potential benefits, but also presents some challenges:
- Benefits:
- Faster problem resolution: Users can quickly find answers to their questions without extensive searching.
- Improved understanding: Complex concepts can be explained more clearly and concisely.
- Increased efficiency: Users spend less time searching for information and more time working.
- Personalized experience: The documentation adapts to the user’s specific needs and context.
- Enhanced accessibility: The AI can translate technical jargon into simpler terms, making the documentation more accessible to a wider audience.
- Drawbacks:
- Potential for inaccurate information: The AI’s responses depend on the quality of the training data and can sometimes be inaccurate or misleading.
- Over-reliance on the AI: Users might become overly dependent on the AI and fail to develop their own understanding of the underlying concepts.
- Lack of human oversight: The AI might not always be able to handle nuanced or complex situations requiring human judgment.
- Security concerns: The AI could potentially be used to access sensitive information or compromise the security of Google Cloud services.
- Maintenance and update challenges: Keeping the AI’s knowledge base up-to-date with the constantly evolving Google Cloud ecosystem requires significant ongoing effort.
Future Directions and Considerations
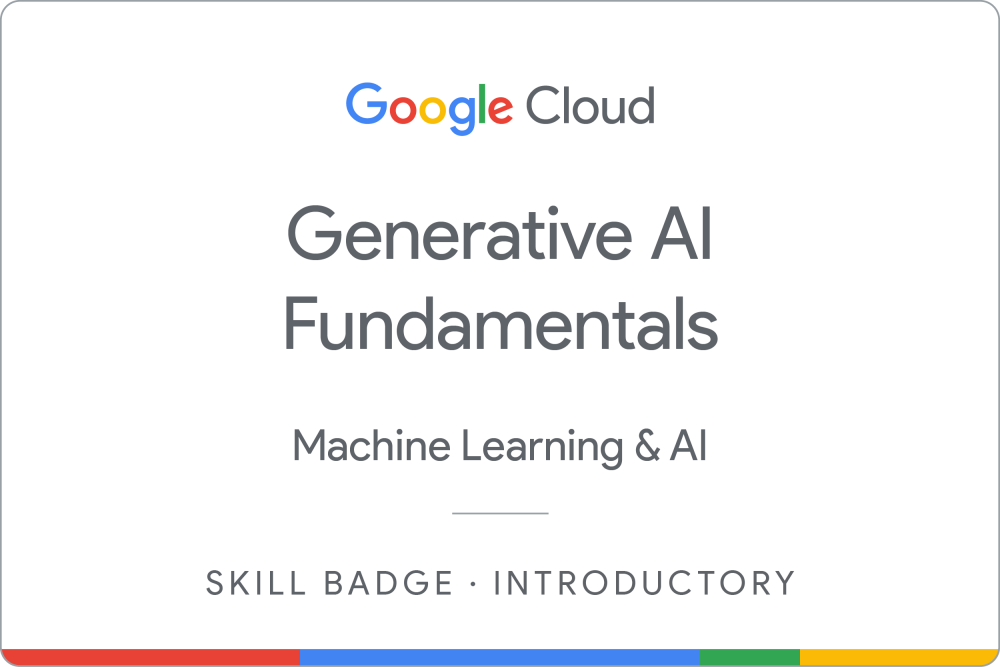
Source: qwiklabs.com
The integration of generative AI into Google Cloud documentation is still in its nascent stages, offering a vast landscape of potential improvements and challenges. Looking ahead, we can anticipate significant advancements that will refine the tool’s capabilities and address its current limitations, leading to a more efficient and user-friendly experience for developers and users alike. This requires careful consideration of both technological progress and ethical implications.The rapid evolution of generative AI models promises substantial enhancements to Google Cloud documentation.
We can expect to see more sophisticated models capable of understanding nuanced queries, generating more accurate and contextually relevant responses, and even proactively suggesting improvements to existing documentation based on user interaction data. Furthermore, advancements in multi-modal AI could allow the integration of visual aids, code examples, and interactive tutorials directly within the generated documentation, significantly enriching the user experience.
Ethical Considerations in Generative AI Documentation
The use of generative AI in documentation raises several ethical considerations. One key concern is the potential for bias in the generated content. If the training data reflects existing biases, the AI model may perpetuate and even amplify these biases in the documentation, potentially leading to unfair or discriminatory outcomes. Another crucial aspect is ensuring the accuracy and reliability of the information generated.
While generative AI models can produce human-quality text, they are not infallible and can sometimes generate inaccurate or misleading information. Robust fact-checking mechanisms and human oversight are essential to mitigate this risk. Finally, the transparency of the AI’s role in documentation creation is vital. Users should be aware when they are interacting with AI-generated content to avoid misunderstandings and maintain trust.
Clear labeling and attribution mechanisms are crucial in this regard.
Long-Term Implications for Google Cloud and its Users
The long-term implications of integrating generative AI into Google Cloud documentation are far-reaching and impactful, affecting both Google Cloud and its users in several ways:
- Enhanced Developer Productivity: Generative AI can significantly accelerate the documentation process, freeing up developers to focus on other critical tasks.
- Improved User Experience: More accurate, comprehensive, and easily accessible documentation will lead to improved user satisfaction and reduced troubleshooting time.
- Increased Efficiency in Documentation Updates: AI can automate the update process, ensuring that the documentation remains current and relevant.
- Potential for Personalized Documentation: AI could tailor documentation to individual user needs and skill levels, providing a more personalized learning experience.
- New Revenue Streams: Google Cloud could explore new revenue streams by offering advanced AI-powered documentation services to other organizations.
- Increased Competition: The adoption of generative AI in documentation could intensify competition among cloud providers.
- Need for New Skillsets: Managing and maintaining AI-powered documentation systems will require new skillsets within Google Cloud’s workforce.
- Concerns about Job Displacement: While AI will increase efficiency, there is a potential for job displacement for some roles involved in traditional documentation creation.
Concluding Remarks
The potential of generative AI to transform Google Cloud documentation is undeniable. From streamlining the creation process and improving accuracy to personalizing the user experience, the benefits are numerous. However, we must also carefully consider the challenges, including potential biases and security risks. By thoughtfully addressing these issues, we can harness the power of AI to create a documentation system that is not only efficient and accurate but also truly user-centric and ethically sound.
The journey towards this AI-powered future is exciting, and I can’t wait to see how it unfolds.
Helpful Answers: Google Cloud Documentation Tool Generative Ai
What are the biggest security concerns with using AI for documentation?
The biggest concerns revolve around data leaks and the potential for AI to generate inaccurate or misleading information that could compromise security. Protecting sensitive information used to train and operate the AI is paramount.
How will this impact smaller Google Cloud users?
Smaller users will benefit greatly from easier access to information and more personalized guidance, overcoming the learning curve associated with complex cloud services. AI could provide tailored tutorials and support based on their specific needs and usage.
What about the cost of implementing such a system?
The cost will depend on the scale of implementation and the specific AI tools used. There will be costs associated with development, training the AI, and ongoing maintenance. However, the long-term benefits of improved efficiency and reduced support costs could outweigh the initial investment.