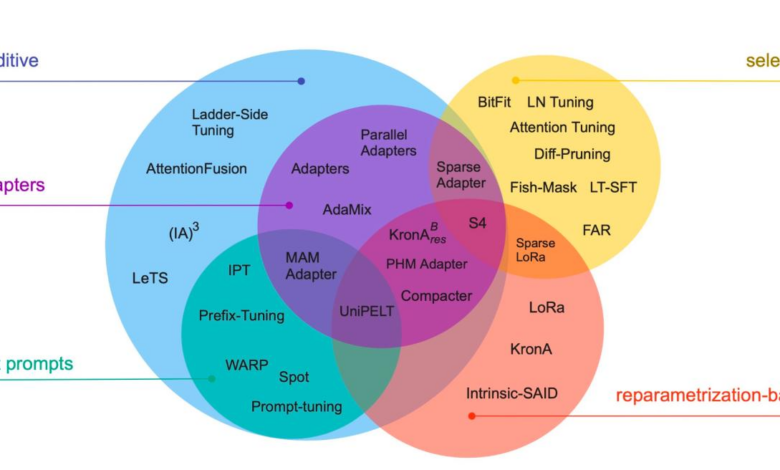
Google Cloud MedLM Generative AI in Healthcare
Google Cloud MedLM Generative AI in healthcare is revolutionizing the industry! Imagine a world where AI assists doctors in diagnosing illnesses faster and more accurately, accelerates drug discovery, and personalizes treatment plans. This isn’t science fiction; it’s the reality MedLM is building. We’ll explore its capabilities, ethical considerations, and the exciting future it promises for patient care.
From analyzing medical images like X-rays and MRIs to streamlining administrative tasks, MedLM offers a powerful suite of tools. We’ll delve into specific use cases, examining how it improves medical image analysis, assists in drug development, personalizes medicine, and even helps manage the administrative burden on healthcare professionals. But this powerful technology also comes with responsibilities; we’ll discuss data security, privacy concerns, and the ethical implications of using AI in healthcare decision-making.
Introduction to Google Cloud MedLM Generative AI in Healthcare
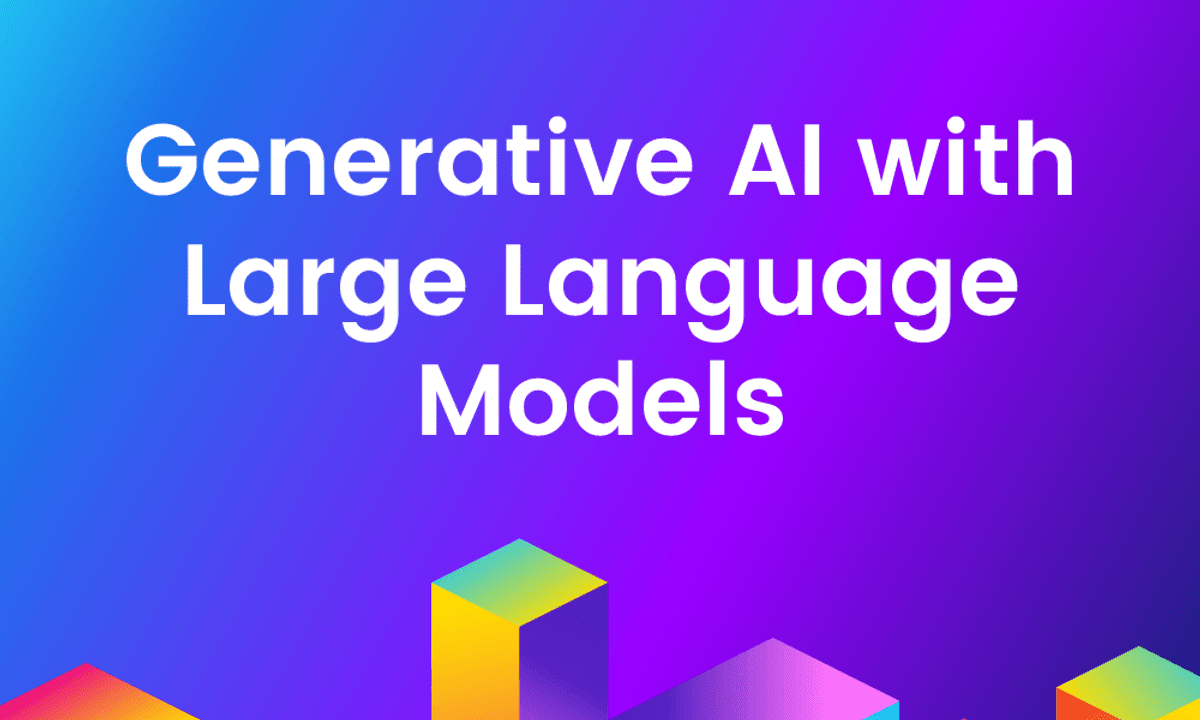
Source: cloudfront.net
Google Cloud MedLM Generative AI represents a significant leap forward in healthcare data analysis. It leverages the power of large language models (LLMs) specifically trained on vast amounts of de-identified medical data, enabling it to perform complex tasks with unprecedented accuracy and efficiency. This technology offers a powerful new tool for researchers, clinicians, and healthcare organizations seeking to improve patient care and advance medical understanding.MedLM’s core functionalities revolve around natural language processing (NLP) and machine learning (ML) techniques.
It can analyze unstructured medical data such as doctor’s notes, patient records, research papers, and clinical trial results. This analysis allows for tasks like generating summaries of patient histories, identifying potential risks and diagnoses, assisting in drug discovery, and accelerating medical research. The system is designed with robust privacy and security measures to protect sensitive patient information.
Potential Applications in Healthcare
MedLM’s potential applications across the healthcare sector are vast and transformative. It can significantly improve the efficiency of administrative tasks, such as automating report generation and coding, freeing up clinicians’ time for patient interaction. In research, MedLM can accelerate the discovery of new treatments and therapies by analyzing massive datasets and identifying patterns that might otherwise be missed.
For clinicians, it offers the potential for improved diagnostic accuracy and personalized medicine by providing insights from a comprehensive analysis of patient data. For instance, MedLM could analyze a patient’s medical history and genetic information to predict the likelihood of developing certain diseases, allowing for proactive interventions. Another example could be its use in analyzing medical images alongside patient records for more accurate and timely diagnoses.
Advantages over Traditional Methods
Traditional methods of healthcare data analysis often rely on manual review of records, which is time-consuming, prone to human error, and limited in scale. MedLM offers several key advantages. First, it significantly accelerates the analysis process, enabling faster insights and quicker decision-making. Second, its ability to handle large volumes of unstructured data surpasses the capabilities of human analysts. Third, MedLM can identify subtle patterns and correlations that might escape human observation, leading to more accurate diagnoses and treatment plans.
For example, MedLM could identify a previously unknown correlation between a specific genetic marker and the response to a particular medication, paving the way for personalized treatment strategies. Finally, the consistency and objectivity of MedLM’s analysis reduce the risk of human bias, contributing to fairer and more equitable healthcare.
Google Cloud’s MedLM generative AI is revolutionizing healthcare diagnostics, potentially streamlining processes and improving accuracy. This is particularly exciting when considering early detection of debilitating diseases; for example, research suggests that a simple eye test might be able to predict dementia risk, as detailed in this fascinating article: can eye test detect dementia risk in older adults.
Integrating such findings into MedLM could lead to proactive interventions and improved patient outcomes, showcasing the transformative potential of AI in healthcare.
Specific Use Cases in Healthcare
Google Cloud MedLM, a generative AI model specifically trained on massive healthcare datasets, offers transformative potential across various medical disciplines. Its ability to process and interpret complex medical information opens doors to improved diagnostics, treatment, and administrative efficiency. Let’s delve into some specific applications.
Medical Image Analysis
MedLM’s application in medical image analysis promises significant advancements in diagnostic accuracy and speed. By leveraging its powerful pattern recognition capabilities, it can analyze various imaging modalities to detect anomalies and assist radiologists in their interpretations.
Image Type | MedLM Application | Improvement over Traditional Methods | Potential Risks |
---|---|---|---|
X-Ray | Automated detection of fractures, pneumonia, and other pathologies; generation of preliminary reports. | Faster diagnosis, reduced workload for radiologists, potentially improved accuracy in detecting subtle anomalies. | Potential for misdiagnosis; reliance on the quality of input data; need for human oversight to validate findings. |
MRI | Segmentation of brain tumors, identification of lesions in various organs, quantitative analysis of tissue characteristics. | More precise measurements and visualizations; assistance in treatment planning; potential for earlier detection of diseases. | Computational cost; potential biases in training data leading to inaccurate results for certain patient populations; need for validation and quality control. |
CT Scan | Detection of internal bleeding, identification of organ damage, assessment of bone fractures. | Improved speed and accuracy in identifying critical injuries, particularly in emergency settings; potential for better triage and treatment decisions. | Dependence on image quality; potential for false positives or negatives; the need for experienced clinicians to interpret the AI’s findings. |
Ultrasound | Assessment of fetal development, detection of abnormalities in internal organs, guidance during minimally invasive procedures. | Improved visualization and quantification of anatomical structures; assistance in real-time during procedures; potential for reduced procedural time. | Challenges in handling image variability due to different ultrasound machines and techniques; reliance on high-quality ultrasound images for accurate analysis. |
Drug Discovery and Development
MedLM can accelerate drug discovery by analyzing vast amounts of biomedical literature, identifying potential drug targets, and predicting drug efficacy and safety. For example, MedLM could analyze genomic data to identify patients likely to respond positively to a specific drug, leading to more personalized treatment strategies. This could significantly reduce the time and cost associated with traditional drug development pipelines.
The model could also assist in predicting potential side effects, leading to safer and more effective medications.
Personalized Medicine and Treatment Planning
MedLM’s ability to process and integrate diverse patient data, including genomic information, medical history, and lifestyle factors, enables the creation of personalized treatment plans. By analyzing this data, MedLM can predict the likelihood of treatment success and identify potential risks, allowing clinicians to tailor treatments to individual patients. For instance, a patient’s genetic profile combined with their medical history could be used to predict their risk of developing certain diseases, enabling proactive interventions.
Streamlining Administrative Tasks
MedLM can automate various administrative tasks within healthcare settings, such as medical record summarization, appointment scheduling, and billing processes. This can free up valuable time for healthcare professionals, allowing them to focus on patient care. For example, MedLM could automatically generate summaries of patient charts, saving physicians time and reducing the risk of errors. It could also analyze billing data to identify potential errors and inconsistencies, improving the accuracy and efficiency of the billing process.
Data Security and Privacy Considerations
Leveraging the power of Google Cloud MedLM Generative AI in healthcare necessitates a robust strategy for safeguarding patient data. The sensitive nature of medical information demands meticulous attention to security and privacy, ensuring compliance with regulations and maintaining patient trust. This section details the crucial considerations for implementing MedLM while adhering to the highest standards of data protection.
Patient Data Privacy Strategy for MedLM
A comprehensive strategy for ensuring patient data privacy when using MedLM must be multifaceted. It begins with implementing strong access controls, limiting access to patient data only to authorized personnel with a legitimate need to know. This includes rigorous authentication and authorization mechanisms, utilizing role-based access control (RBAC) to grant specific permissions based on job roles. Furthermore, data encryption, both in transit and at rest, is paramount.
This protects data from unauthorized access even if a breach occurs. Regular security audits and penetration testing should be conducted to identify and address vulnerabilities proactively. Finally, a comprehensive data loss prevention (DLP) strategy, incorporating both technical and procedural safeguards, is essential to prevent accidental or malicious data leaks. This might involve implementing data masking techniques to redact sensitive information before it’s used for training or inference.
Potential Security Risks and Mitigation Strategies
Employing generative AI in healthcare introduces unique security risks. One key risk is the potential for adversarial attacks, where malicious actors manipulate the input data to elicit unintended or harmful outputs from MedLM. Mitigation strategies include robust input validation and sanitization to prevent the injection of malicious code or data. Another risk stems from the inherent complexity of AI models, making them difficult to fully audit and understand.
This necessitates employing explainable AI (XAI) techniques to enhance transparency and traceability of model decisions. Data breaches, whether through external attacks or insider threats, remain a significant concern. Comprehensive security monitoring, including intrusion detection and security information and event management (SIEM) systems, are vital for early detection and response. Regular employee training on security best practices further reduces the risk of human error.
HIPAA Compliance and MedLM
The Health Insurance Portability and Accountability Act (HIPAA) sets stringent standards for the protection of patient health information (PHI). Using MedLM requires strict adherence to HIPAA regulations, encompassing the Privacy Rule, Security Rule, and Breach Notification Rule. This involves implementing appropriate administrative, physical, and technical safeguards to protect PHI from unauthorized access, use, disclosure, alteration, or destruction. A comprehensive risk assessment should be conducted to identify potential HIPAA vulnerabilities and develop mitigation plans.
Google Cloud’s MedLM generative AI has incredible potential to revolutionize healthcare, offering faster diagnoses and personalized treatments. However, the recent news about hshs prevea close wisconsin hospitals health centers highlights the challenges facing healthcare systems, emphasizing the need for innovative solutions like MedLM to improve efficiency and resource allocation, ultimately benefiting patients. This makes the development and implementation of AI tools like MedLM even more critical for the future of healthcare.
Regular audits and compliance reviews are crucial to demonstrate ongoing adherence to HIPAA requirements. Furthermore, meticulous documentation of all data processing activities is essential for demonstrating compliance to auditors. Failure to comply with HIPAA can result in significant penalties.
Data Anonymization Techniques for MedLM
Various data anonymization techniques can be applied to patient data used with MedLM to mitigate privacy risks. Data masking, replacing sensitive data elements with pseudonyms or randomized values, is a common approach. Generalization involves replacing specific values with broader categories, such as age ranges instead of precise ages. Data perturbation adds random noise to the data, making it difficult to identify individual patients while preserving overall data utility.
Differential privacy adds carefully calibrated noise to query results, making it challenging to infer individual data points from aggregate statistics. The choice of anonymization technique depends on the specific data and the desired level of privacy protection. It’s crucial to select methods that strike a balance between privacy preservation and data utility, ensuring the model’s performance isn’t unduly compromised.
For example, de-identification through removing direct identifiers like names and addresses is a starting point, but more sophisticated techniques like k-anonymity or l-diversity may be necessary to protect against re-identification attacks using quasi-identifiers like age, gender, and zip code.
Ethical Implications and Responsible Use
The integration of generative AI like Google Cloud MedLM into healthcare presents immense opportunities but also raises significant ethical concerns. Responsible development and deployment are paramount to ensure patient safety, fairness, and trust in the technology. Careful consideration must be given to potential biases, transparency, and the overall impact on the doctor-patient relationship.
MedLM, like any AI system trained on data, is susceptible to inheriting and amplifying existing biases present in that data. This can lead to inaccurate or discriminatory outcomes, particularly for underrepresented populations. For example, if the training data predominantly reflects the health experiences of one demographic, the model may be less accurate or reliable when applied to others.
The potential for biased predictions in diagnosis, treatment recommendations, or risk assessment is a serious ethical challenge that demands proactive mitigation strategies.
Bias Mitigation Strategies in MedLM
Addressing bias requires a multi-pronged approach. First, rigorous auditing of the training data is crucial to identify and correct imbalances. This involves careful examination of the dataset for overrepresentation or underrepresentation of specific demographic groups and conditions. Second, techniques like re-weighting samples or employing fairness-aware algorithms can help to adjust the model’s predictions and reduce bias during the training process.
Finally, ongoing monitoring of MedLM’s performance across different patient populations is essential to detect and address any emerging biases after deployment. Regular performance evaluations and comparisons across diverse groups can reveal disparities and inform necessary adjustments. For example, if MedLM consistently underperforms in predicting a particular condition for a specific demographic, it highlights a need for further data collection, algorithm refinement, or additional training data representing that demographic.
Transparency and Explainability of MedLM Outputs
Transparency and explainability are critical for building trust and ensuring accountability. Healthcare professionals need to understand how MedLM arrives at its conclusions to assess the reliability and validity of its recommendations. A “black box” AI system, where the decision-making process is opaque, is unlikely to gain acceptance in the healthcare setting. MedLM’s developers should strive for methods that allow for the interpretation of the model’s reasoning, such as providing explanations for its predictions, highlighting the key features used in decision-making, and quantifying the uncertainty associated with its outputs.
This level of transparency enables clinicians to critically evaluate MedLM’s suggestions, integrate them appropriately into their clinical judgment, and maintain ultimate responsibility for patient care. For example, providing a clear breakdown of the factors contributing to a diagnosis, along with confidence levels for each factor, allows the physician to judge the model’s output against their clinical experience.
Best Practices for Responsible Implementation of MedLM
Implementing MedLM responsibly requires a commitment to ethical guidelines and robust oversight. A comprehensive strategy should include:
The following best practices are essential for the safe and ethical use of MedLM:
- Establish clear guidelines and protocols for the use of MedLM, defining its appropriate applications and limitations within the healthcare setting.
- Implement rigorous data validation and bias mitigation strategies throughout the entire lifecycle of MedLM, from data collection to model deployment and ongoing monitoring.
- Prioritize transparency and explainability, ensuring that healthcare professionals can understand and interpret MedLM’s outputs.
- Foster collaboration and communication between AI developers, healthcare professionals, ethicists, and regulatory bodies to address ethical challenges and ensure responsible innovation.
- Develop mechanisms for ongoing monitoring and evaluation of MedLM’s performance and impact, including the assessment of potential biases and unintended consequences.
- Provide comprehensive training and education for healthcare professionals on the appropriate use and interpretation of MedLM’s outputs.
- Establish robust data security and privacy protocols to protect patient information and maintain confidentiality.
Integration with Existing Healthcare Systems
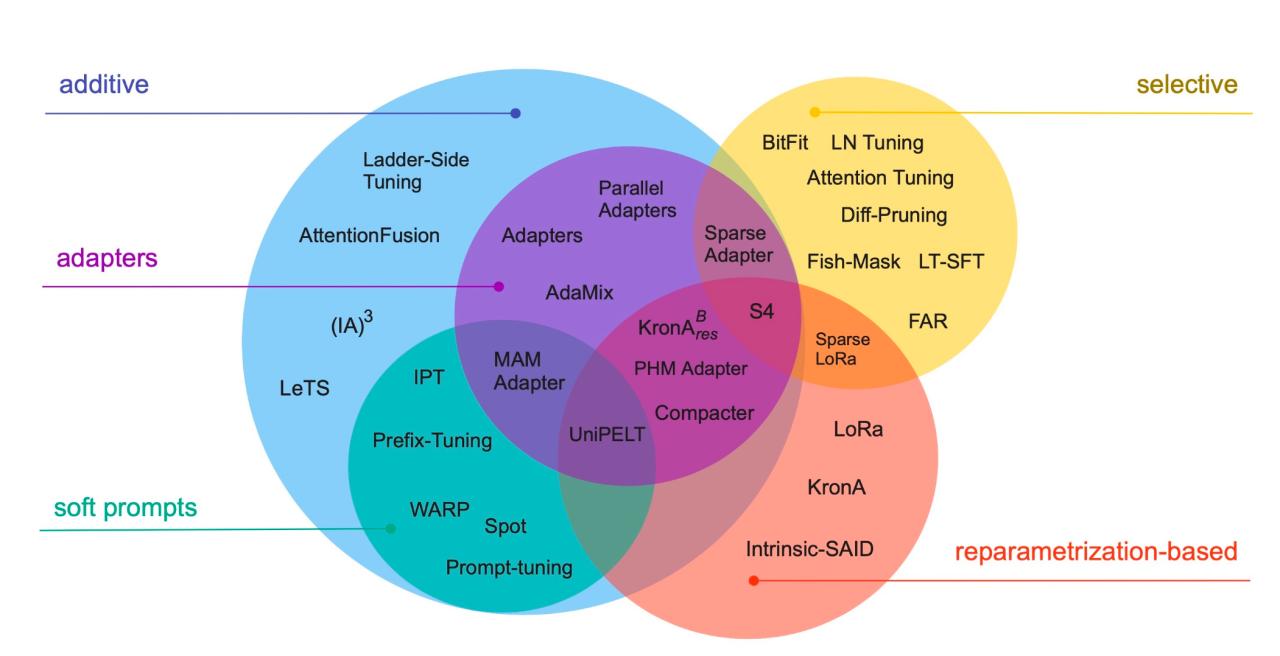
Source: substackcdn.com
Integrating Google Cloud MedLM Generative AI into existing healthcare infrastructures presents both opportunities and challenges. Successful implementation requires careful planning, a phased approach, and a deep understanding of the specific EHR system in use. The goal is seamless data flow and minimal disruption to existing workflows. This section will explore the practical aspects of this integration process.MedLM’s integration with Electronic Health Records (EHR) systems hinges on the ability to securely access and process patient data while adhering to strict privacy regulations like HIPAA.
This involves establishing secure APIs and data transfer protocols to move relevant information between MedLM and the EHR. The complexity of this process varies significantly depending on the EHR system’s architecture and its openness to external integrations. Furthermore, careful consideration must be given to data normalization and transformation to ensure compatibility between the different systems.
MedLM Integration with EHR Systems: A Step-by-Step Guide
Implementing MedLM in a hospital setting is a multi-stage process requiring collaboration between IT specialists, clinicians, and data scientists. A phased rollout minimizes risk and allows for iterative improvements.
- Assessment and Planning: This initial phase involves a thorough assessment of the hospital’s existing IT infrastructure, including its EHR system, network security, and data storage capabilities. The specific needs and use cases for MedLM are also defined, determining the scope of the integration project.
- API Development and Testing: Secure APIs are developed to facilitate data exchange between MedLM and the EHR. Rigorous testing is crucial to ensure data integrity, security, and compliance with relevant regulations. This phase may involve creating custom connectors or leveraging existing EHR APIs.
- Data Migration and Transformation: Patient data relevant to MedLM’s functionalities is extracted from the EHR and transformed into a format compatible with MedLM’s input requirements. Data anonymization and de-identification techniques are applied to ensure patient privacy.
- Pilot Implementation: A small-scale pilot program is implemented in a controlled environment to test the integrated system’s performance and identify any potential issues before full deployment.
- Full Deployment and Monitoring: Once the pilot program is successful, MedLM is fully deployed across the hospital. Continuous monitoring and performance evaluation are essential to ensure the system operates effectively and meets the hospital’s needs.
Integration Challenges Across Different EHR Systems
Different EHR systems possess varying levels of API maturity and integration capabilities. Older, less flexible systems may present significant challenges compared to newer, more modern systems with robust APIs. Furthermore, the specific data formats and structures used by different EHR systems can impact the complexity of data integration. For instance, integrating MedLM with a system using a proprietary, poorly documented API will require significantly more development effort than integrating with a system using a widely adopted standard like FHIR (Fast Healthcare Interoperability Resources).
Differences in data security protocols and authentication methods can also pose challenges.
Examples of Successful Generative AI Integrations in Healthcare
While specific details of MedLM integrations are currently limited due to its recent release, several examples exist of successful generative AI integrations in healthcare settings. For example, several companies have successfully integrated AI models into radiology departments to assist with image analysis and report generation, speeding up diagnostic processes and improving accuracy. Similarly, AI-powered chatbots have been implemented in various healthcare settings to provide patients with basic information, schedule appointments, and answer frequently asked questions, improving patient experience and freeing up staff time.
These successful deployments highlight the potential of generative AI to transform healthcare operations. The key to success in these implementations has been a focus on user-centric design, robust data security, and a phased rollout strategy.
Google Cloud’s MedLM generative AI is poised to revolutionize healthcare, offering powerful tools for diagnosis and treatment planning. This advancement builds on existing AI initiatives like the impressive expansion of Google’s iCAD AI mammography capabilities, as detailed in this article: Google iCAD AI mammography expansion. This shows Google’s commitment to improving healthcare through AI, and we can expect MedLM to further enhance precision and efficiency across various medical specialties.
Future Directions and Potential Advancements
The integration of Google Cloud MedLM generative AI into healthcare is still in its nascent stages, yet its potential to revolutionize the field is immense. The rapid advancements in large language models and their application to complex medical data promise a future where diagnosis, treatment, and patient care are significantly enhanced, leading to improved health outcomes globally. This section explores some of the most promising future directions and potential advancements of MedLM in healthcare.
MedLM’s ability to process and analyze vast amounts of medical data opens doors to previously unimaginable applications. Its potential extends far beyond current uses, impacting various aspects of healthcare delivery and research.
Improved Diagnostic Accuracy and Speed
MedLM’s capacity for pattern recognition and anomaly detection could significantly improve the accuracy and speed of medical diagnoses. Imagine a system that can analyze medical images (X-rays, CT scans, MRIs) with unparalleled speed and precision, identifying subtle anomalies that might be missed by the human eye. Furthermore, by integrating data from electronic health records (EHRs), genetic information, and wearable sensor data, MedLM can provide a more holistic and accurate diagnostic assessment, potentially leading to earlier interventions and better treatment outcomes.
For example, early detection of cancerous tumors through analysis of medical images coupled with patient history could dramatically improve survival rates.
Advanced Prognostic Modeling and Personalized Treatment Plans, Google cloud medlm generative ai in healthcare
By analyzing a patient’s medical history, genetic predisposition, lifestyle factors, and environmental influences, MedLM can create highly personalized prognostic models. This allows for the prediction of disease progression and the identification of high-risk individuals who may benefit from preventative measures or early intervention. Moreover, MedLM can aid in the development of tailored treatment plans, optimizing therapy based on individual patient characteristics and predicted responses.
This personalized approach could significantly improve treatment efficacy and reduce adverse effects. For instance, predicting the likelihood of a patient experiencing a heart attack based on their risk factors and tailoring their medication regimen accordingly.
Accelerated Drug Discovery and Development
MedLM can significantly accelerate the drug discovery and development process by analyzing vast amounts of research data, identifying potential drug candidates, and predicting their efficacy and safety. This could lead to the faster development of new treatments for various diseases, including those currently lacking effective therapies. The ability to analyze complex biological pathways and predict drug interactions could significantly reduce the time and cost associated with bringing new drugs to market.
A real-world example is the accelerated identification of potential drug candidates for rare genetic diseases by analyzing genomic data and identifying potential therapeutic targets.
Addressing Global Health Challenges
MedLM has the potential to address pressing global health challenges. Its ability to translate medical information across languages can facilitate better communication and collaboration between healthcare professionals worldwide. Moreover, MedLM can be deployed in resource-limited settings to provide access to expert medical knowledge and improve diagnostic capabilities, even in areas lacking sufficient medical infrastructure. For example, deploying MedLM in remote areas to aid in the diagnosis of infectious diseases or providing access to medical expertise for patients in underserved communities.
Future Research and Development Areas
Further research is needed to address several crucial areas. These include improving the explainability and transparency of MedLM’s decision-making processes, ensuring data privacy and security, mitigating potential biases in the training data, and establishing ethical guidelines for the responsible use of this technology. Research into the integration of MedLM with other AI technologies, such as computer vision and natural language processing, will also be crucial for realizing its full potential.
Specifically, developing methods for verifying the accuracy and reliability of MedLM’s predictions and ensuring the responsible use of AI in clinical decision-making.
Impact on the Healthcare Industry
The widespread adoption of MedLM will likely transform the healthcare industry, leading to increased efficiency, improved patient outcomes, and reduced healthcare costs. However, this transformation will also require significant changes in healthcare workflows, training programs for healthcare professionals, and regulatory frameworks. The integration of MedLM will necessitate careful consideration of ethical implications, data privacy, and the potential displacement of certain healthcare roles.
The long-term impact will depend on the responsible development and deployment of this powerful technology. A potential scenario is a shift towards a more preventative and personalized approach to healthcare, with a greater emphasis on data-driven decision-making.
Final Summary
The potential of Google Cloud MedLM Generative AI in healthcare is truly transformative. While challenges remain in data security, ethical considerations, and integration with existing systems, the benefits for improved patient care, accelerated research, and streamlined workflows are undeniable. As we move forward, responsible development and implementation will be key to unlocking the full potential of this technology and ensuring its ethical and beneficial use for everyone.
Helpful Answers: Google Cloud Medlm Generative Ai In Healthcare
What is the cost of using Google Cloud MedLM?
Pricing varies depending on usage and specific services utilized. Check Google Cloud’s pricing page for detailed information.
How does MedLM ensure the accuracy of its diagnoses?
MedLM’s accuracy depends on the quality and quantity of data it’s trained on. Ongoing validation and refinement are crucial, and human oversight remains essential in interpreting its results.
What happens if there’s a system failure during a critical procedure relying on MedLM?
Robust redundancy and fail-safe mechanisms are essential. Healthcare providers must have backup plans and protocols in place to handle potential system failures.
Can MedLM replace human doctors?
No. MedLM is a powerful tool to assist healthcare professionals, not replace them. Human expertise and judgment are crucial in healthcare, and AI should augment, not substitute, human capabilities.