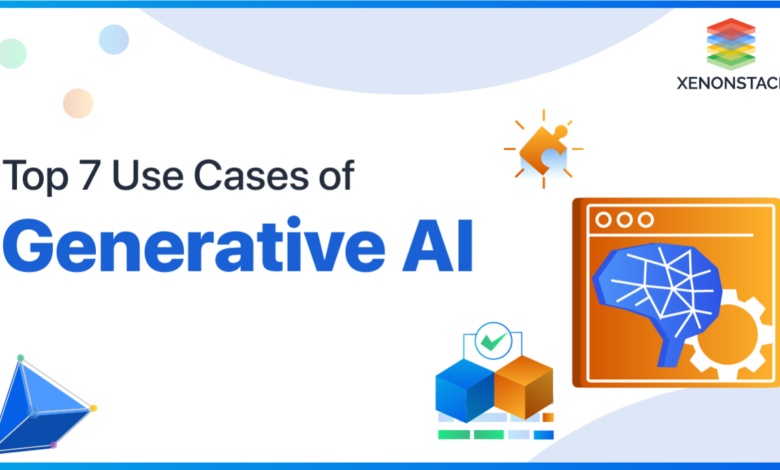
Google Mayo Generative AI Hospital Research
Google Mayo Generative AI Hospital Research: Imagine a future where AI accelerates breakthroughs in medicine, dramatically improving patient care. This collaboration between a tech giant and a leading medical institution is poised to revolutionize how we approach hospital research, tackling complex challenges with unprecedented speed and accuracy. This post delves into the exciting possibilities and potential pitfalls of this partnership, exploring how generative AI is transforming healthcare.
We’ll examine Google’s specific contributions, the ways Mayo Clinic is leveraging this technology, and the broader implications for the future of medical research. From accelerating drug discovery to enhancing medical image analysis, the potential benefits are immense. But, we’ll also discuss crucial ethical considerations, data privacy concerns, and the regulatory hurdles that need to be navigated.
Google’s Role in Generative AI for Healthcare

Source: deltalogix.blog
Google is rapidly expanding its presence in the healthcare sector, leveraging its considerable expertise in artificial intelligence, particularly generative AI, to revolutionize hospital research. This involves significant investments in both developing novel AI algorithms and collaborating with healthcare institutions to implement these technologies. The potential implications are vast, promising to accelerate research timelines, improve diagnostic accuracy, and ultimately, enhance patient care.Google’s current investments and initiatives in generative AI for healthcare are multifaceted.
They encompass the development of sophisticated machine learning models capable of analyzing massive datasets of medical images, patient records, and research literature. These models can identify patterns and insights that would be impossible for humans to detect manually, leading to breakthroughs in disease diagnosis, treatment optimization, and drug discovery. Specific initiatives include collaborations with major hospitals and research institutions to pilot AI-powered diagnostic tools, predictive modeling for patient outcomes, and the creation of AI-driven platforms for accelerating clinical trials.
These partnerships are crucial, providing Google with access to real-world data and clinical expertise, while simultaneously offering hospitals access to cutting-edge AI capabilities.
Potential Benefits of Google’s AI Technologies for Improving Hospital Research Efficiency
The application of Google’s AI technologies holds the potential to significantly enhance the efficiency of hospital research. For example, generative AI models can automate tedious tasks like data entry and cleaning, freeing up researchers to focus on more complex analytical work. Furthermore, these models can accelerate the process of literature review by quickly identifying relevant research papers and summarizing key findings.
This accelerates the pace of discovery, allowing researchers to test hypotheses and develop new treatments more rapidly. In drug discovery, generative AI can design and test potential drug candidates, significantly reducing the time and cost associated with traditional methods. The ability to predict patient outcomes with greater accuracy can also lead to more targeted and effective treatment strategies, ultimately improving patient care and reducing healthcare costs.
Consider the example of a clinical trial for a new cancer drug; AI could analyze patient data to identify subgroups most likely to benefit, leading to a more efficient and effective trial.
Comparison of Google’s Approach to Generative AI in Healthcare with Other Major Tech Companies
Google’s approach to generative AI in healthcare differs somewhat from other major tech companies. While many companies focus on developing specific AI-powered tools for diagnosis or treatment, Google seems to be pursuing a more holistic strategy, investing in both the development of foundational AI models and in partnerships with healthcare institutions to implement these models in real-world settings. This collaborative approach contrasts with some companies that primarily focus on licensing their technology to healthcare providers, rather than engaging in deep collaborative research and development.
Microsoft, for instance, has also made significant investments in AI for healthcare, but their focus has been more on integrating AI into their existing cloud platforms and software solutions. Amazon Web Services also offers cloud-based AI tools for healthcare, but their approach is primarily focused on providing infrastructure and services, rather than developing proprietary AI models for specific healthcare applications.
The different approaches reflect varying corporate strategies and priorities.
Ethical Considerations Surrounding Google’s Involvement in Hospital Research Using Generative AI
The use of generative AI in healthcare raises several ethical considerations, particularly regarding data privacy, algorithmic bias, and the potential displacement of human clinicians. Google’s involvement in this field necessitates a commitment to transparency and responsible AI development. Ensuring the privacy and security of patient data is paramount; rigorous data anonymization and access control mechanisms are essential to prevent breaches and misuse.
Algorithmic bias is another critical concern; AI models trained on biased datasets may perpetuate existing health disparities. Google must actively work to mitigate bias in its algorithms and ensure equitable access to AI-powered healthcare solutions. Finally, the potential displacement of human clinicians through automation must be addressed thoughtfully. The focus should be on augmenting human capabilities, not replacing them entirely, ensuring that AI technologies are used to enhance, not diminish, the role of healthcare professionals.
Mayo Clinic’s Use of Generative AI: Google Mayo Generative Ai Hospital Research
The Mayo Clinic, a global leader in medical research and patient care, is actively exploring the potential of generative AI to revolutionize various aspects of its operations. While specific details about their projects are often kept confidential due to competitive and intellectual property considerations, publicly available information and industry trends suggest a growing commitment to leveraging this technology. This exploration focuses on enhancing research capabilities, improving patient care, and streamlining administrative tasks.
Generative AI’s ability to analyze vast datasets, identify patterns, and generate novel hypotheses makes it a particularly valuable tool for the complex challenges faced in medical research. Its applications range from drug discovery and personalized medicine to improving diagnostic accuracy and accelerating the pace of clinical trials.
Areas of Generative AI Research at Mayo Clinic
The Mayo Clinic’s research into generative AI is likely spread across several key areas. These include the analysis of medical images (radiology, pathology), genomics and proteomics research for identifying disease biomarkers and predicting treatment responses, and the development of more efficient and effective clinical trial designs. While precise project details are limited, the overarching goal appears to be using generative AI to accelerate research timelines, improve diagnostic accuracy, and ultimately, enhance patient outcomes.
Examples of Successful Generative AI Applications in Mayo Clinic Research
While specific case studies remain largely undisclosed, we can infer successful applications based on the capabilities of generative AI and the Mayo Clinic’s research priorities. For instance, generative AI models could be trained on massive datasets of medical images to identify subtle anomalies indicative of diseases like cancer at earlier stages, potentially improving diagnostic accuracy and leading to earlier and more effective interventions.
Similarly, in genomics research, generative AI could be used to predict the likelihood of a patient responding favorably to a specific treatment based on their genetic profile, paving the way for truly personalized medicine. Another area is the analysis of patient records to identify risk factors and predict potential health problems, allowing for proactive interventions and preventative care.
Challenges in Integrating and Implementing Generative AI Technologies at Mayo Clinic
The integration of generative AI into the Mayo Clinic’s established research infrastructure presents several significant challenges. Data privacy and security are paramount concerns, given the sensitive nature of patient information. Ensuring compliance with HIPAA regulations and other relevant data protection laws is crucial. Additionally, the need for robust validation and verification of AI-generated results is essential to maintain the high standards of accuracy and reliability expected in medical research.
Furthermore, the computational resources required to train and deploy sophisticated generative AI models are substantial, necessitating significant investment in computing infrastructure. Finally, the ethical implications of using AI in healthcare, such as bias in algorithms and the potential for job displacement, require careful consideration and proactive mitigation strategies.
Hypothetical Scenario: Enhancing a Mayo Clinic Research Initiative
Imagine a Mayo Clinic research initiative focused on understanding the complex interplay of genetic factors and environmental exposures in the development of Alzheimer’s disease. Generative AI could significantly enhance this research by analyzing massive datasets of genomic information, environmental data (e.g., air quality, exposure to toxins), and clinical records. The AI could identify previously unknown correlations between specific genetic variations, environmental factors, and the progression of Alzheimer’s.
This could lead to the identification of novel biomarkers, the development of targeted preventative strategies, and the acceleration of drug discovery efforts. For example, the AI might uncover a previously unknown interaction between a specific gene variant and exposure to certain heavy metals, significantly increasing the risk of developing Alzheimer’s. This insight could then be used to develop targeted screening programs for high-risk individuals and to guide the development of new therapies.
Generative AI Applications in Hospital Research
Generative AI holds immense potential to revolutionize hospital research, accelerating breakthroughs in various fields and improving the efficiency of existing processes. Its ability to generate novel data, analyze complex patterns, and predict outcomes makes it a powerful tool for tackling challenging research problems. This section explores some key applications within the hospital research setting.
Accelerating Drug Discovery and Development
Generative AI models can significantly accelerate drug discovery and development by generating novel molecular structures with desired properties. Instead of relying solely on traditional trial-and-error methods, researchers can use these models to predict the efficacy and safety of potential drug candidates, drastically reducing the time and cost associated with the drug development pipeline. For example, generative models can be trained on vast datasets of existing drugs and their properties to identify promising new compounds.
These models can then be used to design and synthesize new molecules, which can then be tested in pre-clinical and clinical trials. This approach allows researchers to focus on the most promising candidates, leading to faster development and potentially more effective treatments. One example of this is the use of generative models to design new antibiotics to combat antibiotic-resistant bacteria, a pressing global health concern.
Improving Medical Image Analysis
Generative AI can enhance the accuracy and speed of medical image analysis in research. These models can be trained on large datasets of medical images (X-rays, CT scans, MRIs) to learn the complex patterns associated with different diseases. Once trained, they can then be used to automatically detect and classify abnormalities in new images, assisting researchers in identifying potential diagnoses and monitoring disease progression.
This automation can significantly reduce the workload for radiologists and other medical professionals, allowing them to focus on more complex cases. Furthermore, generative AI can aid in creating synthetic medical images for training other AI models or augmenting existing datasets, addressing the issue of limited real-world data. For example, generative models can be used to create realistic synthetic images of tumors with varying characteristics, providing researchers with a more comprehensive dataset for training diagnostic models.
Comparison of Generative AI Models for Hospital Research Tasks
The choice of generative AI model depends heavily on the specific research task. Below is a comparison of some popular models and their capabilities:
Model | Task | Strengths | Weaknesses |
---|---|---|---|
Variational Autoencoders (VAEs) | Image generation, anomaly detection | Good at generating diverse images, relatively efficient to train | Generated images can sometimes lack fidelity |
Generative Adversarial Networks (GANs) | Image generation, drug discovery | Excellent at generating high-fidelity images, capable of generating complex molecules | Difficult to train, prone to mode collapse |
Transformer-based models | Sequence generation (e.g., protein sequences), text analysis | Excellent at capturing long-range dependencies, highly adaptable | Computationally expensive, requires large datasets |
Graph Neural Networks (GNNs) | Drug discovery, analysis of biological networks | Can effectively model relationships between molecules and biological entities | Can be challenging to train and interpret |
Potential Biases in Generative AI for Hospital Research
It’s crucial to acknowledge the potential for biases in generative AI models used in hospital research. These biases can significantly impact the reliability and fairness of research findings.
Google and Mayo Clinic’s generative AI research in hospitals is fascinating, exploring how AI can improve patient care and streamline workflows. This reminds me of another exciting development: Nuance’s integration of generative AI scribe technology with Epic EHRs, as detailed in this article nuance integrates generative ai scribe epic ehrs. The advancements in AI-powered tools like this are directly relevant to Google and Mayo’s goals of enhancing the efficiency and accuracy of hospital processes.
Ultimately, these initiatives aim to revolutionize healthcare delivery.
- Data Bias: If the training data is not representative of the diverse population being studied, the model may exhibit biases in its predictions, leading to inaccurate or unfair outcomes. For example, a model trained primarily on data from one ethnic group may not perform well on data from other groups.
- Algorithmic Bias: The design and architecture of the model itself can introduce biases. Certain algorithms might inherently favor specific patterns or features in the data, leading to biased results.
- Label Bias: Inaccuracies or inconsistencies in the labeling of training data can also lead to biases in the model’s predictions. This is particularly important in medical image analysis, where the accuracy of diagnoses relies heavily on accurate labeling.
- Confirmation Bias: Researchers might inadvertently select or interpret results that confirm their pre-existing beliefs, even if the model’s predictions are biased.
Data Privacy and Security Concerns
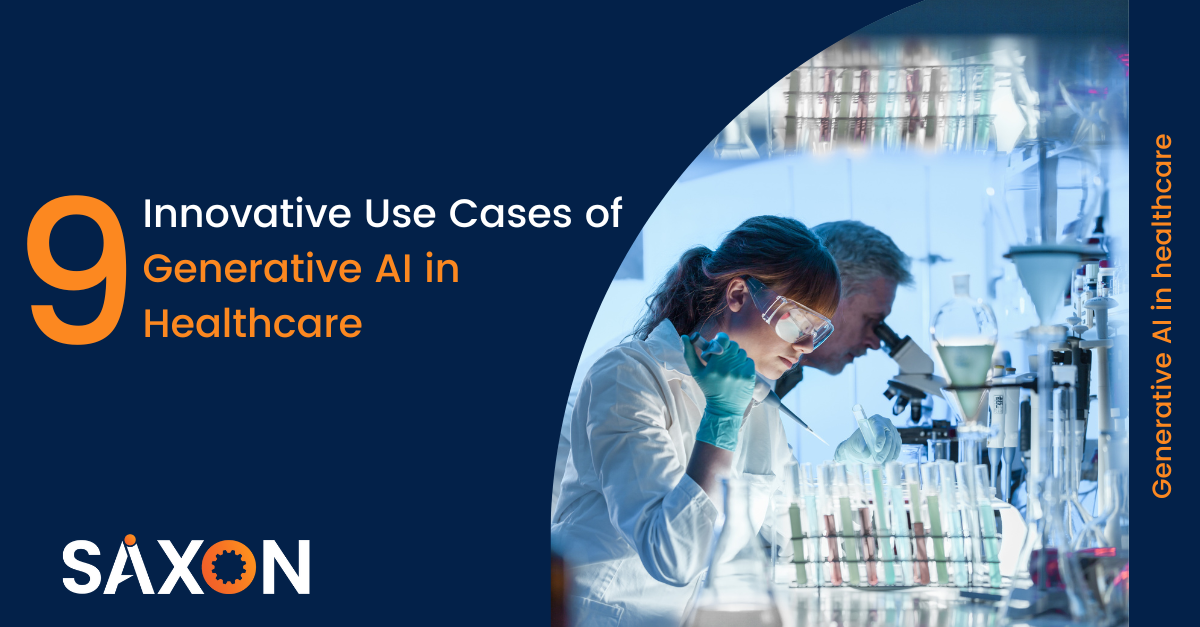
Source: saxon.ai
The integration of generative AI into hospital research presents significant opportunities for advancement, but it also raises serious concerns about patient data privacy and security. The very nature of generative AI, which learns from vast datasets to create new content, necessitates the use of sensitive patient information, making robust security measures paramount. Failure to adequately protect this data could lead to breaches, compromising patient confidentiality and potentially causing significant harm.The sheer volume and sensitivity of health data pose unique challenges.
Google and Mayo Clinic’s generative AI research in healthcare is incredibly exciting, especially considering its potential to revolutionize diagnostics and treatment. Thinking about the challenges faced by smaller facilities, I was reminded of the article on Rural Hospitals Labor Delivery & which highlights the disparities in access to quality care. This makes me wonder how AI could help bridge that gap, potentially offering remote diagnostic support and improving outcomes even in underserved areas.
Ultimately, this Google/Mayo collaboration could be a game-changer for equitable healthcare access.
Generative AI models require substantial training datasets, often including protected health information (PHI) such as medical records, imaging data, and genetic information. This data is subject to strict regulations, and any unauthorized access or disclosure could have severe legal and ethical consequences.
Google and Mayo Clinic’s generative AI research in hospital settings is fascinating, exploring how AI can improve patient care and streamline processes. This makes me wonder about the implications of Mass General Brigham’s recent move to buyout its digital unit, as reported here: Mass General Brigham Buyouts Digital Unit. This kind of consolidation could either accelerate or hinder the adoption of similar AI technologies in other major hospital systems, impacting the future trajectory of Google and Mayo’s research.
Data Privacy Protection Methods
Ensuring the privacy and security of patient data used in generative AI models requires a multi-layered approach. Different techniques offer varying levels of protection, each with its own strengths and weaknesses. Choosing the appropriate methods depends on the specific application, the sensitivity of the data, and the regulatory environment.One common approach is data anonymization, which involves removing or altering identifying information from the dataset.
However, even anonymized data can be re-identified through sophisticated techniques, especially when combined with other publicly available information. Differential privacy, a more robust method, adds carefully calibrated noise to the data, making it difficult to extract individual patient information while preserving the overall statistical properties. Federated learning allows models to be trained on decentralized data sources without directly sharing the data itself, minimizing the risk of breaches.
Homomorphic encryption enables computations to be performed on encrypted data without decryption, offering a high level of security.
Regulatory Landscape and Impact on Hospital Research
The regulatory landscape surrounding the use of AI in healthcare is constantly evolving, but existing regulations like HIPAA in the United States and GDPR in Europe place stringent requirements on the handling of patient data. These regulations mandate data minimization, purpose limitation, and appropriate security measures. Failure to comply can result in significant penalties, including substantial fines and reputational damage.
Furthermore, research institutions must obtain appropriate ethical approvals and informed consent from patients before using their data for AI development. The increasing complexity of AI models and the potential for unintended biases further complicate the regulatory landscape, requiring ongoing adaptation and careful oversight.
Hypothetical Data Security Protocol
A robust data security protocol for protecting sensitive patient information during generative AI research would incorporate several key elements. First, data would be anonymized or pseudonymized using a combination of techniques, including differential privacy and data masking. Second, access to the data would be strictly controlled through role-based access control, with only authorized personnel having permission to access specific datasets.
Third, the entire research process, from data collection to model deployment, would be subject to rigorous auditing and logging to ensure accountability and traceability. Fourth, robust encryption techniques, both in transit and at rest, would be implemented to protect the data from unauthorized access. Finally, regular security assessments and penetration testing would be conducted to identify and address vulnerabilities.
This protocol would also include a comprehensive incident response plan to mitigate the impact of any potential breaches. For example, if a breach were to occur, the protocol would dictate immediate notification of relevant authorities and affected individuals, along with a thorough investigation to determine the cause and extent of the breach and implement corrective actions.
Future Trends and Predictions
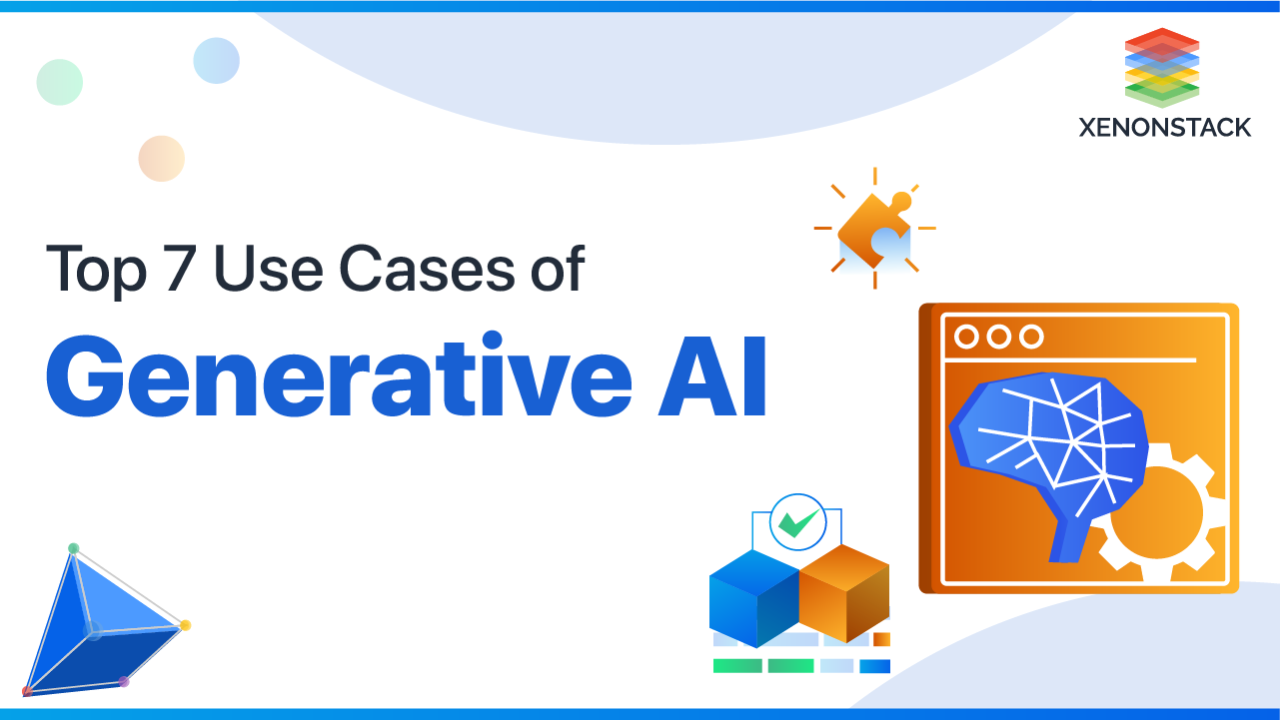
Source: xenonstack.com
Generative AI is poised to dramatically reshape hospital research in the coming decade. Its ability to analyze vast datasets, generate hypotheses, and even design experiments promises a new era of accelerated discovery and improved patient outcomes. We’re moving beyond simple data analysis towards a future where AI actively participates in the scientific process itself.The next five to ten years will likely witness significant advancements in several key areas, leading to breakthroughs that were previously unimaginable.
Enhanced Drug Discovery and Development
Generative AI algorithms will become increasingly sophisticated in their ability to predict the efficacy and safety of new drugs. By analyzing massive datasets of molecular structures and biological activity, these algorithms can design novel drug candidates with improved properties. For example, imagine an AI system predicting the effectiveness of a potential cancer treatment against specific tumor types with far greater accuracy than current methods, significantly reducing the time and cost associated with clinical trials.
This will involve integrating multi-omics data (genomics, proteomics, metabolomics) with clinical trial results to build more robust predictive models. The increased accuracy in early-stage drug development will ultimately lead to faster translation of research into effective therapies.
Personalized Medicine Advancements
The application of generative AI in personalized medicine will move beyond simple risk stratification. We can anticipate AI systems capable of generating personalized treatment plans based on an individual patient’s unique genetic makeup, lifestyle, and medical history. For instance, an AI could design a tailored cancer treatment regimen incorporating specific genetic mutations and the patient’s response to previous therapies, optimizing treatment efficacy while minimizing side effects.
This could involve the generation of synthetic patient data to train and validate models while maintaining patient privacy. The result would be a significant improvement in the effectiveness and safety of medical treatments.
Improved Diagnostic Accuracy, Google mayo generative ai hospital research
Generative AI models will be instrumental in enhancing the accuracy and speed of medical diagnoses. By analyzing medical images (X-rays, CT scans, MRIs), these models can identify subtle anomalies that might be missed by human experts. Imagine an AI system capable of detecting early signs of cancer or other diseases with unparalleled precision, leading to earlier intervention and improved patient survival rates.
This will likely involve the development of explainable AI models to improve the trust and acceptance of these technologies by medical professionals. The increased accuracy and speed of diagnosis will lead to better resource allocation and potentially reduced healthcare costs.
Future Scenario: AI-Driven Cancer Research
Imagine a future scenario in 2030 where a team of researchers is working on a novel cancer therapy. Instead of relying solely on manual experimentation and data analysis, they leverage a powerful generative AI system. This AI analyzes massive datasets of genomic information, protein interactions, and clinical trial results to identify potential drug targets. It then designs multiple drug candidates, predicts their efficacy and toxicity, and even suggests optimal dosing strategies.
The AI also helps design and optimize clinical trials, predicting patient response and identifying potential adverse events. This AI-driven approach dramatically accelerates the drug development process, leading to the creation of a highly effective cancer treatment in a fraction of the time it would have taken using traditional methods. The result is a significant improvement in patient survival rates and a substantial reduction in the overall cost of cancer treatment.
Predicted Growth and Impact: A Visual Representation
Imagine a graph. The X-axis represents time, from 2023 to 2033. The Y-axis represents the impact of generative AI on hospital research, measured by a composite index incorporating factors like speed of drug discovery, diagnostic accuracy improvements, and the number of AI-assisted research publications. The graph shows a relatively slow but steady increase in impact from 2023 to 2028.
Then, from 2028 onwards, the curve sharply ascends, reflecting a rapid acceleration in the adoption and effectiveness of generative AI in hospital research. The steep incline symbolizes a dramatic transformation in the research landscape, with AI becoming an indispensable tool for medical discovery and innovation. The overall shape of the curve resembles a hockey stick, vividly illustrating the transformative potential of generative AI.
Ending Remarks
The partnership between Google and the Mayo Clinic in generative AI for hospital research represents a pivotal moment in medical innovation. While challenges remain – particularly regarding data privacy and ethical considerations – the potential for transformative advancements in healthcare is undeniable. The future of medical research is being shaped by this collaboration, and the implications are far-reaching, promising a future where diagnoses are faster, treatments are more effective, and patient outcomes are significantly improved.
This is just the beginning of a fascinating journey into the intersection of AI and healthcare.
FAQ Summary
What specific types of generative AI models are being used?
The specific models aren’t publicly detailed, but it’s likely a mix of large language models (LLMs) for analyzing text data, and other models specialized for image analysis and drug discovery.
How is patient data anonymized to protect privacy?
Robust anonymization techniques, including differential privacy and federated learning, are likely employed to minimize the risk of identifying individual patients.
What are the biggest hurdles to widespread adoption of AI in hospital research?
Major hurdles include the cost of implementing AI systems, the need for substantial data sets, and the development of reliable validation methods to ensure accuracy and avoid bias.
What are some examples of rival tech companies working in this space?
Companies like IBM Watson Health and Microsoft are also heavily invested in AI for healthcare, often focusing on similar applications to Google and Mayo Clinic’s efforts.