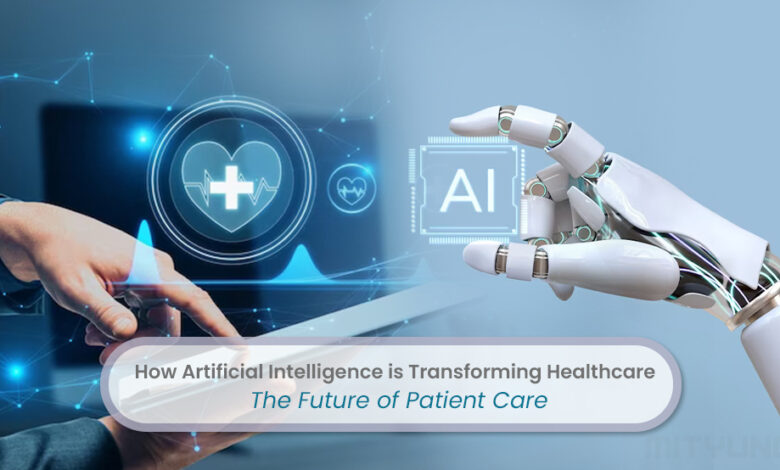
Health AI Governance Trump Admin Standards
Health artificial intelligence governance trump admin standards: Navigating the complex landscape of AI in healthcare during the Trump administration reveals a fascinating blend of opportunity and challenge. This period saw a push for AI’s potential to revolutionize healthcare access, quality, and affordability, yet also grappled with the ethical, legal, and regulatory hurdles inherent in deploying such powerful technology.
We’ll delve into the specific policies, executive orders, and regulatory actions that shaped this era, exploring the successes, shortcomings, and lasting impact on the field.
From analyzing the existing regulatory frameworks and their limitations to examining crucial data privacy concerns under HIPAA, we’ll uncover the key challenges faced in balancing innovation with patient safety and ethical considerations. We’ll also explore the ethical dilemmas surrounding algorithmic bias and the importance of transparency and accountability in AI-driven healthcare decisions. The comparison with international approaches to AI governance will further illuminate the unique context of the Trump administration’s policies and their global implications.
Trump Administration’s Approach to AI in Healthcare
The Trump administration’s approach to artificial intelligence in healthcare was characterized by a focus on promoting innovation and reducing regulatory burdens, while simultaneously emphasizing patient safety and data privacy. While no single, comprehensive AI healthcare policy emerged, the administration’s actions and statements reveal a strategy prioritizing market-driven solutions and technological advancement in the sector.
Stated Policies Regarding AI in Healthcare
The Trump administration’s official pronouncements regarding AI in healthcare were largely incorporated into broader initiatives focused on technological advancement and regulatory reform across various sectors. Emphasis was placed on fostering a regulatory environment conducive to innovation, particularly through streamlining the approval processes for new technologies. This aimed to accelerate the development and deployment of AI-powered tools in healthcare, ultimately improving efficiency and patient outcomes.
Specific policy documents directly addressing AI in healthcare were less common than pronouncements emphasizing broader technological innovation and regulatory streamlining. The administration consistently stressed the potential of AI to address challenges related to healthcare costs, access, and quality.
Executive Orders, Legislation, and Regulatory Actions
While no specific executive orders directly targeted AI in healthcare, several initiatives indirectly impacted the field. For instance, executive orders promoting regulatory reform and reducing bureaucratic hurdles likely fostered a more favorable environment for AI development and deployment in healthcare. Similarly, initiatives focused on data privacy and cybersecurity, although not exclusively focused on AI, played a crucial role in shaping the landscape for AI applications involving sensitive patient data.
The administration’s focus on deregulation arguably created an environment where companies could more easily introduce AI-powered medical devices and software, although this approach also faced criticism regarding potential safety concerns.
Priorities Concerning AI’s Role in Improving Healthcare, Health artificial intelligence governance trump admin standards
The Trump administration’s priorities regarding AI’s role in healthcare largely centered on improving access, quality, and affordability. Improving access was seen as achievable through the expansion of telehealth capabilities and the development of AI-powered diagnostic tools that could reach underserved populations. Quality improvements were expected to result from the enhanced accuracy and efficiency offered by AI in areas such as diagnostics, treatment planning, and drug discovery.
Affordability was to be addressed through increased efficiency and reduced administrative costs enabled by AI-driven automation. The administration frequently cited the potential for AI to accelerate drug development and personalized medicine, leading to cost savings in the long run.
Comparison with Approaches in Other Developed Nations
Compared to other developed nations, the Trump administration’s approach to AI regulation in healthcare was arguably less prescriptive and more focused on market-driven solutions. Countries like the European Union, for example, adopted a more cautious and regulatory approach, emphasizing data privacy and ethical considerations through regulations like GDPR. In contrast, the US administration prioritized fostering innovation and minimizing regulatory barriers, potentially at the expense of more stringent oversight and potential risks associated with rapid technological deployment.
This difference in approach reflects differing priorities and philosophies regarding the balance between innovation and regulation in the development and application of AI in healthcare.
AI Governance Frameworks During the Trump Era
The Trump administration’s approach to AI in healthcare, while not explicitly defined by a single, overarching policy document, was shaped by existing regulatory frameworks and a general emphasis on deregulation and technological advancement. Understanding the existing landscape and its limitations is crucial to analyzing the impact on AI development and deployment during this period.
Regulatory Frameworks Governing AI in Healthcare
Several agencies played significant roles in overseeing AI’s application in healthcare during the Trump years. These agencies, with their existing mandates, attempted to navigate the novel challenges presented by AI technologies. Existing regulations, primarily focused on areas like data privacy (HIPAA), medical device safety (FDA), and clinical trial oversight, were applied, albeit sometimes imperfectly, to the burgeoning field of AI-driven healthcare.
Remember those intense debates surrounding health AI governance and the Trump administration’s standards? Well, with the news that rfk jr confirmed hhs secretary robert f kennedy jr , it’ll be interesting to see how his perspective shapes the future of AI in healthcare. Will we see a shift in priorities regarding data privacy and algorithmic bias under his leadership?
This could significantly impact the implementation and regulation of AI within the health sector, potentially revisiting or revising existing frameworks.
The lack of specific AI-centric regulations meant adaptation and interpretation were key.
Gaps and Limitations in Existing Frameworks
A major gap was the absence of specific regulations tailored to AI. Existing frameworks, designed for traditional medical devices and data handling, struggled to encompass the unique characteristics of AI, such as machine learning algorithms’ evolving nature and the difficulty in establishing clear lines of accountability for AI-driven decisions. The rapid pace of AI development further exacerbated these challenges, leaving regulatory bodies playing catch-up.
This also led to uncertainty for developers and healthcare providers regarding compliance and potential liabilities. For example, determining the appropriate level of pre-market review for AI-powered diagnostic tools presented a significant hurdle.
Challenges of Applying Existing Regulations to Evolving AI Technologies
Applying established regulations to rapidly evolving AI technologies proved difficult. The FDA, for instance, faced the challenge of assessing the safety and efficacy of AI algorithms that could learn and adapt over time, unlike traditional, static medical devices. The dynamic nature of AI made it challenging to define clear standards and testing protocols. Further complicating matters was the potential for algorithmic bias, which existing regulations didn’t fully address.
Remember those debates about health AI governance and the Trump administration’s standards? It all feels a bit distant now, considering the current state of healthcare infrastructure. The news about Steward Health Care’s Ohio hospital closures and a Pennsylvania facility steward ohio hospitals closures pennsylvania facility at risk really highlights how fragile the system is, making the need for robust AI oversight – and perhaps even more funding for healthcare – even more critical.
We need strong standards to prevent further collapses, especially given the potential of AI to both improve and disrupt the system.
This meant the risk of perpetuating existing health disparities through biased AI algorithms was a significant concern.
Impact of the Regulatory Landscape on AI Development and Deployment
The regulatory uncertainty inherent in the existing framework influenced the development and deployment of AI-based healthcare solutions. Some companies chose to focus on areas with clearer regulatory pathways, potentially slowing down innovation in more complex applications. Others pursued strategies of regulatory engagement and collaboration with agencies like the FDA to navigate the ambiguous landscape. The lack of clear guidelines also created potential barriers to entry for smaller companies, potentially favoring larger organizations with greater resources to navigate the complexities of regulatory compliance.
For example, the lengthy and rigorous FDA approval process for AI-powered diagnostic tools slowed down their market entry compared to other digital health solutions.
Key Regulatory Bodies and Their Roles
Regulatory Body | Primary Role Related to AI in Healthcare | Relevant Regulations | Limitations/Challenges |
---|---|---|---|
Food and Drug Administration (FDA) | Oversight of AI-powered medical devices and software as medical devices. | 21 CFR Part 820 (Quality System Regulation), 21 CFR Part 806 (Postmarket Surveillance) | Adapting existing regulations for evolving AI algorithms; balancing innovation with safety. |
Health and Human Services (HHS) | Enforcement of HIPAA regarding patient data privacy and security in AI applications. | Health Insurance Portability and Accountability Act (HIPAA) | Ensuring compliance with HIPAA in the context of AI data usage and machine learning. |
Federal Trade Commission (FTC) | Addressing potential unfair or deceptive practices related to AI-based healthcare marketing and claims. | Federal Trade Commission Act | Regulation of AI-driven marketing and advertising claims; addressing potential bias in AI systems. |
Centers for Medicare & Medicaid Services (CMS) | Oversight of AI’s role in billing and reimbursement for healthcare services. | Medicare and Medicaid statutes and regulations | Determining appropriate reimbursement for AI-driven procedures and services. |
Data Privacy and Security Concerns

Source: abtosoftware.com
The integration of artificial intelligence (AI) into healthcare promises revolutionary advancements, but it also introduces significant data privacy and security challenges. The sheer volume of sensitive patient data required to train and operate these AI systems creates a fertile ground for breaches and misuse, demanding robust safeguards to protect patient confidentiality and trust. This section explores the complexities of data privacy and security in the context of AI-driven healthcare, focusing on relevant legislation, potential risks, and best practices for mitigation.The use of AI in healthcare necessitates the processing of vast quantities of personal health information (PHI), including medical records, genetic data, and wearable sensor readings.
This data is often highly sensitive and protected under various regulations, making its handling particularly crucial.
HIPAA and Other Relevant Legislation
The Health Insurance Portability and Accountability Act (HIPAA) is the cornerstone of US healthcare data privacy legislation. It establishes national standards for protecting sensitive patient health information. HIPAA’s privacy rule dictates how protected health information (PHI) can be used, disclosed, and protected. AI developers and healthcare providers must adhere strictly to these rules when collecting, storing, processing, and sharing patient data for AI applications.
Beyond HIPAA, other regulations, such as the California Consumer Privacy Act (CCPA) and the General Data Protection Regulation (GDPR) in Europe, also apply depending on the location of data storage and processing. Compliance with these varying regulations adds a layer of complexity to AI development and deployment in healthcare. Failure to comply can result in substantial fines and legal repercussions.
Risks Associated with Data Breaches and Unauthorized Access
Data breaches pose a significant threat to patient privacy and safety. Unauthorized access to sensitive health information can lead to identity theft, financial fraud, discrimination, and reputational damage. For instance, a breach exposing genetic information could lead to discrimination in insurance or employment. Similarly, unauthorized access to medical records could result in the inappropriate disclosure of sensitive conditions.
The sophisticated nature of AI systems also makes them potential targets for cyberattacks, potentially leading to the compromise of large amounts of patient data. The consequences of such breaches can be far-reaching, eroding public trust in AI-powered healthcare and impacting the adoption of these technologies.
Best Practices for Securing Patient Data in AI Applications
Protecting patient data in AI applications requires a multi-faceted approach encompassing technical, administrative, and physical safeguards. Strong encryption both at rest and in transit is paramount. Data anonymization and de-identification techniques can minimize the risk of re-identification, although these methods are not foolproof. Regular security audits and penetration testing are crucial to identify vulnerabilities and ensure the effectiveness of security measures.
Implementing robust access control mechanisms, including role-based access control, limits access to sensitive data only to authorized personnel. Furthermore, robust incident response plans are necessary to effectively manage and mitigate the impact of potential data breaches. Employee training on data privacy and security best practices is also essential to foster a culture of security within the organization.
Finally, adopting a privacy-by-design approach, integrating data privacy and security considerations from the initial stages of AI development, is crucial for building trust and ensuring compliance.
Ethical Considerations of AI in Healthcare
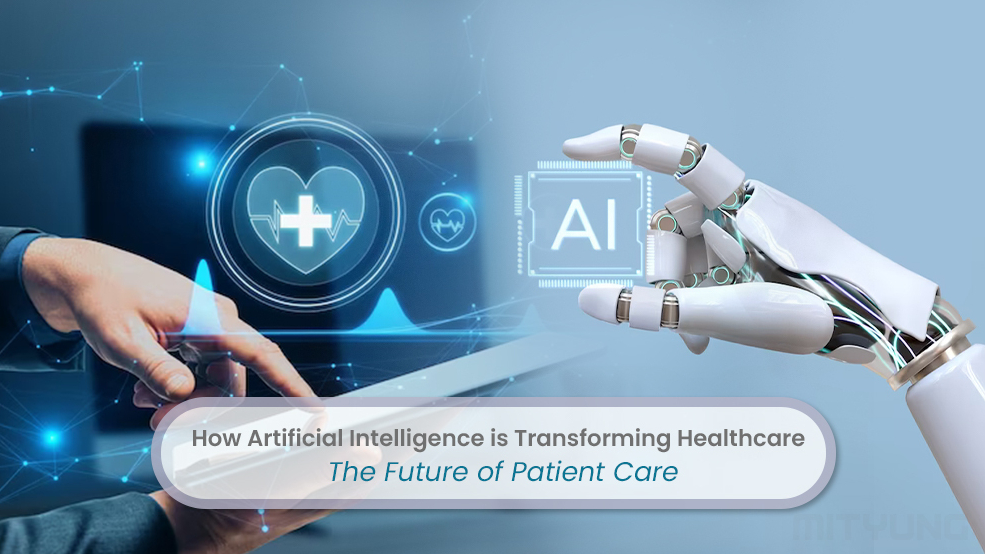
Source: mityung.com
The application of artificial intelligence (AI) in healthcare offers incredible potential for improved diagnostics, personalized treatments, and more efficient workflows. However, this transformative technology also presents a complex web of ethical dilemmas that demand careful consideration and proactive mitigation strategies. Failing to address these issues head-on risks undermining public trust and hindering the responsible integration of AI into the healthcare system.Algorithmic bias and fairness are central to the ethical debate surrounding AI in healthcare.
AI systems are trained on data, and if that data reflects existing societal biases – for example, underrepresentation of certain demographics in clinical trials – the AI system will perpetuate and potentially amplify those biases in its diagnoses and treatment recommendations. This can lead to disparities in care, where certain patient groups receive suboptimal or even discriminatory treatment.
Algorithmic Bias and Fairness in AI-Driven Healthcare Decisions
The inherent risk of bias in AI algorithms stems from the data used to train them. For instance, if an AI system for diagnosing heart disease is primarily trained on data from a predominantly male population, it may be less accurate in diagnosing the condition in women, potentially leading to delayed or missed diagnoses. Similarly, biases related to race, socioeconomic status, or geographic location can lead to unfair and inequitable outcomes.
Mitigating this requires careful curation of training datasets to ensure representation across diverse populations and the development of algorithms that are robust to bias and can identify and flag potential discriminatory outcomes. Ongoing monitoring and auditing of AI systems are crucial to detect and correct for biases that may emerge over time. For example, a system designed to predict readmission rates might inadvertently penalize patients from lower socioeconomic backgrounds due to factors outside of their health status, like access to transportation or healthy food.
Transparency and Accountability in AI Healthcare Systems
Transparency and accountability are paramount to building trust in AI-driven healthcare. Patients and clinicians need to understand how AI systems arrive at their decisions. A “black box” approach, where the decision-making process is opaque, is ethically unacceptable. Transparency involves providing clear explanations of the algorithms used, the data sources, and the limitations of the AI system. Accountability mechanisms are equally crucial.
Clear lines of responsibility must be established for the outcomes generated by AI systems, ensuring that individuals or organizations can be held accountable for errors or harms caused by these systems. This might involve establishing clear oversight committees, implementing robust auditing processes, and developing mechanisms for redress in cases of harm. For example, a transparent system would clearly articulate the weight given to different factors in a risk assessment, allowing for a review of the process if an unfavorable outcome occurs.
Ethical Considerations in AI Governance Frameworks
Ethical considerations must be central to the design and implementation of AI governance frameworks. These frameworks should prioritize patient safety, fairness, and equity. They should also address issues of data privacy, security, and informed consent. Furthermore, governance frameworks should promote collaboration between stakeholders, including clinicians, researchers, policymakers, and ethicists, to ensure a holistic and responsible approach to AI development and deployment.
These frameworks should not only define technical standards but also incorporate ethical guidelines and mechanisms for ongoing evaluation and adaptation in response to emerging challenges and evolving societal values. For example, a robust governance framework might mandate regular audits of AI systems for bias and require human oversight in critical decision-making processes.
Impact on Healthcare Standards
The integration of artificial intelligence (AI) into healthcare is poised to dramatically reshape existing standards and practices. This transformation presents both exciting opportunities and significant challenges, demanding careful consideration of its impact on quality, safety, and efficiency. The potential for AI to revolutionize medical diagnosis, treatment, and patient care is undeniable, but navigating the complexities of implementation requires a proactive and strategic approach.AI’s influence on healthcare standards will be multifaceted.
For instance, the development and validation of AI algorithms require rigorous testing and standardization to ensure accuracy and reliability. This necessitates the creation of new standards for data quality, algorithm transparency, and performance evaluation, potentially modifying existing regulatory frameworks. Furthermore, the ethical implications of AI-driven decision-making, including issues of bias and accountability, will necessitate the development of new ethical guidelines and protocols.
AI’s Effect on Quality, Safety, and Efficiency of Healthcare Delivery
AI has the potential to significantly enhance the quality, safety, and efficiency of healthcare delivery. Improved diagnostic accuracy through AI-powered image analysis, for example, can lead to earlier and more precise interventions, ultimately improving patient outcomes. AI-driven systems can also optimize resource allocation, reducing wait times and improving operational efficiency in hospitals and clinics. However, the integration of AI also raises safety concerns.
The reliance on AI algorithms for critical decisions requires robust validation and oversight to minimize the risk of errors and unintended consequences. Furthermore, the potential for biases in algorithms needs to be addressed to ensure equitable access to quality care. For instance, an AI system trained on data predominantly from one demographic group might produce inaccurate or biased results when applied to patients from different backgrounds.
Transformation of Medical Diagnosis, Treatment, and Patient Care
AI is transforming medical diagnosis, treatment, and patient care across various specialities. In radiology, AI algorithms are assisting radiologists in detecting subtle anomalies in medical images, leading to earlier diagnoses of diseases like cancer. In oncology, AI is being used to personalize cancer treatment plans based on individual patient characteristics and tumor profiles. In cardiology, AI-powered systems are analyzing electrocardiograms (ECGs) to detect arrhythmias and other cardiac abnormalities.
AI is also revolutionizing patient care through the development of virtual assistants and chatbots that provide patients with 24/7 access to medical information and support. However, the widespread adoption of these technologies necessitates careful consideration of the human-machine interface and the potential for deskilling of healthcare professionals. Maintaining a balance between AI augmentation and human expertise is crucial for optimal patient care.
Potential Benefits and Drawbacks of AI Integration into Healthcare Standards
The integration of AI into healthcare standards presents a complex interplay of potential benefits and drawbacks. Careful planning and a measured approach are essential to maximize the benefits while mitigating the risks.
Before the list, it’s important to understand that the success of AI integration hinges on addressing ethical, regulatory, and practical challenges.
- Benefits: Improved diagnostic accuracy, personalized treatment plans, enhanced efficiency, reduced costs, increased access to care, improved patient outcomes, better resource allocation, and development of new therapies and treatments.
- Drawbacks: Data privacy and security concerns, algorithmic bias and fairness issues, lack of transparency and explainability, potential for job displacement, high implementation costs, regulatory hurdles, and the need for extensive training and validation of AI systems.
International Comparisons
The Trump administration’s approach to AI governance in healthcare, characterized by a relatively hands-off regulatory stance compared to its predecessors, provides a stark contrast to the more proactive strategies adopted by other nations. Examining these differences reveals valuable insights into the challenges and opportunities presented by the global adoption of AI in healthcare. This comparison highlights the diverse regulatory landscapes and their implications for innovation and patient safety.The United States, under the Trump administration, largely relied on existing regulations and voluntary guidelines rather than implementing comprehensive, specific AI legislation for healthcare.
This contrasted sharply with the more prescriptive approaches seen in the European Union with the General Data Protection Regulation (GDPR) and the emerging AI Act, which establishes stringent requirements for data protection and algorithmic transparency. Similarly, countries like Canada and Singapore have developed national AI strategies with specific ethical guidelines and regulatory frameworks for the healthcare sector, focusing on responsible innovation and patient well-being.
Regulatory Approaches and Their Impact on Innovation
The differing regulatory approaches significantly influence the pace and direction of AI development in healthcare. The lighter regulatory touch in the US during the Trump era arguably fostered faster innovation, allowing companies greater freedom to experiment and deploy AI solutions. However, this also potentially led to a higher risk of biases in algorithms, data breaches, and a lack of standardization, potentially hindering broader adoption and trust.
In contrast, the stricter regulations in the EU, while potentially slowing down initial innovation, aim to build a more robust and ethical framework, prioritizing patient safety and data privacy. This ultimately might lead to greater long-term trust and wider acceptance of AI-driven healthcare solutions. The Canadian and Singaporean models, with their emphasis on ethical guidelines and collaboration, represent a middle ground, aiming to balance innovation with responsible development.
Navigating the complex landscape of health artificial intelligence governance, especially considering the legacy of Trump administration standards, requires careful consideration. One area often overlooked is the impact of technology on repetitive strain injuries; for example, many tech workers suffer from carpal tunnel syndrome. Thankfully, there are effective non-surgical treatments available, like those outlined in this helpful guide: ways to treat carpal tunnel syndrome without surgery.
Understanding these options is crucial as we build AI systems that prioritize both efficiency and worker well-being, a key aspect of responsible health AI governance.
Data Privacy and Security: A Global Perspective
Data privacy and security are paramount concerns in the deployment of AI in healthcare. The GDPR in the EU sets a high standard for data protection, requiring explicit consent and providing individuals with greater control over their data. This contrasts with the more fragmented and less stringent approach in the US, where data privacy laws vary across states and often lag behind the rapid advancements in AI technology.
This difference creates challenges for international collaboration and data sharing, particularly in clinical trials and research initiatives involving AI. Countries like Singapore have proactively addressed these issues through the establishment of national data protection frameworks specifically designed to accommodate the use of AI in healthcare, aiming for a balance between data utilization for innovation and the protection of individual rights.
Lessons Learned and Best Practices
International comparisons reveal several valuable lessons. A purely laissez-faire approach to AI regulation, while potentially stimulating initial innovation, may ultimately lead to a fragmented and less trustworthy system. Conversely, overly restrictive regulations can stifle innovation and hinder the potential benefits of AI in healthcare. Best practices appear to involve a balanced approach that incorporates: clear ethical guidelines focusing on fairness, transparency, and accountability; robust data protection frameworks that address the unique challenges posed by AI; mechanisms for monitoring and auditing AI systems; and collaborative initiatives involving stakeholders from industry, academia, and government.
The success of these strategies relies on adapting them to the specific contexts and priorities of each nation, while fostering international cooperation to establish common standards and principles for responsible AI development and deployment in healthcare.
Last Recap: Health Artificial Intelligence Governance Trump Admin Standards
The Trump administration’s approach to AI in healthcare, while marked by a focus on innovation, ultimately left a legacy of unanswered questions regarding comprehensive governance. While the potential benefits of AI in healthcare are undeniable, the need for robust ethical frameworks, stringent data protection measures, and international collaboration remains paramount. Understanding this period’s successes and failures is crucial for shaping a future where AI can truly improve healthcare while upholding the highest standards of patient safety, privacy, and ethical practice.
The ongoing conversation about AI governance, spurred by the challenges of this era, continues to inform and shape the responsible development and deployment of this transformative technology.
Commonly Asked Questions
What specific executive orders addressed AI in healthcare during the Trump administration?
While no specific executive orders solely focused on AI in healthcare, several orders related to technology, data, and regulatory reform indirectly impacted the field. Further research into the specifics of those orders is needed to provide a definitive answer.
How did the Trump administration’s approach to AI regulation compare to the Obama administration?
A direct comparison requires detailed analysis of both administrations’ policies. However, a key difference might lie in the emphasis on deregulation versus proactive regulation, potentially impacting the pace of AI adoption in healthcare.
What were some of the major criticisms of the Trump administration’s approach to AI in healthcare?
Criticisms likely centered on perceived gaps in regulatory oversight, potential insufficient attention to ethical concerns, and a possible lack of proactive measures to address algorithmic bias and data security vulnerabilities.