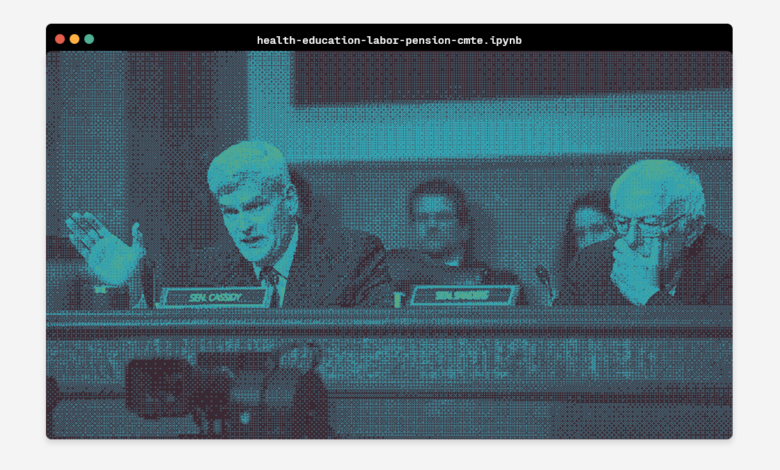
Healthcare AI Senate Finance Committee A Deep Dive
Healthcare AI Senate Finance Committee hearings are captivating the nation’s attention, sparking debate about the future of medicine and the role of artificial intelligence. This isn’t just about tech; it’s about access, costs, ethics, and the very fabric of our healthcare system. We’re diving deep into the discussions, examining the potential benefits and the very real concerns surrounding AI’s integration into patient care.
From cost-saving potential to the critical need for data privacy, the implications are far-reaching and demand careful consideration.
This blog post explores the historical context of the Senate Finance Committee’s involvement in healthcare technology, examining key legislative actions and the voices of various stakeholders – from tech giants to patient advocacy groups. We’ll unpack the complex issues surrounding AI’s impact on healthcare costs and access, especially for underserved communities. Further, we’ll delve into the ethical considerations, data security challenges, and the urgent need for robust regulatory frameworks that safeguard patient privacy while fostering innovation.
AI’s Impact on Healthcare Costs and Access
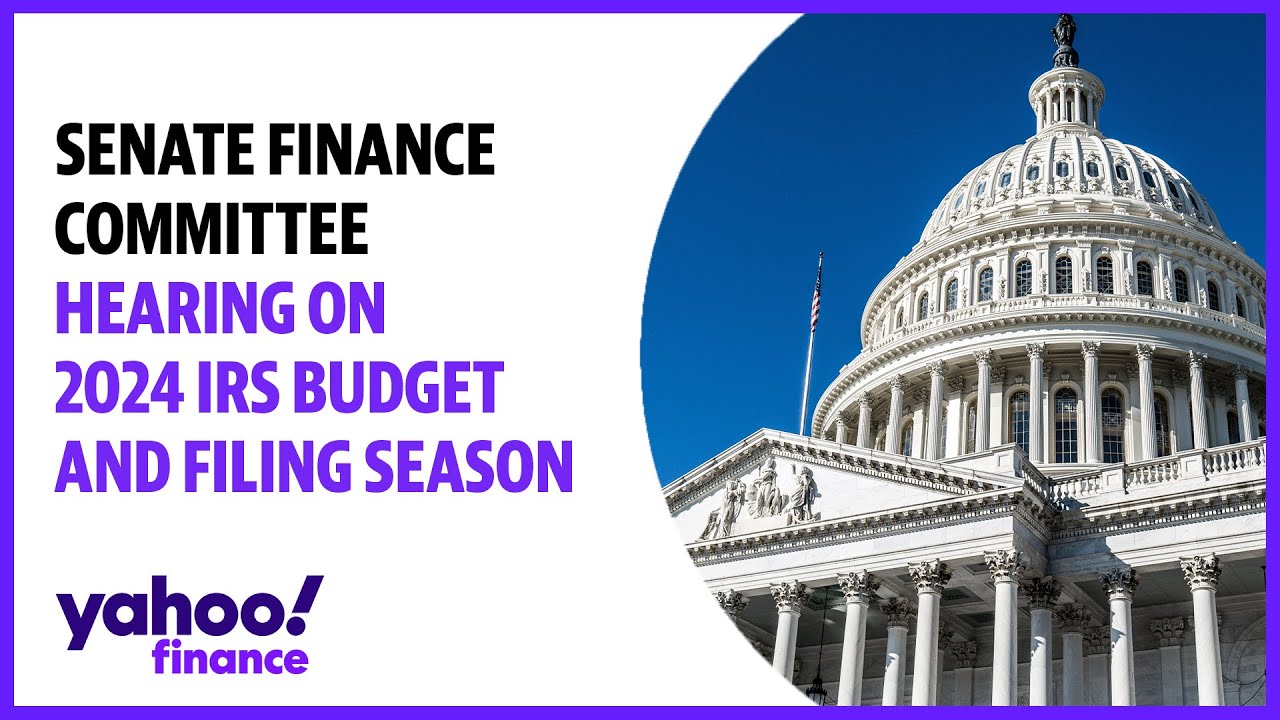
Source: ytimg.com
The integration of artificial intelligence (AI) into healthcare is rapidly transforming the industry, presenting both immense opportunities and significant challenges. While the promise of reduced costs and improved access is alluring, a nuanced understanding of the potential benefits and drawbacks is crucial for responsible implementation and policy development. This exploration will delve into the complex interplay between AI, healthcare expenditures, accessibility, and ethical considerations.
Potential Cost Savings and Increases from AI in Healthcare
AI’s impact on healthcare costs is multifaceted. On one hand, AI-powered diagnostic tools can potentially reduce the need for expensive and time-consuming tests, leading to significant cost savings. For instance, AI algorithms can analyze medical images with a high degree of accuracy, potentially reducing the reliance on radiologists for initial screenings. Furthermore, AI-driven predictive analytics can optimize hospital resource allocation, reducing unnecessary admissions and improving patient flow, thereby lowering operational costs.
Conversely, the initial investment in developing, implementing, and maintaining AI systems can be substantial. The cost of training data, specialized hardware, and skilled personnel required to operate and maintain these systems can represent a considerable upfront expense for healthcare providers. Moreover, the potential for increased liability due to AI-related errors needs to be factored into the overall cost equation.
The long-term cost-effectiveness of AI in healthcare will depend on careful planning, strategic implementation, and ongoing evaluation.
AI’s Role in Improving Healthcare Access, Especially in Underserved Communities
AI has the potential to significantly improve access to healthcare, particularly in underserved communities. Telemedicine platforms powered by AI can connect patients in remote areas with specialists, overcoming geographical barriers. AI-powered diagnostic tools can also assist healthcare providers in underserved areas with limited resources, enabling earlier and more accurate diagnoses. For example, AI algorithms can analyze retinal scans to detect diabetic retinopathy, a leading cause of blindness, even in settings lacking ophthalmologists.
This capability could be particularly beneficial in rural communities or developing countries with limited access to specialized eye care. Furthermore, AI-driven chatbots can provide basic medical information and triage patients, directing them to appropriate care pathways, thus reducing the burden on already strained healthcare systems in underserved areas.
The Healthcare AI Senate Finance Committee’s hearings are crucial, especially considering the rapid advancements in the field. The recent news about adventhealth ceo retire terry shaw highlights the importance of strong leadership in navigating these technological changes within large healthcare systems. This transition at AdventHealth underscores the need for robust AI regulations, a key focus for the committee’s ongoing work.
Ethical Concerns Regarding Equitable Distribution of AI-Driven Healthcare Benefits
The equitable distribution of AI-driven healthcare benefits is a critical ethical concern. The high cost of developing and implementing AI systems could exacerbate existing health disparities, potentially widening the gap between those who have access to advanced AI-powered care and those who do not. Bias in algorithms, trained on datasets that may not represent the diversity of the population, could lead to inaccurate or discriminatory outcomes, further disadvantaging certain groups.
Data privacy and security are also major concerns, particularly in underserved communities where individuals may be more vulnerable to exploitation or data breaches. Addressing these ethical challenges requires a multi-faceted approach, including rigorous algorithm testing for bias, ensuring data privacy and security, and promoting transparency and accountability in the development and deployment of AI healthcare systems.
Policy Proposal Addressing Disparities in Access to AI-Powered Healthcare, Healthcare ai senate finance committee
To mitigate disparities in access to AI-powered healthcare, a comprehensive policy framework is needed. This framework should include: 1) Investment in infrastructure development to ensure equitable access to high-speed internet and digital technologies in underserved communities. 2) Funding for the development and implementation of AI-powered healthcare solutions specifically designed to address the needs of underserved populations, including culturally appropriate interfaces and multilingual capabilities.
3) Strict regulations on data privacy and security, ensuring the protection of sensitive patient information, particularly in vulnerable communities. 4) Establishment of independent oversight bodies to monitor the ethical implications of AI in healthcare and ensure accountability for algorithmic bias and discriminatory outcomes. 5) Incentive programs for healthcare providers to adopt and implement AI technologies in underserved areas.
This multi-pronged approach would help ensure that the benefits of AI in healthcare are shared equitably across all communities.
The Senate Finance Committee’s hearings on AI in healthcare got me thinking about the future of access. News of HSHS Prevea closing Wisconsin hospitals and health centers, as reported in this article , highlights the urgent need for innovative solutions. Could AI help alleviate the strain on remaining facilities and ensure equitable care? The committee’s work is crucial in navigating these complex challenges.
AI’s Role in Improving Healthcare Quality and Outcomes
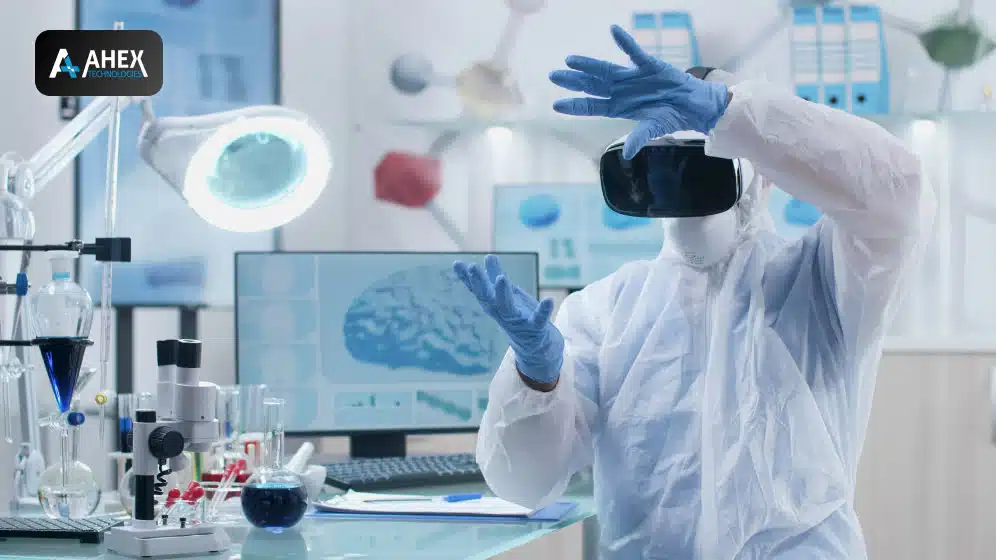
Source: ahex.co
Artificial intelligence is rapidly transforming healthcare, offering the potential to significantly improve the quality of care and patient outcomes. By automating tasks, analyzing vast datasets, and identifying patterns invisible to the human eye, AI is enhancing diagnostic accuracy, streamlining workflows, and personalizing treatment plans. This ultimately leads to better health outcomes and a more efficient healthcare system.
AI-Enhanced Medical Diagnosis
AI algorithms are proving invaluable in improving the accuracy and speed of medical diagnoses. For example, in radiology, AI-powered systems can analyze medical images like X-rays, CT scans, and MRIs, identifying potential anomalies with a high degree of accuracy. These systems can detect subtle signs of disease that might be missed by human radiologists, leading to earlier and more effective interventions.
In pathology, AI assists in analyzing microscopic images of tissue samples, aiding in the diagnosis of cancer and other diseases. This increased diagnostic accuracy translates directly to improved patient outcomes, allowing for earlier treatment and potentially better survival rates. One specific example is the use of AI in detecting diabetic retinopathy, a leading cause of blindness. AI-powered systems can analyze retinal images and identify signs of the disease with accuracy comparable to ophthalmologists, enabling timely intervention and preventing vision loss.
Demonstrably Improved Patient Outcomes through AI
Several studies have demonstrated the positive impact of AI on patient outcomes. For instance, AI-powered risk prediction models have been shown to improve the management of patients with chronic conditions like heart failure. By identifying patients at high risk of adverse events, clinicians can implement preventative measures and adjust treatment plans accordingly, leading to reduced hospitalizations and improved survival rates.
Furthermore, AI-driven robotic surgery systems are enhancing surgical precision and minimizing invasiveness, resulting in faster recovery times and fewer complications for patients. The use of AI in personalized medicine, tailoring treatment plans based on individual patient characteristics, also promises to improve outcomes by optimizing treatment efficacy and minimizing side effects. For example, AI can predict which patients are most likely to respond to a particular drug, allowing for more targeted therapies and reducing the use of ineffective treatments.
Potential Risks Associated with AI in Healthcare
While the benefits of AI in healthcare are substantial, it is crucial to acknowledge the potential risks. One major concern is algorithmic bias. If the data used to train AI algorithms is biased, the resulting algorithms may perpetuate and even amplify existing health disparities. For instance, an algorithm trained on data predominantly from one demographic group might perform poorly when applied to patients from other groups.
The Senate Finance Committee’s hearings on AI in healthcare got me thinking about the fragility of our system. News of Steward Health Care’s Ohio hospital closures and the Pennsylvania facility at risk, as reported in this article steward ohio hospitals closures pennsylvania facility at risk , highlights how crucial efficient resource allocation is. This underscores the need for the committee to consider AI’s potential to improve hospital management and prevent such devastating closures.
Another significant risk is data security and privacy. Healthcare data is highly sensitive, and its unauthorized access or misuse could have serious consequences for patients. Robust security measures are essential to protect patient data from breaches and ensure compliance with data privacy regulations. Finally, the lack of transparency and explainability in some AI algorithms can make it difficult to understand how they arrive at their conclusions, hindering trust and acceptance among healthcare professionals and patients.
AI in Personalized and Preventative Care
AI has the potential to revolutionize personalized and preventative care. By analyzing individual patient data, including genetic information, lifestyle factors, and medical history, AI can identify individuals at high risk of developing specific diseases. This allows for proactive interventions, such as lifestyle modifications or early screening, to prevent or delay the onset of disease. In personalized medicine, AI can help tailor treatment plans to individual patient needs, optimizing treatment efficacy and minimizing side effects.
For example, AI can predict which patients are most likely to respond to a specific cancer therapy, allowing oncologists to select the most effective treatment option for each patient. This approach to care promises to improve health outcomes and enhance the overall patient experience.
Data Privacy and Security Concerns in AI Healthcare
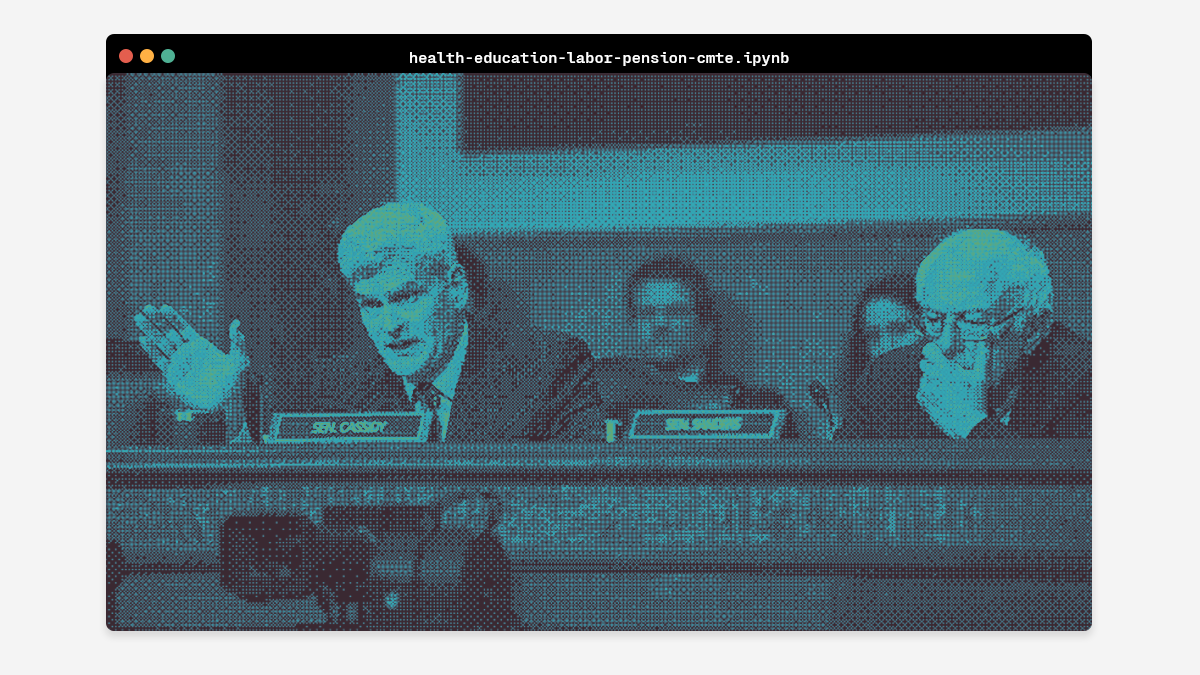
Source: fas.org
The integration of artificial intelligence (AI) into healthcare promises significant advancements, but it also raises serious concerns about patient data privacy and security. The sheer volume of sensitive health information processed by AI algorithms necessitates robust safeguards to prevent breaches and misuse. Balancing the potential benefits of AI with the need to protect individual privacy is a critical challenge for policymakers, healthcare providers, and technology developers.
AI systems in healthcare rely heavily on the collection and analysis of patient data, including protected health information (PHI) such as medical records, genetic information, and lifestyle details. This data is used to train algorithms, personalize treatments, and improve diagnostic accuracy. However, the very nature of this data – its sensitivity and potential for misuse – presents significant legal and ethical challenges.
The potential for discrimination based on biased algorithms, the risk of unauthorized access leading to identity theft or medical fraud, and the erosion of patient trust are all substantial concerns that must be addressed proactively.
Legal and Ethical Challenges Surrounding Patient Data Use in AI Systems
The collection and use of patient data in AI systems must adhere to stringent legal and ethical guidelines. Existing regulations, such as HIPAA in the United States, provide a framework for protecting PHI, but their applicability to AI systems is still evolving. Ethical considerations involve informed consent, data minimization, and ensuring fairness and transparency in algorithmic decision-making. The potential for algorithmic bias, where AI systems perpetuate or amplify existing societal inequalities, is a significant ethical concern requiring careful attention to data quality and algorithm design.
Legal challenges arise from issues of data ownership, liability in case of data breaches, and the interpretation of existing regulations in the context of novel AI technologies. For example, the use of AI for predictive analytics might raise questions about the legal implications of identifying individuals at risk of specific health conditions before they manifest symptoms.
Current Federal Regulations and Proposed Legislation Related to Data Privacy in AI Healthcare
The US government is actively addressing data privacy in AI healthcare through various initiatives. HIPAA, while not explicitly designed for AI, sets the foundation for protecting PHI. However, the increasing complexity of AI applications necessitates more specific regulations. The Health Information Technology for Economic and Clinical Health (HITECH) Act, an amendment to HIPAA, strengthens enforcement and expands privacy protections.
Proposed legislation, such as the American Data Privacy and Protection Act (ADPPA), aims to create a comprehensive federal framework for data privacy that could impact the use of AI in healthcare. These regulatory efforts seek to balance the benefits of AI with the need to protect individual rights and prevent misuse of sensitive data. The ongoing debate focuses on the level of regulation needed, the balance between innovation and protection, and the enforcement mechanisms required to ensure compliance.
Technical Security Measures to Protect Patient Data Used in AI Algorithms
Protecting patient data used in AI algorithms requires a multi-layered approach to security. This includes robust encryption techniques to safeguard data both in transit and at rest, access control mechanisms to limit access to authorized personnel only, and regular security audits to identify and address vulnerabilities. Data anonymization and de-identification techniques can reduce the risk of re-identification, but these methods are not foolproof and their effectiveness depends on the specific techniques used.
Furthermore, employing intrusion detection and prevention systems, implementing strong authentication protocols, and maintaining regular software updates are crucial for mitigating cyber threats. Regular penetration testing and vulnerability assessments can help identify and address potential weaknesses in the system before they can be exploited. The use of blockchain technology is also being explored as a potential method to enhance data security and transparency.
Strategies to Mitigate Risks Associated with Data Breaches in AI-Driven Healthcare Systems
Data breaches can have devastating consequences, leading to financial losses, reputational damage, and erosion of patient trust. Proactive strategies are essential to minimize these risks.
- Implement comprehensive incident response plans to quickly contain and remediate breaches.
- Invest in employee training programs to raise awareness of security threats and best practices.
- Establish robust data governance frameworks to ensure responsible data handling and compliance with regulations.
- Regularly assess and update security protocols to address emerging threats and vulnerabilities.
- Collaborate with cybersecurity experts to leverage their knowledge and experience in securing AI systems.
- Conduct regular security audits and penetration testing to identify and mitigate vulnerabilities.
- Establish clear data retention policies and procedures for secure data disposal.
- Implement strong access control measures, including multi-factor authentication and role-based access control.
The Future of AI Regulation in Healthcare: Healthcare Ai Senate Finance Committee
The rapid advancement of artificial intelligence (AI) in healthcare presents both immense opportunities and significant challenges. While AI promises to revolutionize diagnostics, treatment, and patient care, its deployment requires careful consideration of ethical, safety, and privacy concerns. The absence of a comprehensive and harmonized regulatory framework could stifle innovation or, worse, lead to the widespread adoption of unreliable or harmful AI systems.
Therefore, establishing a robust and adaptable regulatory landscape is paramount.The need for new regulatory frameworks stems from the unique characteristics of AI in healthcare. Unlike traditional medical devices, AI algorithms are constantly learning and evolving, making traditional regulatory approaches insufficient. Furthermore, the complexity of AI systems, coupled with the sensitive nature of patient data, necessitates a multi-faceted regulatory approach that balances the need for innovation with the imperative to protect patients.
International Approaches to AI Regulation
Different countries are exploring diverse approaches to AI regulation, each reflecting their unique priorities and legal traditions. The European Union, for example, is taking a comprehensive approach with its proposed AI Act, classifying AI systems based on risk levels and imposing stricter requirements on high-risk applications, such as those used in medical diagnosis. This contrasts with the more flexible and less prescriptive approach adopted by the United States, which favors a sector-specific regulatory approach, focusing on addressing AI risks within existing regulatory frameworks for medical devices and data privacy.
Canada is also pursuing a risk-based approach, prioritizing the safety and efficacy of AI systems without overly burdening innovation. These varying approaches highlight the complexity of establishing a universally applicable regulatory framework.
Impact of Regulatory Models on AI Adoption
The chosen regulatory model significantly impacts the speed and scale of AI adoption in healthcare. Overly stringent regulations, while protecting patient safety, could stifle innovation and slow the development of potentially life-saving AI technologies. Conversely, a lack of regulation could lead to the proliferation of unreliable or biased AI systems, potentially harming patients and eroding public trust. A balanced approach is crucial, fostering innovation while ensuring accountability and transparency.
For instance, a heavily prescriptive regulatory environment, like a very strict interpretation of the EU AI Act, might delay the market entry of innovative AI-powered diagnostic tools, whereas a lighter regulatory touch, like the US’s approach, could lead to faster adoption but might also increase the risk of less-vetted AI systems entering the market.
A Hypothetical Regulatory Framework for AI in Healthcare
A hypothetical regulatory framework should incorporate several key elements. Firstly, it should adopt a risk-based approach, classifying AI systems based on their intended use and potential impact on patient safety. High-risk applications, such as AI-powered diagnostic tools, would require rigorous pre-market approval and ongoing monitoring. Lower-risk applications could be subject to less stringent requirements. Secondly, the framework should establish clear guidelines for data privacy and security, ensuring compliance with existing regulations like HIPAA (in the US) or GDPR (in the EU).
Thirdly, it should promote transparency and explainability, requiring developers to provide clear documentation of their AI systems and their decision-making processes. Finally, it should establish a robust mechanism for reporting and investigating adverse events, allowing for rapid identification and remediation of safety issues. This framework could incorporate elements from successful models globally, adapting to the specific context of the healthcare industry and national legal frameworks, aiming for a balance between encouraging innovation and protecting patient welfare.
For example, it could require independent audits of AI systems used for high-stakes decision-making, much like the rigorous testing required for new pharmaceuticals. This would provide a level of assurance to patients and regulators alike.
Epilogue
The Senate Finance Committee’s focus on AI in healthcare is a crucial step toward navigating the complex landscape of this rapidly evolving technology. The discussions highlight the immense potential of AI to revolutionize healthcare, offering improved diagnoses, personalized medicine, and increased access to care. However, the ethical considerations, data privacy concerns, and the need for equitable distribution of AI-driven benefits are equally critical.
Moving forward, a balanced approach – one that fosters innovation while prioritizing patient safety and data protection – is paramount to ensuring a future where AI truly benefits all.
User Queries
What specific AI technologies are being discussed by the committee?
The hearings likely cover a range of AI applications, including diagnostic tools, predictive analytics for disease management, robotic surgery assistance, and AI-powered drug discovery.
How does the committee balance innovation with patient safety?
This is a central theme. The committee likely weighs the potential benefits of AI against risks like algorithmic bias, data breaches, and the need for human oversight in medical decision-making.
What international precedents are being considered in the AI regulation discussions?
The committee likely examines regulatory approaches from countries like the EU (GDPR), Canada, and others to inform the development of a US framework.
What role do insurance companies play in these discussions?
Insurance companies are key stakeholders, as the cost and effectiveness of AI-driven healthcare directly impact insurance coverage and premiums.