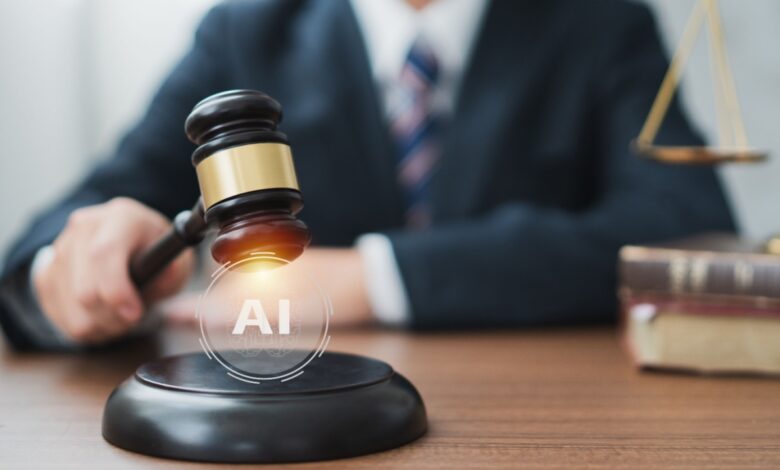
Healthcare AI Governance Oversight A Crucial Look
Healthcare artificial intelligence governance oversight is more than just a buzzword; it’s the critical framework shaping the future of medicine. As AI increasingly integrates into healthcare, from diagnostics to treatment planning, the need for robust governance becomes paramount. This isn’t just about regulations; it’s about ensuring fairness, transparency, and ultimately, patient safety in a world where algorithms are making life-altering decisions.
This exploration delves into the multifaceted challenges and opportunities presented by AI in healthcare. We’ll examine the ethical considerations, legal implications, and technical complexities involved in developing, deploying, and monitoring AI systems. From data privacy and algorithmic bias to accountability and human oversight, we’ll unpack the key elements needed to build a responsible and beneficial AI-powered healthcare ecosystem.
Defining the Scope of Healthcare AI Governance
The rapid integration of artificial intelligence (AI) into healthcare presents both immense opportunities and significant challenges. Effective governance is crucial to ensure responsible innovation, patient safety, and public trust. A robust framework must navigate the complex interplay between technological advancement, ethical considerations, and existing regulatory landscapes.
Healthcare AI governance is a crucial area, needing robust oversight to ensure ethical and responsible development. The sheer cost of new treatments, like the explosion in Medicare GLP-1 spending highlighted in this insightful KFF report, medicare glp1 spending weight loss kff , underscores the need for AI to help optimize resource allocation. Ultimately, effective AI governance can help us navigate these complex financial and ethical challenges in healthcare.
Key Components of a Comprehensive AI Governance Framework in Healthcare
A comprehensive governance framework for AI in healthcare needs several key components working in concert. These include clear definitions of AI systems used, establishing robust data governance policies, ensuring algorithmic transparency and explainability, implementing rigorous testing and validation protocols, and establishing mechanisms for continuous monitoring and improvement. Furthermore, a well-defined framework necessitates accountability and liability structures to address potential harms and ensure responsible use.
Finally, effective stakeholder engagement, including patients, clinicians, researchers, and policymakers, is essential for building trust and fostering collaboration.
Regulatory Oversight versus Ethical Considerations in AI Healthcare
Regulatory oversight and ethical considerations, while intertwined, represent distinct but equally crucial aspects of AI governance in healthcare. Regulatory oversight focuses on compliance with existing laws and regulations, such as data privacy (HIPAA in the US, GDPR in Europe), device safety (FDA regulations for medical devices), and clinical trial standards. Ethical considerations, on the other hand, address broader societal values and principles, such as fairness, transparency, accountability, and patient autonomy.
For instance, an AI diagnostic tool might comply with all relevant regulations but still raise ethical concerns if it disproportionately misdiagnoses patients from certain demographic groups. A strong governance framework must address both regulatory compliance and ethical considerations to ensure responsible AI development and deployment.
Examples of Existing Governance Frameworks for AI in Different Healthcare Sectors
Several sectors within healthcare are grappling with AI governance. The pharmaceutical industry, for example, is developing guidelines for the use of AI in drug discovery and development, focusing on data integrity, model validation, and bias mitigation. Similarly, the diagnostic imaging sector is seeing the emergence of frameworks that address the accuracy, reliability, and clinical validation of AI-powered diagnostic tools.
The FDA in the US plays a significant role in regulating AI-based medical devices, requiring premarket approval for high-risk devices and post-market surveillance for all AI-based medical devices. These existing frameworks highlight the diverse approaches being adopted across different healthcare sectors, each tailored to the specific risks and opportunities presented by AI.
Hypothetical Governance Structure for a New AI-Driven Medical Device
Consider a hypothetical new AI-driven medical device, a smart insulin pump that uses AI to personalize insulin delivery based on real-time glucose readings and other patient data. A robust governance structure for this device would include: (1) a dedicated ethics committee to review the algorithm’s design and potential biases; (2) a rigorous testing and validation process involving clinical trials and simulations to ensure safety and efficacy; (3) a transparent data governance policy outlining data collection, storage, and usage; (4) a post-market surveillance system to monitor device performance and identify potential safety issues; (5) a clear mechanism for reporting adverse events and handling complaints; and (6) a process for obtaining regulatory approvals from relevant bodies such as the FDA.
This multi-faceted approach aims to balance innovation with patient safety and ethical considerations.
Data Privacy and Security in AI Healthcare
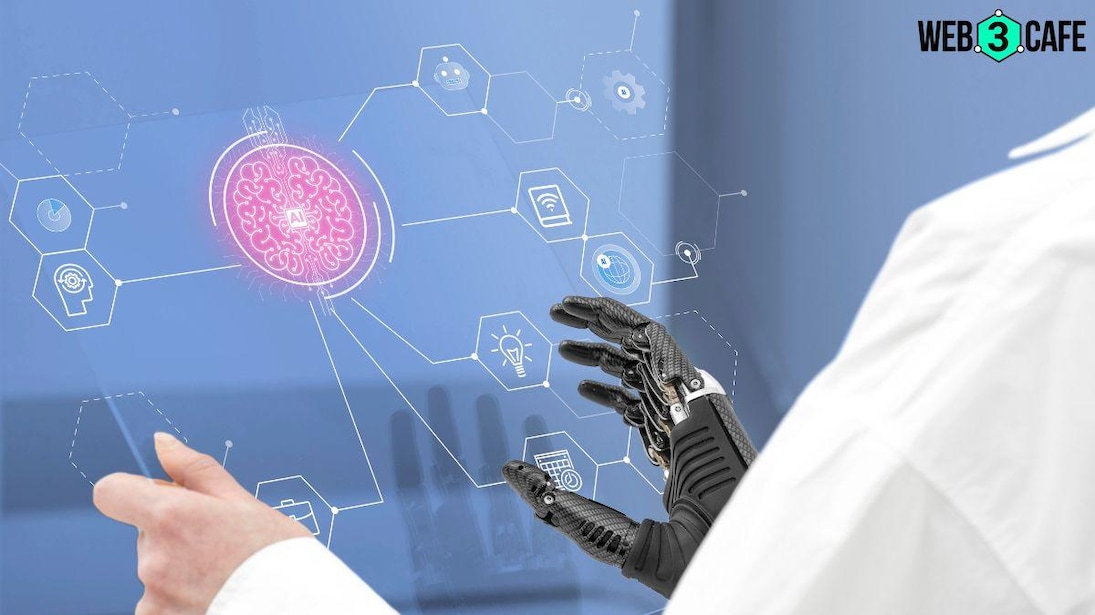
Source: tosshub.com
The integration of artificial intelligence (AI) into healthcare promises significant advancements in diagnosis, treatment, and patient care. However, this progress comes with substantial challenges, particularly concerning the privacy and security of sensitive patient data. AI systems often require vast amounts of data to function effectively, raising concerns about the potential for misuse, unauthorized access, and breaches of confidentiality. Robust governance frameworks are crucial to mitigate these risks and ensure ethical and responsible AI implementation in healthcare.
Challenges of Ensuring Patient Data Privacy within AI Systems
AI algorithms, by their nature, process and analyze large datasets. This inherently increases the risk of data breaches and unauthorized access compared to traditional, less data-intensive systems. The complexity of AI systems themselves, often involving multiple interconnected components and third-party vendors, adds another layer of difficulty in ensuring comprehensive data protection. Furthermore, the use of cloud-based storage and processing for AI applications introduces additional vulnerabilities, especially if appropriate security measures aren’t implemented.
Finally, the potential for re-identification of anonymized data, even with sophisticated anonymization techniques, remains a significant concern. Robust access control mechanisms and rigorous data governance policies are critical to mitigating these risks.
Comparison of Data Anonymization Techniques Used in AI Healthcare
Several techniques exist to anonymize patient data before it’s used in AI systems. These techniques aim to remove or obscure identifying information while preserving the data’s utility for AI model training and analysis. Data masking replaces sensitive data elements with surrogate values, while data generalization replaces specific values with broader categories (e.g., replacing an exact age with an age range).
Pseudonymization replaces identifiers with pseudonyms, allowing data linkage while protecting the original identity. Differential privacy adds carefully calibrated noise to the data, making it difficult to infer individual-level information while preserving statistical properties. Each technique has its strengths and weaknesses; the choice depends on the specific data, the AI application, and the desired level of privacy protection.
For example, differential privacy offers strong privacy guarantees but may reduce the accuracy of the AI model.
Legal Implications of Data Breaches Involving AI-Powered Healthcare Systems
Data breaches involving AI-powered healthcare systems carry significant legal ramifications. Depending on the jurisdiction, organizations may face hefty fines, lawsuits from affected patients, and reputational damage. Regulations like HIPAA in the United States and GDPR in Europe impose strict requirements for data protection and breach notification. Failure to comply with these regulations can result in severe penalties.
Furthermore, the use of AI in healthcare may introduce novel legal challenges, such as determining liability in cases where AI systems make incorrect diagnoses or recommendations leading to harm. Proactive measures to prevent breaches and robust incident response plans are crucial to mitigate legal risks.
Best Practices for Securing Patient Data in AI-Driven Healthcare Applications
Implementing robust security measures is paramount to protecting patient data in AI-driven healthcare. The following checklist Artikels key best practices:
Security Measure | Description | Implementation Steps | Risk Mitigation |
---|---|---|---|
Data Encryption | Encrypting data both at rest and in transit to protect it from unauthorized access. | Implement strong encryption algorithms (e.g., AES-256) and key management systems. | Reduces the risk of data exposure in case of a breach. |
Access Control | Restricting access to patient data based on the principle of least privilege. | Implement role-based access control (RBAC) and multi-factor authentication (MFA). | Limits the potential damage from insider threats and unauthorized access. |
Regular Security Audits | Conducting regular security assessments to identify and address vulnerabilities. | Perform penetration testing, vulnerability scanning, and security audits. | Proactively identifies and mitigates security risks. |
Data Loss Prevention (DLP) | Implementing measures to prevent sensitive data from leaving the organization’s control. | Use DLP tools to monitor data movement and prevent unauthorized transfers. | Reduces the risk of data exfiltration. |
Incident Response Plan | Developing a comprehensive plan to handle data breaches and other security incidents. | Define roles, responsibilities, and procedures for incident response. | Minimizes the impact of security incidents and ensures swift remediation. |
Employee Training | Educating employees about data security best practices and threats. | Provide regular security awareness training to employees. | Reduces the risk of human error and phishing attacks. |
Regular Software Updates | Keeping all software and systems up-to-date with the latest security patches. | Implement automated patching and update processes. | Reduces the risk of exploitation of known vulnerabilities. |
Privacy by Design | Integrating privacy considerations into the design and development of AI systems. | Conduct privacy impact assessments (PIAs) throughout the development lifecycle. | Ensures that privacy is built into the system from the start. |
Algorithmic Bias and Fairness in AI Healthcare
AI is rapidly transforming healthcare, offering the potential for improved diagnostics, personalized treatments, and more efficient resource allocation. However, the increasing reliance on algorithms in healthcare decision-making raises significant concerns about algorithmic bias and its potential to exacerbate existing health disparities. Failing to address these issues could lead to unfair and potentially harmful outcomes for certain patient populations.
Sources of Algorithmic Bias in Healthcare AI
Algorithmic bias in healthcare AI stems from various sources, often intertwined and difficult to isolate. These biases can be introduced at different stages of the AI lifecycle, from data collection and preprocessing to algorithm design and deployment. One key source is biased training data. If the data used to train an AI model disproportionately represents certain demographics or health conditions, the resulting algorithm will likely reflect and amplify these biases in its predictions.
Strong healthcare AI governance oversight is crucial, ensuring ethical use and preventing monopolies. This is especially relevant given recent FTC actions, like the lawsuit against the Block, Novant Health, and Community Health Systems hospital acquisition, as seen in this article: federal trade commission sues block novant health community health systems hospital acquisition. The FTC’s concern over reduced competition highlights the need for proactive AI governance to avoid similar situations arising from concentrated AI healthcare power.
For example, an algorithm trained primarily on data from a predominantly white population may perform poorly when applied to patients of other ethnicities. Another source is flawed algorithm design. Even with unbiased data, poorly designed algorithms can inadvertently create biases through the selection of features or the application of specific statistical methods. Finally, biases can also arise from the way AI systems are interpreted and used by healthcare professionals.
Subconscious biases can influence how clinicians interpret and act upon AI-generated recommendations.
Methods for Detecting and Mitigating Algorithmic Bias
Detecting and mitigating algorithmic bias requires a multi-faceted approach. Rigorous data auditing is crucial, involving careful examination of the training data for imbalances across different demographic groups and health outcomes. This includes assessing the representation of various demographic groups within the dataset and analyzing the prevalence of certain conditions across these groups. Furthermore, techniques like fairness-aware machine learning can be employed during algorithm development.
These techniques aim to explicitly incorporate fairness constraints into the training process, ensuring that the resulting model does not discriminate against certain groups. Post-processing techniques can also be used to adjust the predictions of an already trained model to mitigate bias. Finally, ongoing monitoring and evaluation of the AI system’s performance across different demographic groups are essential to identify and address any emerging biases.
Regular audits and independent reviews can help ensure fairness and transparency.
Ethical Implications of Perpetuating Health Disparities
The use of biased AI systems in healthcare has significant ethical implications, particularly concerning the perpetuation and amplification of existing health disparities. If an AI system consistently provides inaccurate or less effective care to certain demographic groups, it can lead to worsening health outcomes and exacerbate existing inequalities. This raises serious ethical questions about fairness, justice, and equitable access to healthcare.
The potential for biased algorithms to negatively impact vulnerable populations underscores the critical need for careful development, deployment, and ongoing monitoring of AI systems in healthcare. Transparency and accountability are paramount to ensure that AI is used responsibly and ethically.
Case Study: Algorithmic Bias in Cardiovascular Risk Prediction
- Scenario: An AI algorithm is developed to predict the risk of cardiovascular disease based on patient data including age, gender, race, and medical history.
- Bias in Training Data: The training data predominantly includes patients from affluent, predominantly white communities, underrepresenting patients from lower socioeconomic backgrounds and minority ethnic groups who may experience different risk factors and access to care.
- Algorithmic Bias: The algorithm, trained on this biased data, learns to associate certain risk factors more strongly with cardiovascular disease in the overrepresented groups, leading to underestimation of risk in underrepresented groups.
- Impact on Patient Care: As a result, patients from underrepresented groups may be less likely to receive timely and appropriate preventative care or treatment, leading to poorer health outcomes and widening existing health disparities.
- Mitigation Strategies: To mitigate this bias, the developers could augment the training data with more representative samples from diverse populations, use fairness-aware machine learning techniques, and conduct regular audits to monitor the algorithm’s performance across different demographic groups.
Transparency and Explainability of AI in Healthcare
The increasing use of artificial intelligence (AI) in healthcare presents a crucial need for transparency and explainability. While AI algorithms can offer powerful diagnostic and treatment capabilities, their complex nature often makes it difficult to understand how they arrive at their conclusions. This lack of transparency can erode trust, hinder adoption, and even lead to serious medical errors. Ensuring that AI systems are both transparent and explainable is vital for responsible and effective deployment in the healthcare sector.
Transparency and explainability are not merely desirable features; they are essential for building trust with patients, clinicians, and regulators. Without understanding the reasoning behind an AI’s decision, healthcare professionals may hesitate to rely on its recommendations, potentially leading to suboptimal patient care. Furthermore, regulatory bodies require sufficient insight into AI systems to ensure their safety and efficacy, and to establish accountability in case of errors.
Openness regarding the data used, the algorithms employed, and the decision-making process fosters a culture of responsible innovation and accountability.
Techniques for Enhancing AI Interpretability
Several techniques aim to improve the interpretability of AI models used in healthcare. These methods strive to bridge the gap between the complex inner workings of an AI and human understanding. One approach involves using simpler, more easily interpretable models, such as linear regression or decision trees, instead of complex deep learning architectures. However, this often comes at the cost of predictive accuracy.
Alternatively, post-hoc explainability methods can be applied to existing complex models. These techniques analyze the model’s behavior after it’s been trained to provide insights into its decision-making process. Examples include LIME (Local Interpretable Model-agnostic Explanations) and SHAP (SHapley Additive exPlanations), which identify the features most influential in a specific prediction.
Challenges in Balancing Transparency and Intellectual Property Protection, Healthcare artificial intelligence governance oversight
Balancing transparency with the need to protect intellectual property (IP) is a significant challenge. Companies investing heavily in developing AI algorithms are naturally reluctant to fully disclose their trade secrets, fearing competitive disadvantage. However, complete secrecy is incompatible with the need for scrutiny and accountability in healthcare. Finding a suitable compromise requires careful consideration. This could involve selectively disclosing information, focusing on the overall functionality and limitations of the system while protecting the core algorithms.
Alternatively, independent audits by trusted third parties could provide assurance of safety and efficacy without compromising sensitive IP details. Regulations and standards need to carefully balance these competing interests.
Consequences of a “Black Box” AI System in Healthcare
Imagine a “black box” AI system used for diagnosing heart conditions. This system, lacking transparency, predicts a patient’s risk of a heart attack based on an opaque algorithm. The AI flags a low-risk patient as high-risk, leading to unnecessary, invasive, and potentially harmful procedures. The patient experiences complications, and the treating physician is left without understanding why the AI made this incorrect prediction.
In contrast, a transparent system might reveal that the AI overweighted a specific, unreliable input parameter in its assessment. This understanding would allow clinicians to critically evaluate the AI’s output and potentially prevent the negative outcome. This scenario highlights the crucial role of transparency in ensuring patient safety and clinician confidence in AI-driven healthcare.
Accountability and Liability in AI Healthcare: Healthcare Artificial Intelligence Governance Oversight
The increasing integration of artificial intelligence (AI) into healthcare presents a complex challenge: determining accountability when AI systems malfunction and cause harm. Traditional legal frameworks struggle to adapt to the unique characteristics of AI, blurring lines of responsibility and raising concerns about patient safety and trust. Understanding and addressing the issue of accountability is crucial for fostering responsible AI development and deployment in healthcare.
Effective healthcare artificial intelligence governance oversight is crucial, especially as AI’s role expands. To understand the scale of this challenge, consider the incredible advancements showcased at the ai most exciting healthcare technology center connected medicine upmc , highlighting the need for robust regulatory frameworks to ensure responsible AI implementation in healthcare. Without proper oversight, the potential benefits of AI could be overshadowed by ethical and safety concerns.
Challenges in Assigning Accountability for AI Errors
Assigning accountability for errors or harms caused by AI systems in healthcare is fraught with difficulties. Unlike human clinicians, AI lacks agency and intent. Determining whether a mistake stems from flawed algorithms, inadequate training data, improper implementation, or human oversight failure requires careful investigation. This process is often complex and time-consuming, involving multiple stakeholders – developers, healthcare providers, hospitals, and regulatory bodies.
Consider, for instance, a diagnostic AI that misinterprets a medical image, leading to a delayed or incorrect diagnosis. Is the developer responsible for algorithmic flaws? The hospital for insufficient validation procedures? The clinician for not critically reviewing the AI’s output? Establishing clear lines of responsibility in such scenarios is a major hurdle.
Legal Approaches to Establishing Liability for AI-Related Medical Malpractice
Several legal approaches are being explored to address liability for AI-related medical malpractice. One approach focuses on product liability, holding AI developers responsible for defects in their algorithms or software. Another approach centers on negligence, requiring healthcare providers to demonstrate reasonable care in selecting, implementing, and monitoring AI systems. A third approach considers a hybrid model, combining elements of product liability and negligence, recognizing the shared responsibility between developers and healthcare providers.
The legal precedents are still developing, and the outcome of lawsuits involving AI-related medical errors will significantly shape future legal interpretations. For example, a case might involve arguing that the AI developer failed to adequately test the algorithm for edge cases, leading to a predictable failure that resulted in patient harm, while simultaneously arguing that the hospital failed to properly train staff on the limitations of the AI system.
The Role of Insurance in Mitigating AI Risks
Insurance plays a vital role in mitigating the risks associated with AI in healthcare. Professional liability insurance for healthcare providers needs to adapt to cover potential damages arising from AI-related incidents. Product liability insurance for AI developers protects them from lawsuits related to defective algorithms or software. Cybersecurity insurance becomes increasingly important given the potential for data breaches and cyberattacks targeting AI systems.
The insurance industry is still developing specific policies for AI, and premiums are likely to reflect the perceived risks associated with different AI applications. For example, insurers may offer tailored policies for hospitals using AI for diagnostic purposes, accounting for the higher potential for harm compared to using AI for administrative tasks.
Framework for Establishing Accountability in AI-Driven Healthcare Organizations
Establishing a clear framework for accountability within AI-driven healthcare organizations requires a multi-faceted approach. This includes developing comprehensive risk assessment procedures for AI systems, establishing clear protocols for human oversight and intervention, implementing robust data governance policies to ensure data quality and security, and creating transparent mechanisms for reporting and investigating AI-related incidents. Furthermore, organizations should invest in ongoing training for healthcare professionals on the appropriate use and limitations of AI systems.
A crucial element of this framework is the establishment of an independent review board to investigate AI-related incidents and make recommendations for improvements. This board should have expertise in AI, healthcare, and law, ensuring a balanced and informed assessment of each case. Such a framework aims to promote a culture of safety and responsibility, fostering trust in AI-driven healthcare while minimizing potential risks.
The Role of Human Oversight in AI Healthcare
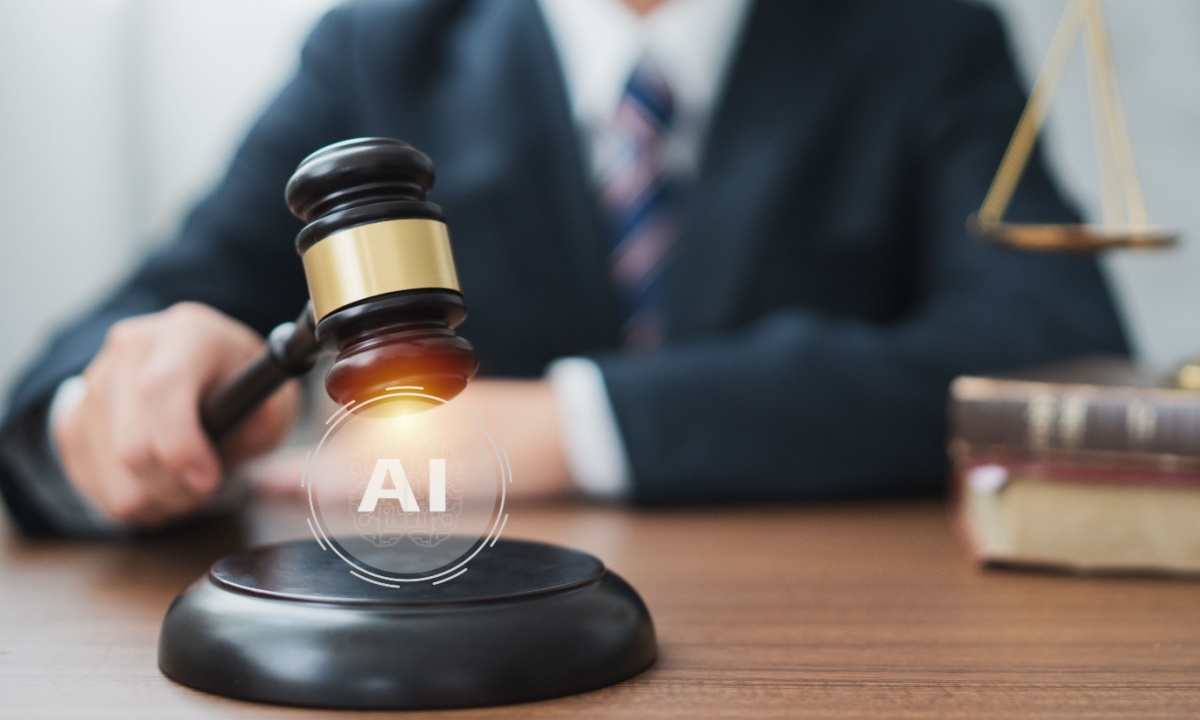
Source: pymnts.com
The integration of artificial intelligence (AI) into healthcare offers transformative potential, but its success hinges critically on robust human oversight. AI algorithms, however sophisticated, are tools; their effectiveness and ethical application depend entirely on human expertise guiding their development, deployment, and ongoing evaluation. Without this crucial human element, the risks associated with AI in healthcare – from biased diagnoses to compromised patient safety – become significantly amplified.
Human experts play multifaceted roles throughout the AI lifecycle, ensuring alignment with clinical best practices, ethical considerations, and patient well-being. This involves not only technical proficiency but also a deep understanding of the limitations of AI and the nuanced context of healthcare delivery. A collaborative approach, where AI augments human capabilities rather than replacing them, is paramount for responsible AI implementation.
Essential Roles of Human Experts in AI Healthcare
Human experts are indispensable at every stage of AI development and implementation in healthcare. During development, clinicians and data scientists collaborate to define clinical needs, validate datasets, and design algorithms that meet specific healthcare requirements. Deployment requires careful planning and integration into existing workflows, ensuring seamless interaction between AI systems and healthcare professionals. Ongoing monitoring and evaluation by experts are crucial for identifying potential biases, errors, or unintended consequences, facilitating continuous improvement and adaptation.
Best Practices for Human-in-the-Loop Systems
Designing effective human-in-the-loop systems is vital for mitigating risks and maximizing the benefits of AI in healthcare. This involves creating systems where AI provides support and recommendations, but human experts retain ultimate decision-making authority. Clear guidelines and protocols should define when human intervention is required, ensuring timely and appropriate oversight. User interfaces should be intuitive and informative, enabling clinicians to easily understand AI outputs and assess their reliability.
Regular training and education for healthcare professionals are also crucial, enabling them to effectively utilize and interpret AI-generated insights. For instance, a system assisting in cancer diagnosis might flag potential issues, but a radiologist retains the final say on the diagnosis, ensuring accuracy and minimizing the impact of potential AI errors.
Continuous Monitoring and Evaluation of AI Systems
Continuous monitoring and evaluation are essential for ensuring the ongoing safety and efficacy of AI systems in healthcare. This involves tracking key performance indicators (KPIs), such as diagnostic accuracy, treatment outcomes, and user satisfaction. Regular audits and independent reviews should be conducted to identify any biases, errors, or unintended consequences. Feedback mechanisms should be in place to collect data from clinicians and patients, providing valuable insights for improving AI algorithms and workflows.
The process should be iterative, with ongoing refinement and adaptation based on real-world performance and emerging evidence.
A Hypothetical Scenario Illustrating Effective Human Oversight
Imagine a hospital deploying an AI system to predict patient deterioration. The AI, trained on historical data, identifies a patient at risk of sepsis. However, a nurse, reviewing the AI’s assessment alongside the patient’s overall clinical picture, notes inconsistencies. The patient’s vital signs are stable, and the AI’s prediction relies heavily on a single, potentially unreliable data point.
The nurse, exercising clinical judgment, decides against immediate intervention, opting instead for closer monitoring. This demonstrates how human oversight can prevent potentially harmful interventions based on flawed AI predictions, thus improving patient safety and avoiding unnecessary treatment.
Future Directions in Healthcare AI Governance
The rapid advancement of artificial intelligence (AI) in healthcare presents both immense opportunities and significant governance challenges. Successfully navigating this landscape requires proactive and adaptable frameworks that anticipate future technological developments and ethical considerations. Failing to do so risks hindering innovation while simultaneously increasing the potential for harm. This section explores emerging challenges, the influence of new technologies, and recommendations for creating robust and future-proof governance structures for AI in healthcare.
Emerging Challenges and Opportunities in Healthcare AI Governance
The increasing complexity of AI systems, coupled with their integration into critical healthcare processes, necessitates a more nuanced approach to governance. Challenges include ensuring data privacy and security in increasingly interconnected systems, mitigating algorithmic bias across diverse patient populations, and establishing clear lines of accountability when AI systems make critical decisions. Opportunities lie in leveraging AI to improve the efficiency and effectiveness of regulatory processes, promoting transparency and explainability in AI decision-making, and fostering collaboration between stakeholders to develop shared standards and best practices.
This collaborative approach is essential for building trust and ensuring responsible AI adoption.
The Impact of New Technologies on AI Healthcare Governance
Blockchain technology offers the potential to enhance data security and transparency in healthcare AI. By creating a secure, immutable record of data usage and AI model development, blockchain can increase accountability and build trust among patients and providers. Federated learning, which allows AI models to be trained on decentralized datasets without sharing sensitive patient information, offers a privacy-preserving approach to AI development and deployment.
These technologies, along with advancements in explainable AI (XAI), present significant opportunities to address key governance challenges and promote responsible innovation. For example, imagine a scenario where a blockchain records every access and modification of a patient’s medical data used to train an AI diagnostic tool. This creates an auditable trail, enhancing transparency and accountability.
Recommendations for Developing Adaptive and Flexible Governance Frameworks
Developing adaptive governance frameworks requires a multi-pronged approach. First, a focus on iterative regulatory processes is crucial. Regulations should be designed to be adaptable to the rapid pace of technological change, allowing for adjustments based on real-world experience and emerging challenges. Second, fostering collaboration between regulators, AI developers, healthcare providers, and patient advocacy groups is vital for developing shared standards and best practices.
Finally, investing in research and development to understand the long-term impacts of AI on healthcare is crucial for informing future governance decisions. This collaborative, adaptive approach ensures that governance keeps pace with technological advancements without stifling innovation.
Roadmap for Future Research and Development in Healthcare AI Governance
A robust roadmap for future research and development in healthcare AI governance should prioritize several key areas:
- Developing standardized methods for assessing and mitigating algorithmic bias in diverse healthcare settings.
- Exploring the ethical implications of using AI in decision-making processes that affect patient care, particularly in situations with high stakes.
- Investigating the effectiveness of different regulatory approaches to ensure patient data privacy and security in the context of AI.
- Creating frameworks for evaluating the transparency and explainability of AI systems used in healthcare.
- Developing methods for establishing clear lines of accountability and liability when AI systems make errors or cause harm.
- Researching the optimal balance between human oversight and AI autonomy in healthcare settings.
- Evaluating the impact of emerging technologies, such as blockchain and federated learning, on healthcare AI governance.
Last Word
Navigating the complex landscape of healthcare artificial intelligence governance oversight requires a multi-pronged approach. It demands collaboration between policymakers, healthcare professionals, AI developers, and ethicists. By proactively addressing the challenges of bias, transparency, and accountability, we can harness the transformative potential of AI while safeguarding patient well-being and upholding the highest ethical standards. The future of healthcare hinges on our ability to responsibly integrate this powerful technology.
User Queries
What are the biggest risks associated with AI in healthcare?
Major risks include algorithmic bias leading to unfair treatment, data breaches compromising patient privacy, lack of transparency making it difficult to understand AI decisions, and the difficulty in assigning accountability for errors.
How can we ensure fairness and equity in AI-driven healthcare?
Fairness requires diverse and representative datasets, rigorous testing for bias, ongoing monitoring of AI performance, and careful consideration of potential disparities in access to AI-powered healthcare.
What role do patients play in AI healthcare governance?
Patients need to be informed about how AI is used in their care, have access to clear explanations of AI-driven decisions, and be empowered to participate in discussions about the ethical implications of AI in healthcare.
What is the future of AI governance in healthcare?
The future likely involves adaptive governance frameworks that can evolve with technological advancements, increased international collaboration on standards, and a greater emphasis on patient-centric approaches to AI development and deployment.