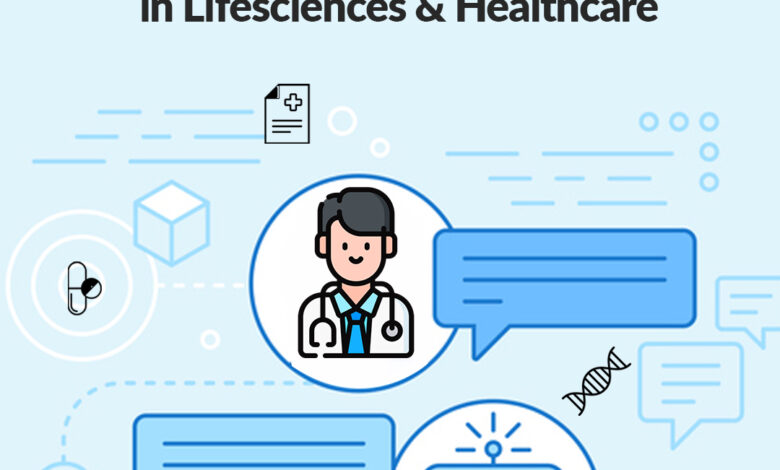
Healthcare Generative AI Adoption KLAS Research Insights
Healthcare generative AI adoption KLAS research reveals a rapidly evolving landscape. This exploration dives deep into the current state of generative AI in healthcare, examining its transformative potential across drug discovery, personalized medicine, and medical imaging. We’ll analyze the driving forces behind adoption, alongside the significant hurdles and ethical considerations that need addressing. This includes a close look at KLAS’s key findings and their implications for the future of healthcare.
From analyzing the various generative AI models employed (like LLMs, GANs, and VAEs) to understanding the regulatory complexities across different regions, we’ll paint a comprehensive picture. We’ll also delve into specific use cases, showcasing successful implementations and highlighting areas needing further development. Ultimately, we aim to provide a clear understanding of the opportunities and challenges facing the healthcare industry as it navigates this exciting, yet complex, technological frontier.
Healthcare Generative AI Landscape
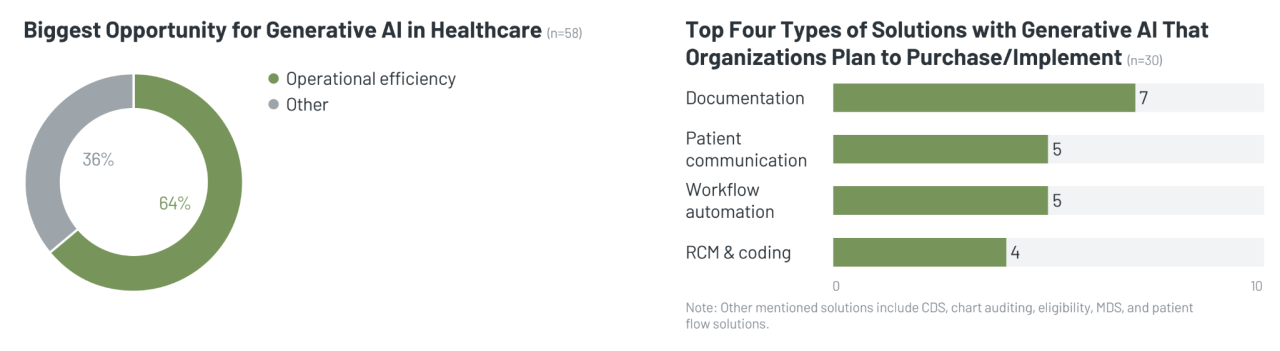
Source: hitconsultant.net
The healthcare industry is experiencing a transformative shift with the rapid advancement and adoption of generative AI. This technology, capable of creating new content ranging from text and images to code and even synthetic data, holds immense potential to revolutionize various aspects of healthcare, from drug discovery to personalized medicine. However, its implementation is still in its early stages, presenting both exciting opportunities and significant challenges.
Current State of Generative AI Adoption in Healthcare
Currently, the adoption of generative AI in healthcare is characterized by a mix of enthusiastic exploration and cautious implementation. While several companies and research institutions are actively developing and deploying generative AI solutions, widespread integration remains limited due to factors such as data privacy concerns, regulatory hurdles, and the need for robust validation and verification of AI-generated outputs. The focus is largely on specific applications where the potential benefits outweigh the risks, such as drug discovery and medical imaging analysis.
Many applications are still in the research and development phase, with pilot programs testing the efficacy and safety of these technologies in real-world settings.
My latest research on healthcare generative AI adoption, focusing on KLAS data, highlights the exciting potential for AI-driven solutions. This is particularly relevant given the recent breakthroughs in xenotransplantation, as evidenced by the FDA’s approval of clinical trials for pig kidney transplants in humans, which you can read more about here: fda approves clinical trials for pig kidney transplants in humans.
Such advancements could significantly impact the data sets used in training these AI models, potentially leading to even more sophisticated and effective healthcare applications.
Key Players and Their Roles
Several key players are shaping the healthcare generative AI landscape. Large technology companies like Google, Microsoft, and Amazon are contributing through their cloud platforms and AI development tools, providing the infrastructure and resources for researchers and healthcare providers. Pharmaceutical giants are investing heavily in generative AI for drug discovery and development, while specialized AI companies are focusing on developing specific healthcare applications, such as AI-powered diagnostic tools and personalized treatment plans.
Academic institutions and research hospitals play a crucial role in fundamental research, algorithm development, and validation of these technologies. For example, Google’s DeepMind has made significant contributions to protein folding prediction using AlphaFold, impacting drug discovery. Companies like Atomwise utilize AI to accelerate drug discovery, and PathAI focuses on improving the accuracy and efficiency of pathology diagnostics.
Types of Generative AI Models Used in Healthcare
Several generative AI model types are finding applications in healthcare. Large Language Models (LLMs), such as those based on transformer architectures, are used for tasks like generating medical reports, summarizing patient records, and assisting with clinical decision-making. Generative Adversarial Networks (GANs) are employed for generating synthetic medical images for training other AI models or augmenting datasets to improve the robustness of AI algorithms.
Variational Autoencoders (VAEs) are utilized for dimensionality reduction and data generation, often applied to medical imaging data for noise reduction or anomaly detection. The choice of model depends heavily on the specific application and the nature of the data being used.
Generative AI Applications in Healthcare
The table below showcases some key applications of generative AI in healthcare:
Application Area | AI Model Type | Key Features | Potential Benefits |
---|---|---|---|
Drug Discovery | LLMs, GANs | Molecule generation, prediction of drug efficacy and toxicity | Accelerated drug development, reduced costs, improved drug efficacy |
Medical Image Analysis | GANs, VAEs | Image generation, noise reduction, anomaly detection | Improved diagnostic accuracy, faster diagnosis, personalized treatment planning |
Personalized Medicine | LLMs | Generation of personalized treatment plans based on patient data | Improved treatment outcomes, reduced side effects, increased patient satisfaction |
Clinical Trial Design | LLMs | Optimization of clinical trial design, patient recruitment | Reduced costs, faster clinical trials, improved efficiency |
Adoption Drivers and Barriers: Healthcare Generative Ai Adoption Klas Research
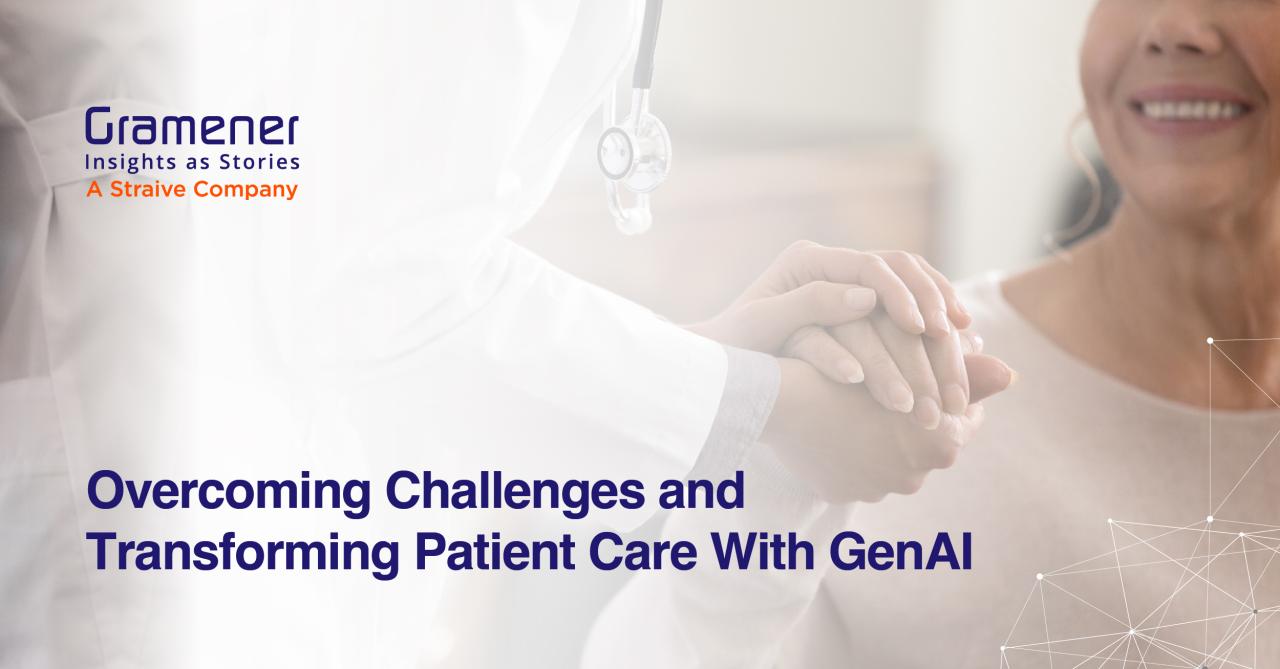
Source: gramener.com
The adoption of generative AI in healthcare is a complex interplay of powerful incentives and significant hurdles. While the potential benefits are transformative, real-world implementation faces considerable challenges, particularly concerning data privacy, regulatory compliance, and the need for robust validation. Understanding these driving forces and obstacles is crucial for navigating the path towards widespread and responsible AI integration within the healthcare sector.
Driving Forces Behind Generative AI Adoption in Healthcare
Several key factors are propelling the adoption of generative AI in healthcare. The most significant is the potential for improved efficiency and cost reduction. Generative AI can automate tasks like medical image analysis, report generation, and drug discovery, freeing up clinicians’ time and reducing operational costs. Furthermore, the ability of generative AI to analyze vast datasets and identify patterns invisible to the human eye offers the promise of improved diagnostic accuracy and personalized treatment plans.
This personalized approach, tailored to individual patient needs and characteristics, is another significant driver, as is the potential for accelerating research and development in areas such as drug discovery and disease modeling. The increasing availability of high-quality healthcare data, coupled with advancements in AI algorithms and computing power, further fuels this adoption.
Obstacles to Widespread Generative AI Adoption
Despite the considerable potential, several barriers hinder the widespread adoption of generative AI in healthcare. Data privacy and security are paramount concerns. Healthcare data is highly sensitive, and ensuring its protection is crucial. The risk of data breaches and unauthorized access poses a significant obstacle. Furthermore, the lack of standardized data formats and interoperability issues between different healthcare systems complicate the integration of AI tools.
The need for robust validation and regulatory approval processes also adds complexity and slows down adoption. Many healthcare professionals also express concerns about the “black box” nature of some AI algorithms, lacking transparency and explainability in their decision-making processes. Finally, the significant investment required for infrastructure, training, and maintenance can be prohibitive for some healthcare organizations.
Regulatory Landscape for AI in Different Regions
The regulatory landscape for AI in healthcare varies significantly across different regions. The European Union, with its General Data Protection Regulation (GDPR), has stringent data privacy regulations that impact the development and deployment of AI systems. The United States, while lacking a single, comprehensive AI regulatory framework, relies on a patchwork of existing regulations related to data privacy, medical devices, and healthcare information.
Other regions, such as Canada and Japan, are also developing their own regulatory frameworks, each with its own specific requirements and approaches. This diversity in regulatory approaches creates challenges for companies seeking to deploy AI solutions globally, necessitating compliance with varying standards and requirements across different jurisdictions. A lack of global harmonization in these regulations remains a major hurdle.
Examples of Successful and Unsuccessful Generative AI Implementations
Successful implementations often involve focusing on specific, well-defined tasks with readily available, high-quality data. For example, some AI systems have shown promise in accurately identifying cancerous lesions in medical images, significantly improving diagnostic accuracy and reducing the workload on radiologists. Conversely, unsuccessful implementations frequently stem from inadequate data quality, unrealistic expectations, or a lack of integration with existing workflows.
For instance, a project aiming to use generative AI for personalized treatment recommendations might fail due to insufficient patient data or the inability to seamlessly integrate the AI system into the clinical decision-making process. The success of generative AI in healthcare ultimately depends on a careful assessment of the specific application, data availability, and integration capabilities within the existing healthcare infrastructure.
Specific Applications of Generative AI in Healthcare
Generative AI, with its ability to create new data instances that resemble the training data, is rapidly transforming healthcare. Its applications span from accelerating drug discovery to personalizing patient care, offering the potential to revolutionize how we approach health and disease. This section will explore several key areas where generative AI is making a significant impact.
Generative AI in Drug Discovery and Development
Generative AI models are proving invaluable in accelerating the drug discovery and development process, a traditionally lengthy and expensive undertaking. These models can generate novel molecular structures with desired properties, significantly reducing the time and resources needed to identify potential drug candidates. For example, generative models can be trained on vast datasets of known drug molecules and their associated properties (e.g., efficacy, toxicity).
The model then uses this knowledge to generate new molecules predicted to possess improved characteristics. This significantly reduces the need for extensive and costly laboratory experiments in the initial stages of drug development. Furthermore, generative AI can predict the effectiveness of drugs against specific diseases and identify potential side effects, thereby improving the success rate of clinical trials.
One notable example is the use of generative models to design novel antibiotics to combat drug-resistant bacteria, a growing global health concern.
Generative AI in Personalized Medicine and Treatment Planning, Healthcare generative ai adoption klas research
Personalized medicine aims to tailor treatments to individual patients based on their unique genetic makeup, lifestyle, and medical history. Generative AI can play a crucial role in this process by analyzing patient data to create personalized treatment plans. For instance, generative models can be trained on patient data to predict the likelihood of a patient responding to a particular treatment.
This allows clinicians to select the most effective treatment strategy for each patient, potentially improving treatment outcomes and reducing adverse effects. Furthermore, generative AI can be used to simulate the effects of different treatments on individual patients, allowing clinicians to explore various treatment options and select the optimal approach. This approach can be particularly beneficial for patients with complex or rare diseases where standard treatment protocols may not be effective.
Generative AI in Medical Imaging Analysis
Medical imaging plays a vital role in diagnosis and treatment planning. Generative AI can significantly enhance the analysis of medical images by improving image quality, detecting subtle anomalies, and assisting in the development of new diagnostic tools. For example, generative models can be trained on large datasets of medical images to generate synthetic images that augment existing datasets. This is particularly useful in cases where obtaining sufficient real-world data is challenging.
Furthermore, generative AI can be used to improve the accuracy and efficiency of image segmentation and classification tasks, helping radiologists and other clinicians to more accurately identify and diagnose diseases. Consider the improved detection of tumors in MRI scans, where generative AI can enhance the contrast and clarity of the images, enabling earlier and more precise detection.
Generative AI in Patient Care and Support
Generative AI is also finding applications in improving patient care and support. For instance, generative models can be used to create personalized educational materials for patients, explaining complex medical information in a clear and accessible manner. These models can also generate chatbots that can answer patient questions, provide support, and schedule appointments, improving patient engagement and satisfaction. Furthermore, generative AI can be used to develop virtual assistants that can monitor patient health remotely, alerting clinicians to potential problems.
This could lead to earlier intervention and improved patient outcomes, particularly for patients with chronic conditions who require ongoing monitoring. An example might be a virtual assistant that analyzes patient-reported data and identifies potential signs of an impending exacerbation of a chronic respiratory illness, prompting the patient to contact their physician.
Ethical and Societal Implications
The integration of generative AI into healthcare presents a plethora of ethical and societal challenges that demand careful consideration. While the potential benefits are immense, the responsible development and deployment of these technologies are paramount to ensure equitable access, minimize harm, and uphold patient trust. Ignoring these implications could lead to significant negative consequences, undermining the very benefits generative AI promises.
Data Privacy and Security Concerns
Generative AI models require vast amounts of sensitive patient data for training and operation. This raises serious concerns about data privacy and security. Breaches could expose protected health information (PHI), leading to identity theft, financial loss, and reputational damage for patients. Robust security measures, including encryption, access controls, and anonymization techniques, are crucial to mitigate these risks.
Furthermore, adherence to regulations like HIPAA in the US and GDPR in Europe is non-negotiable. Transparency regarding data usage and clear consent protocols are also essential to build and maintain patient trust. For example, a hypothetical breach of a generative AI system used for diagnosing medical images could expose patient identities and sensitive medical conditions, leading to severe consequences.
Potential Biases in Generative AI Models
Generative AI models are trained on data, and if that data reflects existing societal biases (e.g., racial, gender, socioeconomic), the models will likely perpetuate and even amplify these biases in their outputs. This can lead to inaccurate diagnoses, inappropriate treatment recommendations, and unequal access to care. Mitigation strategies include careful curation of training datasets to ensure representation of diverse populations, algorithmic auditing to identify and correct biases, and the development of fairness-aware algorithms.
For instance, a bias in a model trained primarily on data from a specific demographic group might lead to misdiagnosis for patients from other groups.
Impact on the Healthcare Workforce
The introduction of generative AI in healthcare will undoubtedly impact the healthcare workforce. While some fear job displacement, a more nuanced perspective suggests a shift in roles and responsibilities. Generative AI can automate routine tasks, freeing up healthcare professionals to focus on more complex and patient-centric activities. However, it’s crucial to invest in reskilling and upskilling initiatives to equip the workforce with the necessary competencies to collaborate effectively with AI systems.
Successful integration will require a collaborative approach, where humans and AI work together to improve patient care. For example, radiologists could use AI to assist with image analysis, allowing them to focus on complex cases and interpretation requiring human expertise.
Responsible Development and Deployment of Generative AI in Healthcare
Responsible development and deployment of generative AI in healthcare requires a multi-faceted approach. This includes establishing clear ethical guidelines and regulatory frameworks, promoting transparency and explainability in AI systems, ensuring accountability for AI-driven decisions, and fostering public engagement and education. Collaborative efforts between researchers, clinicians, ethicists, policymakers, and the public are essential to navigate the complex ethical landscape and ensure that generative AI benefits all members of society equitably.
A crucial aspect is the development of robust mechanisms for oversight and accountability, to address potential harms and ensure responsible use. This could involve independent audits of AI systems and mechanisms for reporting and addressing adverse events.
Future Trends and Predictions
The integration of generative AI into healthcare is still in its nascent stages, yet its potential to revolutionize the industry is undeniable. We’re moving beyond the initial hype cycle, and a clearer picture of the technology’s trajectory is beginning to emerge. This section explores the future trends and predictions shaping the adoption of generative AI in healthcare over the next decade.The future of generative AI in healthcare hinges on several converging factors: increased computational power, the availability of larger and more diverse datasets, advancements in model explainability and trustworthiness, and a growing acceptance within the regulatory landscape.
These factors will not only drive broader adoption but also lead to the development of more sophisticated and specialized applications.
Generative AI Model Specialization and Refinement
Generative AI models are becoming increasingly specialized for specific healthcare tasks. Instead of general-purpose models, we’ll see a rise in models fine-tuned for tasks like drug discovery, personalized medicine, and medical image analysis. For example, a model trained on a massive dataset of oncology images might be far more accurate in detecting cancerous lesions than a general-purpose medical image analysis model.
This specialization will lead to improved accuracy, efficiency, and clinical relevance. We can expect to see a move away from large, general models towards smaller, more efficient, and task-specific models, optimized for deployment in resource-constrained environments like rural clinics.
Enhanced Data Security and Privacy Measures
As generative AI models rely heavily on patient data, robust security and privacy measures will be paramount. This will involve the development of advanced encryption techniques, federated learning approaches that allow model training on decentralized data sources without direct data sharing, and stronger regulatory frameworks to ensure patient data protection. The increasing emphasis on data privacy regulations like GDPR and HIPAA will drive innovation in secure AI development and deployment.
We can envision a future where differential privacy techniques are routinely implemented, allowing for the analysis of sensitive data while protecting individual identities.
Explainable AI (XAI) and Trust Building
One of the major barriers to widespread AI adoption is the “black box” nature of many current models. The lack of transparency in how AI models arrive at their conclusions can erode trust among clinicians and patients. The development of explainable AI (XAI) techniques will be crucial for building confidence and ensuring responsible AI implementation. XAI aims to make AI decision-making processes more transparent and understandable, allowing clinicians to assess the reliability and validity of AI-generated insights.
This will involve developing methods to visualize and interpret the internal workings of generative AI models, enabling clinicians to understand the reasoning behind their recommendations.
Integration with Existing Healthcare Systems
Successful integration of generative AI into existing healthcare workflows will require seamless interoperability with Electronic Health Records (EHRs) and other healthcare information systems. This involves developing APIs and standardized data formats to facilitate data exchange between AI systems and existing infrastructure. A scenario where AI-generated reports are directly integrated into EHRs, providing clinicians with real-time insights and recommendations, is likely within the next 5-10 years.
This will streamline clinical workflows and improve efficiency.
Potential Research Areas for Further Investigation
The successful and ethical implementation of generative AI in healthcare requires ongoing research. Key areas for future investigation include:
- Developing more robust and reliable methods for evaluating the performance and safety of generative AI models in healthcare settings.
- Investigating the ethical and societal implications of using AI for decision-making in healthcare, including issues of bias, fairness, and accountability.
- Exploring the potential for generative AI to address health disparities and improve access to quality healthcare in underserved communities.
- Developing innovative methods for training and validating generative AI models on diverse and representative datasets to mitigate bias and improve generalizability.
- Investigating the long-term effects of generative AI on healthcare professionals’ roles and responsibilities.
KLAS Research Insights
KLAS, a renowned healthcare research firm, provides invaluable insights into the adoption and impact of generative AI within the healthcare sector. Their reports offer a unique perspective, combining quantitative data with qualitative feedback from healthcare providers, painting a comprehensive picture of the current state and future trajectory of this rapidly evolving technology. This analysis will focus on key KLAS findings, comparing them with other market research and highlighting implications for healthcare organizations.
KLAS research consistently emphasizes the early stages of generative AI adoption in healthcare. While the potential benefits are widely recognized, practical implementation faces significant hurdles. This contrasts somewhat with more optimistic projections from some technology-focused market research firms, which often focus more on the technological potential than on the practical realities of implementation within complex healthcare environments.
Key Findings on Generative AI Adoption in Healthcare
KLAS reports reveal a cautious yet optimistic approach to generative AI among healthcare providers. Early adopters are primarily large, well-resourced organizations with dedicated IT departments and a strong commitment to innovation. These organizations are often exploring use cases in areas like clinical documentation, drug discovery, and patient communication, but full-scale integration remains limited due to concerns around data privacy, regulatory compliance, and the need for robust validation processes.
The research also highlights a significant skills gap, with many organizations lacking the expertise needed to effectively implement and manage generative AI systems.
Trends and Patterns Identified in KLAS Data
A significant trend identified by KLAS is the focus on specific, high-value use cases. Rather than attempting broad-scale implementation, organizations are prioritizing areas where generative AI can deliver immediate and measurable benefits. This strategic approach minimizes risk and allows for a more controlled evaluation of the technology’s impact. Another key pattern is the growing importance of vendor partnerships.
Healthcare organizations are increasingly relying on technology vendors to provide not only the AI tools but also the necessary support, training, and integration services. This reflects the complexity of integrating AI into existing healthcare workflows.
My latest research on healthcare generative AI adoption, specifically focusing on KLAS research findings, highlights the increasing importance of AI in diagnostics. A prime example of this trend is the exciting news about Google iCAD AI mammography expansion , which shows how AI is improving early detection and potentially saving lives. This successful implementation further supports the KLAS data suggesting a significant upward trajectory for AI adoption within the healthcare sector.
Comparison with Other Market Research Data
While KLAS focuses heavily on the practical implementation challenges faced by healthcare providers, other market research often emphasizes the immense potential of generative AI in healthcare. This difference in perspective highlights the gap between theoretical potential and practical realization. For instance, while some market reports predict exponential growth in generative AI adoption, KLAS data suggests a more gradual and measured approach, emphasizing the need for careful planning, validation, and risk mitigation.
This cautious approach is grounded in the reality of working with sensitive patient data and the need for rigorous quality assurance.
My latest deep dive into healthcare generative AI adoption, specifically the Klas research, highlighted some fascinating implications for patient care. Understanding the nuances of these AI tools is crucial, especially when considering conditions like stroke, where early intervention is vital. Learning about the risk factors that make stroke more dangerous is key to developing effective AI-driven preventative strategies.
Ultimately, this research underscores the potential for AI to improve healthcare outcomes, particularly in time-sensitive situations like stroke management.
Implications of KLAS Research for Healthcare Organizations
KLAS research underscores the importance of a phased and strategic approach to generative AI adoption. Organizations should prioritize high-value use cases, focus on building internal expertise, and forge strong partnerships with reputable technology vendors. Furthermore, careful consideration must be given to data privacy, regulatory compliance, and the need for robust validation processes. Ignoring these crucial factors could lead to significant challenges, wasted resources, and potential harm to patients.
Organizations should also actively monitor KLAS and other relevant research to stay informed about best practices and emerging trends in this rapidly evolving field. The insights provided by KLAS research are crucial for making informed decisions and mitigating the risks associated with AI adoption.
End of Discussion
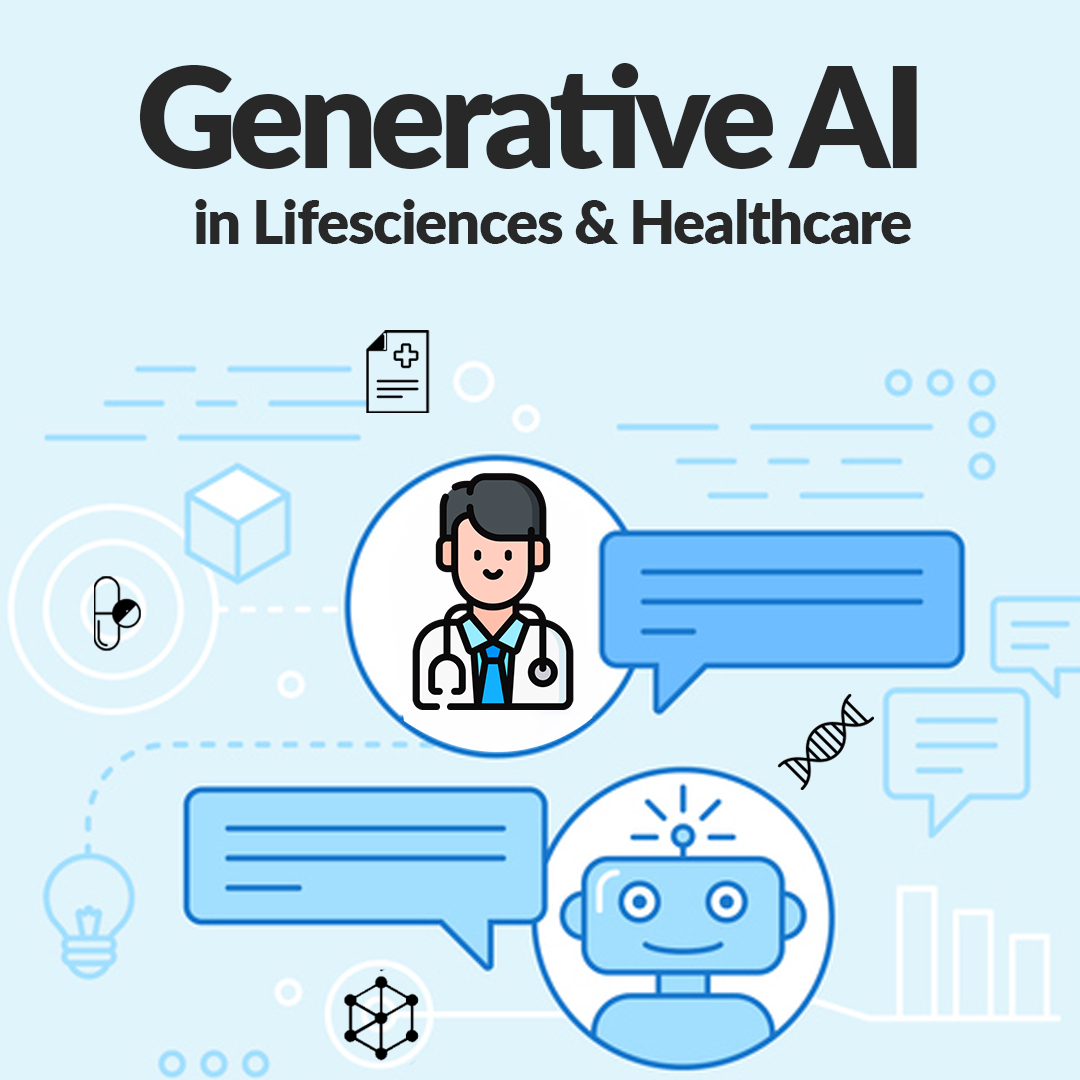
Source: nextgeninvent.com
The integration of generative AI into healthcare promises a revolution in patient care, drug discovery, and medical research. However, responsible adoption requires careful consideration of ethical implications, data privacy, and potential biases. KLAS research offers invaluable insights into the current adoption landscape, providing a roadmap for healthcare organizations looking to leverage the power of generative AI while mitigating its risks.
The future is undoubtedly shaped by this technology, and navigating it successfully requires a proactive and thoughtful approach.
Essential Questionnaire
What are the biggest risks associated with using generative AI in healthcare?
Major risks include data breaches, algorithmic bias leading to inaccurate diagnoses or treatments, and the potential displacement of healthcare professionals. Ensuring robust data security and developing bias mitigation strategies are crucial.
How is KLAS research different from other market research on AI in healthcare?
KLAS focuses heavily on provider perspectives and experiences, offering valuable insights into real-world adoption challenges and successes. Other research may focus more on technological advancements or market size predictions.
What is the role of regulatory bodies in the adoption of generative AI in healthcare?
Regulatory bodies play a critical role in ensuring safety, efficacy, and ethical use of AI in healthcare. They establish guidelines, approve AI-based medical devices, and monitor compliance to protect patients and maintain public trust.