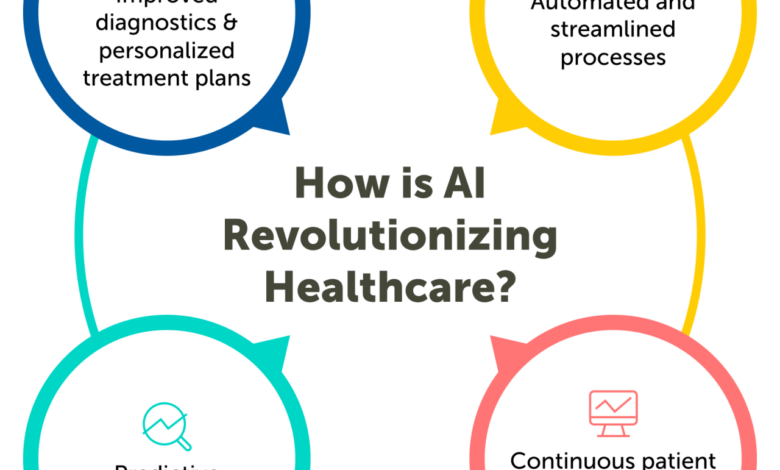
HHS Healthcare AI Strategic Plan A Deep Dive
HHS Healthcare AI Strategic Plan: It’s a mouthful, right? But behind that title lies a potentially revolutionary blueprint for how artificial intelligence will reshape healthcare. This isn’t just about robots replacing doctors; it’s about leveraging AI’s power to improve diagnostics, accelerate drug discovery, streamline administration, and ultimately, deliver better patient care. Think faster diagnoses, personalized treatments, and a more efficient healthcare system – all powered by AI.
This plan is ambitious, and I’m diving in to explore its promises and potential pitfalls.
The plan Artikels a multi-phased approach, addressing everything from the practical applications of AI in various medical fields to the crucial ethical considerations and data privacy concerns that come with such a significant technological shift. We’ll unpack the specifics of its proposed AI initiatives, delve into the potential benefits and challenges, and examine how the HHS aims to navigate the complex landscape of AI implementation in healthcare.
Get ready for a fascinating journey into the future of medicine!
HHS Healthcare AI Strategic Plan Overview
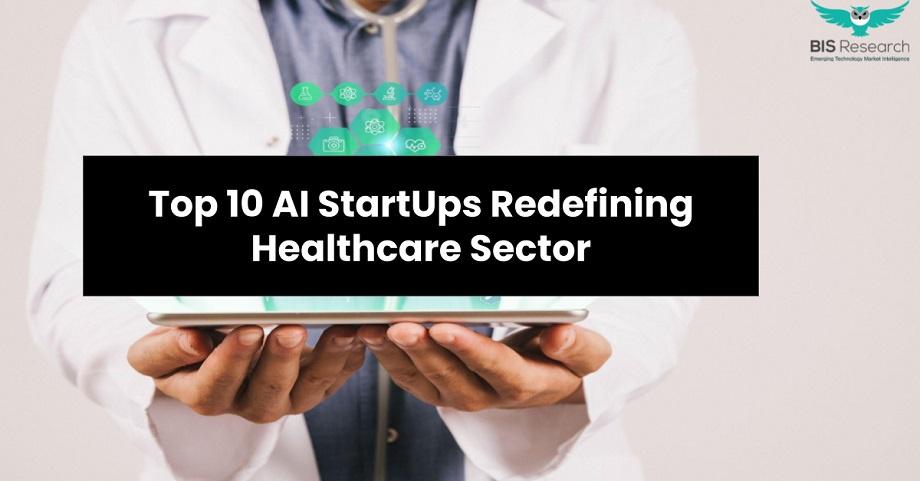
Source: imgur.com
The Department of Health and Human Services (HHS) Healthcare AI Strategic Plan Artikels a comprehensive approach to leveraging artificial intelligence (AI) to improve healthcare in the United States. It aims to accelerate the responsible development and deployment of AI to enhance the quality, efficiency, and equity of healthcare services. The plan acknowledges both the immense potential and the potential risks associated with AI in healthcare, emphasizing the need for ethical considerations and robust safeguards throughout the process.
Key Goals and Objectives of the HHS Healthcare AI Strategic Plan
The HHS Healthcare AI Strategic Plan sets ambitious goals, focusing on several key areas. These include improving patient care through more accurate diagnoses and personalized treatments, streamlining administrative processes to reduce costs and improve efficiency, advancing public health surveillance and response capabilities, and fostering innovation and collaboration within the healthcare AI ecosystem. Specific objectives revolve around developing AI-powered tools for early disease detection, enhancing healthcare access for underserved populations, and ensuring data privacy and security.
The plan also emphasizes the need for a skilled workforce capable of developing, implementing, and managing AI systems in healthcare.
Timeline and Phases of Implementation
While a precise, publicly available, detailed timeline with specific phases isn’t readily accessible, the implementation of the HHS Healthcare AI Strategic Plan is an ongoing process. It involves a phased approach, likely starting with pilot programs and focused initiatives before wider-scale deployment. This iterative approach allows for continuous evaluation and adjustment based on real-world results and feedback. The process involves collaboration between various HHS agencies, private sector partners, and academic institutions.
Examples of AI Initiatives Mentioned in the Plan
The HHS Healthcare AI Strategic Plan encompasses a broad range of AI initiatives. Examples include the development of AI-powered diagnostic tools for diseases like cancer and heart disease, the use of AI to predict and prevent hospital readmissions, and the application of AI to improve the efficiency of administrative tasks such as claims processing. The plan also highlights the potential of AI to improve public health surveillance by analyzing large datasets to identify and respond to outbreaks more effectively.
For instance, AI could be used to analyze social media data to detect early signs of an influenza outbreak or predict the spread of infectious diseases.
Key Components of the HHS Healthcare AI Strategic Plan
Component | Goal | Implementation Strategy | Expected Outcome |
---|---|---|---|
AI-Powered Diagnostics | Improve accuracy and speed of disease diagnosis | Develop and validate AI algorithms for image analysis, predictive modeling, and risk stratification. Partner with healthcare providers for pilot testing and implementation. | Earlier and more accurate diagnoses, leading to improved patient outcomes and reduced healthcare costs. |
AI for Administrative Efficiency | Reduce administrative burden and costs | Develop AI-powered tools for automating tasks such as claims processing, appointment scheduling, and medical record management. | Streamlined administrative processes, reduced costs, and improved efficiency for healthcare providers. |
AI for Public Health Surveillance | Enhance disease surveillance and outbreak response | Develop AI-powered systems for analyzing large datasets to identify patterns and predict outbreaks. Improve communication and coordination among public health agencies. | Faster detection and response to public health threats, reducing morbidity and mortality. |
AI Workforce Development | Build a skilled workforce in AI for healthcare | Invest in education and training programs to develop expertise in AI for healthcare professionals and researchers. Support the development of AI-related curricula in medical schools and other healthcare training programs. | Increased availability of skilled professionals to develop, implement, and manage AI systems in healthcare. |
AI Applications in Healthcare within the HHS Plan
The HHS Healthcare AI Strategic Plan Artikels a vision for leveraging artificial intelligence to revolutionize healthcare delivery, research, and administration. This involves a multifaceted approach, deploying AI across various sectors to improve efficiency, accuracy, and patient outcomes. The plan doesn’t just focus on the technology itself, but also on the ethical considerations and infrastructure needed for successful implementation.
The plan details several key areas where AI can significantly impact healthcare. These applications range from improving diagnostic accuracy and accelerating drug discovery to streamlining administrative processes and enhancing public health surveillance. However, the successful integration of AI into these areas faces significant hurdles, including data privacy concerns, algorithmic bias, and the need for robust infrastructure and skilled personnel.
AI in Medical Diagnostics
AI algorithms, particularly deep learning models, are being developed to analyze medical images (X-rays, CT scans, MRIs) and other patient data to assist in the detection and diagnosis of diseases. For instance, AI can identify subtle patterns indicative of cancer or other conditions that might be missed by the human eye, leading to earlier and more effective interventions. The accuracy of these AI-powered diagnostic tools is constantly improving, and they are increasingly being integrated into clinical workflows.
Challenges include ensuring the reliability and generalizability of these algorithms across diverse patient populations and integrating them seamlessly into existing healthcare systems.
AI in Drug Discovery and Development
AI is accelerating the drug discovery process by analyzing vast amounts of biological data to identify potential drug candidates and predict their efficacy and safety. Machine learning models can predict the likelihood of success for new drugs, reducing the time and cost associated with traditional drug development. For example, AI has been used to identify promising drug candidates for various diseases, including cancer and Alzheimer’s disease, leading to faster clinical trials and ultimately, quicker access to new treatments.
The HHS healthcare AI strategic plan is a huge undertaking, aiming to revolutionize healthcare delivery. It will be fascinating to see how this plan unfolds under the leadership of the newly confirmed HHS Secretary, as reported by rfk jr confirmed hhs secretary robert f kennedy jr. His perspectives on healthcare could significantly shape the implementation and priorities of this AI initiative, potentially impacting its overall success.
However, challenges remain in validating AI-generated predictions and ensuring that AI-designed drugs are safe and effective.
AI in Healthcare Administration
AI can significantly improve the efficiency and effectiveness of healthcare administration by automating tasks such as scheduling appointments, managing medical records, and processing insurance claims. This can free up healthcare professionals to focus on patient care, reducing wait times and improving overall patient experience. Natural Language Processing (NLP) is also being utilized to analyze unstructured data like doctor’s notes and patient records to extract meaningful information for better care coordination and population health management.
The implementation of AI in administration faces challenges in data security, interoperability between different systems, and the need for careful consideration of potential job displacement.
Comparison of AI Approaches, Hhs healthcare ai strategic plan
The HHS plan touches upon various AI approaches, including machine learning and deep learning. Machine learning involves training algorithms on large datasets to identify patterns and make predictions. Deep learning, a subset of machine learning, uses artificial neural networks with multiple layers to analyze complex data, often achieving higher accuracy in tasks such as image recognition and natural language processing.
While both approaches are valuable, deep learning often requires significantly more data and computational power. The choice of approach depends on the specific application and the availability of resources.
The HHS healthcare AI strategic plan aims to revolutionize healthcare delivery, but its implementation faces significant legal hurdles. The recent Supreme Court decision, as reported in this article scotus overturns chevron doctrine healthcare , could significantly impact regulatory oversight of AI in healthcare, potentially altering the timeline and scope of the HHS plan. This shift necessitates a reevaluation of the plan’s regulatory compliance strategies.
Potential Benefits of AI Applications in Healthcare
The potential benefits of implementing AI in healthcare, as envisioned by the HHS plan, are substantial. Below is a summary of the anticipated advantages for each application area:
The successful integration of AI across these areas requires careful planning, collaboration, and a commitment to addressing the ethical and practical challenges. However, the potential rewards are immense, promising a future where healthcare is more efficient, effective, and equitable for all.
- AI in Medical Diagnostics: Improved diagnostic accuracy, earlier disease detection, reduced diagnostic errors, personalized treatment plans.
- AI in Drug Discovery and Development: Accelerated drug development, reduced costs, identification of novel drug candidates, improved drug efficacy and safety.
- AI in Healthcare Administration: Streamlined administrative processes, reduced wait times, improved patient experience, better resource allocation, enhanced care coordination.
Data Privacy and Security Considerations
The HHS Healthcare AI Strategic Plan recognizes the paramount importance of protecting sensitive patient data. The plan Artikels a comprehensive framework for safeguarding patient information throughout the lifecycle of AI development, deployment, and use within the healthcare system. This includes robust security measures, stringent data governance policies, and adherence to relevant regulations and compliance standards. The goal is to ensure responsible innovation while maintaining the highest level of patient privacy and trust.The plan details a multi-layered approach to data security, encompassing technical, administrative, and physical safeguards.
This includes encryption of data both in transit and at rest, access control mechanisms based on the principle of least privilege, regular security audits and vulnerability assessments, and rigorous employee training programs on data security best practices. Furthermore, the plan emphasizes the importance of data minimization, only collecting and using the minimum necessary data for AI applications, reducing the potential impact of any breach.
Data Breach Response Strategy
A hypothetical scenario: Imagine a data breach involving a compromised server hosting patient data used for an AI-powered diagnostic tool. The breach exposes protected health information (PHI) for approximately 500 patients. The HHS plan’s response protocol would immediately activate, triggering a multi-stage incident response. This involves containment of the breach, investigation to determine the root cause and extent of the compromise, notification of affected individuals and relevant regulatory bodies (like OCR), remediation of vulnerabilities, and implementation of preventative measures to avoid future occurrences.
The plan mandates regular simulations of such events to ensure readiness and effectiveness of the response mechanisms. Detailed post-incident analysis would then be conducted to improve future preparedness.
Relevant Regulations and Compliance Standards
The plan explicitly addresses the key regulations and compliance standards governing the use of AI in healthcare, prioritizing adherence to HIPAA (Health Insurance Portability and Accountability Act), the 21 CFR Part 11 (electronic records and signatures), and NIST Cybersecurity Framework. Compliance with these standards is not just a matter of legal obligation; it is crucial for maintaining public trust and ensuring the ethical and responsible use of AI in healthcare.
The plan incorporates mechanisms for continuous monitoring and auditing to ensure ongoing compliance with these regulations and evolving best practices.
Robust Data Governance Strategies
The successful implementation of AI in healthcare hinges on robust data governance. The HHS plan emphasizes the following strategies:
- Data Inventory and Classification: A comprehensive inventory of all healthcare data used in AI applications, categorized by sensitivity level and access restrictions.
- Data Access Control: Implementing granular access control measures, ensuring that only authorized personnel with a legitimate need can access specific data sets.
- Data Security Policies and Procedures: Establishing clear and comprehensive policies and procedures for data security, including encryption, access control, and incident response.
- Data Retention and Disposal: Defining clear guidelines for the retention and secure disposal of data, adhering to legal and regulatory requirements.
- Regular Audits and Assessments: Conducting regular audits and assessments to monitor compliance with data governance policies and identify potential vulnerabilities.
- Data Privacy Impact Assessments: Conducting thorough data privacy impact assessments (DPIAs) before deploying any new AI application, to identify and mitigate potential privacy risks.
Ethical Implications of AI in Healthcare
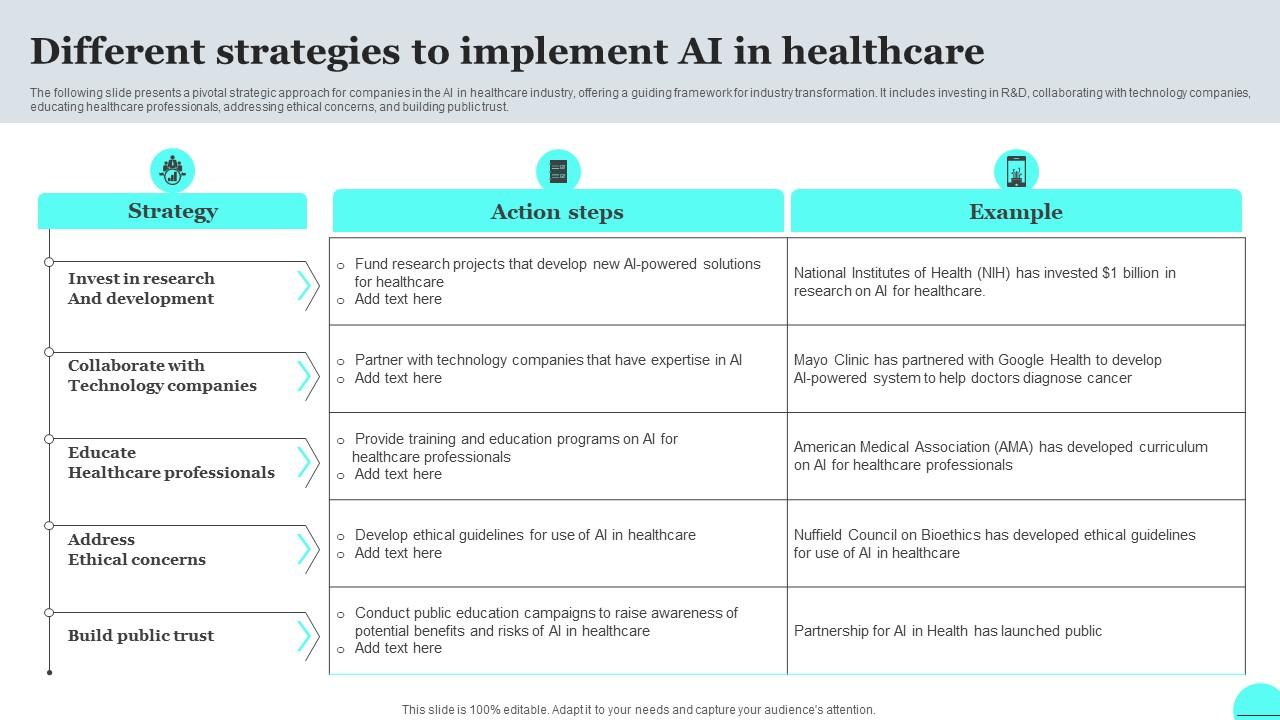
Source: slideteam.net
The integration of artificial intelligence (AI) into healthcare presents immense opportunities for improved diagnostics, treatment, and patient care. However, this transformative potential is inextricably linked to significant ethical considerations that demand careful attention. Failing to address these ethical implications risks undermining public trust and hindering the responsible adoption of AI in the healthcare sector. This section explores the key ethical challenges and the strategies Artikeld in the HHS Healthcare AI Strategic Plan to mitigate them.
Algorithmic bias and fairness are paramount concerns. AI algorithms are trained on data, and if that data reflects existing societal biases – for example, underrepresentation of certain demographic groups or historical prejudices – the resulting AI system will likely perpetuate and even amplify these biases in healthcare decisions. This can lead to disparities in access to care, diagnostic accuracy, and treatment outcomes, exacerbating existing health inequities.
Algorithmic Bias and Fairness in AI-Driven Healthcare Decisions
The HHS Healthcare AI Strategic Plan directly addresses the issue of algorithmic bias by emphasizing the need for diverse and representative datasets used in AI algorithm training. The plan promotes the development and use of methods to detect and mitigate bias in algorithms, including techniques like fairness-aware machine learning and rigorous auditing processes. Furthermore, the plan encourages transparency in algorithm development and deployment, allowing for scrutiny and identification of potential biases.
For example, if an algorithm trained primarily on data from a predominantly white population is used to diagnose skin cancer, it might be less accurate in identifying the disease in patients with darker skin tones, due to the limited representation of diverse skin types in the training data. The plan advocates for addressing this by ensuring diverse representation in datasets and employing techniques to reduce bias in the algorithms themselves.
Transparency and Accountability in AI-Driven Healthcare
Ensuring transparency and accountability in AI-driven healthcare decisions is crucial for maintaining public trust and fostering responsible innovation. The plan proposes mechanisms for documenting the decision-making processes of AI systems, including detailing the data used, the algorithms employed, and the rationale behind the decisions made. This allows for review and identification of potential errors or biases. Furthermore, the plan stresses the importance of establishing clear lines of responsibility and accountability for AI-driven decisions, identifying who is responsible when an AI system makes an incorrect diagnosis or recommends an inappropriate treatment.
This might involve establishing oversight boards or committees to review AI algorithms and their outputs, ensuring that human oversight remains a key component of the AI healthcare system.
Addressing Potential Biases in AI Algorithms
The HHS Healthcare AI Strategic Plan Artikels several strategies to address potential biases in AI algorithms. These include: (1) Promoting the use of diverse and representative datasets in algorithm training; (2) Developing and applying bias detection and mitigation techniques; (3) Implementing rigorous testing and validation procedures; (4) Establishing mechanisms for ongoing monitoring and evaluation of AI systems for bias; and (5) Encouraging collaboration between AI developers, healthcare providers, and ethicists to ensure ethical considerations are integrated throughout the AI development lifecycle.
For instance, a bias mitigation technique could involve weighting data points from underrepresented groups more heavily during training to compensate for historical imbalances.
Ethical Dilemmas, Impact, and Mitigation Strategies
Ethical Dilemma | Impact | Mitigation Strategy (HHS Plan) | Example |
---|---|---|---|
Algorithmic Bias leading to unequal access to care | Disproportionately affects vulnerable populations, exacerbating health disparities. | Develop and utilize bias detection and mitigation techniques; ensure diverse and representative datasets. | An AI system prioritizing patients based on socioeconomic status, resulting in delayed care for low-income individuals. |
Lack of transparency in AI decision-making | Erodes trust in AI systems and hinders accountability. | Establish mechanisms for documenting AI decision-making processes; ensure explainability and interpretability of algorithms. | An AI system recommending a treatment without providing a clear explanation of its reasoning. |
Data privacy and security breaches related to AI | Compromises sensitive patient information, leading to potential harm and legal repercussions. | Implement robust data privacy and security protocols; comply with relevant regulations (e.g., HIPAA). | A data breach exposing patient medical records used to train an AI algorithm. |
Over-reliance on AI leading to diminished clinical judgment | Reduces the role of human expertise and potentially compromises patient safety. | Maintain human oversight and ensure that AI systems are used to augment, not replace, clinical judgment. | A physician solely relying on an AI diagnosis without conducting a thorough physical examination. |
Impact and Future Directions
The HHS Healthcare AI Strategic Plan promises a transformative impact on healthcare delivery and patient outcomes. Its successful implementation will not only improve efficiency and reduce costs but also lead to more accurate diagnoses, personalized treatments, and ultimately, better health for all Americans. The plan’s far-reaching effects will be felt across the entire healthcare ecosystem, from individual providers to large hospital systems and research institutions.The plan’s projected impact encompasses several key areas.
Improved diagnostic accuracy, enabled by AI-powered image analysis and predictive modeling, will lead to earlier interventions and better treatment outcomes for conditions like cancer and cardiovascular disease. Personalized medicine, tailored to individual genetic profiles and lifestyle factors, will become more prevalent, leading to more effective therapies and fewer adverse drug reactions. Administrative tasks, such as scheduling and billing, will be streamlined, freeing up healthcare professionals to focus on patient care.
Furthermore, the improved efficiency will likely translate to cost savings for both patients and the healthcare system as a whole. For example, the reduction in hospital readmissions due to more accurate diagnoses and personalized care plans could generate substantial cost savings over time, mirroring successes seen in early AI adoption in areas like fraud detection.
Projected Improvements in Healthcare Delivery
The HHS plan envisions a future where AI assists in every aspect of healthcare delivery. Imagine a scenario where AI-powered diagnostic tools routinely flag potential issues during routine check-ups, allowing for early intervention and preventing serious illnesses. Consider personalized treatment plans, dynamically adjusting based on a patient’s real-time data, ensuring optimal effectiveness and minimizing side effects. Remote patient monitoring systems, powered by AI, could provide continuous health data analysis, allowing for proactive intervention and reducing hospital visits.
This could be especially beneficial for managing chronic conditions like diabetes and heart failure, reducing hospitalizations and improving quality of life for millions. Finally, AI-driven administrative systems could streamline the entire patient journey, from appointment scheduling to billing, improving efficiency and patient satisfaction.
Future Areas of Focus
Emerging trends in AI, such as federated learning and explainable AI (XAI), will shape the future direction of the HHS plan. Federated learning, which allows for collaborative AI model training without sharing sensitive patient data, will be crucial for protecting patient privacy while maximizing the benefits of data sharing. XAI, which focuses on making AI decision-making processes more transparent and understandable, will build trust and ensure accountability in AI-driven healthcare.
Furthermore, the plan will likely need to address the growing importance of AI in drug discovery and development, leveraging AI’s ability to accelerate the identification and development of new therapies. Finally, the ethical and societal implications of AI in healthcare will require ongoing attention and adaptation of the plan’s guidelines.
Fostering Innovation and Collaboration
The HHS plan emphasizes a collaborative approach to AI research and development, involving researchers, clinicians, technology developers, and policymakers. This collaborative approach will involve public-private partnerships, fostering innovation through shared resources and expertise. The plan will likely incentivize the development and adoption of AI-powered healthcare solutions through grants, regulatory streamlining, and other initiatives. Furthermore, it will prioritize the development of standardized data formats and interoperability standards to ensure seamless data exchange between different healthcare systems and AI platforms.
Open-source initiatives and data sharing agreements will be key to accelerating innovation and ensuring widespread access to AI-powered healthcare tools. The creation of a national AI healthcare consortium, comprised of leading researchers, clinicians, and industry experts, could serve as a central hub for collaboration and knowledge sharing.
A Realized Future Scenario
Imagine a future where AI has become an integral part of the healthcare system, seamlessly integrated into every aspect of care delivery. A patient experiencing chest pains goes to their local clinic, where AI-powered diagnostic tools quickly analyze an electrocardiogram (ECG), detecting a potential heart attack and alerting the physician immediately. The patient is swiftly transported to a hospital, where AI-driven robotic surgery assists in performing a life-saving procedure.
The HHS healthcare AI strategic plan aims to revolutionize patient care, and initiatives like telehealth are key. A great example of this in action is the expansion of convenient care options, such as those offered by the humana centerwell primary care centers walmart , which leverage technology to improve access and efficiency. Ultimately, these models align with the HHS’s broader goals for AI-driven healthcare improvements.
Post-surgery, the patient is monitored remotely through AI-powered wearables, which continuously track vital signs and alert healthcare providers to any potential complications. Personalized medicine ensures the patient receives the most effective treatment regimen, tailored to their individual genetic makeup and lifestyle. Administrative tasks are automated, ensuring a smooth and efficient patient experience. This is not science fiction; it’s the potential reality enabled by the HHS Healthcare AI Strategic Plan.
The improved diagnostics, personalized treatment, and streamlined administrative processes lead to better patient outcomes, reduced healthcare costs, and a more equitable healthcare system for all.
Epilogue
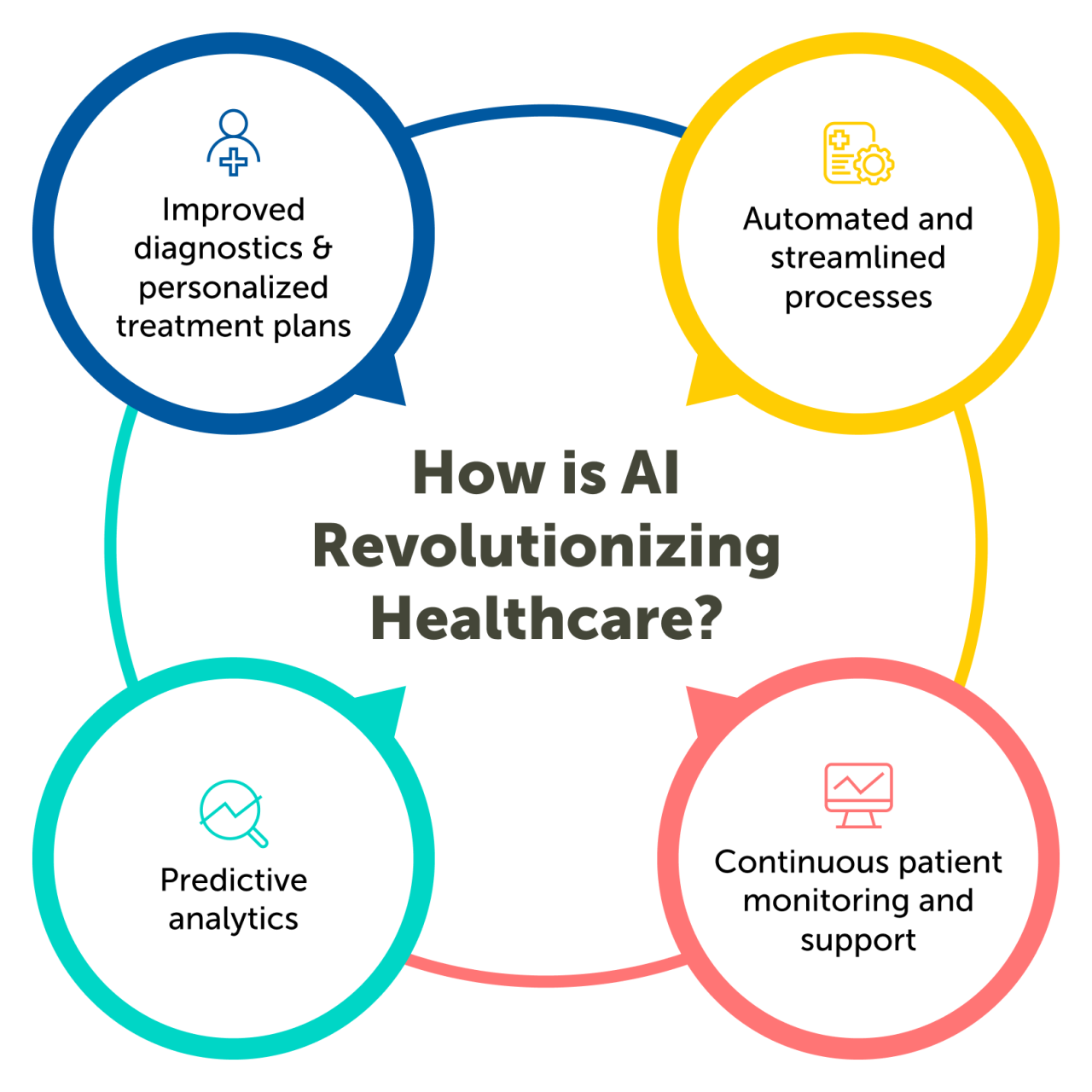
Source: fusemachines.com
The HHS Healthcare AI Strategic Plan represents a bold step towards a future where AI plays a central role in improving healthcare delivery. While challenges remain – particularly around data privacy, ethical considerations, and algorithmic bias – the potential benefits are immense. The plan’s focus on transparency, accountability, and robust data governance is encouraging. Ultimately, the success of this plan hinges on effective collaboration, responsible innovation, and a commitment to using AI to improve patient outcomes and create a more equitable healthcare system for everyone.
It’s a plan worth watching closely, and I’ll be keeping you updated on its progress.
Commonly Asked Questions
What are the biggest risks associated with implementing this plan?
The biggest risks involve potential data breaches, algorithmic bias leading to unfair treatment, and the need for significant investment in infrastructure and training.
How will the plan address potential job displacement due to AI?
The plan likely addresses this indirectly by focusing on upskilling and reskilling healthcare professionals to work alongside AI, rather than being replaced by it. Specific details on this aspect may require further investigation of the plan itself.
What is the timeline for the complete implementation of the plan?
The plan’s timeline isn’t explicitly detailed in the overview provided. More research is needed to pinpoint the exact timeframe for its complete implementation.