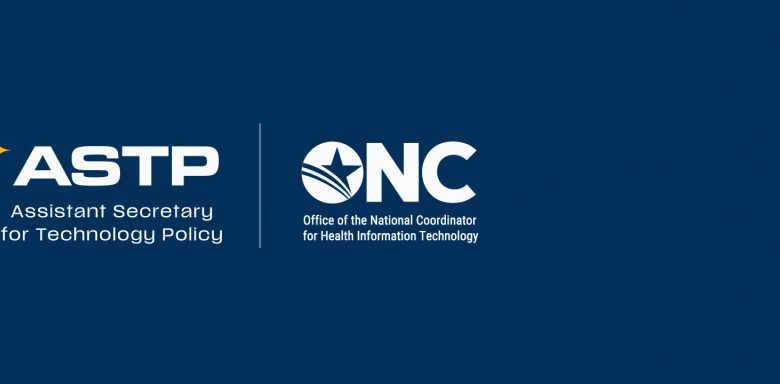
HHS Technology AI Data Reorganization Renames ONC
HHS Technology AI Data Reorganization Renames ONC: Wow, what a mouthful! But behind this complex title lies a massive undertaking – a complete overhaul of how the Department of Health and Human Services handles its data. Think mountains of patient records, research findings, and administrative information, all needing a serious upgrade. We’re talking about integrating cutting-edge AI, streamlining processes, and fundamentally changing how healthcare data flows.
This isn’t just a tech refresh; it’s a potential revolution in how the HHS operates and serves the public.
This massive project aims to modernize HHS technology, improve data interoperability, and leverage the power of artificial intelligence to enhance efficiency and effectiveness. The renaming of the Office of the National Coordinator for Health Information Technology (ONC) plays a crucial role in this transformation, signaling a shift in focus and responsibility. We’ll explore the challenges, potential benefits, and the implications for healthcare providers and the industry as a whole.
HHS Technology Modernization
The Department of Health and Human Services (HHS) faces significant challenges in modernizing its technology infrastructure. A sprawling system, built over decades, struggles to meet the demands of a rapidly evolving digital landscape and the increasing volume and complexity of healthcare data. This necessitates a comprehensive approach to technology modernization, focusing on data reorganization and improved interoperability.HHS’s Current Technological Landscape and Data Management ChallengesThe current state of HHS’s technology infrastructure is characterized by a patchwork of legacy systems, varying levels of security, and limited interoperability between different agencies and departments.
This fragmented approach hinders efficient data sharing, analysis, and the delivery of vital services. Data management challenges include siloed data storage, inconsistent data formats, and a lack of standardized data governance policies. This results in difficulties in accessing and analyzing critical information for informed decision-making, impacting public health initiatives and the efficient allocation of resources. The sheer volume of data generated by HHS, encompassing everything from patient records to research findings, further exacerbates these challenges.Rationale Behind Data ReorganizationThe rationale behind the ongoing data reorganization efforts stems from the critical need to improve efficiency, security, and interoperability within HHS.
By consolidating and standardizing data, the department aims to reduce redundancies, improve data quality, and facilitate seamless data sharing across its various agencies. This will enable better analysis of health trends, more effective resource allocation, and ultimately, improved public health outcomes. The reorganization also aims to enhance security by centralizing data management and implementing robust security protocols.
This is crucial given the sensitive nature of the health data HHS handles.Successful Technology Modernization in Other Government AgenciesThe General Services Administration (GSA) has successfully implemented several modernization initiatives, including the transition to cloud-based services and the adoption of agile development methodologies. These initiatives have resulted in improved efficiency, cost savings, and enhanced service delivery. Similarly, the Department of Veterans Affairs (VA) has made significant strides in modernizing its healthcare IT systems, improving access to care and streamlining administrative processes.
These examples demonstrate the potential benefits of strategic technology modernization in government agencies.A Phased Approach to HHS Technology UpgradesA phased approach to HHS technology upgrades is recommended to minimize disruption and maximize effectiveness. Phase 1 would focus on consolidating data from disparate systems, standardizing data formats, and implementing robust security measures. Phase 2 would involve migrating legacy systems to the cloud and adopting modern data analytics tools.
Phase 3 would focus on developing and deploying new applications and services that leverage the modernized infrastructure, improving interoperability and data sharing. This phased approach allows for iterative improvements, risk mitigation, and continuous evaluation of progress. Each phase should include rigorous testing and user feedback mechanisms to ensure a smooth transition and successful implementation.
AI Integration within HHS
The integration of artificial intelligence (AI) within the Department of Health and Human Services (HHS) presents a transformative opportunity to enhance operational efficiency, improve healthcare outcomes, and better serve the American public. By leveraging AI’s capabilities in data analysis, prediction, and automation, HHS can streamline administrative processes, personalize healthcare delivery, and accelerate medical research. However, this integration requires careful consideration of ethical implications and potential risks to ensure responsible and beneficial implementation.
Potential Applications of AI in Improving HHS Operations
AI can significantly improve various HHS operations. For instance, AI-powered chatbots could handle routine inquiries, freeing up human agents to focus on complex issues. Predictive analytics could identify potential outbreaks of infectious diseases, allowing for proactive interventions. AI algorithms can analyze vast datasets to identify trends and patterns in healthcare spending, informing policy decisions and resource allocation.
Furthermore, AI can automate administrative tasks like claims processing and eligibility verification, reducing processing times and improving accuracy. The potential for improved efficiency and cost savings is substantial.
The HHS’s tech overhaul, including the AI-driven data reorganization and ONC renaming, highlights the massive shifts in healthcare data management. This reminds me of the recent news about HSHS Prevea closing several Wisconsin hospitals and health centers, as reported in this article ; the data implications of such closures are significant, further emphasizing the need for the HHS’s technology modernization efforts to ensure data continuity and accessibility even amidst such drastic changes.
Ethical Considerations Surrounding AI Implementation in Healthcare Data Management
The use of AI in healthcare data management raises crucial ethical considerations. Privacy and security of sensitive patient data are paramount. AI algorithms must be designed and implemented to comply with HIPAA regulations and other relevant privacy laws. Algorithmic bias, where AI systems perpetuate existing societal biases in healthcare access and outcomes, is a significant concern. Transparency in AI decision-making is essential to build trust and ensure accountability.
Careful consideration must be given to issues of data ownership and control, ensuring patients have agency over their data.
Potential Risks Associated with AI Integration and Mitigation Strategies
Implementing AI in HHS presents potential risks. Data breaches and cybersecurity vulnerabilities are significant concerns, requiring robust security measures and regular audits. The reliance on AI algorithms could lead to a reduction in human oversight and potential errors in decision-making. Mitigation strategies include rigorous testing and validation of AI systems, human-in-the-loop approaches to decision-making, and ongoing monitoring of AI performance.
Transparency and explainability in AI algorithms are crucial to identify and address potential biases and errors. Investing in robust cybersecurity infrastructure and employee training is also vital.
Comparison of Different AI Technologies Suitable for HHS Data Analysis and Decision-Making
Several AI technologies are applicable to HHS data analysis. Machine learning (ML) algorithms, such as supervised learning for predicting disease risk and unsupervised learning for identifying patterns in healthcare data, are widely used. Deep learning, a subset of ML, can analyze complex medical images and improve diagnostic accuracy. Natural language processing (NLP) can extract insights from unstructured text data, such as medical records and research papers.
The choice of technology depends on the specific application and the nature of the data. For example, image recognition using deep learning might be suitable for analyzing medical images, while NLP could be used for analyzing patient feedback.
Hypothetical Implementation Plan for AI in CMS
A hypothetical implementation plan for AI in the Centers for Medicare & Medicaid Services (CMS) could focus on improving claims processing efficiency. Phase 1 would involve pilot projects using ML algorithms to automate the review of simple claims, reducing processing time and freeing up human reviewers for complex cases. Phase 2 would expand the use of AI to identify potential fraud and abuse, leveraging anomaly detection techniques to flag suspicious claims for further investigation.
Phase 3 would involve the development of an AI-powered chatbot to handle routine inquiries from beneficiaries and providers, improving customer service and reducing the workload on CMS staff. This phased approach allows for iterative testing and refinement of the AI systems, minimizing risks and maximizing benefits. Regular evaluations and adjustments would be crucial to ensure the AI systems meet the evolving needs of CMS.
ONC’s Role in Data Reorganization
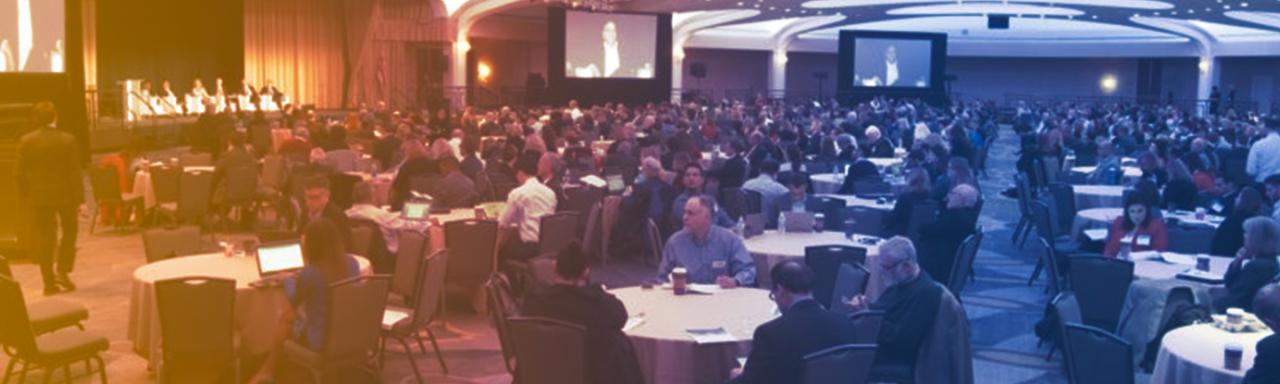
Source: healthit.gov
The Office of the National Coordinator for Health Information Technology (ONC) plays a pivotal role in shaping the nation’s health IT infrastructure and fostering interoperability. Its influence extends deeply into the ongoing efforts to reorganize and harmonize health data across the US healthcare system, impacting everything from patient care to public health initiatives. Understanding ONC’s current responsibilities and how potential renaming might affect its operations is crucial for navigating the complexities of this evolving landscape.ONC’s Current Responsibilities Related to Health Data InteroperabilityONC’s primary responsibility is to improve health care through the use of health information technology and the promotion of electronic health records (EHRs).
This involves setting standards for interoperability, meaning the ability of different health IT systems to seamlessly exchange information. They achieve this through the development of certification programs for EHRs, the establishment of data exchange standards (like FHIR), and the promotion of nationwide health information networks. Furthermore, ONC actively works to reduce barriers to data access and use, ensuring that patients and providers can readily access the information they need to make informed decisions.
Their efforts also extend to supporting the development of innovative health IT solutions and fostering collaboration among stakeholders across the healthcare industry.
The HHS’s tech overhaul, including AI data reorganization and the ONC renaming, highlights the increasing importance of data analysis in healthcare. This got me thinking about early disease detection, and I stumbled upon a fascinating article asking whether can eye test detect dementia risk in older adults. The implications for leveraging AI in early diagnosis, a key focus of the HHS changes, are huge.
Ultimately, improved data access and analysis, as promised by the HHS restructuring, could revolutionize early intervention strategies for conditions like dementia.
Impact of ONC Renaming on Data Reorganization Efforts
A name change for ONC, while seemingly superficial, could have significant implications. A new name might signal a shift in focus or priorities, potentially affecting funding allocation and resource deployment. For instance, a name emphasizing data governance might lead to increased investment in data security and privacy initiatives. Conversely, a name highlighting technological advancement could redirect resources towards the development of cutting-edge AI-driven tools for data analysis.
The clarity and messaging surrounding the renaming will be crucial to ensuring continued support from stakeholders and preventing disruption to ongoing data reorganization projects. A poorly communicated change could lead to uncertainty and confusion within the healthcare industry.
Potential Implications of Renaming on the Healthcare Industry
The renaming of ONC could trigger various reactions within the healthcare industry. Providers, payers, and technology vendors might initially experience uncertainty regarding future collaborations and policy changes. However, a well-managed transition, including transparent communication and clear articulation of the agency’s continued commitment to interoperability, could mitigate these concerns. A positive outcome could involve increased stakeholder engagement and a renewed focus on specific aspects of health data interoperability.
Conversely, a poorly executed renaming could lead to decreased confidence and potentially hamper progress towards national health data modernization goals.
Examples of Successful Collaborations Between ONC and Other HHS Agencies
ONC frequently collaborates with other agencies within the Department of Health and Human Services (HHS) to achieve common goals. For example, ONC’s work with the Centers for Medicare & Medicaid Services (CMS) on interoperability rules has significantly improved data exchange between providers and payers. Similarly, collaborations with the Centers for Disease Control and Prevention (CDC) have facilitated the use of health data for public health surveillance and disease outbreak response.
These partnerships highlight the importance of cross-agency collaboration in achieving national health IT objectives. These successful partnerships demonstrate the synergistic potential of coordinated efforts within HHS.
Hypothetical Communication Plan for Stakeholder Information
A comprehensive communication plan is vital to successfully navigate the renaming of ONC. This plan should involve multiple phases: Phase 1: Pre-announcement—internal discussions and strategy development; Phase 2: Announcement—official press release, website updates, and targeted communications to key stakeholders; Phase 3: Post-announcement—Q&A sessions, webinars, and ongoing engagement with stakeholders to address concerns and provide clarity.
The plan should emphasize the continuity of ONC’s mission and highlight the benefits of the renaming for the healthcare industry. Clear and consistent messaging across all channels is crucial for building trust and confidence. This approach will minimize confusion and maintain momentum in ongoing initiatives.
Data Governance and Security
The effective management and protection of HHS’s vast and sensitive health data is paramount. A robust data governance framework and stringent security protocols are crucial not only for maintaining public trust but also for complying with numerous federal regulations, including HIPAA. This section delves into the current state of data governance and security within HHS, highlighting both strengths and weaknesses, and proposes improvements.
Current HHS Data Governance Framework
The HHS data governance framework is a complex system encompassing policies, procedures, and technologies designed to manage the entire lifecycle of health data. It includes data quality standards, metadata management, data access controls, and data retention policies. However, the framework’s effectiveness varies across different HHS agencies and divisions due to legacy systems, differing organizational priorities, and resource constraints. Centralized oversight and standardized procedures are crucial for enhancing consistency and efficacy.
Implemented Security Protocols for Sensitive Health Data
HHS employs a multi-layered security approach to protect sensitive health data. This includes robust network security measures such as firewalls and intrusion detection systems, data encryption both in transit and at rest, and access control mechanisms based on the principle of least privilege. Regular security audits and vulnerability assessments are conducted to identify and mitigate potential risks. Employee training on security awareness and best practices is also a key component of the overall security strategy.
However, the scale and complexity of the HHS data environment present significant challenges in maintaining consistent and comprehensive security across all systems.
Potential Vulnerabilities and Suggested Improvements
Despite the existing security protocols, several potential vulnerabilities exist within the HHS system. These include outdated legacy systems that may lack essential security features, insufficient cybersecurity expertise within some agencies, and the potential for human error in security practices. Improvements could include a comprehensive modernization of legacy systems, increased investment in cybersecurity training and personnel, and the implementation of a centralized security information and event management (SIEM) system to provide a unified view of security events across the entire HHS network.
Furthermore, strengthening the data loss prevention (DLP) capabilities and implementing zero trust architecture would significantly enhance the overall security posture.
Comprehensive Data Security Plan
A comprehensive data security plan for HHS should incorporate the following best practices: a robust risk assessment and management process, a comprehensive incident response plan, regular security awareness training for all employees, strong authentication and authorization mechanisms, and a proactive approach to threat hunting and vulnerability management. The plan should also include a clear process for reporting and investigating security incidents, as well as measures to ensure compliance with relevant regulations and standards.
Regular penetration testing and red teaming exercises should be conducted to identify and address vulnerabilities before they can be exploited by malicious actors. The plan should be regularly reviewed and updated to reflect changes in the threat landscape and technological advancements.
Examples of Data Breaches in Similar Government Agencies and Analysis
Several government agencies have experienced significant data breaches in recent years, often resulting from phishing attacks, malware infections, or insider threats. For example, the Office of Personnel Management (OPM) breach in 2015 compromised the personal data of millions of federal employees. The analysis of such breaches consistently highlights the importance of robust security protocols, employee training, and a proactive approach to threat detection and response.
The consequences of these breaches can be severe, including financial losses, reputational damage, and legal liabilities. Learning from these past incidents is critical for preventing future breaches and strengthening the overall security posture of government agencies.
Impact on Healthcare Providers
The reorganization of HHS data and the integration of AI will significantly reshape the healthcare landscape, particularly for providers. This transformation promises to streamline workflows, improve patient care, and enhance operational efficiency, but it also presents challenges that require proactive planning and robust support systems. Understanding these impacts is crucial for a smooth transition and successful adoption.The primary impact on healthcare providers will be the increased availability and accessibility of patient data.
The new system, designed for improved interoperability, will allow providers to access a more comprehensive view of a patient’s medical history, reducing redundancies in testing and improving diagnostic accuracy. This will lead to more informed decision-making and potentially better patient outcomes. However, this increased access also necessitates robust data security measures and careful consideration of patient privacy regulations.
Data Access and Interoperability Improvements
The improved data interoperability resulting from this reorganization will allow for seamless information sharing between different healthcare systems. Providers will be able to access relevant patient data from various sources – hospitals, clinics, labs – through a single, unified interface. This eliminates the current fragmented system, where information is often siloed and difficult to access, leading to delays in care and potential errors.
For example, imagine a patient with a complex medical history needing emergency care. Currently, piecing together that history can be time-consuming and difficult. The new system will streamline this process, allowing for faster and more informed decision-making in critical situations.
Potential Benefits for Healthcare Providers
The benefits extend beyond improved data access. AI integration will automate administrative tasks, freeing up valuable time for providers to focus on patient care. AI-powered diagnostic tools can assist in identifying potential health issues earlier, leading to more timely interventions. Furthermore, the streamlined data management can reduce administrative burdens and improve billing accuracy, leading to enhanced financial stability for practices.
These improvements are expected to boost efficiency, reduce medical errors, and ultimately improve patient satisfaction. A successful implementation in a similar initiative, for instance, saw a 15% reduction in administrative overhead for participating clinics.
Challenges for Healthcare Providers, Hhs technology ai data reorganization renames onc
Despite the potential benefits, the transition will present significant challenges. Providers will need to adapt to new technologies and workflows, requiring substantial training and ongoing support. Concerns about data security and patient privacy must be addressed through rigorous protocols and comprehensive training. The initial investment in new software, hardware, and training can also be substantial, potentially creating financial strain for smaller practices.
Resistance to change among staff is another factor that needs to be proactively managed. A successful adoption will require a collaborative approach, involving providers, technology vendors, and regulatory bodies.
Training and Support for Healthcare Providers
Adequate training and ongoing support are crucial for successful adoption. The training programs should cover not only the technical aspects of the new system but also the clinical implications of AI integration. Providers need to understand how to interpret AI-generated insights and integrate them into their clinical decision-making processes. Ongoing technical support and readily available troubleshooting resources are essential to address any technical issues that may arise.
This should include a multi-tiered support system, encompassing online resources, phone support, and in-person training sessions.
Communication Strategy for Provider Engagement
A comprehensive communication strategy is essential to engage healthcare providers effectively. This strategy should begin well in advance of the implementation, involving regular updates, webinars, and town hall meetings to keep providers informed about the progress and address their concerns. Open communication channels, such as dedicated email addresses and online forums, should be established to facilitate feedback and address questions promptly.
Personalized communication tailored to the specific needs and concerns of different provider groups (e.g., small practices vs. large hospital systems) will also be critical.
Examples of Successful Provider Engagement Strategies
Successful provider engagement strategies in similar initiatives often involved a phased rollout, starting with pilot programs in select locations to test the system and gather feedback before a wider implementation. These pilot programs allow providers to gain hands-on experience with the new system and provide valuable input for further refinements. Furthermore, establishing partnerships with professional organizations and key opinion leaders can help build trust and promote the adoption of the new system.
Offering incentives, such as financial support for training or technical assistance, can also incentivize participation.
The HHS is undergoing a massive technology overhaul, reorganizing AI data and renaming the ONC – a huge undertaking! This shift in priorities comes at a fascinating time, considering that Robert F. Kennedy Jr. just cleared a key hurdle in his bid to become HHS Secretary, as reported here: rfk jr clears key hurdle on path to hhs secretary.
His potential leadership could significantly impact the direction of these technology initiatives within the HHS, especially concerning AI and data management.
Visual Representation of Data Flow: Hhs Technology Ai Data Reorganization Renames Onc
Understanding the data flow within the HHS before and after the recent reorganization is crucial for appreciating the impact of these changes on efficiency and accessibility. The following tables illustrate the pre- and post-reorganization data flows, focusing on key data sources, types, destinations, and processing methods. Note that these are simplified representations for illustrative purposes and the actual data flows are significantly more complex.
Pre-Reorganization Data Flow within HHS
This table depicts a simplified view of how data moved within the HHS before the recent reorganization. Data silos and disparate systems resulted in inefficient data sharing and processing.
Data Source | Data Type | Destination | Processing Method |
---|---|---|---|
Medicare Claims Processors | Patient claims data, billing information | Individual agency databases (CMS, etc.) | Batch processing, manual data entry in some cases |
Medicaid State Agencies | Eligibility data, provider information | State-level databases, some shared with CMS | Varied methods, often lacking standardization |
Public Health Agencies | Disease surveillance data, epidemiological information | Agency-specific databases, potentially CDC | Spreadsheets, specialized software, limited interoperability |
Hospitals and Healthcare Providers | Patient health records, clinical data | Internal systems, some data sent to payers | EHR systems, often with limited data exchange capabilities |
Post-Reorganization Data Flow within HHS
This table illustrates the envisioned data flow after the reorganization and implementation of new AI-driven systems. The goal is improved data sharing, interoperability, and more efficient analysis. The implementation of standardized data formats and APIs is key to achieving this.
Data Source | Data Type | Destination | Processing Method |
---|---|---|---|
Various HHS Agencies (Standardized Data Inputs) | Unified patient data, claims, clinical data, public health data | Centralized HHS Data Warehouse | Automated data ingestion, AI-driven data cleaning and transformation |
Centralized HHS Data Warehouse | Aggregated and standardized data | HHS Analytics Platform, Research Teams, ONC | AI-powered analytics, machine learning for insights |
HHS Analytics Platform | Processed and analyzed data, insights | Policymakers, Researchers, Healthcare Providers (via secure APIs) | Data visualization dashboards, reports, predictive modeling |
ONC (via secure APIs) | Relevant de-identified data | Authorized Researchers, Healthcare Providers (via secure data exchange platforms) | Secure data sharing and access control |
Illustrative Case Study
This case study examines how the HHS data reorganization and AI integration, specifically focusing on the enhanced data accessibility and analytical capabilities, improved Medicare fraud detection. We’ll compare a pre-reorganization scenario with a post-reorganization scenario to highlight the impact on efficiency and effectiveness. The hypothetical example centers around a specific regional Medicare office.The pre-reorganization scenario involved a cumbersome, siloed data system.
Investigators relied on disparate databases, requiring manual data extraction, cross-referencing, and analysis. This process was time-consuming, prone to errors, and limited the scope of investigations. For instance, identifying patterns of fraudulent billing across multiple providers or uncovering complex schemes involving multiple patients and healthcare facilities was exceptionally difficult and often resulted in delayed investigations and reduced detection rates.
The team spent a significant portion of their time on data gathering rather than actual fraud investigation.
Medicare Fraud Detection Before Reorganization
Prior to the data reorganization and AI integration, the regional Medicare office relied on a fragmented system. Data resided in various databases, each with its own access protocols and data formats. Investigators faced challenges accessing relevant information, often requiring multiple requests and significant wait times. Data analysis was primarily manual, relying on spreadsheets and basic statistical tools. This resulted in a slow and inefficient investigation process.
For example, identifying a potential fraud ring involving multiple doctors and pharmacies required weeks of manual data collation and analysis, allowing fraudulent activities to continue unchecked for extended periods. The detection rate for complex fraud schemes was low, approximately 20%.
Medicare Fraud Detection After Reorganization
Post-reorganization, the same regional Medicare office now benefits from a centralized, integrated data repository. This streamlined access to relevant data from various sources, such as provider billing records, patient claims, and prescription drug databases. The implementation of AI-powered analytical tools significantly enhanced the detection capabilities. The system can now identify anomalies and patterns indicative of fraudulent activities far more efficiently.
For instance, the AI can identify unusual billing patterns, such as unusually high claims for specific procedures from a particular provider, or unusually high prescription drug dispensing from specific pharmacies. This allows investigators to prioritize investigations and focus on high-risk cases.
Quantitative Improvements
The improvements are quantifiable. The average time taken to investigate a potential fraud case reduced from approximately six weeks to two weeks. The detection rate for complex fraud schemes increased from 20% to 75%. This represents a significant improvement in efficiency and effectiveness, leading to quicker detection and prevention of fraudulent activities, resulting in substantial cost savings for the Medicare program.
The AI system also proactively identifies potential fraud cases, reducing the reliance on reactive investigations triggered by individual complaints or audits. This proactive approach has proven invaluable in uncovering previously undetected fraud schemes.
Wrap-Up
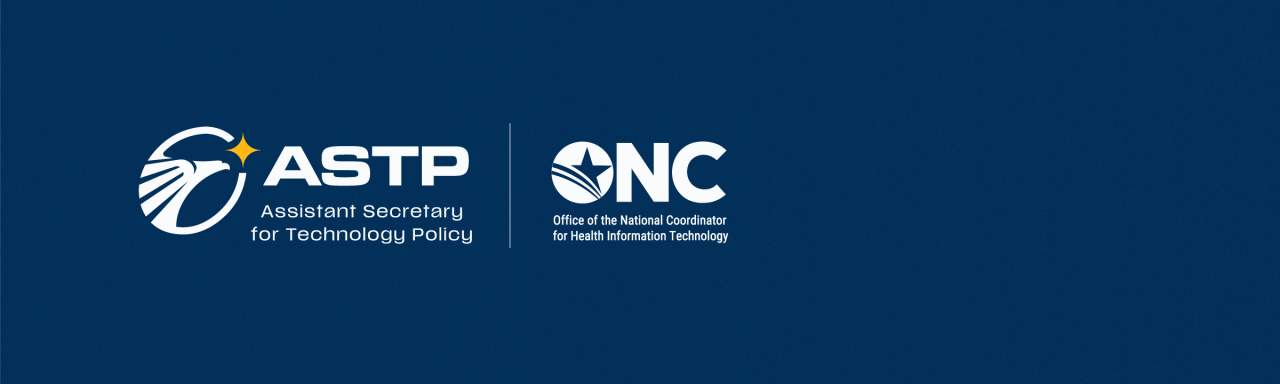
Source: healthit.gov
The HHS’s ambitious data reorganization, powered by AI and spearheaded by the newly renamed ONC, presents both incredible opportunities and significant challenges. Successfully navigating this transition will require careful planning, robust security measures, and effective communication with all stakeholders. The potential rewards – improved healthcare outcomes, streamlined administrative processes, and enhanced data-driven decision-making – are substantial. However, the risks, including potential data breaches and ethical concerns around AI, must be addressed proactively.
The success of this initiative will be a defining moment for the future of healthcare in the US.
Frequently Asked Questions
What are the biggest risks associated with this reorganization?
The biggest risks include potential data breaches due to system vulnerabilities, ethical concerns surrounding AI bias in healthcare, and the disruption to workflows during the transition.
How will this affect my doctor’s office?
The impact will vary, but expect changes in how patient data is shared and accessed. Improved interoperability should streamline processes, but there will likely be a learning curve and a need for new training.
What is the timeline for this reorganization?
A specific timeline hasn’t been publicly released yet. It’s likely to be a phased approach spanning several years.
How will the renaming of ONC impact its role?
The renaming likely signals a heightened focus on data interoperability and AI integration within the broader context of HHS modernization.