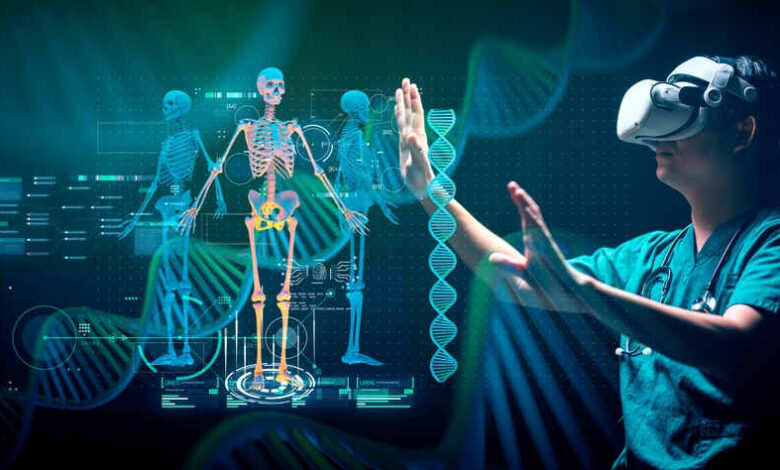
Hospital AI Evaluation AI Bias & Health Affairs
Hospital AI evaluation ai bias health affairs – Hospital AI evaluation, AI bias, and health affairs are inextricably linked. We’re diving deep into the critical issue of algorithmic bias in healthcare, exploring how it impacts patient care, resource allocation, and overall health equity. This isn’t just about faulty code; it’s about the potential for AI to perpetuate and even amplify existing societal inequalities within our hospitals. We’ll examine real-world examples, discuss strategies for mitigation, and consider the crucial role of regulatory bodies in ensuring fair and ethical AI implementation.
This exploration will cover various types of bias, from subtle data imbalances to more overt discriminatory outcomes. We’ll dissect how biased algorithms can lead to misdiagnosis, inappropriate treatment plans, and unequal access to resources. Furthermore, we’ll delve into the ethical considerations, proposing practical solutions and policy recommendations to navigate this complex landscape and build a future where AI enhances, rather than hinders, equitable healthcare for all.
Defining AI Bias in Healthcare: Hospital AI Evaluation Ai Bias Health Affairs
AI is rapidly transforming healthcare, offering the potential for improved diagnostics, personalized treatments, and more efficient resource allocation. However, the increasing reliance on AI algorithms also introduces significant risks, particularly the pervasive issue of AI bias. Understanding and mitigating this bias is crucial to ensuring equitable and effective healthcare for all.AI bias in healthcare refers to systematic and repeatable errors in an AI system that create unfair or discriminatory outcomes for certain groups of patients.
This bias can stem from various sources, leading to disparities in diagnosis, treatment, and access to care. It’s not a matter of malicious intent, but rather a consequence of flawed data, algorithm design, or both.
Types of AI Bias in Healthcare
The manifestation of bias in healthcare AI is multifaceted. Several types of bias can independently or collectively influence the accuracy and fairness of these systems. These biases can subtly or overtly disadvantage specific patient populations.
Algorithmic Bias Affecting Patient Outcomes
Algorithmic bias can significantly impact patient care across multiple stages. For example, a biased diagnostic tool trained on data predominantly representing a certain demographic might misdiagnose conditions in patients from underrepresented groups. Similarly, treatment recommendations generated by a biased algorithm could lead to inappropriate or suboptimal care, potentially worsening health outcomes for specific populations. In resource allocation, biased algorithms could unfairly prioritize patients from certain demographics for limited resources such as organ transplants or specialized treatments.For instance, imagine a skin cancer detection AI trained primarily on images of light-skinned individuals.
This algorithm might exhibit lower accuracy in detecting skin cancer in patients with darker skin tones, leading to delayed diagnosis and potentially poorer prognoses. This is a clear example of how data bias – the skewed representation of certain demographics in the training data – translates into algorithmic bias with real-world consequences. Another example could be an AI system used for predicting hospital readmission risk.
If the training data underrepresents certain socioeconomic groups, the system might inaccurately predict the risk for those groups, leading to inadequate discharge planning and increased readmissions. This, in turn, could disproportionately affect patients from disadvantaged communities.
Societal Impact of Biased AI in Healthcare
The societal implications of biased AI in healthcare are profound. Biased algorithms can exacerbate existing health disparities, leading to inequitable access to quality care. This can disproportionately affect vulnerable populations, including racial and ethnic minorities, individuals from lower socioeconomic backgrounds, and those with disabilities. The resulting health inequities can have long-term consequences on individual well-being, community health, and societal trust in healthcare systems.
The lack of access to accurate and unbiased AI-driven healthcare can lead to increased morbidity and mortality rates in already disadvantaged communities, widening the health gap between different segments of society. The erosion of trust in healthcare systems due to perceived or actual biases further compounds the problem, potentially hindering efforts to improve health outcomes for all.
Evaluating AI Systems for Bias Mitigation
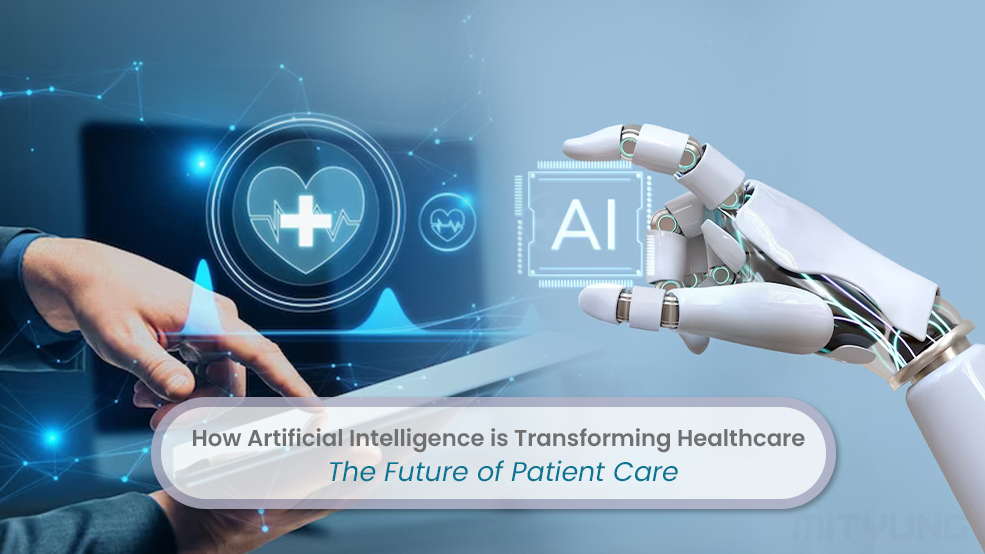
Source: mityung.com
AI bias in healthcare is a significant concern, potentially leading to disparities in diagnosis, treatment, and overall patient outcomes. Effectively mitigating this bias requires a multi-faceted approach encompassing the entire AI lifecycle, from data collection to deployment and ongoing monitoring. This involves proactively identifying potential sources of bias and implementing strategies to minimize their impact.
Strategies for identifying and mitigating bias during the development and deployment of AI systems in hospitals involve a rigorous and iterative process. This process requires careful consideration at every stage, from data acquisition to model validation and post-deployment monitoring. It is not a one-time fix, but rather an ongoing commitment to fairness and equity.
Bias Identification Strategies
Identifying bias requires a combination of technical and human-centered approaches. Technical methods include analyzing the training data for imbalances in representation across different demographic groups, examining model predictions for disparities, and using fairness-aware algorithms. Human-centered approaches involve engaging diverse stakeholders, including clinicians, patients, and community members, to identify potential biases and evaluate the fairness of the AI system. For example, a review of the training data for a diagnostic tool might reveal an underrepresentation of patients from certain racial or socioeconomic backgrounds, potentially leading to biased predictions for those groups.
A multidisciplinary team could then work to address this imbalance by actively seeking out and including data from underrepresented populations.
Bias Mitigation Techniques
Once bias is identified, various mitigation techniques can be employed. Data preprocessing methods, such as re-sampling or re-weighting, can adjust for imbalances in the training data. Algorithmic fairness constraints can be incorporated during model training to ensure that the model does not discriminate against specific groups. Furthermore, post-processing methods can modify the model’s predictions to reduce disparities.
For instance, if a model consistently underpredicts the risk of a certain condition for a particular demographic group, a post-processing step might adjust the predictions to ensure more equitable risk assessment. However, it’s crucial to remember that these methods aren’t always perfect and may require careful consideration to avoid unintended consequences.
Checklist for Evaluating AI Fairness and Equity
A comprehensive checklist is crucial for evaluating the fairness and equity of AI algorithms in healthcare. This checklist should cover various aspects of the AI system’s development and deployment.
The following checklist provides a framework for a thorough evaluation:
- Data Collection and Preprocessing: Assess the representativeness of the training data, including demographic characteristics and clinical factors. Examine data collection methods for potential biases and evaluate preprocessing steps for fairness implications.
- Algorithm Design and Training: Evaluate the algorithm’s design for potential biases and assess the training process for fairness concerns. Consider the use of fairness-aware algorithms and metrics.
- Model Validation and Testing: Conduct rigorous validation and testing on diverse datasets to assess the model’s performance across different demographic groups. Use appropriate fairness metrics to quantify disparities.
- Deployment and Monitoring: Establish a monitoring system to track the AI system’s performance in real-world settings and identify potential biases over time. Implement mechanisms for feedback and continuous improvement.
- Transparency and Explainability: Ensure the AI system is transparent and explainable, allowing clinicians and patients to understand its decision-making process and identify potential biases.
Methods for Assessing Bias in AI-Driven Diagnostic Tools and Treatment Recommendations
Several methods can be used to assess bias in AI-driven diagnostic tools and treatment recommendations. These methods often involve comparing the model’s performance across different subgroups defined by demographic or clinical characteristics.
Common methods include:
- Disparate Impact Analysis: This method compares the positive predictive value (PPV) or negative predictive value (NPV) of the AI system across different demographic groups. Significant differences may indicate bias.
- Equalized Odds: This approach assesses whether the AI system achieves similar true positive rates (TPR) and true negative rates (TNR) across different groups.
- Calibration Analysis: This examines whether the AI system’s predicted probabilities accurately reflect the true probabilities of the outcome across different groups.
For example, in a study evaluating an AI-driven diagnostic tool for heart disease, disparate impact analysis might reveal that the tool has a significantly lower PPV for women compared to men. This would suggest potential bias in the tool’s predictions. Further investigation could then be undertaken to identify the root cause of this disparity and implement appropriate mitigation strategies.
The Role of Health Affairs in Addressing AI Bias
The increasing integration of artificial intelligence (AI) into healthcare presents immense opportunities for improved patient care, but also significant risks if not implemented ethically and responsibly. Addressing AI bias is paramount to ensuring fairness, equity, and trust in these systems. Health affairs, encompassing regulatory bodies and health policy, play a crucial role in mitigating these risks and fostering the beneficial application of AI in healthcare.The use of AI in hospitals necessitates a robust regulatory framework to prevent and mitigate bias.
This framework should address data collection, algorithm development, deployment, and ongoing monitoring. Without careful oversight, AI systems can perpetuate and even amplify existing health disparities, leading to unequal access to care and potentially harmful outcomes for certain patient populations.
Regulatory Oversight and Health Policy in Addressing AI Bias
Regulatory bodies must establish clear guidelines and standards for the development, validation, and deployment of AI-based healthcare tools. These guidelines should mandate rigorous testing for bias, transparency in algorithmic design, and mechanisms for accountability when errors occur. Health policy should incentivize the development and adoption of AI systems that demonstrate fairness and equity across diverse patient populations. This might involve funding research into bias detection and mitigation techniques, establishing certification processes for AI-based healthcare tools, and providing financial support for hospitals implementing bias-mitigation strategies.
For example, the FDA’s increasing involvement in regulating AI-based medical devices reflects a growing awareness of the need for regulatory oversight in this area. Their focus on pre-market review and post-market surveillance helps ensure the safety and effectiveness of these technologies.
Ethical Considerations: Fairness and Accountability
The ethical implications of AI in healthcare are profound. Fairness demands that AI systems do not discriminate against patients based on race, gender, socioeconomic status, or other protected characteristics. Accountability requires that developers and deployers of AI systems are responsible for the outcomes produced by their algorithms. This includes establishing clear lines of responsibility for addressing errors or biases identified after deployment.
For instance, if an AI system consistently misdiagnoses a condition in a specific demographic group, there must be a clear process for identifying the source of the bias, rectifying the algorithm, and addressing any harm caused. The concept of explainable AI (XAI) becomes crucial here, allowing clinicians and regulators to understand the reasoning behind an AI’s decision, making it easier to identify and address potential biases.
Policy Proposal: Best Practices for Ethical AI in Healthcare, Hospital AI evaluation ai bias health affairs
This policy proposal Artikels best practices for the ethical development and implementation of AI in healthcare settings. It aims to foster responsible innovation while ensuring fairness, accountability, and patient safety.
Hospital AI evaluation is crucial, especially considering the potential for AI bias in healthcare. The implications of algorithmic fairness are huge, and the recent Supreme Court decision, as reported in this article scotus overturns chevron doctrine healthcare , could significantly impact regulatory oversight of these technologies. This means we need even more rigorous testing and transparency in how these AI systems are developed and deployed to prevent exacerbating existing health disparities.
Recommendation | Justification | Implementation Strategy | Potential Challenges |
---|---|---|---|
Mandate rigorous bias testing for all AI-based healthcare tools before deployment. | To ensure fairness and prevent discrimination against specific patient populations. | Establish standardized testing protocols and certification processes. Develop publicly accessible bias detection tools. | Defining and measuring bias can be complex. Lack of standardized datasets for testing. |
Require transparency in algorithmic design and decision-making processes. | To enable scrutiny and accountability. To foster trust in AI systems. | Develop guidelines for documenting algorithmic design choices and providing explanations for AI-generated outputs. | Balancing transparency with the need to protect intellectual property. |
Establish clear lines of responsibility for addressing errors or biases in AI systems. | To ensure accountability and prevent harm to patients. | Develop mechanisms for reporting and investigating incidents related to AI bias. | Determining liability in cases of AI-related errors. |
Invest in research and development of bias mitigation techniques. | To continuously improve the fairness and accuracy of AI systems. | Fund research on bias detection and mitigation, and support the development of tools and techniques for addressing bias. | The complexity of bias in AI systems. The need for interdisciplinary collaboration. |
Case Studies of AI Bias in Hospitals
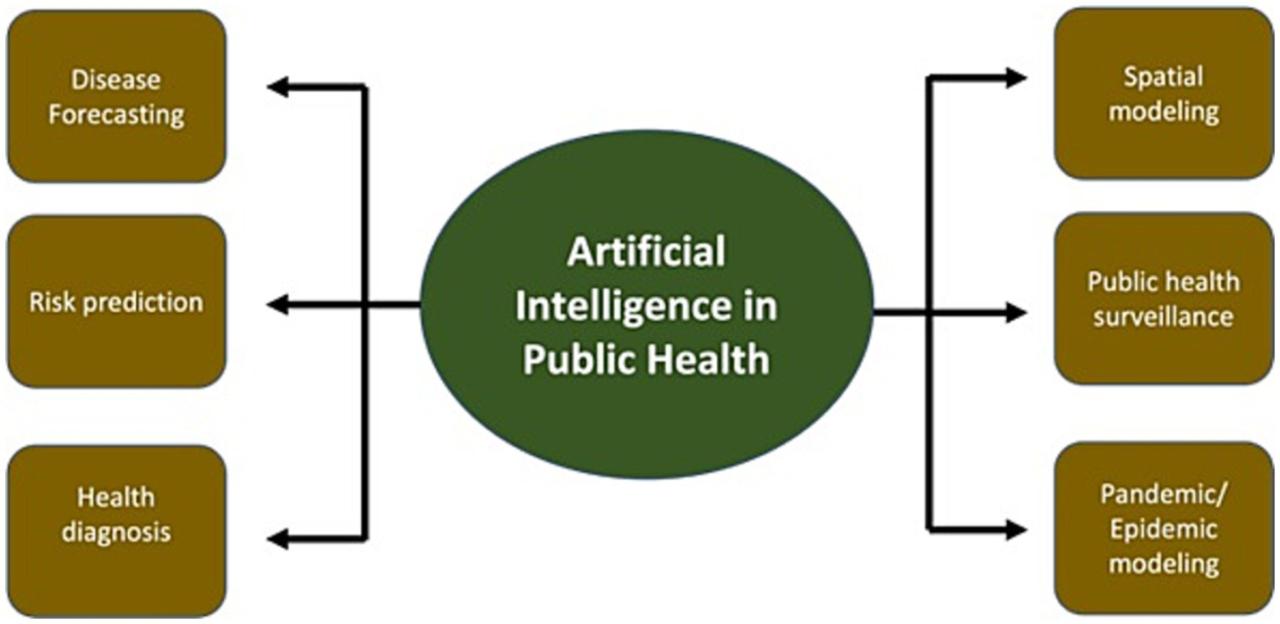
Source: frontiersin.org
AI bias in healthcare is no longer a hypothetical concern; it’s a tangible problem with real-world consequences. The deployment of AI systems in hospitals, while promising increased efficiency and improved patient outcomes, has revealed instances where algorithmic bias has led to disparities in care and potentially harmful results. Examining specific case studies allows us to understand the mechanisms of this bias and its impact.
AI Bias in Cardiovascular Risk Prediction
One area where AI bias has manifested significantly is in cardiovascular risk prediction. Several studies have shown that algorithms trained on datasets predominantly representing one demographic group (e.g., white, male) perform poorly when applied to other groups. This disparity can lead to misdiagnosis, delayed treatment, and ultimately, poorer health outcomes for underrepresented populations. For example, an algorithm trained primarily on data from Caucasian patients might underpredict the risk of heart disease in African American patients, even when presenting with similar symptoms.
This could lead to a delay or omission of crucial interventions.
“Our findings highlight the importance of carefully considering the representativeness of training data and the potential for algorithmic bias in cardiovascular risk prediction models.”
Excerpt from a hypothetical research paper on AI bias in cardiovascular risk prediction.
Hospital AI evaluation is crucial, especially considering the potential for AI bias in healthcare. The recent confirmation of Robert F. Kennedy Jr. as HHS Secretary, as reported by this article , will significantly impact how these evaluations are conducted and regulated. His stance on health policy could reshape the future of AI bias mitigation within the hospital system, influencing everything from algorithm design to data collection practices.
Algorithmic Bias in Diagnostic Imaging
AI is increasingly used in diagnostic imaging, such as analyzing X-rays and CT scans. However, biases in the training data can lead to inaccurate diagnoses for certain patient groups. For instance, an algorithm trained primarily on images of patients with a specific skin tone might struggle to accurately identify anomalies in patients with different skin tones. This could result in missed diagnoses, delayed treatment, and potentially life-threatening consequences.
The lack of diversity in training data exacerbates this issue, leading to algorithms that are not robust enough to handle the diversity of the patient population.
“The algorithm showed significantly lower accuracy in detecting fractures in patients with darker skin tones compared to lighter skin tones, highlighting the need for more diverse and representative datasets in the training of AI diagnostic tools.”
Hospital AI evaluations are crucial, but concerns about AI bias in healthcare are rightfully prominent. Accurate diagnosis is paramount, and a recent study explores whether a simple eye test can help predict dementia risk in older adults, as discussed in this insightful article: can eye test detect dementia risk in older adults. This highlights the need for unbiased AI tools in hospitals, as early detection methods like this could significantly improve patient outcomes and inform the development of fairer AI systems.
Excerpt from a hypothetical news article reporting on AI bias in diagnostic imaging.
Bias in Patient Triage and Resource Allocation
AI systems are also being used to assist in patient triage and resource allocation within hospitals. However, biases in these systems can lead to unequal access to care. For instance, an algorithm designed to prioritize patients based on severity of illness might inadvertently prioritize patients from certain socioeconomic backgrounds or with specific demographic characteristics, potentially neglecting patients in need of urgent care.
This could exacerbate existing health inequalities and further marginalize vulnerable populations. The design and implementation of these systems need to be carefully scrutinized to ensure equitable access to care for all patients.
“Our analysis revealed that the AI-driven triage system disproportionately prioritized patients from higher socioeconomic groups, leading to longer wait times and potentially worse outcomes for patients from lower socioeconomic backgrounds.”
Excerpt from a hypothetical study on AI bias in hospital resource allocation.
Future Directions for Fair and Equitable AI in Healthcare
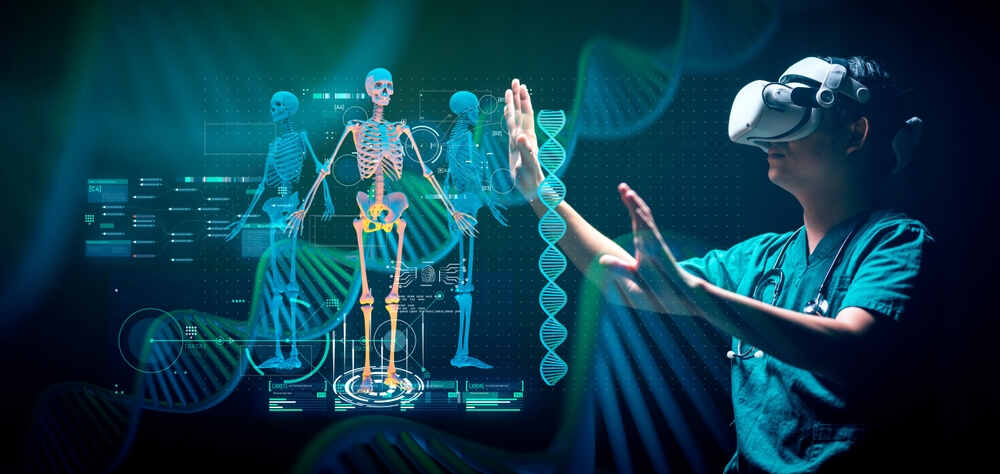
Source: thinkml.ai
The ethical deployment of AI in healthcare requires a proactive approach to mitigate bias and ensure equitable access to beneficial technologies. Moving forward, technological advancements, improved transparency, and robust policy changes are crucial for realizing the full potential of AI while minimizing harm. This necessitates a concerted effort from researchers, developers, clinicians, and policymakers alike.Technological Advancements to Reduce Bias in AI-Powered Healthcare SystemsThe development of more robust and unbiased AI models hinges on several key technological advancements.
Firstly, improved data collection methods are vital. This includes actively seeking diverse and representative datasets that accurately reflect the patient population, addressing historical biases embedded in existing healthcare data. Secondly, algorithmic advancements are needed to develop more sophisticated bias detection and mitigation techniques. This includes incorporating fairness-aware algorithms that explicitly consider and correct for potential biases during model training and deployment.
Thirdly, the use of federated learning allows multiple institutions to collaboratively train AI models on decentralized datasets, mitigating the risk of bias introduced by single-source data. Finally, continuous monitoring and auditing of AI systems in real-world settings are essential to identify and address emerging biases over time. For example, a hospital system using AI for risk stratification might find that its model disproportionately flags certain demographic groups for higher risk, necessitating a review of the data and algorithms used.
Explainable AI (XAI) and Transparency in AI-Driven Medical Decision-Making
Explainable AI (XAI) is paramount to building trust and ensuring accountability in AI-driven medical decision-making. XAI techniques provide insights into the reasoning behind AI’s predictions, allowing clinicians to understand why a particular diagnosis or treatment recommendation was made. This transparency fosters trust between patients and healthcare providers, empowers clinicians to critically evaluate AI’s recommendations, and facilitates the identification and correction of errors or biases.
For instance, an XAI system might not only predict a patient’s likelihood of developing a certain condition but also explain which factors contributed most significantly to that prediction, allowing for a more nuanced and informed clinical judgment. The lack of transparency in “black box” AI systems hinders the adoption and trust in AI-powered healthcare solutions, while XAI promotes responsible innovation.
Visual Representation of the Future Landscape of Fair and Equitable AI in Healthcare
Imagine a vibrant landscape. At its center stands a robust, interconnected network representing diverse healthcare institutions. Data streams, depicted as multicolored rivers, flow into this central network from various sources, each stream representing a different demographic group. These data streams are carefully filtered and processed through advanced algorithms (represented as shimmering, multifaceted crystals) designed to detect and mitigate bias.
The resulting insights flow out from the central network as clear, transparent streams, nourishing individual healthcare systems. Surrounding this core are strong regulatory bodies (represented as sturdy, well-defined mountains) ensuring ethical guidelines and accountability are upheld. This landscape is further enhanced by a bright sun symbolizing continuous research and development in XAI and bias mitigation technologies. Finally, bridges connect this central network to communities, highlighting equitable access to AI-powered healthcare services for all demographics.
This visual illustrates a future where AI is not only powerful but also fair, transparent, and accessible to all.
Epilogue
Ultimately, addressing AI bias in healthcare requires a multifaceted approach. It demands a commitment from developers, healthcare providers, regulators, and policymakers alike. By understanding the nuances of algorithmic bias, implementing robust evaluation strategies, and advocating for ethical guidelines, we can harness the transformative potential of AI while safeguarding against its inherent risks. The journey towards fair and equitable AI in healthcare is ongoing, but with diligent effort and collaboration, we can build a system that truly serves all patients.
FAQ Corner
What are the long-term consequences of unchecked AI bias in hospitals?
Unmitigated bias can lead to worsening health disparities, erosion of public trust in AI-driven healthcare, and potentially even legal repercussions for hospitals and developers.
How can patients advocate for themselves in the face of potentially biased AI systems?
Patients should be aware of their rights, actively seek second opinions, and report any concerns about potentially biased diagnoses or treatment recommendations to hospital administration and regulatory bodies.
What role do insurance companies play in addressing AI bias?
Insurers have a vested interest in ensuring fair and accurate AI-driven diagnoses and treatments, as biased systems could lead to increased costs and inefficient resource allocation. Their involvement in data collection and algorithm auditing is crucial.