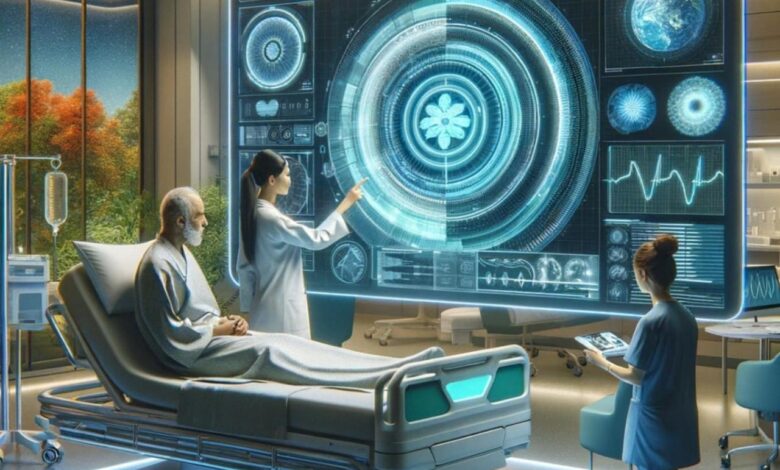
In Healthcare Build Trust Through AI Innovation
In healthcare build trust through innovation with generative ai – In healthcare, building trust through innovation with generative AI is no longer a futuristic fantasy; it’s rapidly becoming a reality. Imagine a world where AI personalizes patient experiences, making healthcare more accessible and understandable. This isn’t just about slick technology; it’s about fostering genuine connection and confidence between patients and providers. We’ll explore how generative AI is revolutionizing healthcare, from streamlining administrative tasks to accelerating drug discovery, all while addressing crucial ethical considerations and prioritizing data security.
Get ready to dive into a fascinating journey where technology meets compassion.
This post will delve into the practical applications of generative AI in healthcare, examining its potential to improve patient care, enhance efficiency, and ultimately, build stronger, more trusting relationships between patients and the healthcare system. We’ll look at real-world examples, explore potential challenges, and discuss the importance of responsible AI implementation. The goal? To paint a clear picture of how AI can be a powerful tool for good in healthcare, transforming the way we deliver and experience care.
The Role of Generative AI in Enhancing Patient Trust
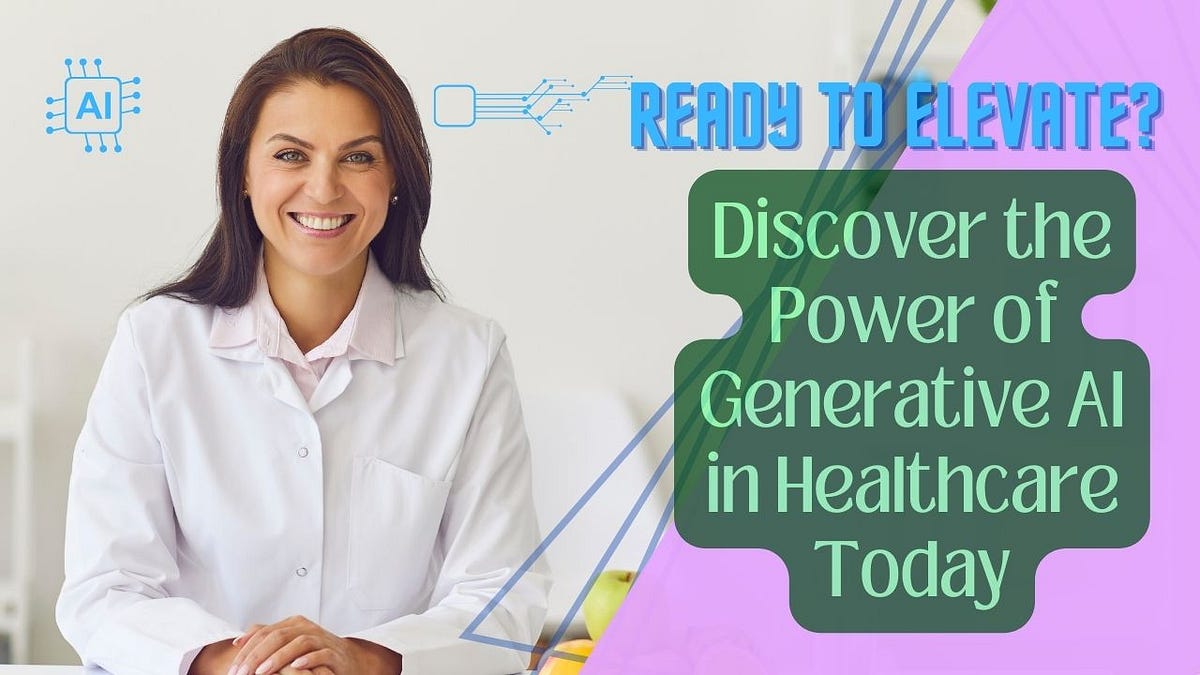
Source: medium.com
Generative AI holds immense potential to revolutionize healthcare by fostering trust between patients and providers. Its ability to personalize experiences, improve communication, and simplify complex medical information can significantly enhance patient satisfaction and confidence in their care. This ultimately leads to better health outcomes and a more positive overall healthcare experience.
Personalized Patient Experiences Through Generative AI
Generative AI can create highly personalized patient experiences by tailoring information and communication to individual needs and preferences. Imagine a system that automatically generates appointment reminders in a patient’s preferred language, adapts the complexity of medical explanations based on their health literacy level, and even provides personalized wellness plans based on their lifestyle and health history. This level of personalization demonstrates care and consideration, fostering trust and improving adherence to treatment plans.
Building trust in healthcare through AI innovation means showing tangible results. A key step towards this is improving primary care access, and the recent news that the cms launches primary care medicare model aco is a significant move in that direction. This initiative, coupled with responsible AI implementation, can foster greater patient confidence and ultimately strengthen the healthcare system’s overall trustworthiness.
Improving Communication and Transparency with AI-Powered Tools
AI-powered chatbots and virtual assistants can significantly improve communication and transparency. These tools can answer frequently asked questions, provide updates on test results, and schedule appointments, freeing up healthcare professionals to focus on more complex tasks. The immediate and readily available information reduces patient anxiety associated with waiting for responses and enhances transparency in the care process. Furthermore, AI can analyze patient data to identify potential communication barriers and suggest strategies for improved interaction, leading to more effective and empathetic communication.
Creating Easily Understandable Medical Information
Generative AI can transform complex medical jargon into easily digestible information for patients. AI algorithms can simplify medical reports, treatment plans, and educational materials, making them accessible to individuals with varying levels of health literacy. This ensures patients understand their conditions, treatments, and options, empowering them to actively participate in their healthcare decisions and strengthening their trust in the medical professionals involved.
For example, AI could generate personalized summaries of medical reports, using plain language and visuals to explain complex information.
Hypothetical Scenario: AI Improving Patient Trust in Oncology
Consider a patient, Sarah, diagnosed with breast cancer. Traditionally, receiving a cancer diagnosis involves overwhelming amounts of information, complex medical terminology, and lengthy waiting periods for appointments and test results. This often leads to anxiety, confusion, and a lack of trust in the healthcare system.However, with AI, Sarah’s experience could be vastly different. An AI-powered chatbot could immediately provide her with clear, concise information about her diagnosis in plain language, answering her initial questions and alleviating immediate anxiety.
The system could also schedule follow-up appointments, send reminders, and provide personalized resources tailored to her specific situation and emotional needs. Regular updates on test results would be delivered promptly via the chatbot, maintaining transparency and reducing uncertainty. Furthermore, the AI could generate personalized treatment plans and educational materials, ensuring Sarah fully understands her options and feels empowered to make informed decisions.
Method | Description | Traditional Approach | AI-Enhanced Approach |
---|---|---|---|
Appointment Scheduling | Scheduling appointments with healthcare providers | Phone calls, waiting on hold, potential scheduling conflicts | AI-powered scheduling system, immediate availability, personalized reminders |
Information Access | Accessing medical information and test results | Long wait times for results, difficulty understanding medical jargon | Instant access to test results and personalized summaries in plain language |
Communication | Communication with healthcare providers | Limited access to providers, potential communication barriers | AI-powered chatbot for immediate answers, improved communication with providers |
Education | Understanding medical conditions and treatment options | Complex medical terminology, limited resources | Personalized educational materials in plain language, tailored to individual needs |
Improving Healthcare Efficiency and Outcomes Through AI Innovation
Generative AI is poised to revolutionize healthcare, not just by improving patient trust, but also by dramatically enhancing efficiency and ultimately, patient outcomes. Its ability to process vast amounts of data and generate insightful predictions offers unprecedented opportunities to streamline workflows, accelerate diagnoses, and accelerate drug discovery. This leads to better resource allocation, faster treatment, and ultimately, improved health for individuals and populations.
The integration of generative AI across various healthcare sectors promises a significant shift towards a more efficient and effective system. This efficiency boost isn’t just about speed; it’s about freeing up valuable human resources – our healthcare professionals – to focus on what truly matters: providing compassionate and personalized patient care.
Streamlining Administrative Tasks with Generative AI
Generative AI can automate numerous administrative tasks, reducing the burden on healthcare staff. Imagine AI handling appointment scheduling, generating personalized patient summaries, processing insurance claims, and even drafting routine correspondence. This automation frees up doctors, nurses, and administrative staff to dedicate more time to direct patient interaction and complex medical tasks. For example, an AI-powered system could analyze patient records to identify individuals at risk of readmission, allowing proactive interventions to prevent hospital readmissions, saving time and resources while improving patient care.
This frees up valuable time for healthcare professionals to focus on direct patient care.
AI-Driven Diagnostic Tools: Improved Accuracy and Speed
Generative AI is rapidly transforming diagnostic capabilities. AI algorithms can analyze medical images (X-rays, CT scans, MRIs) with remarkable accuracy and speed, often exceeding human capabilities in detecting subtle anomalies. For instance, an AI system trained on thousands of chest X-rays can identify signs of pneumonia or lung cancer with greater precision and speed than a human radiologist, leading to earlier diagnosis and treatment, improving patient outcomes and building confidence in the accuracy of medical assessments.
Early and accurate diagnosis is crucial in many life-threatening conditions, increasing patient survival rates and improving their overall health.
Generative AI in Drug Discovery and Development
The pharmaceutical industry is embracing generative AI to accelerate drug discovery and development. AI can analyze massive datasets of molecular structures and biological information to identify potential drug candidates, predict their efficacy, and optimize their design. This dramatically reduces the time and cost associated with traditional drug development, leading to faster access to life-saving medications. For example, generative AI has been used to identify novel compounds with potential antiviral activity, significantly accelerating the development of treatments for infectious diseases.
The potential to quickly develop effective treatments for emerging diseases or previously incurable conditions offers substantial benefits to patients and society.
Ethical Considerations and Benefits of AI in Healthcare
The integration of AI in healthcare presents both ethical considerations and substantial benefits. Careful consideration of these aspects is crucial to building trust and ensuring responsible implementation.
It is essential to weigh the potential benefits against the ethical considerations to ensure responsible and trustworthy implementation of AI in healthcare.
- Ethical Considerations:
- Data privacy and security: Protecting sensitive patient information is paramount.
- Algorithmic bias: Ensuring fairness and avoiding discriminatory outcomes is critical.
- Transparency and explainability: Understanding how AI algorithms arrive at their conclusions is crucial for building trust.
- Responsibility and accountability: Determining liability in case of errors or adverse events.
- Benefits:
- Improved accuracy and speed of diagnosis, leading to better patient outcomes.
- Increased efficiency and reduced costs in healthcare delivery.
- Accelerated drug discovery and development, resulting in faster access to life-saving treatments.
- Enhanced patient experience through personalized care and improved communication.
Addressing Data Privacy and Security Concerns in AI-Driven Healthcare
The integration of generative AI into healthcare promises significant advancements, but it also introduces considerable challenges related to data privacy and security. Maintaining patient trust is paramount, and this requires a proactive and robust approach to safeguarding sensitive health information. Failing to address these concerns could severely undermine the adoption and effectiveness of AI in healthcare.
Generative AI models, by their nature, require vast amounts of data for training and operation. This data often includes highly sensitive Protected Health Information (PHI), necessitating stringent security measures to prevent breaches and misuse. The potential for unauthorized access, data leakage, or even manipulation of AI-generated outputs poses significant risks. Addressing these concerns requires a multi-faceted strategy encompassing technical, legal, and ethical considerations.
Data Minimization and Anonymization Techniques
Implementing data minimization and anonymization strategies is crucial. Data minimization involves collecting and processing only the minimum necessary data for the intended purpose. Anonymization techniques, such as differential privacy and data masking, help remove or obscure identifying information from datasets used to train and operate AI models. For example, instead of using a patient’s full name, a unique identifier could be used, and sensitive details like addresses could be generalized to a broader geographic area.
Building trust in healthcare through AI innovation is crucial, especially given recent challenges. News of Steward Health Care’s Ohio hospital closures and the potential closure of a Pennsylvania facility, as reported in this article steward ohio hospitals closures pennsylvania facility at risk , highlights the need for transparency and efficiency. Generative AI can help improve both, fostering trust by optimizing resource allocation and improving patient care.
These techniques significantly reduce the risk of re-identification and subsequent privacy violations.
Secure Data Storage and Access Control
Robust security measures are needed to protect sensitive data throughout its lifecycle. This includes employing encryption techniques both in transit and at rest, implementing strict access control mechanisms to limit access to authorized personnel only, and regularly auditing systems for vulnerabilities. Employing multi-factor authentication and intrusion detection systems can further enhance security. Consider a hypothetical scenario where a hospital uses a cloud-based AI system for diagnostic support.
Strict access controls would ensure that only qualified medical professionals with appropriate credentials can access the system and patient data.
AI System Vulnerability Mitigation
AI systems themselves can be vulnerable to attacks such as adversarial attacks, where malicious actors introduce subtle changes to input data to manipulate the AI’s output. Robust model validation and testing procedures are crucial to identify and mitigate such vulnerabilities. Regular security assessments and penetration testing can help identify weaknesses in the AI system and its infrastructure. For example, a model trained to detect cancerous cells might be susceptible to an adversarial attack that could cause it to misclassify benign cells as cancerous, leading to unnecessary treatment.
Employing techniques like adversarial training can help enhance the robustness of AI models against such attacks.
Building trust in healthcare through AI innovation is crucial, especially with the potential of generative AI to revolutionize patient care. The recent confirmation of Robert F. Kennedy Jr. as HHS Secretary, as reported by this article , will undoubtedly impact this trajectory. His leadership will shape how we integrate these advancements, and careful consideration of public trust will be key to successful implementation of AI in healthcare.
Hypothetical Policy for Responsible Data Handling in AI-Powered Healthcare
This policy Artikels best practices for responsible data handling in AI-powered healthcare applications:
1. Data Minimization and Anonymization: Only collect and process the minimum necessary data, employing appropriate anonymization techniques whenever possible.
2. Secure Data Storage and Access Control: Implement robust security measures, including encryption, access controls, and regular security audits.
3. Transparency and Accountability: Maintain clear documentation of data usage and AI model development, ensuring transparency and accountability.
4. Data Governance and Compliance: Establish a comprehensive data governance framework to ensure compliance with relevant regulations and ethical guidelines.
5. Continuous Monitoring and Improvement: Implement continuous monitoring of AI systems and data security practices, making improvements based on findings.
Key Regulations and Guidelines Related to Data Privacy in AI Healthcare, In healthcare build trust through innovation with generative ai
Several regulations and guidelines govern the use of personal data in healthcare, particularly in the context of AI. Understanding and adhering to these is crucial for maintaining patient trust and avoiding legal repercussions. These include:
The Health Insurance Portability and Accountability Act (HIPAA) in the United States, the General Data Protection Regulation (GDPR) in the European Union, and similar data protection laws in other countries. These regulations set forth stringent requirements for the handling of PHI, including consent, data security, and breach notification. Adherence to these regulations is non-negotiable for any organization deploying AI-powered healthcare applications.
The Future of AI and Trust in Healthcare: In Healthcare Build Trust Through Innovation With Generative Ai
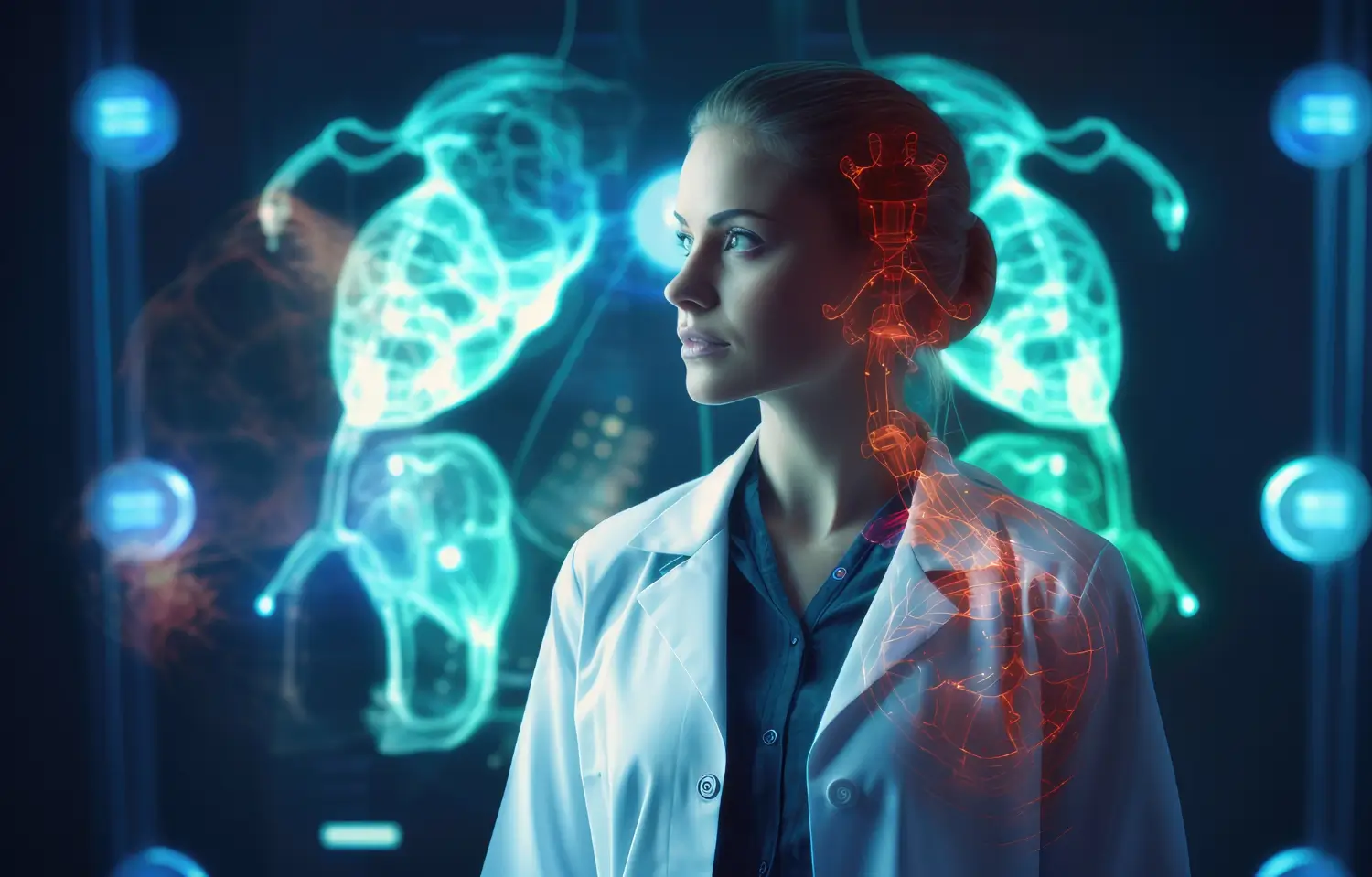
Source: scopicsoftware.com
The integration of artificial intelligence, particularly generative AI, into healthcare holds immense promise, but its successful implementation hinges on addressing critical challenges to ensure patient trust and equitable access. Building this trust requires proactive measures to mitigate biases, continuously monitor AI systems, and explore responsible applications that demonstrably improve patient care. Failure to do so risks exacerbating existing health disparities and undermining the potential benefits of this transformative technology.Addressing biases in AI algorithms is paramount for building trust and ensuring equitable access to healthcare.
AI models are trained on data, and if that data reflects existing societal biases (e.g., racial, socioeconomic), the AI system will perpetuate and even amplify those biases in its diagnoses, treatment recommendations, and resource allocation. For example, an AI system trained on data primarily from one demographic group might misdiagnose or undertreat patients from other groups. To mitigate this, rigorous data auditing and bias detection techniques are crucial.
Furthermore, diverse and representative datasets are needed for training, along with algorithmic fairness techniques to ensure unbiased outcomes. Transparency in the development and deployment of these algorithms is also essential to foster trust and accountability.
Bias Mitigation in AI Algorithms
Addressing bias requires a multi-pronged approach. Firstly, careful curation of training datasets is crucial. This involves actively seeking and incorporating data from diverse populations to ensure fair representation. Secondly, algorithmic fairness techniques need to be integrated during the model development phase. These techniques can include methods like re-weighting data points to counteract biases, or using fairness-aware optimization algorithms.
Finally, continuous monitoring and evaluation of the AI system’s performance across different demographic groups is vital to identify and correct any emerging biases. This involves regular audits and independent reviews to ensure the AI is performing equitably and not disproportionately impacting certain patient populations.
Continuous Monitoring and Evaluation of AI Systems
Continuous monitoring and evaluation are essential for maintaining patient trust and safety. AI systems, like any complex technology, can experience errors or drift over time. Regular performance checks, including both technical audits and clinical reviews, are necessary to identify and address any issues. This should include tracking key performance indicators (KPIs) such as accuracy, precision, and recall, as well as monitoring for unexpected or harmful outputs.
Furthermore, mechanisms for feedback and reporting from healthcare professionals and patients are crucial for identifying potential problems and improving the AI system’s reliability and safety. For instance, a system flagging a high-risk patient might be reviewed by a clinician to assess the accuracy of the alert, while a patient reporting an unsatisfactory experience can lead to system improvements.
Future Applications of Generative AI in Healthcare
Generative AI offers exciting possibilities for enhancing trust and improving patient outcomes. For example, personalized medicine could be revolutionized through AI-generated treatment plans tailored to individual patient characteristics and genetic profiles. AI could also generate more comprehensive and easily understandable patient education materials, improving adherence to treatment plans. Moreover, generative AI can assist in drug discovery and development by accelerating the identification of potential drug candidates and predicting their efficacy and safety.
Finally, AI-powered virtual assistants could provide patients with 24/7 access to reliable medical information and support, enhancing patient engagement and promoting proactive healthcare management. The successful implementation of these applications requires careful consideration of ethical implications and rigorous testing to ensure accuracy and safety.
The Interconnectedness of Innovation, Trust, and Patient Care
Imagine a three-dimensional model. At the center is a sphere representing “Patient Care,” symbolizing the core goal of healthcare. Radiating outwards are two interconnected spirals: one representing “Innovation” (depicting the advancements in AI and its applications) and the other representing “Trust” (depicting the necessary confidence in AI’s reliability and ethical implementation). The spirals intertwine and support each other, showing that innovation in AI is only beneficial if it fosters trust, and strong trust in AI enhances the effectiveness and impact of innovation in improving patient care.
The closer the spirals are to the “Patient Care” sphere, the more impactful the innovation and trust are in achieving positive patient outcomes. Breaks or weakening in either spiral would negatively impact the overall system and the delivery of patient care.
Outcome Summary
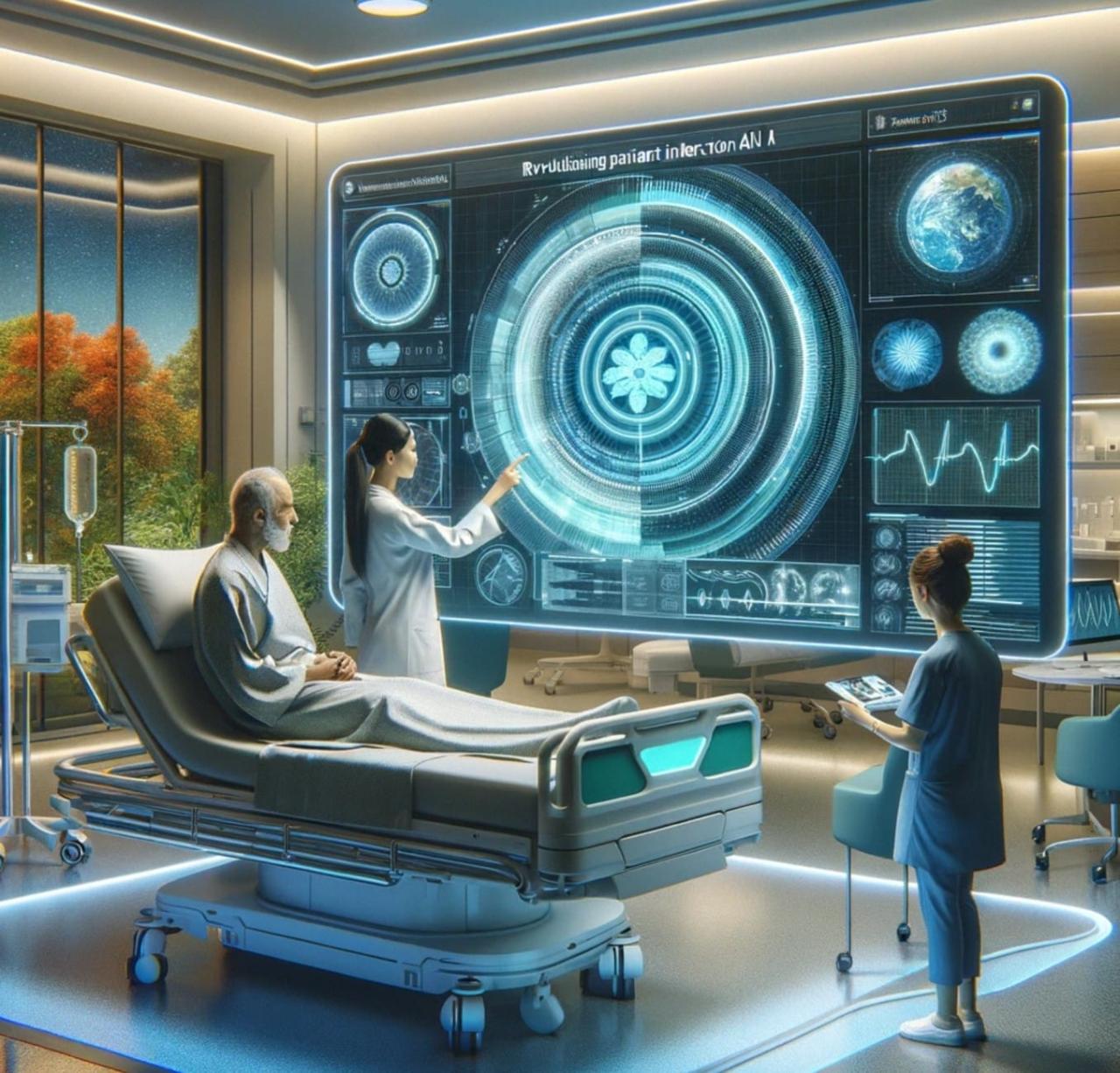
Source: infuy.com
The integration of generative AI in healthcare presents a remarkable opportunity to reshape the patient experience, improve efficiency, and foster unprecedented levels of trust. While challenges related to data security and ethical considerations exist, proactive measures and responsible implementation can mitigate these risks. The future of healthcare is undoubtedly intertwined with AI, promising a more personalized, efficient, and ultimately, more trustworthy system for everyone.
By embracing innovation responsibly, we can unlock the transformative potential of AI to create a healthier and more equitable future for all.
Popular Questions
What are the biggest risks associated with using AI in healthcare?
The biggest risks include data breaches, algorithmic bias leading to unfair treatment, and the potential for misdiagnosis due to errors in AI systems. Robust security measures, careful algorithm design, and thorough human oversight are crucial to mitigate these risks.
How can patients ensure their data is protected when AI is used in their care?
Patients should ask their healthcare providers about their data privacy policies, especially regarding AI usage. Look for providers who are transparent about their data security practices and comply with relevant regulations like HIPAA (in the US).
Will AI replace healthcare professionals?
No, AI is intended to augment, not replace, healthcare professionals. It will handle repetitive tasks, improve diagnostic accuracy, and provide support to clinicians, ultimately allowing them to focus more on patient interaction and complex cases.
How can we ensure AI in healthcare is equitable and accessible to everyone?
Addressing algorithmic bias is key. This requires diverse datasets for training AI models and ongoing monitoring to detect and correct any disparities in outcomes. Furthermore, ensuring affordable access to AI-powered healthcare tools is essential for equitable care.