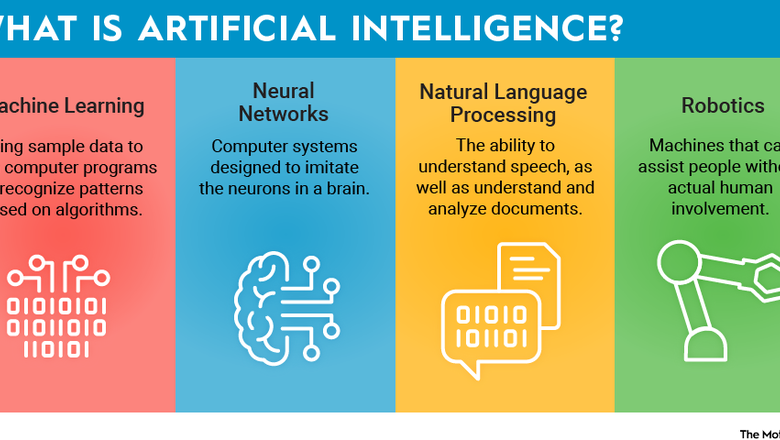
Mickey Tripathi, ONC, AI, EHRs Documentation Burden
Micky tripathi onc healthcare ai ehrs documentation burden – Mickey Tripathi, ONC, healthcare AI, and EHRs: the documentation burden. It’s a mouthful, isn’t it? But it’s also a huge issue facing healthcare providers today. We’re drowning in paperwork, and the promise of AI to lighten the load feels both exciting and maybe a little overwhelming. This post dives into Tripathi’s key role in pushing AI adoption within the ONC, exploring how AI-powered tools are tackling this problem, and considering the ethical implications we need to navigate along the way.
Get ready for a deep dive into the future of healthcare documentation!
This exploration will cover Tripathi’s specific contributions to the Office of the National Coordinator for Health IT (ONC) and his advocacy for using AI to reduce the administrative burden on healthcare providers. We’ll look at practical AI solutions like NLP and voice recognition, examining their potential benefits and limitations. We’ll also consider the ethical and regulatory aspects of implementing AI in EHRs, ensuring responsible innovation.
Mickey Tripathi’s Role in ONC Healthcare AI Initiatives: Micky Tripathi Onc Healthcare Ai Ehrs Documentation Burden
Mickey Tripathi, during his tenure as National Coordinator for Health Information Technology, played a pivotal role in shaping the Office of the National Coordinator for Health IT’s (ONC) approach to artificial intelligence (AI) in healthcare. His vision focused on leveraging AI to improve healthcare efficiency, quality, and patient outcomes while simultaneously addressing the significant challenges posed by the current electronic health record (EHR) system.
This involved a multifaceted strategy encompassing policy changes, technological advancements, and public awareness campaigns.Tripathi’s advocacy for AI’s role in mitigating the EHR documentation burden was a central theme of his leadership. He recognized that the excessive administrative burden placed on clinicians by EHRs was a major contributor to burnout and reduced the time available for direct patient care. He championed the use of AI-powered tools to automate administrative tasks, streamline workflows, and ultimately lighten the load on healthcare providers.
This involved actively promoting the development and adoption of AI solutions capable of intelligently processing medical information, reducing the need for manual data entry and improving the overall efficiency of EHR systems.
Tripathi’s Policy Initiatives Related to AI and EHRs
Tripathi’s contributions extended beyond mere advocacy. He actively championed several key policies and initiatives designed to foster the responsible and effective integration of AI into healthcare. These initiatives often focused on interoperability, data privacy, and ethical considerations. For instance, he pushed for the development of standardized APIs and data exchange formats to ensure seamless integration of AI tools with existing EHR systems.
This facilitated the sharing of data between different healthcare organizations, enabling the development of more robust and accurate AI models. Furthermore, he emphasized the importance of patient data privacy and security in the development and deployment of AI-powered healthcare applications. He advocated for robust security measures and transparent data governance frameworks to protect patient information and build trust in AI-driven healthcare solutions.
Specific examples of policies directly influenced by Tripathi are difficult to isolate and attribute solely to him due to the collaborative nature of ONC initiatives. However, his influence is evident in the ONC’s overall strategic direction toward AI adoption in healthcare.
Examples of Tripathi’s Public Statements on AI and EHR Interoperability
While precise transcripts of every public statement are not readily accessible in a centralized repository, Tripathi consistently emphasized the potential of AI to improve healthcare through numerous speeches, articles, and interviews. His public pronouncements consistently highlighted the need for interoperable EHR systems to support AI development. He often stressed that AI’s potential could only be fully realized if data could flow freely and securely between different systems.
This focus on interoperability underscored his belief that AI should not be a siloed technology but rather a component of a broader, integrated healthcare ecosystem. His public communications often emphasized the need for collaborative efforts among stakeholders – including clinicians, technology developers, and policymakers – to ensure the responsible and ethical development and deployment of AI in healthcare.
The overarching message consistently centered around the need for thoughtful, patient-centric AI implementation, rather than simply adopting technology for technology’s sake.
AI Solutions for EHR Documentation Burden Reduction
The increasing administrative burden on healthcare providers, largely driven by the complexities of Electronic Health Records (EHRs), is a significant concern. AI offers a powerful toolkit to alleviate this burden, improving efficiency and allowing clinicians to focus more on patient care. Several AI-powered solutions are emerging to streamline documentation processes, leading to better patient outcomes and increased job satisfaction for healthcare professionals.
AI-Powered Tools for EHR Documentation
The following table summarizes some key AI-powered tools designed to reduce the documentation burden. These tools leverage various AI techniques, from natural language processing to machine learning, to automate tasks and improve efficiency.
Tool Name | Functionality | Benefits | Limitations |
---|---|---|---|
Voice Recognition Software | Converts spoken dictation into text within the EHR. | Faster documentation, reduced typing time, improved workflow. | Accuracy can be affected by background noise or accents; requires robust training data for optimal performance. May require editing. |
Predictive Text and Auto-Completion | Suggests likely words or phrases based on the context of the documentation. | Faster typing, reduced errors, improved consistency. | Limited by the available vocabulary and understanding of medical terminology; may not always suggest the most appropriate phrase. |
Automated Coding Systems | Automatically assigns ICD and CPT codes based on the clinical documentation. | Reduces coding time, improves coding accuracy, minimizes billing errors. | Requires high-quality clinical documentation as input; may not always capture the nuances of complex cases; ongoing monitoring and human oversight are needed. |
Natural Language Processing (NLP) for Clinical Note Summarization | Extracts key information from lengthy clinical notes, generating concise summaries. | Improved efficiency in reviewing patient records, easier identification of key findings. | Requires sophisticated NLP algorithms; accuracy can vary depending on the complexity of the notes and the quality of the training data. May require human review. |
Natural Language Processing (NLP) in EHR Documentation Automation
NLP plays a crucial role in automating EHR documentation. It allows computers to understand, interpret, and generate human language, enabling applications such as automated clinical note generation, summarization, and coding. For example, NLP algorithms can analyze unstructured clinical notes to extract key information like diagnoses, medications, and allergies, automatically populating structured fields within the EHR. This significantly reduces the manual effort required for data entry and improves data consistency.
Furthermore, NLP can power systems that flag potential inconsistencies or omissions in documentation, leading to more complete and accurate records.
Comparison of AI Approaches to Clinical Documentation Improvement
Predictive text offers a relatively simple approach, focusing on accelerating typing speed and reducing errors. Voice recognition provides a more significant leap forward by eliminating typing entirely, but requires high-quality audio and robust speech-to-text capabilities. Automated coding leverages NLP and machine learning to analyze clinical notes and assign appropriate codes, greatly reducing the workload on coding staff. However, each approach has limitations.
Micky Tripathi’s work on using AI to lighten the EHR documentation burden in healthcare is fascinating. Thinking about the complexities of managing patient data, it made me think about the challenges faced by families, like dealing with the complexities of a condition such as Tourette Syndrome. Learning about effective strategies, like those outlined in this helpful article on strategies to manage Tourette syndrome in children , highlights how crucial comprehensive care plans are, mirroring the need for efficient systems in healthcare, which is what Tripathi’s AI work aims to improve.
Ultimately, both focus on streamlining processes for better outcomes.
Predictive text may not always suggest the most appropriate term. Voice recognition can struggle with noisy environments or unusual accents. Automated coding requires careful validation to ensure accuracy and compliance with coding guidelines.
Hypothetical AI-Driven Patient Intake and Documentation System
A hypothetical AI-driven system could streamline patient intake by utilizing NLP to process patient questionnaires and intake forms. The system could extract key demographic information, medical history, and current symptoms, automatically populating the EHR. Voice recognition could be integrated to allow patients to provide their information verbally, potentially reducing literacy barriers. The system could then use this information to pre-populate relevant sections of the clinical note, using predictive text to suggest appropriate terms and phrases.
Finally, the system could leverage automated coding to assign initial codes based on the collected information. Such a system would require robust security measures to protect patient data and rigorous testing to ensure accuracy and reliability. This system would be designed to reduce the administrative burden on both patients and clinicians. Human oversight would remain crucial for complex cases or situations requiring clinical judgment.
Impact of AI on EHR Usability and Workflow
The integration of artificial intelligence (AI) into Electronic Health Records (EHRs) promises a significant transformation of healthcare workflows, impacting everything from clinician usability to patient interaction. While challenges exist, the potential benefits are substantial, offering the possibility of a more efficient, accurate, and ultimately, more patient-centered system.AI’s impact on EHR usability and workflow is multifaceted, encompassing both improvements and potential drawbacks.
Understanding these aspects is crucial for successfully implementing AI-driven solutions and maximizing their positive impact on healthcare delivery.
AI-Enhanced EHR Usability for Clinicians
AI can significantly improve EHR usability for clinicians by automating time-consuming tasks. For example, AI-powered tools can automatically extract relevant information from patient notes, reducing the time spent on manual data entry and chart review. Natural language processing (NLP) allows for voice-to-text transcription, enabling clinicians to document patient encounters more efficiently without interrupting the flow of the conversation.
Furthermore, AI can personalize the EHR interface, presenting clinicians with only the most relevant information based on the patient’s condition and the context of the encounter. This reduces cognitive overload and allows clinicians to focus on patient care. Imagine a system that automatically highlights critical lab results or flags potential drug interactions, proactively alerting clinicians to potentially dangerous situations.
Challenges in Integrating AI into EHR Workflows
Despite the potential benefits, integrating AI into EHR workflows presents several challenges. One significant hurdle is data quality. AI algorithms require large, high-quality datasets to function effectively. Inconsistent or incomplete data can lead to inaccurate predictions and unreliable results. Another challenge is the need for robust data security and privacy measures.
AI systems process sensitive patient information, and stringent safeguards are necessary to prevent breaches and maintain patient confidentiality. Furthermore, clinicians need adequate training and support to effectively use AI-powered tools. Resistance to change and a lack of understanding can hinder the successful adoption of new technologies. Finally, the high cost of developing, implementing, and maintaining AI systems can be a barrier for smaller healthcare organizations.
AI’s Influence on Clinician-Patient Interaction
AI can improve clinician-patient interaction by freeing up clinicians’ time to focus on patient engagement. By automating administrative tasks, AI allows clinicians to spend more time building rapport with patients, listening to their concerns, and providing personalized care. AI-powered chatbots can handle routine inquiries, providing patients with quick access to information and reducing the burden on clinical staff. However, it is crucial to ensure that AI tools enhance, not replace, human interaction.
The use of AI should always be transparent to the patient, and clinicians should remain responsible for the overall patient care experience. The goal is to use AI to augment, not substitute, the human element in the doctor-patient relationship.
AI’s Role in Improving EHR Data Quality and Reducing Errors
AI-powered tools can significantly improve data quality and reduce errors in EHR documentation. AI algorithms can identify inconsistencies and missing information in patient records, prompting clinicians to clarify or complete data entries. Real-time alerts can flag potential errors, such as medication discrepancies or conflicting diagnoses. Furthermore, AI can help standardize data entry, ensuring consistency across different healthcare providers and facilities.
This improved data quality enhances the accuracy of clinical decision-making, improves patient safety, and facilitates more effective population health management. For example, an AI system could detect a potential medication allergy based on previous records and alert the clinician before a prescription is written, preventing a potentially serious adverse event.
Ethical and Regulatory Considerations of AI in EHRs
The integration of artificial intelligence (AI) into Electronic Health Records (EHRs) offers transformative potential for healthcare, promising improved efficiency and patient care. However, this progress necessitates careful consideration of the ethical and regulatory landscape. Failing to address these concerns could lead to unintended consequences, undermining trust and hindering the responsible adoption of this powerful technology.
Data Privacy and Bias in AI-driven Clinical Documentation
AI algorithms rely heavily on data for training and operation. The use of patient data in AI for clinical documentation raises significant privacy concerns. Protecting patient health information (PHI) is paramount, and AI systems must adhere to stringent data privacy regulations like HIPAA in the United States and GDPR in Europe. Furthermore, biases present in the training data can lead to AI systems perpetuating and even amplifying existing healthcare disparities.
For example, an algorithm trained on data primarily from one demographic group might misdiagnose or provide suboptimal treatment recommendations for patients from underrepresented groups. Mitigating bias requires careful data curation, algorithmic transparency, and ongoing monitoring for fairness and equity.
Existing Regulations and Guidelines for AI in Healthcare
The regulatory landscape for AI in healthcare is still evolving, but several key guidelines and regulations are emerging. The FDA, for instance, is increasingly involved in regulating AI-based medical devices, including those integrated into EHRs. These regulations often focus on ensuring the safety and effectiveness of the AI system before it can be deployed clinically. Other organizations, such as the National Institute of Standards and Technology (NIST), are developing frameworks and guidelines for trustworthy AI, emphasizing aspects like transparency, explainability, and robustness.
Mickey Tripathi’s work highlighting the EHR documentation burden in healthcare is crucial, especially considering the physical toll on clinicians. The constant typing and clicking can lead to repetitive strain injuries, like carpal tunnel syndrome, which, thankfully, often has non-surgical treatment options. Check out this helpful resource on ways to treat carpal tunnel syndrome without surgery to learn more about preventing this.
Ultimately, reducing this burden through AI solutions, as advocated by Tripathi, is key to both improving clinician well-being and patient care.
Compliance with these regulations and guidelines is crucial for responsible AI implementation in healthcare.
Potential Risks of Relying on AI for Medical Decision-Making
The reliance on AI for medical decision-making based on EHR data presents several potential risks:
- Inaccurate diagnoses or treatment recommendations: AI algorithms are only as good as the data they are trained on. Inaccurate or incomplete data can lead to erroneous conclusions, potentially harming patients.
- Lack of transparency and explainability: Some AI algorithms, particularly deep learning models, are “black boxes,” making it difficult to understand how they arrive at their conclusions. This lack of transparency can hinder clinical trust and make it challenging to identify and correct errors.
- Data security breaches: AI systems often require access to sensitive patient data, making them potential targets for cyberattacks. Breaches could lead to the exposure of PHI and compromise patient privacy.
- Algorithmic bias perpetuating health disparities: As mentioned earlier, biases in training data can lead to AI systems discriminating against certain patient populations.
- Over-reliance on AI and diminished clinical judgment: Clinicians must maintain critical thinking and clinical judgment, even when using AI-powered tools. Over-reliance on AI could lead to missed diagnoses or inappropriate treatment decisions.
The Importance of Human Oversight in AI-powered EHR Systems
Human oversight is crucial for the successful and ethical implementation of AI-powered EHR systems. Clinicians must retain ultimate responsibility for patient care, using AI as a supportive tool rather than a replacement for their expertise. This requires ongoing monitoring of AI performance, regular audits to detect and correct biases, and mechanisms for human intervention when necessary. Furthermore, robust training programs are needed to equip healthcare professionals with the knowledge and skills to effectively utilize and interpret AI-generated insights.
A collaborative approach, where AI augments human capabilities, is essential to maximizing the benefits of this technology while minimizing the risks.
Future Directions for AI and EHRs
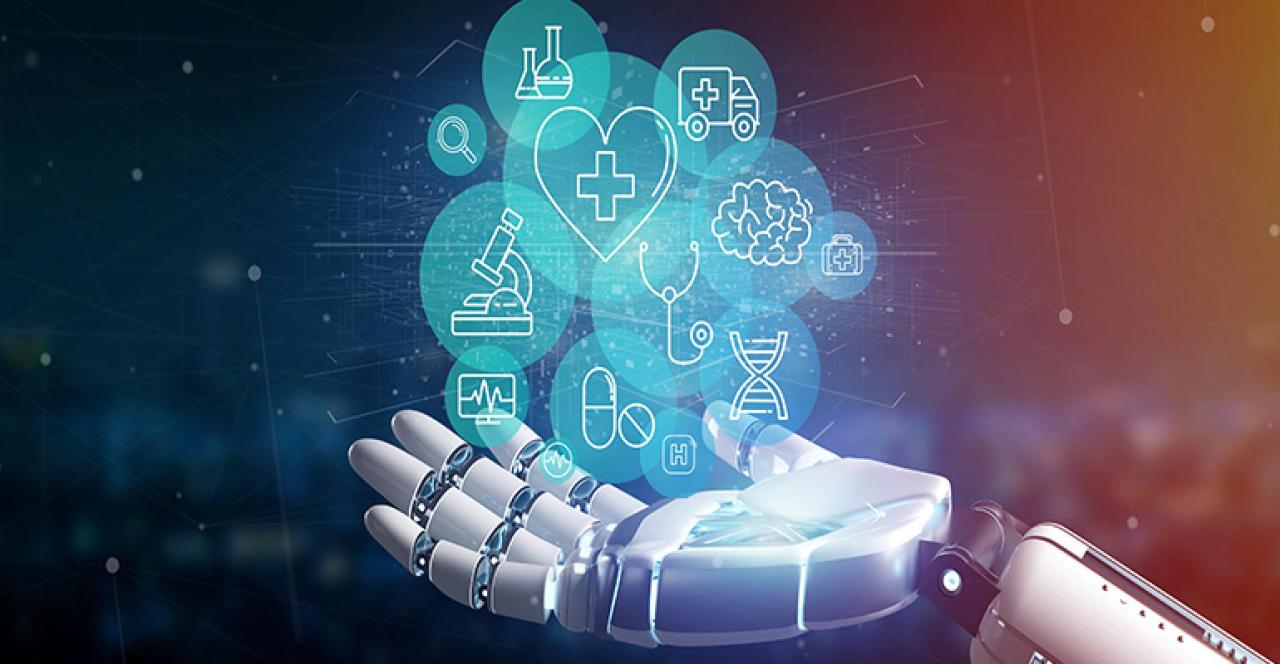
Source: techhong.com
The integration of artificial intelligence (AI) into electronic health records (EHRs) is still in its nascent stages, but the potential for revolutionizing healthcare is immense. We’ve already seen AI tackle some of the most pressing challenges, like reducing documentation burden. However, the future holds even more transformative possibilities, promising a more efficient, accurate, and patient-centric healthcare system.
Micky Tripathi’s work on using AI in healthcare to tackle EHR documentation burdens is fascinating. It made me think about how even seemingly simple things, like diet, impact our overall health and well-being. This led me to an interesting article discussing nutritional differences between the sexes: are women and men receptive of different types of food and game changing superfoods for women.
Understanding these differences could inform better patient care, which ties back to Tripathi’s efforts to streamline healthcare documentation and improve patient outcomes.
The next decade will likely witness a dramatic shift in how AI interacts with EHRs, moving beyond simple automation to truly intelligent systems that anticipate needs and proactively improve care. This evolution will hinge on advancements in technology, a focus on interoperability, and a commitment to ethical considerations.
Advancements in AI Technology for EHR Documentation
Further reductions in EHR documentation burden will come from more sophisticated AI algorithms. Natural language processing (NLP) will become even more nuanced, accurately interpreting complex medical language, including physician notes, patient reports, and research papers. This will allow for automated summarization, data extraction, and even predictive analytics based on patient history and current trends. For example, an AI system could analyze a patient’s chart and flag potential drug interactions or adverse effects before they occur, proactively alerting the physician.
Machine learning models will also improve in their ability to personalize documentation workflows, learning individual physician preferences and adapting accordingly. This might involve automatically generating sections of notes based on established patterns or suggesting relevant codes and diagnoses.
The Role of Interoperability in AI Adoption, Micky tripathi onc healthcare ai ehrs documentation burden
The widespread adoption of AI-powered EHR solutions critically depends on interoperability. Currently, data silos between different healthcare systems hinder the development and deployment of robust AI models. To fully realize the potential of AI, seamless data exchange is necessary. Imagine a scenario where AI systems across multiple hospitals can access and analyze a patient’s complete medical history, regardless of where they received care.
This would allow for more accurate diagnoses, personalized treatment plans, and reduced medical errors. Standardized data formats and APIs are essential for achieving this level of interoperability. Initiatives like FHIR (Fast Healthcare Interoperability Resources) are crucial steps in this direction, paving the way for a more connected and AI-ready healthcare ecosystem.
A Hypothetical Scenario: AI Transforming Healthcare
Consider a future where a patient with a chronic condition, say diabetes, uses a smart wearable device that continuously monitors their blood glucose levels. This data is automatically integrated into their EHR, triggering an AI system to analyze the trends and identify potential problems. If a concerning pattern emerges, the AI system automatically alerts both the patient and their physician, suggesting adjustments to their medication or lifestyle.
The physician, meanwhile, uses an AI-powered assistant to generate comprehensive patient notes, reducing their administrative burden and freeing up more time for direct patient care. The AI assistant also proactively identifies relevant research and guidelines, ensuring the physician is up-to-date on the latest best practices. This streamlined workflow leads to improved patient outcomes, increased physician satisfaction, and a more efficient healthcare system overall.
This scenario highlights the potential for AI to transform healthcare from a reactive to a proactive model, empowering both providers and patients to make informed decisions and achieve better health outcomes.
Last Point
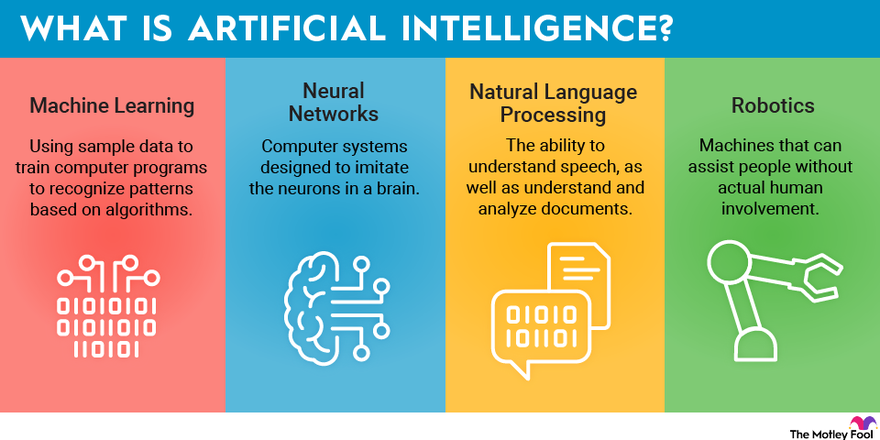
Source: foolcdn.com
So, where does that leave us? The potential of AI to revolutionize healthcare documentation is undeniable. Mickey Tripathi’s leadership in pushing for AI adoption within the ONC is crucial in making this a reality. But we need to proceed cautiously, addressing the ethical concerns and regulatory hurdles to ensure responsible and equitable implementation. The future of healthcare documentation hinges on a thoughtful approach, balancing technological advancements with human oversight and a commitment to patient well-being.
Let’s work together to create a future where healthcare professionals can focus on what truly matters: their patients.
FAQ Overview
What specific AI tools are currently being used to reduce EHR documentation burden?
Several tools utilize NLP for automated note generation, voice-to-text for dictation, and predictive text to speed up data entry. Specific examples vary widely depending on the vendor and EHR system.
What are the biggest privacy concerns related to AI in EHRs?
Maintaining patient data privacy is paramount. Concerns include unauthorized access, data breaches, and the potential for AI algorithms to inadvertently reveal sensitive information.
How does human oversight play a role in AI-driven EHR systems?
Human oversight is crucial for ensuring accuracy, addressing biases in algorithms, and maintaining ethical standards. Humans should always review and validate AI-generated information before making clinical decisions.