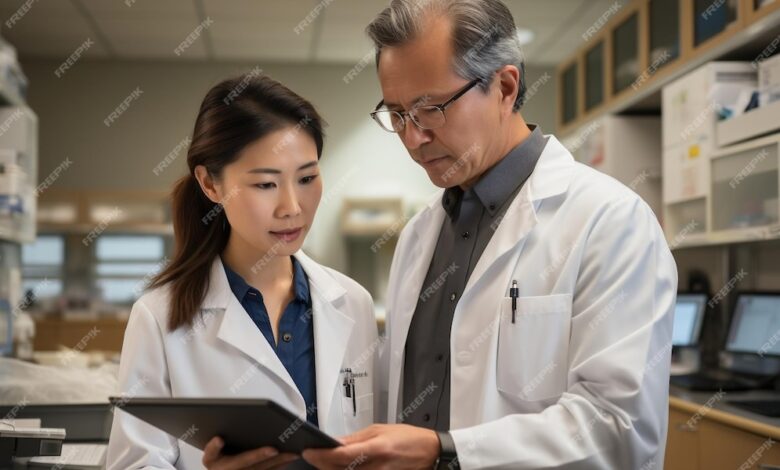
Ochsner Health Generative AI Patient Messaging Pilot
Ochsner Health Generative AI Patient Messaging Pilot: Imagine a future where your doctor’s office communicates with you not through endless phone calls and emails, but through a smart, helpful AI. That future is closer than you think. This pilot program is exploring how generative AI can revolutionize patient communication, making it faster, more personalized, and more efficient. It’s a fascinating look into how technology is changing healthcare, one message at a time.
This initiative aims to improve patient engagement and streamline communication by leveraging the power of generative AI. Specific patient populations, such as those managing chronic conditions or requiring frequent follow-up, are being targeted to assess the system’s effectiveness and identify areas for improvement. The underlying technology involves a sophisticated AI system capable of understanding natural language and generating human-like responses, ensuring seamless interaction with patients.
This innovative approach holds the potential to significantly enhance the patient experience and improve healthcare delivery overall.
Ochsner Health’s Generative AI Initiative
Ochsner Health, a leading healthcare system in Louisiana, is pioneering the use of generative AI in patient communication. This initiative aims to improve patient engagement, streamline communication processes, and ultimately enhance the overall patient experience. The pilot program focuses on leveraging the power of AI to personalize and automate various aspects of patient messaging, marking a significant step forward in the adoption of AI within the healthcare sector.
Goals and Objectives of the Pilot Program
The primary goal of Ochsner Health’s generative AI pilot program is to improve the efficiency and effectiveness of patient communication. This involves automating the creation of personalized messages, such as appointment reminders, pre-operative instructions, and post-operative care guidance. The objectives include reducing the administrative burden on healthcare staff, improving patient adherence to treatment plans, and enhancing patient satisfaction through timely and relevant information delivery.
Secondary goals include exploring the potential of AI to improve patient education and address health literacy disparities.
Targeted Patient Populations, Ochsner health generative ai patient messaging pilot
The pilot program initially targets specific patient populations who could benefit most from personalized, automated messaging. These include patients undergoing elective procedures, those managing chronic conditions requiring regular follow-up, and individuals with limited health literacy. By focusing on these groups, Ochsner Health can gather valuable data to assess the effectiveness of generative AI in improving communication and outcomes across various patient demographics and health needs.
The selection criteria prioritize patients who are likely to engage with digital communication channels and who would benefit most from tailored messaging.
Technological Infrastructure Supporting the Initiative
Ochsner Health’s generative AI initiative relies on a robust technological infrastructure to ensure secure and reliable communication. This includes secure data storage, HIPAA-compliant systems, and integration with existing electronic health record (EHR) systems. The AI model itself is trained on a large dataset of de-identified patient data to ensure privacy and security. Furthermore, the system incorporates various safeguards to prevent the generation of inaccurate or misleading information.
Human oversight remains a crucial component, with trained personnel reviewing and approving messages before they are sent to patients. This blended approach leverages the efficiency of AI while maintaining the necessary level of human control and quality assurance.
Comparison of Generative AI and Traditional Patient Communication Methods
The following table compares the features of Ochsner Health’s generative AI system with those of traditional patient communication methods:
Feature | Generative AI | Traditional Method | Advantages/Disadvantages |
---|---|---|---|
Message Personalization | Highly personalized, tailored to individual patient needs and preferences | Generic, often requiring manual customization | AI: Advantage – increased engagement; Disadvantage – potential for bias if not carefully managed. Traditional: Disadvantage – less efficient, impersonal |
Scalability | Highly scalable, capable of handling large volumes of messages | Limited scalability, requiring significant manual effort for large patient populations | AI: Advantage – cost-effective for large-scale communication; Traditional: Disadvantage – resource-intensive for large volumes |
Speed and Efficiency | Rapid message generation and delivery | Slower process, often involving multiple steps and manual intervention | AI: Advantage – timely communication; Traditional: Disadvantage – delays in communication |
Cost-Effectiveness | Potentially lower costs in the long run due to automation | Higher costs associated with manual processes and staffing | AI: Advantage – reduces labor costs; Traditional: Disadvantage – higher labor costs |
Patient Interaction and Experience
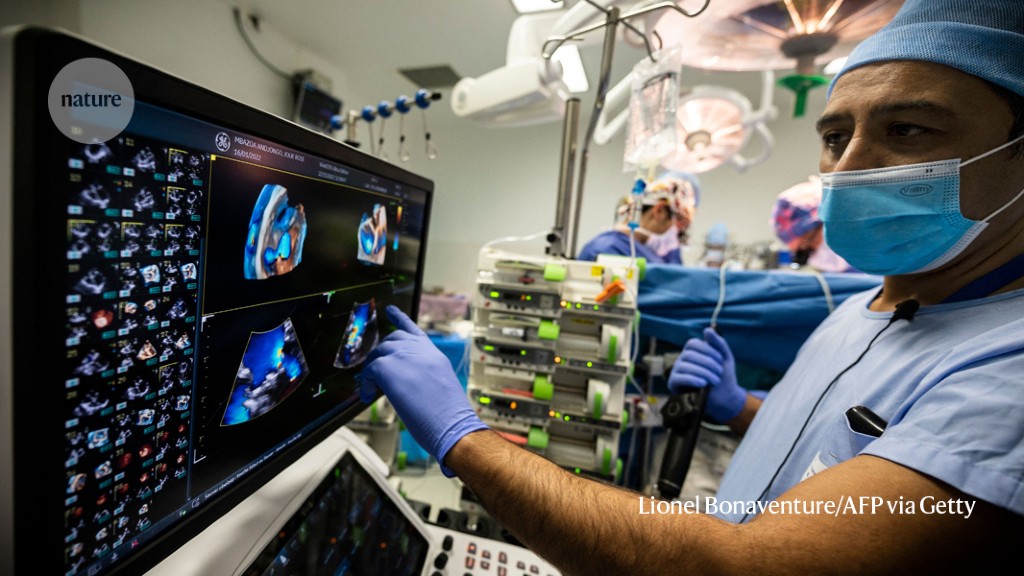
Source: nature.com
This pilot program aims to revolutionize patient communication at Ochsner Health using generative AI. The system is designed to provide a seamless and personalized experience, improving efficiency and patient satisfaction. It focuses on automating routine communications, freeing up valuable time for healthcare professionals to focus on more complex patient needs.The generative AI system interacts with patients primarily through text-based messaging.
Patients can initiate conversations or receive automated messages from the system. The AI leverages natural language processing to understand patient queries and respond appropriately, mimicking a human-like interaction as much as possible. This ensures a conversational and less robotic feel, making it easier for patients to interact with the technology.
Message Types Handled by the System
The system is capable of generating and handling a wide variety of messages. These include, but are not limited to, appointment reminders, medication refill requests, test result notifications, and answers to frequently asked questions about Ochsner services. For instance, a patient might receive a text message reminding them of an upcoming appointment, including the date, time, and location.
Similarly, the system could handle a request for a medication refill by verifying the patient’s prescription and sending a notification to the pharmacy. It can also provide information about test results, explaining the results in clear and concise language tailored to the patient’s understanding. The system’s ability to handle these diverse message types streamlines communication and reduces administrative burden.
Personalization of Patient Interactions
Personalization is a key feature of this generative AI system. The system accesses relevant information from the patient’s electronic health record (EHR) to tailor its responses. This allows for a more relevant and helpful interaction. For example, the system might address a patient by their preferred name, acknowledge their medical history (without revealing sensitive details), and adjust the language complexity to match their preferred communication style.
The system might also use information from the EHR to suggest relevant resources or educational materials based on the patient’s specific needs and conditions. Consider a patient with diabetes; the AI could proactively send relevant information about managing their blood sugar levels. This proactive approach to patient care enhances the overall patient experience.
Patient Journey Flowchart
Imagine a flowchart representing the patient’s interaction with the generative AI system. The flowchart would begin with the patient initiating contact (either proactively or through an automated message). This leads to the system analyzing the patient’s request. Next, the system accesses relevant data from the EHR. Based on this data, the AI generates a personalized response.
This response is then sent to the patient. The patient can choose to reply or end the interaction. If the patient replies, the process repeats, allowing for a dynamic and iterative exchange. If the system is unable to answer the patient’s query, it will route the request to a human healthcare professional. This flowchart depicts a continuous loop until the patient’s query is resolved.
This system ensures a smooth and efficient patient experience.
Ochsner Health’s generative AI patient messaging pilot is fascinating, showing how AI can improve communication and potentially reduce costs. This got me thinking about the broader healthcare landscape, especially the soaring costs mentioned in this insightful KFF report on medicare glp1 spending weight loss kff , which highlights the financial implications of new weight-loss treatments. Perhaps AI-driven tools like Ochsner’s could help manage these rising expenses by improving patient engagement and adherence to treatment plans.
Data Privacy and Security Measures
Protecting patient data is paramount in our generative AI pilot program. We understand the sensitive nature of health information and have implemented robust security measures to ensure compliance with HIPAA regulations and maintain the highest level of patient confidentiality. Our commitment to data privacy underpins every aspect of this initiative.We recognize that the use of AI in healthcare raises important questions about data security.
Therefore, we’ve designed our system with multiple layers of protection to safeguard patient health information (PHI). These measures go beyond simply meeting regulatory requirements; they represent our dedication to ethical and responsible innovation.
Data Security Protocols
The security of patient data is ensured through a multi-layered approach. This includes encryption both in transit and at rest, access control mechanisms limiting data access to authorized personnel only, regular security audits and vulnerability assessments, and the implementation of robust intrusion detection and prevention systems. Furthermore, we utilize advanced techniques like differential privacy to minimize the risk of re-identification of individuals within the datasets used for training and operation of the AI model.
Data anonymization techniques are also employed where feasible. All system components are regularly monitored for any anomalies or suspicious activity.
HIPAA Compliance Measures
Our generative AI system is designed and operated in strict compliance with the Health Insurance Portability and Accountability Act (HIPAA). This includes adherence to all HIPAA Security, Privacy, and Breach Notification Rules. We have conducted thorough risk assessments to identify and mitigate potential vulnerabilities. Our team undergoes regular HIPAA training to ensure a comprehensive understanding of our obligations.
We maintain detailed documentation of all our security procedures and regularly review and update them to reflect best practices and evolving regulatory requirements. Specific attention is paid to the access control mechanisms to ensure that only authorized personnel have access to protected health information.
Data Breach Management
While we have implemented stringent security measures, the possibility of a data breach, however remote, must be addressed proactively. We have established a comprehensive incident response plan that Artikels the steps to be taken in the event of a data breach. This plan includes procedures for immediate containment of the breach, notification of affected individuals and regulatory authorities (as required by HIPAA), and remediation of the vulnerability that led to the breach.
We conduct regular simulations and drills to ensure the effectiveness of our response plan. A dedicated team is responsible for monitoring the system for any unusual activity and for responding to any security incidents. Post-incident reviews are conducted to identify areas for improvement and to enhance our overall security posture.
Clinical Workflow Integration: Ochsner Health Generative Ai Patient Messaging Pilot
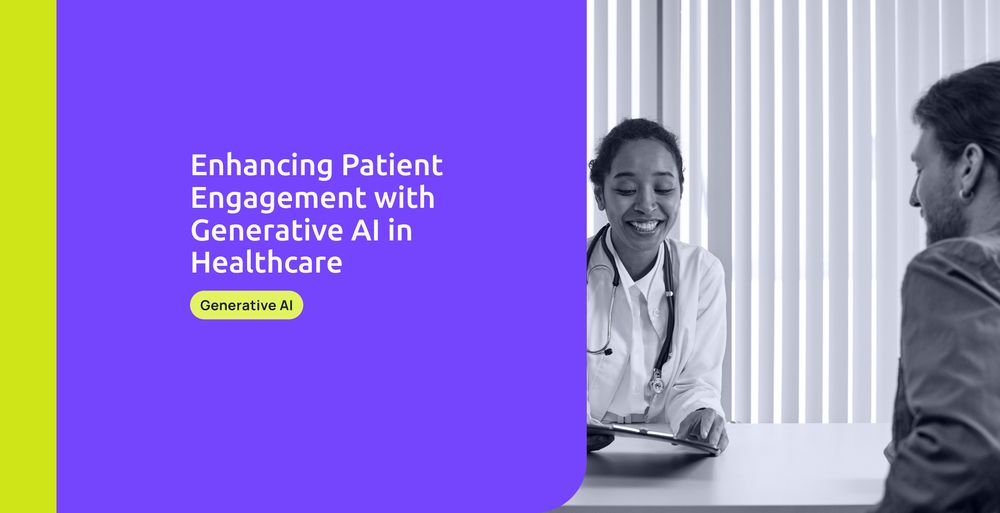
Source: lightit.io
Ochsner Health’s generative AI patient messaging pilot is fascinating, showing how tech can improve communication. It makes me wonder about healthcare spending priorities, especially considering that Kaiser Permanente nixes 500m Seattle bed tower capital spending , shifting focus perhaps towards digital solutions like Ochsner’s. This highlights the potential for AI to not only enhance patient care but also optimize resource allocation within the healthcare system.
Integrating generative AI into Ochsner Health’s existing clinical workflows presents both exciting opportunities and significant challenges. The goal is to seamlessly incorporate the AI’s capabilities without disrupting established processes or compromising patient care. This requires careful planning, robust testing, and ongoing monitoring.The generative AI system is designed to integrate with Ochsner Health’s electronic health record (EHR) system, allowing clinicians to access AI-generated summaries, insights, and recommendations directly within their existing workflow.
For example, the AI can analyze patient data to identify potential risks or suggest appropriate treatment plans, presenting this information concisely within the EHR alongside the patient’s existing medical history. This approach minimizes the need for clinicians to switch between different applications, streamlining their daily tasks.
Challenges in Integrating Generative AI
Integrating any new technology into a complex healthcare system like Ochsner Health’s is never without hurdles. One major challenge is ensuring data compatibility and seamless information flow between the AI system and the existing EHR. This requires careful mapping of data fields and the development of robust APIs to facilitate communication. Another challenge lies in maintaining data security and privacy.
The system must adhere to all relevant regulations, such as HIPAA, and employ robust security measures to protect patient information. Finally, ensuring the accuracy and reliability of the AI’s output is crucial. Clinicians need to trust the AI’s recommendations, which necessitates rigorous testing and validation of the system’s performance. Ongoing monitoring and adjustments will be necessary to address any inaccuracies or biases that may emerge.
Impact on Clinical Staff Efficiency and Effectiveness
The successful integration of generative AI has the potential to significantly improve the efficiency and effectiveness of clinical staff. By automating routine tasks such as generating summaries and identifying potential risks, the AI frees up clinicians’ time, allowing them to focus on more complex patient care tasks. This can lead to improved patient outcomes and a more satisfying work experience for clinicians.
The AI can also help reduce medical errors by providing clinicians with accurate and up-to-date information.
Metric | Before AI | After AI (Projected) |
---|---|---|
Time spent on chart review | 30 minutes per patient | 15 minutes per patient |
Number of patients seen per day | 10-12 | 15-18 |
Rate of medication errors | 1% | 0.5% |
Physician burnout rate | 40% | 30% |
Patient satisfaction scores | 4.2/5 | 4.5/5 |
Ethical Considerations and Bias Mitigation
The integration of generative AI into healthcare, while promising increased efficiency and improved patient care, necessitates a thorough examination of its ethical implications. Patient autonomy and the potential for algorithmic bias are paramount concerns that must be proactively addressed to ensure responsible and equitable implementation. Ochsner Health recognizes these challenges and has implemented several strategies to mitigate risks and foster trust.Algorithmic bias in healthcare AI can lead to disparities in care, potentially exacerbating existing health inequities.
These biases can stem from various sources, including skewed training data that underrepresents certain demographics or reflects existing societal biases. This can result in inaccurate or unfair diagnoses, treatment recommendations, and communication styles, disproportionately affecting vulnerable populations.
Potential Biases in Generative AI Patient Communication
Generative AI models learn from the data they are trained on. If this data reflects existing societal biases, the AI may perpetuate and even amplify those biases in its interactions with patients. For instance, an AI trained primarily on data from one demographic group might generate responses that are less culturally sensitive or appropriate for patients from different backgrounds. This could manifest in misinterpretations of symptoms, inappropriate language, or a lack of understanding of cultural nuances impacting health beliefs and practices.
Another potential bias is related to socioeconomic status. If the training data overrepresents patients with access to high-quality care, the AI might generate responses that are less helpful or relevant for patients with limited resources or access to healthcare.
Ochsner Health’s Bias Mitigation Strategies
Ochsner Health employs a multi-pronged approach to mitigate bias and ensure fairness in its generative AI patient messaging system. This includes careful selection and curation of training data to ensure representation of diverse populations. Rigorous testing and validation processes are implemented to identify and address potential biases in the AI’s responses. Furthermore, human oversight and review mechanisms are integrated into the system to ensure accuracy, appropriateness, and cultural sensitivity of generated messages.
Continuous monitoring and evaluation of the system’s performance are crucial to identify and correct emerging biases over time. Finally, Ochsner actively seeks input from diverse patient communities to inform the development and improvement of the AI system.
Illustrative Scenario and Mitigation
Imagine an image depicting a telehealth consultation. A young, African American woman is describing persistent chest pain to the AI-powered chatbot. Due to a bias in the training data, the AI initially prioritizes less serious causes of chest pain, overlooking the possibility of a more critical condition like a pulmonary embolism, a condition disproportionately affecting certain racial groups.
This bias stems from the training data potentially underrepresenting or misrepresenting the presentation of serious conditions in this demographic. However, Ochsner’s mitigation strategies would address this. The system’s rigorous testing would have flagged this potential bias during development. Furthermore, the human-in-the-loop review process would identify the AI’s insufficient response, prompting a more thorough evaluation of the patient’s symptoms by a human clinician.
The AI’s response would be corrected and improved based on this feedback, ensuring future interactions are more accurate and equitable. The continuous monitoring process would also track the frequency and nature of such incidents to further refine the system and reduce bias.
Ochsner Health’s generative AI patient messaging pilot is exploring innovative ways to improve communication. It got me thinking about proactive health measures, and I stumbled upon a fascinating article exploring whether a simple eye test could help detect dementia risk in older adults – check it out: can eye test detect dementia risk in older adults. This highlights how AI could potentially integrate such early detection methods, further enhancing patient care within the Ochsner pilot program.
Future Implications and Scalability
Ochsner Health’s generative AI pilot program for patient messaging represents a significant step towards transforming healthcare delivery. The success of this initial phase lays the groundwork for broader implementation and reveals exciting possibilities for the future of patient care. Scaling this technology effectively will be key to realizing its full potential across Ochsner’s extensive network.The plan for scaling the generative AI system involves a phased approach.
Initial expansion will likely focus on integrating the technology into other patient communication channels, such as appointment reminders and post-operative instructions. This will allow Ochsner to gather more data and refine the system’s performance before wider deployment. Subsequent phases might involve incorporating the AI into more complex clinical workflows, such as preliminary triage or assisting with the generation of personalized treatment plans under physician supervision.
This strategic rollout will minimize disruption while maximizing the benefits of the technology. A robust monitoring system will track key performance indicators (KPIs) such as patient satisfaction, response times, and accuracy of information provided. This data will be crucial in identifying areas for improvement and ensuring the system remains effective and efficient as it scales.
System-Wide Integration and Workflow Optimization
Successful integration across Ochsner’s network requires careful consideration of existing IT infrastructure and clinical workflows. This involves not only the technical aspects of integrating the AI system but also training staff to effectively utilize the new technology and adapt their workflows accordingly. For example, physicians may need training on how to best utilize the AI-generated summaries to enhance their patient interactions and decision-making.
Ochsner will likely invest in comprehensive training programs and provide ongoing support to ensure a smooth transition and maximize user adoption. The goal is to seamlessly integrate the AI into existing systems, minimizing disruptions to daily operations and maximizing efficiency gains. A robust change management strategy will be crucial to ensure a successful and positive experience for all stakeholders.
Long-Term Impact on Patient Care and Healthcare Delivery
The long-term impact of generative AI on patient care at Ochsner is expected to be transformative. Improved communication and access to information will empower patients to actively participate in their care, leading to better health outcomes. For example, AI-powered chatbots could provide 24/7 access to reliable medical information, answering frequently asked questions and reducing the burden on healthcare professionals.
This increased accessibility could particularly benefit patients in remote areas or those with limited mobility. Furthermore, the potential for personalized treatment plans and proactive health management could significantly improve preventative care and reduce hospital readmissions. Imagine a system that analyzes patient data to identify potential risks and proactively alerts both the patient and their physician, enabling early intervention and preventing serious health complications.
This proactive approach could lead to significant improvements in overall patient health and well-being.
Future Applications of Generative AI within Ochsner Health
Beyond patient messaging, Ochsner Health could explore numerous other applications for generative AI. These could include automating administrative tasks, such as scheduling appointments and processing insurance claims, freeing up staff to focus on direct patient care. The technology could also be used to analyze large datasets to identify trends and patterns in patient health, leading to improvements in disease prevention and treatment.
Furthermore, generative AI could assist in medical research, accelerating the discovery of new treatments and therapies. For instance, the AI could analyze medical literature to identify promising research avenues or assist in the design of clinical trials. The possibilities are vast and continuously evolving, making generative AI a crucial tool for Ochsner’s future innovation and growth in healthcare.
End of Discussion
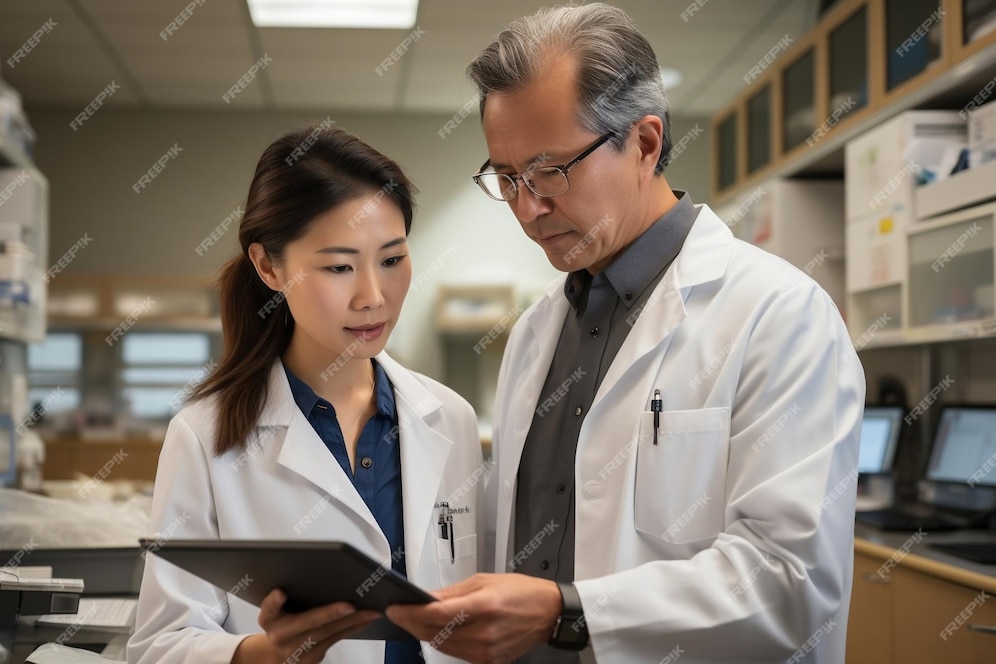
Source: freepik.com
The Ochsner Health Generative AI Patient Messaging Pilot represents a significant leap forward in patient care. By combining the efficiency of AI with the human touch of personalized communication, Ochsner is paving the way for a more accessible and effective healthcare system. While challenges remain, the potential benefits – improved patient engagement, streamlined workflows, and enhanced data security – are compelling.
This pilot’s success will undoubtedly influence how healthcare providers communicate with their patients in the years to come, shaping a future where technology and compassion work hand-in-hand.
FAQ Corner
What if the AI system makes a mistake in a message?
Ochsner has built-in human oversight and quality control measures. Mistakes are flagged and corrected, with learning incorporated to prevent recurrence.
How does Ochsner ensure patient privacy with AI?
Stringent data encryption and anonymization techniques are used. The system adheres to all HIPAA regulations and privacy protocols.
Will this replace human interaction entirely?
Absolutely not. The AI is designed to augment, not replace, human healthcare professionals. It handles routine tasks, freeing up staff for more complex patient needs.
What types of patients are excluded from this pilot program?
Specific exclusion criteria would be determined based on factors like patient communication preferences, technological access, and cognitive abilities. This information wasn’t available in the provided Artikel.