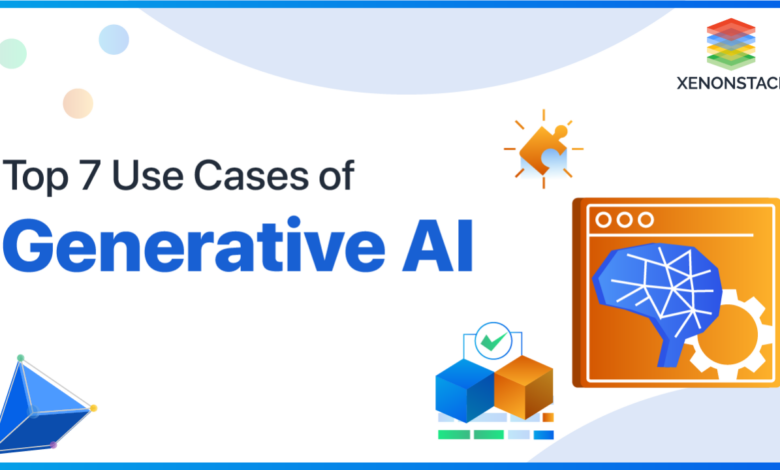
Nextgen Healthcare Generative AI, Clinical Docs, Ambient Assist
Nextgen healthcare generative AI clinical documentation ambient assist: Imagine a future where doctors spend less time on paperwork and more time with patients. That’s the promise of this revolutionary technology. By leveraging the power of generative AI, we can automate the creation of patient summaries, freeing up clinicians to focus on what truly matters – providing the best possible care.
But this isn’t just about efficiency; it’s about integrating seamless ambient assist technology to create a truly personalized and proactive healthcare experience. This post dives into the exciting possibilities, challenges, and ethical considerations surrounding this rapidly evolving field.
This blend of artificial intelligence and ambient assistance promises a radical shift in how healthcare is delivered. We’ll explore how generative AI can analyze diverse data sources – from EHRs to wearable sensors – to generate comprehensive and accurate patient summaries. We’ll also discuss the crucial role of data management, security, and user interface design in ensuring this technology is both effective and ethical.
Finally, we’ll look ahead to the future, considering the potential for even more personalized and proactive healthcare experiences powered by AI.
Generative AI in Clinical Documentation
The integration of generative AI into clinical workflows holds immense potential to revolutionize healthcare, particularly in the realm of clinical documentation. This technology offers the possibility of automating tedious tasks, freeing up clinicians’ time for patient care and ultimately improving both efficiency and patient outcomes. However, careful consideration of ethical and practical implications is crucial for responsible implementation.
Next-gen healthcare is all about leveraging AI, like generative AI for clinical documentation and ambient assist. This tech can be a game-changer in identifying and managing high-risk patients, for example, by flagging individuals with multiple risk factors for stroke, which, as outlined in this helpful article on risk factors that make stroke more dangerous , can significantly impact recovery.
Understanding these factors allows for proactive intervention and ultimately improves patient outcomes, a key goal of next-gen healthcare AI.
Workflow for Automated Patient Summary Generation
Generative AI can streamline the creation of patient summaries by consolidating information from diverse sources. The following workflow illustrates this process:
Data Source | AI Processing Steps | Output Format | Example |
---|---|---|---|
Electronic Health Record (EHR) | Data extraction, natural language processing (NLP) to identify key findings, structured data conversion | Structured data (e.g., JSON) | Patient demographics, diagnoses, medications, allergies, lab results, vital signs |
Physician Notes | NLP to summarize key clinical observations, identify relevant findings, and filter out irrelevant information | Structured data (e.g., JSON) | Summary of chief complaint, physical exam findings, and physician assessment |
Radiology Reports | NLP to extract key findings and observations from radiology reports | Structured data (e.g., JSON) | Description of imaging findings, relevant measurements, and interpretation |
Nursing Notes | NLP to summarize nursing observations, vital signs, and patient response to treatment | Structured data (e.g., JSON) | Record of patient’s pain levels, response to medications, and any notable events |
Consolidated Data | Generative AI model assembles structured data into a comprehensive, coherent patient summary. | Narrative summary, suitable for inclusion in the EHR | A concise and accurate summary of the patient’s condition, treatment, and prognosis. |
Benefits of Generative AI for Clinical Documentation
The use of generative AI in clinical documentation offers significant advantages, particularly in improving efficiency and mitigating physician burnout. Automating the generation of patient summaries reduces the administrative burden on physicians, allowing them to focus more on direct patient interaction and complex medical decision-making. This can lead to improved job satisfaction and a reduction in burnout rates, a significant concern within the medical profession.
For instance, a study by the Mayo Clinic showed that physicians spend an average of two hours per day on administrative tasks, time that could be better spent on patient care. Generative AI can significantly reduce this burden.
Risks and Challenges of Generative AI in Clinical Documentation
Despite its potential benefits, the implementation of generative AI in clinical documentation presents several risks and challenges. Data privacy is paramount; robust security measures are necessary to protect sensitive patient information. Accuracy is another critical concern; AI models must be rigorously validated to ensure the reliability of generated summaries. Liability is a complex issue; determining responsibility for errors or omissions in AI-generated documentation requires careful consideration of legal and ethical frameworks.
For example, an inaccurate diagnosis generated by the AI could have severe legal repercussions.
Comparison of Generative AI Models for Clinical Documentation
Several generative AI models are suitable for clinical documentation tasks, each with its strengths and weaknesses. Large language models (LLMs) like GPT-3 and similar models excel at generating human-quality text but require substantial computational resources and careful fine-tuning for medical applications to ensure accuracy. Other models, such as those based on recurrent neural networks (RNNs), may be more efficient for specific tasks but may lack the versatility of LLMs.
The choice of model depends on factors such as the specific clinical documentation task, the available computational resources, and the desired level of accuracy and speed. Cost is also a significant factor; the cost of training and deploying these models can be substantial, requiring careful cost-benefit analysis.
Ambient Assist Technology Integration
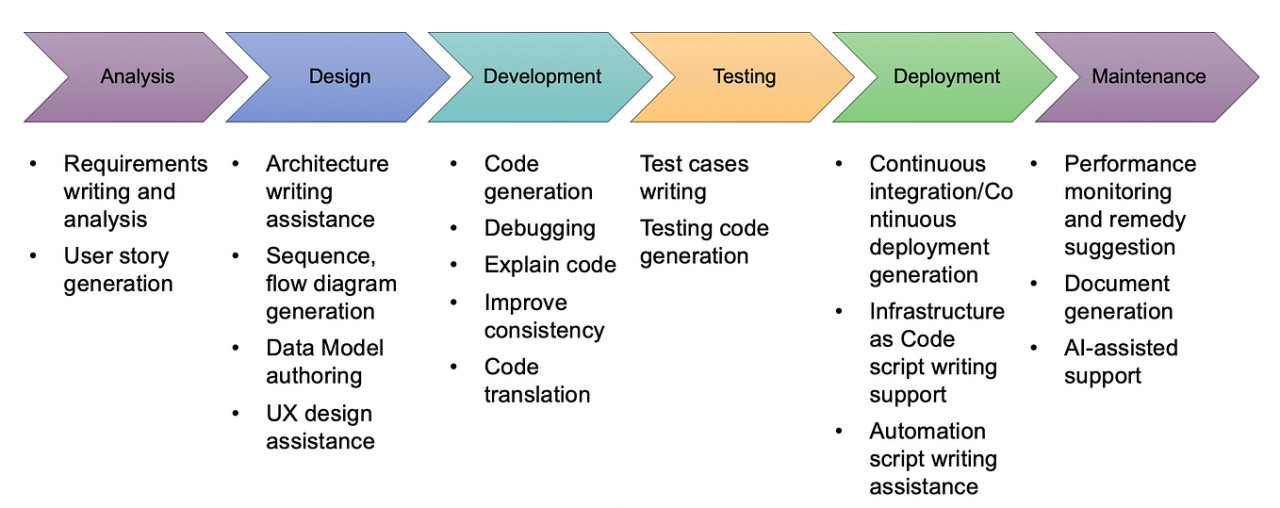
Source: medium.com
Imagine a future hospital where clinicians aren’t bogged down by paperwork, where patient data is instantly accessible, and where proactive interventions prevent potential crises. This isn’t science fiction; it’s the promise of integrating ambient assist technology with generative AI in healthcare. This powerful combination streamlines workflows, enhances patient care, and fundamentally reshapes the clinical experience.Ambient assist technology, encompassing voice assistants, smart sensors, and wearable devices, provides a continuous stream of contextual data.
Coupled with generative AI’s ability to process and interpret this data, we can create a system that anticipates needs, automates tasks, and empowers clinicians to focus on what truly matters: the patient.
A Scenario Illustrating Ambient Assist and Generative AI Integration
Let’s consider a busy oncology ward. A nurse, Dr. Anya Sharma, is attending to Mr. Jones, a patient experiencing post-chemotherapy nausea. As Dr.
Sharma speaks to Mr. Jones, an ambient microphone discreetly captures the conversation. The system, using natural language processing (NLP), identifies key symptoms and contextual information. This information is instantly fed into the generative AI system, which cross-references Mr. Jones’s electronic health record (EHR), lab results, and treatment plan.
The AI then suggests appropriate interventions, such as adjusting medication dosages or recommending additional comfort measures, presenting this information to Dr. Sharma in a concise, easy-to-understand format on her smart glasses or wrist device.
- Real-time Data Acquisition: Ambient sensors continuously monitor patient vital signs, activity levels, and environmental factors.
- Automated Documentation: The AI automatically generates progress notes, documenting observations and interventions, freeing up clinicians’ time.
- Proactive Alerts: The system proactively alerts the care team to potential issues, such as a patient’s deteriorating condition or medication errors.
- Personalized Recommendations: The AI uses machine learning to personalize treatment plans and recommendations based on individual patient data and preferences.
- Enhanced Communication: Seamless integration with communication platforms facilitates efficient collaboration among the care team.
Ethical Implications of Ambient Assist and Generative AI
The integration of ambient assist technology and generative AI raises important ethical considerations. Ensuring patient autonomy and data security is paramount. Transparency is crucial; patients must be fully informed about how their data is being collected and used. Strict adherence to data privacy regulations, such as HIPAA, is non-negotiable. Robust security measures, including encryption and access controls, are essential to prevent unauthorized access and data breaches.
Furthermore, algorithms must be rigorously tested and validated to minimize bias and ensure fairness in care delivery. The potential for algorithmic bias leading to discriminatory outcomes must be actively addressed through ongoing monitoring and evaluation. Clinicians must retain ultimate responsibility for decision-making, using the AI as a supportive tool, not a replacement for human judgment.
Beyond Clinical Documentation: Expanding Use Cases
The applications of ambient assist technology integrated with generative AI extend far beyond clinical documentation.
- Medication Management: The system can track medication adherence, identify potential drug interactions, and alert clinicians to potential adverse events.
- Patient Monitoring: Continuous monitoring of vital signs and activity levels allows for early detection of deterioration and timely intervention.
- Remote Patient Monitoring: The technology enables remote monitoring of patients in their homes, reducing hospital readmissions and improving patient outcomes.
Enhancing the Patient Experience
Ambient assist technology, when thoughtfully implemented, can significantly enhance the patient experience.
- Personalized Care: The system can provide personalized information and support, tailored to individual patient needs and preferences.
- Proactive Care: By anticipating potential problems, the system can provide proactive interventions, preventing complications and improving patient comfort.
- Improved Communication: Easy access to information and direct communication channels empower patients to actively participate in their care.
Next-Generation Healthcare Data Management
The successful implementation of generative AI in clinical documentation and ambient assist hinges on robust data management strategies. The sheer volume, velocity, and variety of healthcare data present unique challenges, demanding careful planning for storage, security, and ethical considerations. Effective data management is not just a technical issue; it’s fundamental to ensuring the accuracy, reliability, and ethical use of AI in healthcare.
Data Management Plan for Generative AI Models, Nextgen healthcare generative ai clinical documentation ambient assist
A comprehensive plan is crucial for managing the extensive datasets needed to train and operate generative AI models for clinical applications. This involves careful consideration of data types, storage methods, and security protocols. The following table Artikels a potential approach:
Data Type | Storage Method | Security Measures |
---|---|---|
Electronic Health Records (EHRs) | Cloud-based storage with HIPAA-compliant encryption | Access control lists (ACLs), data masking, regular security audits |
Medical Images (DICOM) | High-performance storage systems (e.g., object storage) with de-identification capabilities | Encryption at rest and in transit, granular access control |
Clinical Notes (free text) | Database optimized for natural language processing (NLP) tasks | Differential privacy techniques, data anonymization before storage |
Sensor Data (ambient assist) | Time-series database with real-time processing capabilities | Data aggregation and anonymization, secure data streams |
Data Anonymization and De-identification Techniques
Protecting patient privacy is paramount. Data anonymization and de-identification are essential techniques to ensure compliance with regulations like HIPAA. Anonymization involves removing identifying information like names, addresses, and medical record numbers. De-identification goes further, employing techniques like data perturbation (adding random noise) or generalization (replacing specific values with broader categories) to minimize the risk of re-identification. For example, instead of storing a patient’s exact age, a range (e.g., 40-49) might be used.
These methods allow for the use of data in AI model training while safeguarding individual privacy.
Potential Data Sources for Training Generative AI Models
Several data sources can be leveraged to train generative AI models for next-generation healthcare applications. The quality and diversity of these sources significantly impact the performance and generalizability of the models.
The following sources offer valuable data for training:
- Electronic Health Records (EHRs): A primary source of structured and unstructured clinical data.
- Medical Imaging Data (DICOM): Provides visual information crucial for radiology and pathology AI applications.
- Clinical Notes and Reports: Rich source of unstructured text data containing clinical observations and diagnoses.
- Wearable Sensor Data: Offers insights into patient activity levels, sleep patterns, and vital signs.
- Publicly Available Datasets: Datasets from research institutions and government agencies, after appropriate ethical review and de-identification.
- Claims Data: Provides information on diagnoses, procedures, and costs.
Data Quality and Validation Processes
Ensuring the accuracy and reliability of AI-generated clinical documentation requires rigorous data quality and validation processes. Data cleaning, error correction, and consistency checks are crucial steps. Validation involves comparing AI-generated documentation against gold-standard references (e.g., expert-reviewed notes) to assess accuracy and identify areas for improvement. Regular audits and feedback loops help maintain data quality and refine the AI models over time.
For instance, a system might compare the AI-generated summary of a patient visit with the physician’s final note, highlighting discrepancies for review and correction, leading to continuous model improvement.
Next-gen healthcare is all about efficiency, and generative AI for clinical documentation and ambient assist is a huge step forward. However, the news about Steward Health Care’s struggles, including steward ohio hospitals closures pennsylvania facility at risk , highlights the challenges facing even established systems. This makes the development and implementation of AI-driven solutions even more critical for improving healthcare access and quality in the future.
User Interface and Experience Design: Nextgen Healthcare Generative Ai Clinical Documentation Ambient Assist
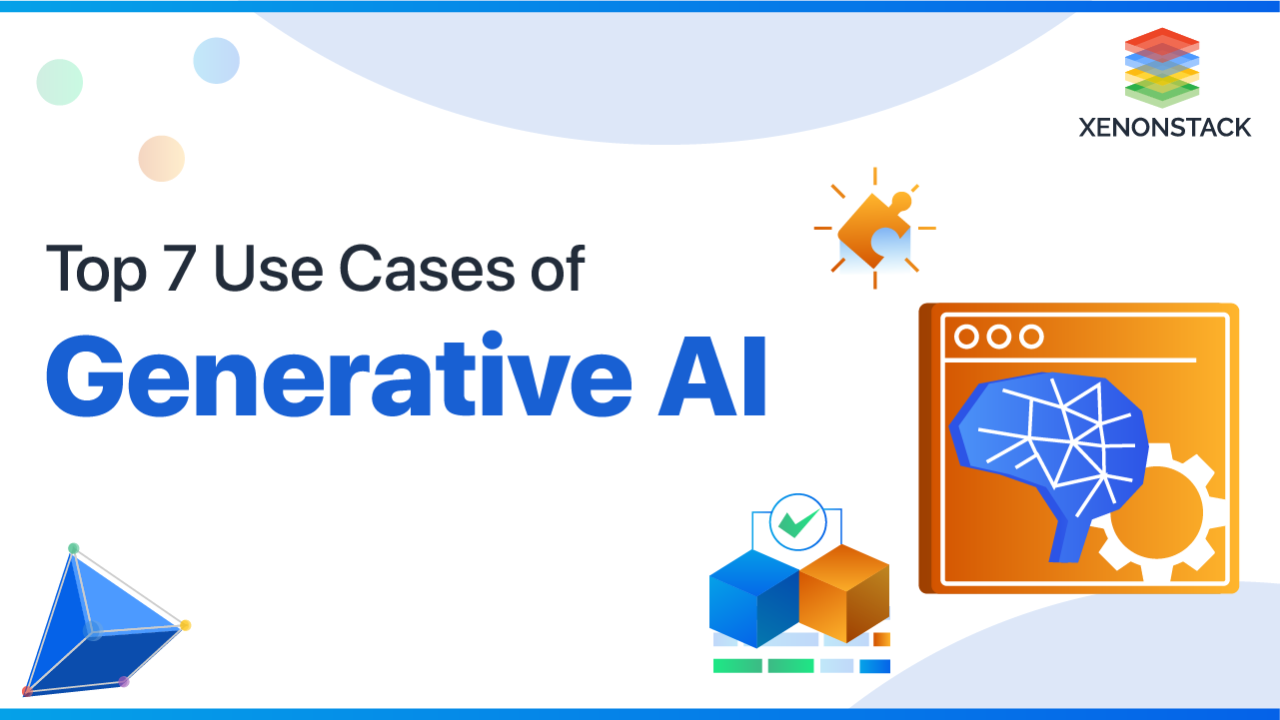
Source: xenonstack.com
Designing an intuitive and user-friendly interface is paramount for the successful adoption of any AI-powered healthcare system. A poorly designed interface can lead to frustration, errors, and ultimately, a reluctance to use the technology, negating its potential benefits. The interface must seamlessly integrate generative AI capabilities for clinical documentation and ambient assist, streamlining workflows and enhancing the efficiency of healthcare professionals.The ideal user interface should prioritize clarity, efficiency, and minimal cognitive load.
This means reducing unnecessary clicks, providing clear visual cues, and presenting information in a concise and easily digestible format. The system should anticipate user needs and proactively offer relevant information and assistance, minimizing the need for extensive searching or manual input.
Interface Design Details
The proposed interface will feature a modular design, allowing for customization based on individual user roles and preferences. The central workspace will display a dynamic, context-aware dashboard. This dashboard will adapt based on the user’s current task and location, showing relevant patient information, suggested documentation entries generated by the AI, and ambient data from connected devices. For example, if a physician is examining a patient, the dashboard will display the patient’s vitals, medical history, and AI-generated progress notes, alongside a visual representation of the patient’s room layout for easy access to equipment and medication information.
A clean, uncluttered layout using a consistent color scheme will ensure visual clarity. Large, easily clickable buttons and icons will facilitate quick access to frequently used functions. Voice-activated commands will supplement the visual interface, enabling hands-free operation in situations where typing is impractical. The system will also incorporate customizable keyboard shortcuts for experienced users. Error prevention features, such as intelligent input suggestions and real-time validation, will minimize the risk of data entry errors.
The Importance of User Feedback
User feedback is crucial throughout the entire design and development process. Iterative design, incorporating user feedback at each stage, ensures that the system meets the actual needs and expectations of healthcare professionals. This feedback can be collected through various methods, including usability testing, surveys, and in-depth interviews. Early and continuous user involvement can identify usability issues and potential areas for improvement early on, saving time and resources in the long run.
For example, feedback from nurses might reveal that the system’s voice-recognition capabilities are insufficient for noisy hospital environments, leading to improvements in noise cancellation and speech recognition algorithms. Physician feedback could highlight the need for more detailed AI-generated summaries of complex medical cases.
Integrating Generative AI into Existing EHR Systems
There are several approaches to integrating generative AI into existing EHR systems. One approach is to develop a standalone application that interacts with the EHR system via APIs. This approach offers greater flexibility but requires careful management of data security and interoperability. Another approach is to directly integrate the AI capabilities into the existing EHR software, which requires more extensive modification but could provide a more seamless user experience.
A third approach involves creating a modular add-on that can be easily integrated into various EHR systems, providing a more versatile solution. The choice of integration approach will depend on factors such as the specific EHR system, the level of customization required, and the resources available. For instance, a large hospital system with a highly customized EHR might opt for direct integration, while a smaller clinic might prefer a modular add-on for greater flexibility.
Visual Representation of the User Interface
Imagine a large central screen displaying a patient’s name, age, and relevant medical history in a clear, concise format. To the right, a smaller window displays real-time vital signs from connected monitors. Below the main patient information, a section displays AI-generated progress notes, with options to edit or accept the suggestions. On the left-hand side, a menu provides quick access to frequently used functions, such as ordering tests, prescribing medication, and accessing patient imaging.
The overall color scheme is calming and professional, using a light gray background with blue accents. Icons are clear and easily identifiable, and text is large and easy to read. A small, unobtrusive voice command icon sits in the bottom right corner. The entire interface is designed to be clean, uncluttered, and easy to navigate, prioritizing efficiency and minimizing cognitive load.
Future Directions and Innovations
Generative AI’s impact on healthcare is just beginning. While its current applications in clinical documentation and ambient assist are transformative, the potential extends far beyond these initial steps. We’re on the cusp of a revolution where AI can personalize care, accelerate research, and ultimately improve patient outcomes in ways we can only begin to imagine. This section explores some of the most promising avenues for future development and innovation.
The integration of generative AI into healthcare is not simply about automating tasks; it’s about augmenting human capabilities and creating a more efficient, effective, and personalized healthcare system. The key to unlocking its full potential lies in addressing concerns around trust and transparency, and fostering further research into its capabilities and limitations.
Next-gen healthcare is all about streamlining workflows, and generative AI for clinical documentation and ambient assist is a huge step forward. This tech is transforming how we handle patient data, and to see how leading companies are approaching this, check out this article on Salesforce Healthcare AI and Sean Kennedy’s insights salesforce healthcare ai sean kennedy. Ultimately, these advancements in AI will help us focus more on patient care, freeing up time spent on administrative tasks.
Explainable AI (XAI) and Building Trust
Explainable AI is crucial for building trust and acceptance of AI-powered healthcare systems. Patients and clinicians need to understand how AI arrives at its conclusions and recommendations. Without transparency, it’s difficult to identify and correct errors, leading to hesitation in adoption. XAI techniques, such as providing clear visualizations of the decision-making process or offering natural language explanations of AI-generated reports, are essential for bridging this trust gap.
For instance, an XAI system might explain a diagnosis not only by stating the diagnosis but also by highlighting the specific medical images and patient data points that contributed to the conclusion. This level of transparency empowers clinicians to validate the AI’s findings and increases patient confidence in the treatment plan.
Future Research and Development Areas
The following areas require further research and development to fully realize the potential of generative AI in healthcare:
Significant advancements are needed to ensure the responsible and ethical implementation of generative AI in healthcare. This involves careful consideration of data privacy, bias mitigation, and the potential for unintended consequences.
- Improved Data Handling and Privacy: Developing robust methods for handling sensitive patient data while maintaining privacy and complying with regulations like HIPAA is paramount. This includes exploring federated learning techniques to train AI models on decentralized datasets without compromising patient confidentiality.
- Bias Mitigation in AI Models: Generative AI models can inherit biases present in the training data, leading to unfair or discriminatory outcomes. Research is needed to develop methods for identifying and mitigating these biases, ensuring equitable access to AI-powered healthcare.
- Enhanced Model Robustness and Generalizability: Current generative AI models may struggle with out-of-distribution data or unexpected inputs. Improving their robustness and generalizability is critical for reliable performance in diverse clinical settings.
- Integration with Electronic Health Records (EHRs): Seamless integration of generative AI with existing EHR systems is essential for efficient data access and clinical workflow optimization. This includes developing standardized APIs and data formats.
- Human-AI Collaboration Frameworks: Research should focus on designing effective human-AI collaboration frameworks that leverage the strengths of both clinicians and AI systems, optimizing clinical decision-making.
Personalized Healthcare Delivery and Improved Patient Outcomes
Generative AI has the potential to revolutionize personalized healthcare by tailoring treatments and interventions to individual patient needs. For example:
By leveraging patient data, including medical history, genetic information, and lifestyle factors, generative AI can create personalized treatment plans, predict potential health risks, and develop targeted preventative strategies. This approach moves away from a one-size-fits-all approach towards a more precise and effective model of care.
- Personalized Drug Discovery: Generative AI can accelerate drug discovery by designing novel molecules with specific therapeutic properties, potentially leading to more effective and safer treatments for various diseases.
- Predictive Modeling for Disease Risk: AI can analyze patient data to identify individuals at high risk of developing specific diseases, enabling proactive interventions and early treatment. For example, predicting the likelihood of a heart attack based on a patient’s lifestyle, genetic predisposition, and medical history.
- Personalized Treatment Recommendations: Generative AI can analyze a patient’s unique characteristics to recommend the most appropriate treatment options, maximizing efficacy and minimizing side effects. This could involve recommending specific dosages, therapies, or combinations of treatments.
- Improved Patient Engagement: AI-powered chatbots and virtual assistants can provide personalized support and education to patients, improving adherence to treatment plans and promoting better health outcomes. Imagine a chatbot that reminds a patient to take their medication, answers their questions about their condition, and provides tailored lifestyle advice.
Final Thoughts
The integration of generative AI and ambient assist technology in healthcare represents a giant leap forward, promising to revolutionize clinical documentation and patient care. While challenges related to data privacy, accuracy, and ethical considerations remain, the potential benefits – improved efficiency, reduced burnout, and enhanced patient experiences – are too significant to ignore. The future of healthcare is undeniably intertwined with AI, and the journey we’ve begun promises a future where technology empowers both clinicians and patients to achieve better health outcomes.
Questions Often Asked
What are the biggest privacy concerns with using AI in healthcare?
Protecting patient data is paramount. Robust anonymization techniques, secure data storage, and adherence to strict regulations like HIPAA are crucial to mitigate privacy risks.
How accurate is AI-generated clinical documentation?
Accuracy depends heavily on the quality of the training data and the AI model used. Continuous monitoring, validation, and human oversight are essential to ensure accuracy and reliability.
Will AI replace doctors?
No, AI is meant to augment, not replace, healthcare professionals. It will handle time-consuming tasks, allowing clinicians to focus on complex patient interactions and critical decision-making.
What is the cost of implementing this technology?
The cost varies depending on the scale of implementation and the specific AI solutions chosen. Initial investment will be significant, but potential long-term cost savings through increased efficiency should be considered.