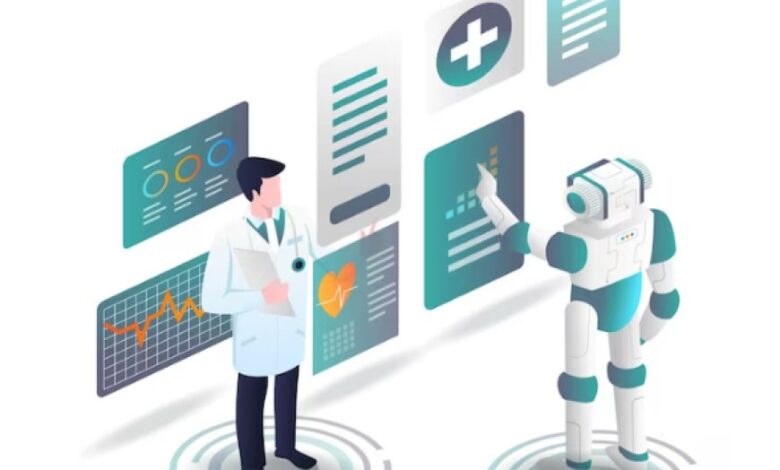
Notable Artificial Intelligence Agents in Healthcare
Notable artificial intelligence agents healthcare – Notable artificial intelligence agents in healthcare are revolutionizing the medical field, offering unprecedented opportunities to improve diagnosis, treatment, and drug discovery. From analyzing medical images to personalizing treatment plans based on individual genetic profiles, AI is rapidly becoming an indispensable tool for healthcare professionals. This exploration delves into the various types of AI agents, their applications, benefits, and ethical considerations, painting a picture of both the incredible potential and the challenges that lie ahead.
We’ll examine specific examples of AI agents already making a tangible impact, discuss the underlying technologies powering them, and explore the future trajectory of AI in healthcare, including the exciting advancements in natural language processing and machine learning. We’ll also address the crucial ethical questions surrounding data privacy, algorithmic bias, and the potential societal implications of widespread AI adoption.
Get ready for a fascinating journey into the world of AI and its transformative role in healthcare!
Types of AI Agents in Healthcare
The integration of artificial intelligence (AI) into healthcare is rapidly transforming how we diagnose, treat, and manage diseases. AI agents, specifically, are playing increasingly crucial roles, offering sophisticated solutions to complex challenges. Understanding the various types of AI agents currently deployed is key to appreciating their impact and potential.
AI Agent Types in Healthcare
Several distinct types of AI agents are revolutionizing healthcare. These agents leverage various AI techniques, including machine learning, deep learning, and natural language processing, to perform specific tasks and improve healthcare delivery. Their functionalities and applications vary considerably.
Categorization and Comparison of AI Agents
The following table provides a comparative overview of five key types of AI agents in healthcare, highlighting their functions, applications, and examples.
Agent Type | Function | Application | Example |
---|---|---|---|
Diagnostic AI Agents | Analyze medical images (X-rays, CT scans, MRIs) and patient data to assist in diagnosis. | Early detection of diseases like cancer, faster diagnosis of conditions like pneumonia, and improved accuracy in identifying abnormalities. | IBM Watson Oncology, which analyzes patient data and medical literature to suggest treatment options. |
Robotic Surgery Assistants | Assist surgeons during minimally invasive procedures, offering enhanced precision and control. | Performing complex surgeries with greater accuracy and less invasiveness, leading to faster recovery times and reduced complications. | The da Vinci Surgical System, a robotic surgical system used in various procedures. |
Virtual Assistants/Chatbots | Provide patients with information, schedule appointments, and answer basic medical questions. | Improving patient engagement, reducing administrative burden on healthcare providers, and providing 24/7 access to information. | Babylon Health, a chatbot that provides medical advice and triages patients. |
Predictive Analytics Agents | Analyze patient data to predict the likelihood of future health events, such as hospital readmissions or disease progression. | Identifying patients at high risk of adverse events, allowing for proactive interventions and improved resource allocation. | Systems that predict the risk of hospital readmissions based on patient demographics, medical history, and treatment received. |
Drug Discovery and Development Agents | Accelerate the process of identifying and developing new drugs and therapies by analyzing vast datasets of biological information. | Reducing the time and cost associated with drug development, leading to faster availability of new treatments. | AI-powered platforms used by pharmaceutical companies to identify potential drug candidates and predict their efficacy. |
Benefits and Limitations of AI Agents
Each type of AI agent offers unique benefits but also presents certain limitations. For instance, diagnostic AI agents can significantly improve diagnostic accuracy and speed, but they require extensive training data and may not be suitable for all types of medical images. Robotic surgery assistants enhance surgical precision but require significant investment and specialized training. Virtual assistants improve patient access to information but may not be able to handle complex medical queries.
Predictive analytics agents can improve resource allocation but raise concerns about data privacy and potential biases in the algorithms. Finally, drug discovery agents accelerate the process of developing new treatments, but the validation of AI-generated predictions remains crucial. Careful consideration of these benefits and limitations is essential for responsible implementation.
AI Agents in Diagnosis and Treatment
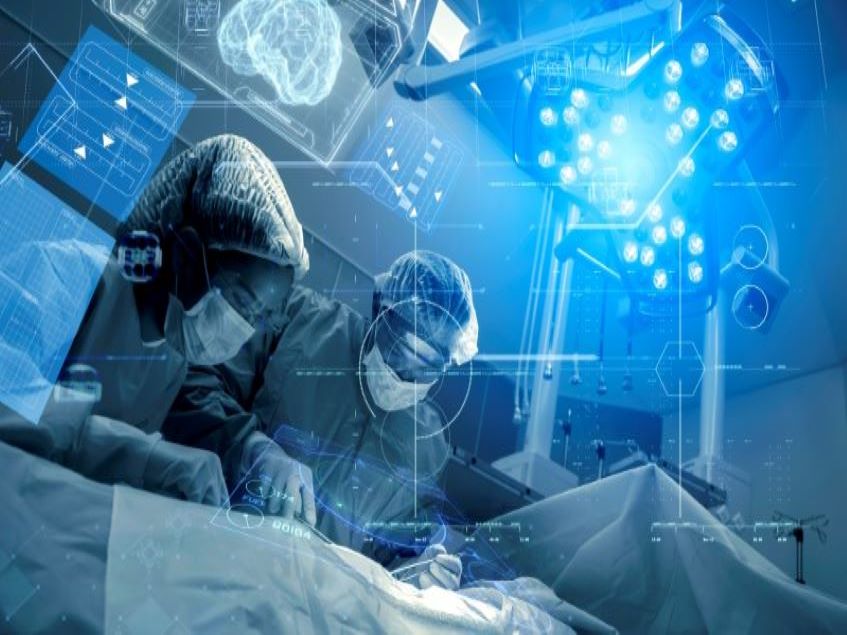
Source: aiasiainsights.com
The integration of artificial intelligence (AI) agents into healthcare is rapidly transforming diagnostic procedures and treatment strategies, leading to more accurate diagnoses, personalized treatment plans, and improved patient outcomes. These AI systems leverage machine learning algorithms to analyze vast amounts of medical data, identifying patterns and insights that might be missed by human clinicians. This enhanced capability promises to revolutionize healthcare, particularly in areas where early and accurate diagnosis is crucial.AI agents are proving invaluable in enhancing diagnostic accuracy across numerous medical specialties.
By analyzing medical images (like X-rays, CT scans, and MRIs), pathology slides, and electronic health records (EHRs), AI algorithms can detect subtle anomalies that might be overlooked by the human eye. This leads to faster and more accurate diagnoses, ultimately improving treatment outcomes and potentially saving lives.
AI-Enhanced Diagnostic Accuracy
The application of AI in diagnostic imaging is particularly noteworthy. For instance, in radiology, AI algorithms are trained on massive datasets of medical images, learning to identify patterns associated with various diseases. This allows them to detect subtle signs of cancer, such as small nodules in lung scans, often earlier than human radiologists. Similarly, in pathology, AI can analyze microscopic images of tissue samples, assisting pathologists in identifying cancerous cells or other abnormalities with greater accuracy and speed.
In cardiology, AI can analyze electrocardiograms (ECGs) to detect arrhythmias and other heart conditions with higher sensitivity and specificity than traditional methods. These are just a few examples of how AI is revolutionizing diagnostic accuracy across different medical fields.
Personalized Treatment Plans via AI
AI agents are also playing a significant role in personalizing treatment plans. By analyzing a patient’s genetic information, medical history, lifestyle factors, and other relevant data, AI can predict the likelihood of treatment success and tailor treatment strategies to maximize benefits and minimize risks. For example, in oncology, AI can help determine the optimal chemotherapy regimen based on a patient’s tumor characteristics and genetic profile.
In cardiology, AI can personalize treatment plans for heart failure patients based on their individual risk factors and response to previous treatments. This personalized approach improves treatment efficacy and reduces adverse events.
AI Agent Assisting in a Complex Case: A Hypothetical Scenario, Notable artificial intelligence agents healthcare
Consider a patient presenting with unusual neurological symptoms, including unexplained weakness, cognitive decline, and visual disturbances. A human neurologist, using traditional diagnostic methods, might find it challenging to pinpoint the cause. However, an AI agent, integrated into the hospital’s diagnostic system, could analyze the patient’s medical history, imaging data (MRI, CT scans), genetic information, and even environmental factors.
The AI could cross-reference this data with a vast database of medical literature and case studies, identifying rare neurological conditions that might otherwise be missed. The AI could then suggest specific diagnostic tests, prioritize them based on probability, and even suggest potential treatment options based on the identified condition and the patient’s individual characteristics. The neurologist, working in collaboration with the AI agent, would have a significantly enhanced ability to diagnose and treat this complex case efficiently and accurately, ultimately improving patient outcomes.
AI Agents in Drug Discovery and Development
The pharmaceutical industry is undergoing a significant transformation, fueled by the rapid advancements in artificial intelligence. AI agents are proving to be invaluable tools, accelerating the traditionally lengthy and expensive process of drug discovery and development. Their ability to analyze massive datasets, identify patterns, and predict outcomes is revolutionizing how new therapies are brought to market.AI agents are significantly accelerating drug discovery and development by automating tasks, analyzing complex data sets, and improving prediction accuracy.
This leads to faster timelines, reduced costs, and ultimately, more effective treatments for patients.
AI-Accelerated Drug Discovery Stages
The drug development pipeline is a complex, multi-stage process. AI agents are now being implemented across several key stages, impacting each with varying degrees of success. A visual representation would show a flowchart starting with Target Identification and progressing through Lead Optimization, Preclinical Testing, Clinical Trials, and finally, Regulatory Approval. Each stage would have branches indicating the application of AI for tasks such as data analysis, prediction modeling, and simulation.
For example, in Target Identification, AI could analyze genomic data to identify potential drug targets. In Lead Optimization, AI could predict the efficacy and safety of various candidate molecules. In Clinical Trials, AI could predict patient response and optimize trial design.
AI is revolutionizing healthcare, offering incredible potential in diagnosis and treatment. For example, AI’s ability to analyze vast datasets could lead to better understanding of neurological conditions like Tourette Syndrome, and inform the development of more effective therapies. Learning more about successful management strategies, like those outlined in this helpful article on strategies to manage Tourette Syndrome in children , is crucial.
This kind of knowledge helps inform the development of AI tools tailored to specific patient needs, ultimately leading to more personalized and effective healthcare.
Examples of Successful AI Applications in Drug Discovery
Several examples highlight the impact of AI in drug development. Atomwise, for example, used AI to identify potential treatments for Ebola and multiple sclerosis. Their AI platform rapidly screened millions of molecules, significantly reducing the time and resources needed for traditional screening methods. Exscientia, another prominent player, leveraged AI to design and optimize drug candidates, leading to the successful entry of a novel drug into clinical trials – a remarkable achievement in a relatively short timeframe compared to traditional methods.
These successes demonstrate the potential of AI to dramatically accelerate the drug development process.
AI Agents in Predicting Drug Efficacy and Safety
Predicting the efficacy and safety of a drug candidate is crucial, yet notoriously challenging. AI algorithms, trained on vast datasets of chemical structures, biological activity, and clinical trial data, are improving our ability to make these predictions. For instance, machine learning models can predict the likelihood of a drug candidate being effective against a specific disease based on its molecular properties and interactions with biological targets.
AI is revolutionizing healthcare, from diagnosis to personalized treatment. But effective treatment also relies on individual needs, which brings us to nutrition. Understanding these differences is crucial, and a fascinating article explores whether are women and men receptive of different types of food and game-changing superfoods for women , which could inform AI’s development of tailored health plans.
Ultimately, integrating nutritional data into AI healthcare agents could significantly improve patient outcomes.
Similarly, AI can identify potential side effects by analyzing the drug’s interactions with various biological systems and comparing it to known adverse events from similar compounds. This predictive capability reduces the risk of investing resources in candidates with low probability of success or high safety concerns, leading to more efficient allocation of resources and faster development of safer and more effective drugs.
One example is the use of AI to predict the potential for drug-drug interactions, a significant factor in patient safety. By analyzing existing drug interaction databases and chemical properties, AI models can flag potential interactions early in the development process, allowing for modifications or mitigating strategies to be implemented.
Ethical and Societal Implications
The integration of AI agents into healthcare promises revolutionary advancements, but this progress comes with significant ethical and societal considerations. The potential benefits are undeniable, but we must carefully navigate the complex landscape of data privacy, algorithmic bias, and potential societal disruption to ensure responsible and equitable implementation. Failing to address these concerns risks exacerbating existing inequalities and undermining public trust in both AI and the healthcare system itself.The use of AI in healthcare raises several crucial ethical dilemmas.
Perhaps the most pressing is the issue of data privacy. AI agents require vast amounts of patient data to function effectively, raising concerns about the security and confidentiality of sensitive medical information. Breaches could have devastating consequences for individuals and erode public confidence in the use of AI in healthcare. Furthermore, algorithmic bias, where AI systems reflect and amplify existing societal biases, presents a significant challenge.
If training data is skewed, the AI agent’s diagnoses, treatment recommendations, and even drug development efforts may disproportionately affect certain demographic groups, leading to unfair or discriminatory outcomes.
Data Privacy and Security Concerns
The sheer volume of sensitive patient data required to train and operate AI agents necessitates robust security measures. Data breaches, even minor ones, could expose private medical information, leading to identity theft, discrimination, or even physical harm. Therefore, stringent data encryption, access control, and auditing protocols are paramount. Furthermore, transparent data governance frameworks, outlining data usage, storage, and disposal policies, are crucial to build and maintain public trust.
The implementation of differential privacy techniques, which add noise to individual data points while preserving aggregate trends, can also help mitigate privacy risks. For example, a hospital implementing an AI system for predicting patient readmissions needs to guarantee that individual patient data is anonymized or pseudonomized to prevent identification and protect patient privacy.
Algorithmic Bias and Fairness
AI algorithms are only as good as the data they are trained on. If the training data reflects existing societal biases – for example, underrepresentation of certain racial or ethnic groups – the resulting AI agent may perpetuate and even amplify these biases in its diagnoses, treatment recommendations, or drug development. This can lead to disparities in healthcare access and quality, disproportionately affecting vulnerable populations.
Mitigating algorithmic bias requires careful curation of training data, employing techniques to detect and correct bias, and ongoing monitoring of the AI agent’s performance across different demographic groups. For instance, an AI system used to assess the risk of heart disease might misclassify individuals from a specific ethnic group if the training data predominantly includes data from another group.
Societal Impacts: Job Displacement and Access to Care
The widespread adoption of AI agents in healthcare could lead to job displacement for some healthcare professionals. While AI is unlikely to completely replace human clinicians, it could automate certain tasks, potentially leading to workforce restructuring. However, AI could also create new job roles focused on AI development, maintenance, and ethical oversight. The key is to manage the transition strategically, providing retraining and upskilling opportunities for affected workers.
Simultaneously, AI has the potential to significantly improve access to healthcare, particularly in underserved areas where healthcare professionals are scarce. Telemedicine powered by AI agents can bring expert medical advice to remote locations, and AI-powered diagnostic tools can improve the efficiency and accuracy of healthcare delivery. For example, an AI-powered chatbot could provide initial triage and support to patients in remote areas, improving access to healthcare while freeing up human clinicians to focus on more complex cases.
AI is revolutionizing healthcare, from diagnostics to personalized medicine. But the accessibility of these advancements is crucial, and that’s where initiatives like the partnership between Humana and Walmart, offering primary care through humana centerwell primary care centers walmart , become significant. These accessible locations could greatly impact how quickly AI-driven healthcare solutions reach patients, ultimately accelerating the benefits of notable artificial intelligence agents in healthcare.
Regulatory Frameworks and Guidelines
To ensure the responsible development and deployment of AI agents in healthcare, robust regulatory frameworks and guidelines are essential. These should address data privacy, algorithmic bias, transparency, accountability, and patient safety.
- Data Privacy and Security Standards: Strict regulations governing the collection, storage, use, and disposal of patient data are necessary, incorporating principles of data minimization, purpose limitation, and accountability.
- Algorithmic Transparency and Explainability: Regulations should require developers to explain how their AI agents make decisions, enabling scrutiny and identification of potential biases.
- Bias Detection and Mitigation: Guidelines should mandate the use of techniques to detect and mitigate algorithmic bias, ensuring fairness and equity in healthcare delivery.
- Independent Audits and Oversight: Regular independent audits of AI systems should be conducted to verify compliance with regulations and identify potential risks.
- Liability and Accountability Frameworks: Clear guidelines are needed to determine liability in cases of AI-related errors or harm, ensuring accountability for both developers and healthcare providers.
- Ethical Review Boards: Establishment of specialized ethical review boards to evaluate the ethical implications of AI applications in healthcare is crucial.
Future Trends and Challenges
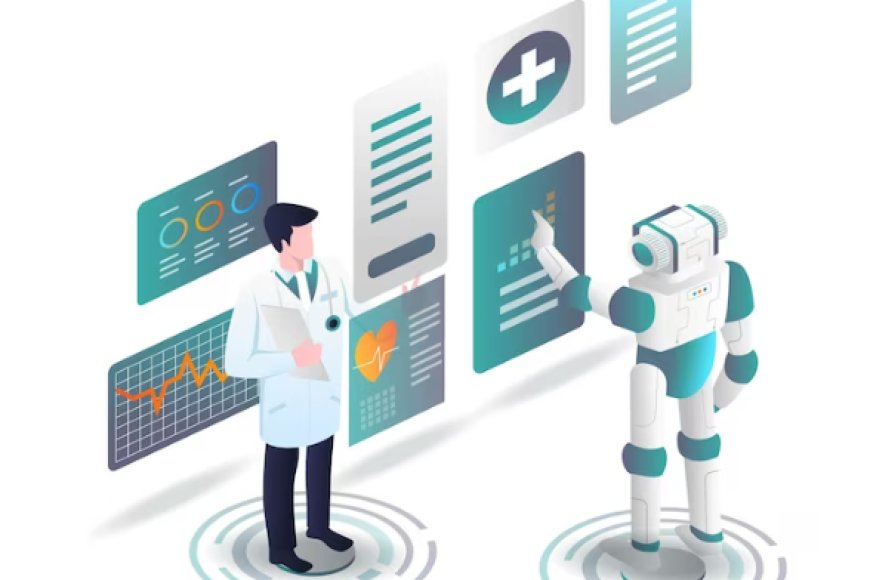
Source: ashokveda.com
The next five to ten years promise a dramatic reshaping of healthcare through the continued advancement and wider adoption of AI agents. We’re moving beyond the initial stages of experimentation towards a future where AI seamlessly integrates into clinical workflows, improving efficiency, accuracy, and patient outcomes. However, significant hurdles remain before this vision becomes a reality.The trajectory of AI agent development in healthcare will be marked by increased specialization and integration.
Instead of general-purpose AI, we’ll see a rise in AI agents tailored to specific medical specialties, such as oncology, cardiology, or radiology. These specialized agents will leverage vast amounts of data specific to their domain, leading to more accurate diagnoses and personalized treatment plans. Furthermore, we can expect greater interoperability between different AI agents and existing healthcare information systems, creating a more cohesive and efficient healthcare ecosystem.
For example, an AI agent specializing in radiology could seamlessly share findings with an AI agent managing a patient’s overall care plan.
Technological Challenges Hindering Wider Adoption
Several key technological challenges need to be addressed for wider AI agent adoption. Data availability and quality remain significant obstacles. AI algorithms thrive on large, high-quality datasets, but access to such data is often limited by privacy concerns, data silos, and inconsistencies in data formats across different healthcare systems. Furthermore, the development of robust and explainable AI models is crucial for building trust among clinicians and patients.
“Black box” AI models, where the decision-making process is opaque, are difficult to validate and may not be readily accepted in healthcare settings where transparency and accountability are paramount. Finally, ensuring the security and privacy of sensitive patient data is essential to prevent breaches and maintain public trust. This requires the implementation of strong cybersecurity measures and adherence to strict data privacy regulations.
Impact of NLP and Machine Learning Advancements
Advancements in natural language processing (NLP) will dramatically improve the communication and interaction between AI agents and healthcare professionals. NLP-powered agents will be able to process and understand complex medical records, research papers, and patient communications more accurately and efficiently. This will facilitate more informed decision-making, personalized medicine, and improved patient care. For example, an NLP-powered AI agent could analyze a patient’s medical history and current symptoms to quickly identify potential diagnoses and suggest appropriate treatment options.Simultaneously, advancements in machine learning, particularly deep learning, will enhance the predictive capabilities of AI agents.
More sophisticated algorithms will be able to analyze vast datasets to identify patterns and predict patient outcomes with greater accuracy. This will enable proactive interventions, early disease detection, and personalized risk assessment. For instance, an AI agent trained on a large dataset of patient records could predict the likelihood of a patient developing a specific condition, allowing for early preventative measures to be taken.
The combination of improved NLP and machine learning will empower AI agents to become increasingly sophisticated and indispensable tools in healthcare.
Illustrative Examples of Notable AI Agents

Source: expediensolutions.com
The field of AI in healthcare is rapidly evolving, with numerous agents demonstrating significant potential. While many are still in development or limited deployment, several have already made substantial contributions to diagnosis, treatment, and drug discovery. This section will highlight three such agents, detailing their functionalities, applications, and impact.
Notable AI Agents in Healthcare
Below is a table summarizing three notable AI agents and their contributions. These agents represent different approaches and applications within the broader field of AI-driven healthcare. Note that the rapid pace of development means newer, more impactful agents may emerge soon.
Agent Name | Function | Application Area | Impact |
---|---|---|---|
IBM Watson Oncology | Provides evidence-based treatment recommendations for cancer patients by analyzing patient data and medical literature. | Oncology (Cancer Treatment) | Improved treatment planning, potentially leading to better patient outcomes and reduced treatment-related side effects. Has assisted oncologists in making more informed decisions, although its widespread adoption and overall impact remain subjects of ongoing research and debate. |
PathAI | Uses deep learning algorithms to analyze pathology images, assisting pathologists in diagnosing diseases like cancer. | Pathology (Disease Diagnosis) | Increased diagnostic accuracy and efficiency, potentially reducing diagnostic errors and improving patient care. Helps standardize pathology readings and potentially reduces the workload on pathologists, allowing them to focus on more complex cases. |
Atomwise | Employs AI to discover and design novel drug molecules. | Drug Discovery and Development | Accelerated drug discovery process, leading to the identification of potential drug candidates for various diseases more quickly and cost-effectively than traditional methods. Has shown success in identifying potential treatments for various diseases, including Ebola and other viral infections. |
Underlying Algorithms and Technologies
The AI agents described above leverage various algorithms and technologies. IBM Watson Oncology utilizes natural language processing (NLP) to understand medical literature and patient data, combined with machine learning algorithms to generate treatment recommendations. PathAI relies heavily on deep learning convolutional neural networks (CNNs) to analyze and interpret complex medical images. Atomwise employs a combination of deep learning and other computational techniques to model molecular interactions and predict the efficacy of potential drug candidates.
These agents often incorporate large datasets of medical information, including patient records, medical images, and research publications, to train their algorithms and improve their performance. The specific algorithms and technologies employed are constantly evolving, with ongoing research focusing on improving accuracy, efficiency, and robustness.
Ultimate Conclusion: Notable Artificial Intelligence Agents Healthcare
The integration of artificial intelligence agents into healthcare is undeniably reshaping the landscape of medicine. While challenges remain, particularly in addressing ethical concerns and ensuring equitable access, the potential benefits are immense. From faster and more accurate diagnoses to personalized treatments and accelerated drug discovery, AI is poised to revolutionize how we approach healthcare, leading to improved patient outcomes and a more efficient, effective healthcare system.
The future holds exciting possibilities, but responsible development and deployment are paramount to ensure that AI benefits all of humanity.
FAQs
What are the biggest risks associated with using AI in healthcare?
Major risks include data breaches compromising patient privacy, algorithmic bias leading to unfair or inaccurate diagnoses, and the potential for job displacement among healthcare professionals.
How can we ensure fairness and avoid bias in AI-driven healthcare decisions?
Rigorous testing, diverse datasets representing all populations, and ongoing monitoring for bias are crucial. Transparency in algorithms and explainable AI are also vital steps.
Will AI replace doctors and nurses?
No, AI is designed to augment, not replace, human healthcare professionals. It will assist doctors and nurses, improving their efficiency and accuracy, but human expertise and empathy will remain essential.
What is the role of regulation in the development of AI in healthcare?
Regulations are crucial to ensure safety, ethical considerations, and patient data privacy. Clear guidelines and oversight are necessary to promote responsible innovation and prevent misuse.