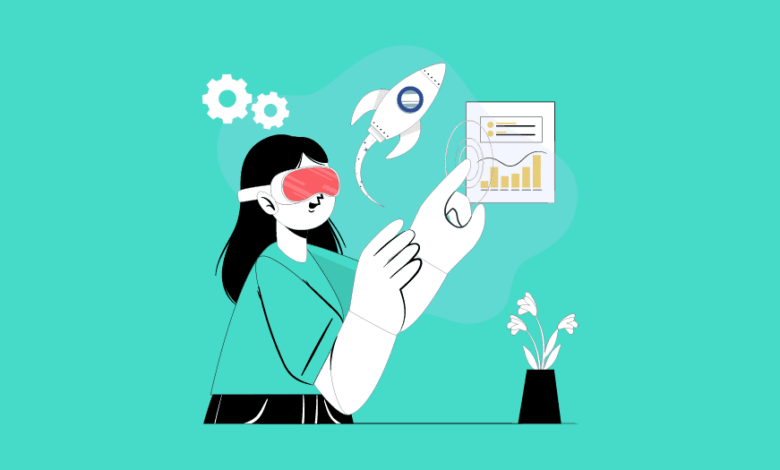
Placing AI at the Heart of Healthcare
Placing AI at the heart of healthcare isn’t just a futuristic fantasy; it’s a rapidly unfolding reality transforming how we diagnose, treat, and manage illnesses. From AI-powered diagnostic tools that speed up detection to personalized treatment plans tailored to individual genetic profiles, the potential is breathtaking. This revolution promises faster, more accurate diagnoses, leading to better patient outcomes and a more efficient healthcare system overall.
But it’s not without its challenges; ethical considerations, data privacy, and algorithmic bias are crucial aspects we must address as we integrate this powerful technology.
This blog post delves into the exciting possibilities and the critical challenges of using artificial intelligence in healthcare. We’ll explore its applications in various medical fields, discuss the ethical implications, and look at the future of AI’s role in improving global health. Get ready for a deep dive into a world where algorithms are collaborating with doctors to improve the lives of patients everywhere.
AI-Driven Diagnostics and Treatment
The integration of artificial intelligence (AI) into healthcare promises a revolution in diagnostics and treatment, offering the potential to significantly improve accuracy, speed, and personalization of care. AI algorithms, trained on vast datasets of medical images, patient records, and research literature, are rapidly transforming how we diagnose and treat diseases. This shift towards AI-driven healthcare is not merely about automation; it’s about augmenting human expertise, enabling clinicians to make more informed decisions and ultimately, deliver better patient outcomes.AI’s potential in improving the accuracy and speed of medical diagnoses is substantial.
By analyzing complex medical data far more quickly than a human, AI can identify subtle patterns and anomalies that might be missed by the human eye. This leads to faster diagnosis and, in many cases, earlier intervention.
AI Applications in Medical Specialties
AI is finding applications across a wide range of medical specialties. In radiology, AI algorithms are used to detect tumors in mammograms, CT scans, and MRIs with remarkable accuracy. In pathology, AI assists in identifying cancerous cells in tissue samples, improving the consistency and speed of diagnoses. In ophthalmology, AI can detect diabetic retinopathy from retinal images, helping to prevent vision loss.
Cardiologists are utilizing AI to analyze electrocardiograms (ECGs) and identify potential cardiac events. These are just a few examples of the many ways AI is enhancing diagnostic capabilities.
Personalized Treatment Plans Based on Patient Data
AI’s ability to process and analyze large volumes of patient-specific data, including genomic information, opens up exciting possibilities for personalized medicine. AI algorithms can analyze a patient’s medical history, genetic profile, lifestyle factors, and other relevant data to predict the likelihood of developing certain diseases and to tailor treatment plans accordingly. This personalized approach moves beyond a “one-size-fits-all” model, leading to more effective treatments and reduced side effects.For example, AI can help oncologists select the most effective chemotherapy regimen for a cancer patient based on their tumor’s genetic profile.
In diabetes management, AI algorithms can analyze continuous glucose monitor data to optimize insulin delivery, preventing hypoglycemic episodes and improving overall glycemic control. Similarly, in mental health, AI can personalize treatment plans by identifying specific symptoms and recommending appropriate interventions.
AI-Assisted Diagnostics: A Hypothetical Scenario
The following scenario illustrates how AI-assisted diagnostics could lead to earlier disease detection and improved patient outcomes.
Patient Presentation | AI Diagnostic Input | Traditional Diagnostic Approach | Outcome Comparison |
---|---|---|---|
A 50-year-old woman presents with persistent fatigue, unexplained weight loss, and mild abdominal discomfort. | AI analysis of blood tests and imaging data flags a high probability of pancreatic cancer, suggesting further investigation. | Initial blood tests and imaging might not reveal clear indicators of pancreatic cancer, leading to further investigations only after more pronounced symptoms. | AI-assisted diagnosis leads to earlier detection, enabling timely intervention and potentially improving survival rates. Traditional approach might result in delayed diagnosis and reduced treatment efficacy. |
AI in Drug Discovery and Development
The pharmaceutical industry is undergoing a significant transformation, driven by the integration of artificial intelligence (AI). AI’s ability to analyze vast datasets and identify complex patterns is revolutionizing the traditionally lengthy and expensive drug discovery and development process, offering the potential to bring life-saving medications to market faster and more efficiently. This shift promises to address unmet medical needs and improve patient outcomes globally.
AI’s Role in Accelerating Drug Discovery
AI accelerates drug discovery by automating and optimizing various stages of the process. It can analyze massive amounts of biological data, identify potential drug targets, design novel drug molecules, predict their efficacy and safety, and even personalize treatment strategies. This speed and efficiency are crucial in reducing the time and cost associated with bringing new drugs to market, a process that typically takes over a decade and billions of dollars.
Stages of Drug Development Where AI is Applied
AI’s influence spans the entire drug development pipeline. From the initial identification of potential drug targets to the final stages of clinical trials, AI algorithms are proving invaluable.
Imagine a future where AI streamlines every aspect of healthcare, from diagnosis to treatment. Even with setbacks like the recent news about Walmart Health’s closure, as reported in this article, despite Walmart Health’s closure, the company healthcare destination Scott Bowman , the potential for AI remains huge. This innovative technology could help overcome challenges and ultimately improve patient care, making healthcare more efficient and accessible for everyone.
- Target Identification and Validation: AI can analyze genomic data, proteomics data, and other biological information to identify promising drug targets – specific molecules or pathways involved in disease processes. This process, traditionally relying on extensive laboratory work, is significantly sped up by AI’s ability to sift through and analyze vast datasets.
- Lead Compound Identification and Optimization: Once a target is identified, AI can design and optimize potential drug candidates (lead compounds). AI algorithms can predict the properties of molecules, allowing researchers to screen millions of potential candidates and select the most promising ones for further development, reducing the need for extensive and time-consuming laboratory synthesis and testing.
- Preclinical Development: AI can predict the efficacy and safety of lead compounds before they enter human clinical trials. This involves using AI models to simulate the behavior of drugs in the body, predicting their absorption, distribution, metabolism, and excretion (ADME) properties, and assessing potential toxicity. This significantly reduces the risk of failure in later stages.
- Clinical Trial Design and Patient Selection: AI can analyze patient data to identify subgroups most likely to benefit from a specific treatment, improving the efficiency and success rate of clinical trials. It can also optimize trial design, reducing the time and cost required to complete the process. For example, AI can predict which patients are most likely to respond positively to a particular drug, allowing for more focused and effective clinical trials.
Comparison of Traditional and AI-Driven Drug Discovery
Traditional drug discovery relies heavily on trial-and-error experimentation, often involving lengthy laboratory processes and extensive animal testing. This approach is slow, expensive, and has a high failure rate. In contrast, AI-driven approaches leverage the power of data analysis and machine learning to predict the success or failure of drug candidates early in the development process, reducing costs and time to market.
Feature | Traditional Drug Discovery | AI-Driven Drug Discovery |
---|---|---|
Speed | Slow (10-15 years) | Faster (potential for significant reduction) |
Cost | Very high (billions of dollars) | Potentially lower |
Success Rate | Low | Potentially higher |
Data Analysis | Limited by manual analysis | Enables analysis of massive datasets |
Target Identification | Relies heavily on experimentation | Uses AI to predict and identify targets |
AI-Powered Drug Discovery Pipeline Flowchart
[A visual representation would be placed here. Imagine a flowchart with boxes representing each stage: Target Identification (box 1), Lead Compound Identification (box 2), Preclinical Development (box 3), Clinical Trials (box 4), and Regulatory Approval (box 5). Arrows connect the boxes indicating the flow of the process. Box 1 would show AI analyzing large datasets to identify targets.
Box 2 depicts AI designing and optimizing potential drug molecules. Box 3 illustrates AI predicting ADME properties and toxicity. Box 4 shows AI assisting in patient selection and trial design. Box 5 represents the final regulatory approval stage.] The entire process is iterative, with feedback loops allowing for adjustments and optimization at each stage. For example, results from preclinical development might inform adjustments to lead compound optimization, improving the chances of success in clinical trials.
AI-Enhanced Medical Imaging and Analysis
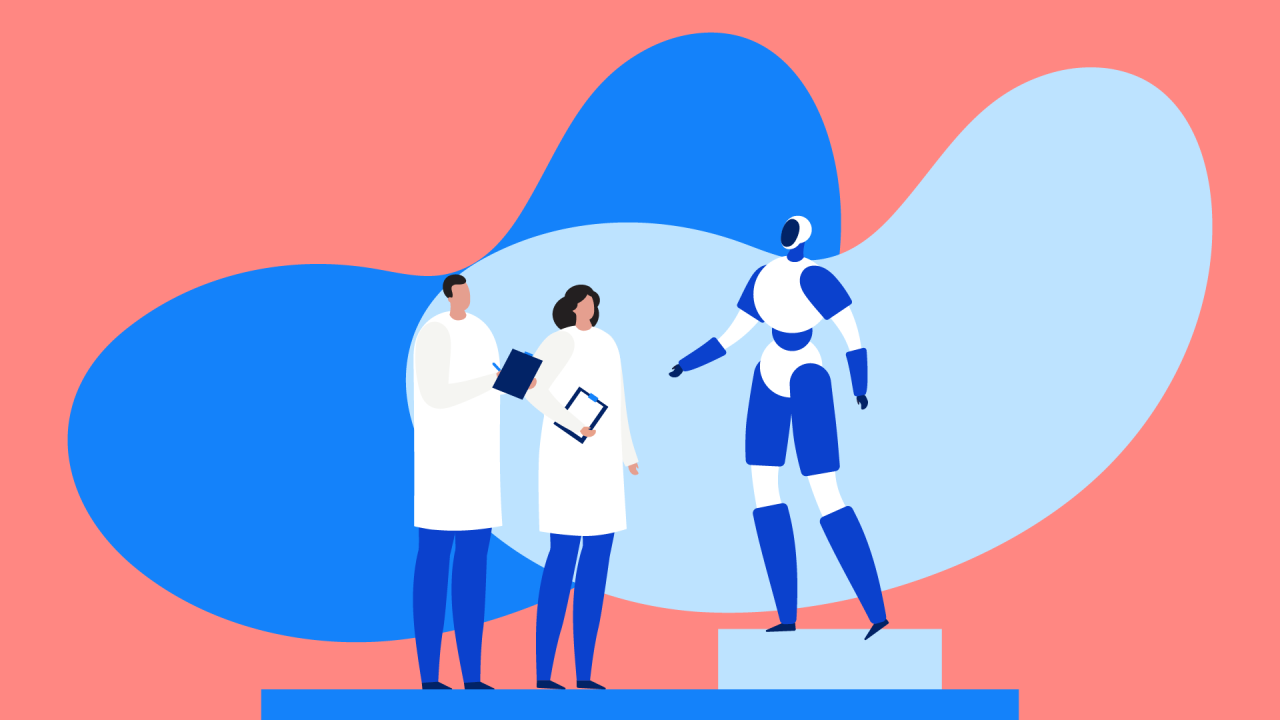
Source: healthcaretransformers.com
The integration of artificial intelligence (AI) into medical imaging is revolutionizing healthcare, promising faster, more accurate diagnoses and improved patient outcomes. AI algorithms are proving invaluable in analyzing complex medical images, assisting radiologists and pathologists, and ultimately leading to better treatment decisions. This enhanced analysis is particularly impactful in the early detection of diseases like cancer, where timely intervention is crucial.AI algorithms significantly improve the interpretation of medical images like X-rays, CT scans, and MRIs by identifying subtle patterns and anomalies often missed by the human eye.
These algorithms are trained on vast datasets of medical images, learning to recognize characteristics associated with various diseases. This allows for faster processing of images, highlighting areas of concern for radiologists and pathologists, thereby reducing diagnostic delays and improving accuracy. For instance, AI can detect minute nodules in lung scans indicative of early-stage lung cancer, often before they are visible to a human observer.
Similarly, in pathology, AI can analyze microscopic tissue samples, identifying cancerous cells with a high degree of accuracy, assisting in the grading and staging of tumors.
AI Applications in Radiology and Pathology
AI is rapidly transforming both radiology and pathology. In radiology, AI-powered systems can automatically detect and classify fractures, tumors, and other abnormalities in X-rays, CT scans, and MRIs. These systems can also quantify the size and extent of lesions, providing radiologists with quantitative data to aid in treatment planning. In pathology, AI algorithms can analyze digitized microscopic images of tissue samples, identifying cellular structures and patterns associated with various diseases.
This automated analysis can improve the efficiency and accuracy of disease diagnosis, especially in cases involving complex or subtle findings. For example, AI algorithms are being used to detect subtle changes in breast tissue indicative of early-stage breast cancer, leading to earlier diagnosis and treatment. Another example involves the analysis of blood smears for the detection of malaria parasites, offering quicker and more reliable results than manual microscopic examination.
Benefits of AI-Powered Image Analysis for Early Disease Detection
AI-powered image analysis offers substantial benefits for the early detection of cancers and other diseases. The increased accuracy and speed of AI-driven analysis allows for faster diagnosis, enabling earlier intervention and potentially improving treatment outcomes. Studies have shown that AI algorithms can detect cancers with higher sensitivity and specificity than human radiologists alone, particularly in detecting subtle lesions. This improved accuracy can lead to earlier diagnosis, when treatment is often more effective.
AI in healthcare is revolutionizing diagnostics and treatment, offering personalized medicine based on individual needs. Understanding these needs includes nutritional factors, and that’s where this fascinating article comes in: check out are women and men receptive of different types of food and game changing superfoods for women to see how dietary differences impact health outcomes. This data, integrated with AI, could further refine personalized healthcare plans, ensuring optimal patient well-being.
Moreover, the efficiency gains from AI-powered analysis allow radiologists and pathologists to handle a larger volume of cases, addressing the increasing demand for diagnostic services. For example, AI-assisted screening for diabetic retinopathy has shown significant improvements in early detection rates, preventing vision loss in many patients.
AI Assistance in Radiologists’ and Pathologists’ Workflow
AI can significantly streamline the workflow of radiologists and pathologists. Here’s how:
- Prioritization of Cases: AI algorithms can prioritize cases based on the likelihood of critical findings, allowing radiologists and pathologists to focus their attention on the most urgent cases first.
- Automated Image Preprocessing: AI can automate time-consuming tasks such as image segmentation, noise reduction, and artifact correction, freeing up radiologists and pathologists to focus on interpretation.
- Quantitative Measurements: AI can provide accurate quantitative measurements of lesions, such as size, volume, and density, aiding in treatment planning and monitoring disease progression.
- Second Opinion and Quality Control: AI can provide a second opinion on image interpretation, helping to reduce diagnostic errors and ensure consistency in diagnosis.
- Reporting and Documentation: AI can automate the generation of radiology and pathology reports, reducing administrative burden and improving efficiency.
AI-Powered Patient Monitoring and Management
AI is rapidly transforming healthcare, and one of the most promising areas is patient monitoring and management. The ability to continuously track vital signs, activity levels, and other health metrics remotely offers unprecedented opportunities to improve patient outcomes, reduce healthcare costs, and enhance the overall patient experience. This shift towards proactive, personalized care is driven by advancements in wearable sensor technology, sophisticated AI algorithms, and secure telehealth platforms.AI’s role in remote patient monitoring involves the seamless integration of various technologies to provide continuous, real-time insights into a patient’s health status.
This enables early detection of potential problems, allowing for timely interventions and preventing serious complications.
Remote Patient Monitoring Using Wearable Sensors and Telehealth Platforms
Wearable sensors, such as smartwatches and patches, continuously collect physiological data like heart rate, blood pressure, blood oxygen levels, and activity levels. This data is transmitted wirelessly to a secure cloud-based platform where AI algorithms analyze it for patterns and anomalies. For example, an AI system might detect an irregular heartbeat pattern indicative of atrial fibrillation, alerting both the patient and their healthcare provider.
Telehealth platforms then facilitate remote consultations, allowing healthcare professionals to provide timely advice, adjustments to medication, or even schedule in-person visits if necessary. This proactive approach reduces the need for frequent hospital visits, minimizing costs and improving patient comfort. A study published in the Journal of the American Medical Informatics Association showed a significant reduction in hospital readmissions among patients using remote monitoring systems incorporating AI-powered alerts for early detection of deterioration.
Another example is the use of remote monitoring for patients with chronic conditions like heart failure or diabetes, allowing for proactive management of their condition and preventing acute exacerbations that would otherwise require hospitalization.
Ethical Considerations in AI-Powered Patient Data Privacy and Security
The use of AI in patient monitoring raises important ethical considerations, particularly concerning data privacy and security. Patient health data is highly sensitive, and its unauthorized access or misuse could have severe consequences. Robust security measures are crucial to protect this data from breaches and ensure patient confidentiality. Strategies for ensuring data protection include employing strong encryption protocols, implementing strict access control mechanisms, and adhering to data privacy regulations such as HIPAA (in the US) and GDPR (in Europe).
Imagine AI revolutionizing healthcare, personalizing treatments based on individual needs. This personalized approach is crucial for conditions like Tourette Syndrome, where effective management varies greatly. For insightful strategies on managing this in children, check out this helpful resource: strategies to manage Tourette syndrome in children. Ultimately, integrating AI into healthcare means tailoring treatments like these to maximize positive outcomes for every patient.
Furthermore, obtaining informed consent from patients is paramount before collecting and using their data. Transparency about how the data will be used and who will have access to it is essential to build trust and ensure ethical data handling practices. AI systems should also be designed with built-in mechanisms to ensure fairness and avoid bias in their analysis and decision-making processes.
For instance, algorithms should be trained on diverse datasets to prevent inaccurate or discriminatory outcomes based on factors like race, gender, or socioeconomic status.
System Architecture of an AI-Powered Remote Patient Monitoring System
The system architecture can be described as follows: First, wearable sensors collect patient data (heart rate, blood pressure, etc.). This data is then transmitted wirelessly to a secure data gateway. The gateway acts as an intermediary, ensuring data security and integrity. The data is then transferred to a cloud-based data storage and processing unit. This unit employs advanced encryption and access control mechanisms.
AI algorithms, residing in the cloud, analyze the data in real-time, looking for patterns and anomalies. These algorithms can be a combination of machine learning models for anomaly detection, predictive modeling, and personalized recommendations. The results of the AI analysis are then transmitted to a user interface (UI), accessible to both patients and healthcare providers. The UI displays relevant health metrics, alerts, and recommendations.
Finally, a secure communication module enables remote consultations between patients and healthcare providers through video conferencing and messaging functionalities. This entire system is designed with security and privacy at its core, adhering to relevant regulations and ethical guidelines.
Challenges and Ethical Considerations of AI in Healthcare
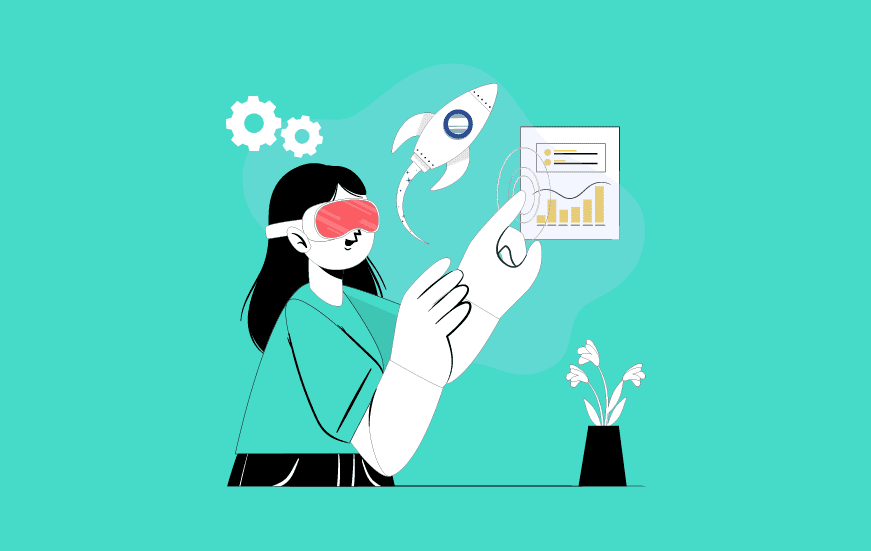
Source: datasciencecentral.com
The integration of artificial intelligence into healthcare, while promising immense benefits, presents a complex array of challenges and ethical considerations that must be carefully navigated. Successfully deploying AI in healthcare requires not only technological advancements but also a robust framework addressing data privacy, algorithmic fairness, and responsible implementation. Failure to do so risks exacerbating existing inequalities and undermining public trust.
Data Availability and Quality
The effectiveness of AI algorithms hinges critically on the quality and quantity of the data used to train them. Healthcare data is often fragmented, inconsistent, and incomplete, posing significant hurdles to developing accurate and reliable AI models. Furthermore, access to this data is frequently restricted due to privacy regulations and institutional barriers. For instance, a hospital system might have vast amounts of patient data, but sharing this across different systems to build a larger, more representative dataset is often legally and logistically difficult.
This lack of readily available, high-quality data significantly limits the development and validation of AI applications.
Algorithmic Bias and Fairness, Placing ai at the heart of healthcare
AI algorithms are trained on data, and if that data reflects existing societal biases, the algorithms will inevitably perpetuate and even amplify those biases. For example, an AI diagnostic tool trained primarily on data from one demographic group might be less accurate when applied to patients from other groups, leading to misdiagnosis and potentially harmful consequences. Addressing algorithmic bias requires careful data curation, rigorous algorithm testing across diverse populations, and ongoing monitoring for unintended discriminatory outcomes.
Transparency in algorithm design and deployment is crucial to identify and mitigate these biases.
Regulatory Hurdles and Legal Liability
The rapid advancement of AI in healthcare outpaces the development of comprehensive regulatory frameworks. The lack of clear guidelines on data privacy, algorithm validation, and liability in case of AI-related errors creates uncertainty and hinders widespread adoption. For example, determining liability when an AI-driven diagnostic tool makes an incorrect diagnosis that leads to patient harm is a complex legal issue that needs careful consideration and well-defined regulations.
This regulatory uncertainty creates significant barriers for developers and healthcare providers alike.
Algorithmic Transparency and Accountability
Many AI algorithms, particularly deep learning models, operate as “black boxes,” making it difficult to understand how they arrive at their decisions. This lack of transparency raises concerns about accountability. If an AI system makes a critical error, it can be challenging to identify the cause and hold the responsible parties accountable. Establishing mechanisms for algorithmic transparency and accountability is essential to build trust and ensure responsible AI deployment.
This might involve developing techniques for explaining AI decisions and creating clear lines of responsibility for AI-related outcomes.
Potential Benefits and Risks of AI in Healthcare
Benefit | Risk | Mitigation Strategy |
---|---|---|
Improved diagnostic accuracy | Algorithmic bias leading to inaccurate diagnoses for certain demographics | Rigorous testing on diverse datasets, ongoing monitoring for bias, and explainable AI techniques. |
Personalized medicine and treatment | Data privacy breaches and misuse of sensitive patient information | Robust data security measures, anonymization techniques, and adherence to strict privacy regulations. |
Increased efficiency and reduced costs | Job displacement for healthcare professionals | Reskilling and upskilling programs for healthcare workers, focusing on collaboration between humans and AI. |
Enhanced drug discovery and development | Lack of transparency and accountability in AI-driven decisions | Development of explainable AI models, clear lines of responsibility, and independent audits. |
Improved patient access to care | Exacerbation of existing health inequalities due to unequal access to AI-powered technologies | Equitable distribution of AI-powered tools, focusing on underserved populations, and addressing digital literacy gaps. |
Conclusion: Placing Ai At The Heart Of Healthcare
The integration of AI in healthcare is a transformative journey, one brimming with potential to revolutionize how we approach health and wellness. While challenges remain, particularly in addressing ethical concerns and ensuring equitable access, the advancements we’ve seen and continue to witness are undeniable. The future of healthcare is undoubtedly intertwined with AI, and by thoughtfully navigating the complexities, we can harness its power to create a healthier and more equitable world for all.
Essential FAQs
What are the biggest risks associated with AI in healthcare?
The biggest risks include algorithmic bias leading to inaccurate diagnoses or treatments, data breaches compromising patient privacy, and the potential for over-reliance on AI, diminishing the role of human expertise.
How can we ensure fairness and avoid bias in AI algorithms used for healthcare?
Careful data curation, diverse and representative datasets, rigorous testing and validation, and ongoing monitoring for bias are crucial steps in ensuring fairness and mitigating bias in AI algorithms.
Will AI replace doctors?
No. AI is designed to augment and assist healthcare professionals, not replace them. Doctors will remain essential for clinical judgment, patient interaction, and ethical decision-making.
What are the regulatory hurdles for implementing AI in healthcare?
Regulatory hurdles vary by country but often include issues around data privacy (GDPR, HIPAA), algorithm validation and safety, and liability in case of AI-related errors.