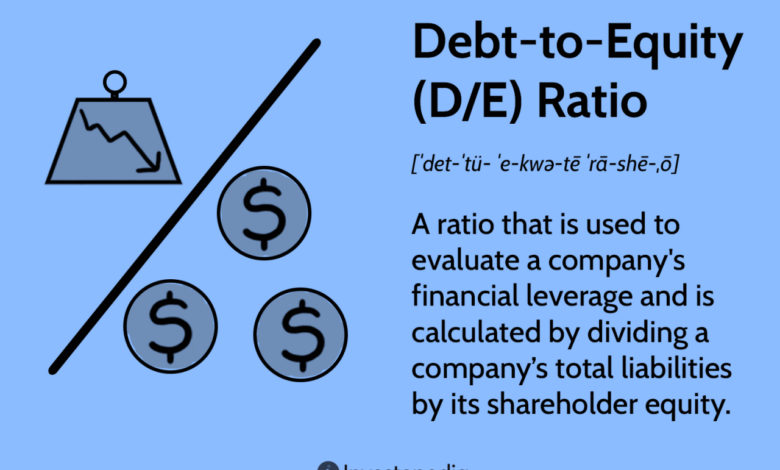
Rethinking Area Deprivation Index Srilekha Palles Health Equity Work
Rethinking area deprivation index effective measures health equity Srilekha Palle – Rethinking Area Deprivation Index: effective measures for health equity, Srilekha Palle’s groundbreaking work challenges traditional methods. We often rely on simple socioeconomic indicators to understand health disparities, but what if those measures miss the bigger picture? This post dives into Srilekha Palle’s insightful critiques and explores how a more nuanced approach can help us achieve true health equity, particularly in underserved communities.
We’ll examine her proposed improvements, explore innovative data sources, and discover how this can lead to more effective interventions and resource allocation.
Srilekha Palle’s research offers a crucial lens through which to examine existing area deprivation indices (ADIs). Her work highlights the limitations of relying solely on socioeconomic factors and proposes a multi-dimensional approach incorporating qualitative data and community perspectives. This shift is vital for understanding the complex interplay of factors contributing to health disparities, moving beyond simple correlations to a deeper understanding of the lived experiences that shape health outcomes.
Introduction to Area Deprivation Indices and Health Equity
Area deprivation indices (ADIs) are composite measures designed to quantify the relative socioeconomic disadvantage of geographic areas. They typically combine several indicators, reflecting factors like income, education, employment, housing quality, and access to resources, to create a single score representing the overall level of deprivation within a specific area. Understanding ADIs is crucial for addressing health equity because they reveal geographic variations in health outcomes linked to socioeconomic circumstances.ADIs are fundamentally linked to health equity because they highlight areas where populations experience disproportionately poorer health due to their socioeconomic circumstances.
Areas with high ADI scores often exhibit higher rates of chronic diseases, lower life expectancy, and increased infant mortality, illustrating the strong correlation between socioeconomic disadvantage and health outcomes. The aim of using ADIs is to identify these areas of need and inform targeted interventions to improve health equity.
Limitations of Traditional ADI Methodologies
Traditional ADI methodologies often suffer from several limitations that hinder their ability to accurately reflect the complex realities of health disparities. One key limitation is the reliance on aggregate data, which masks the heterogeneity within areas. For instance, a neighborhood might have a high average income, yet contain pockets of extreme poverty, which wouldn’t be captured by the overall ADI score.
This leads to an inaccurate representation of the needs of vulnerable subgroups within seemingly affluent areas. Furthermore, traditional ADIs often lack the granularity to capture the dynamic nature of deprivation, failing to account for changes in social and economic conditions over time.
Examples of ADIs Failing to Capture Nuances of Health Disparities
Consider a scenario where an ADI relies heavily on income as a measure of deprivation. A community with a high proportion of working-class individuals who rely on stable, albeit low-paying jobs, might be classified as deprived, despite having a strong sense of community and access to social support networks. This contrasts with a wealthy neighborhood where a small percentage of residents are experiencing homelessness or poverty, but the overall ADI score remains low due to the high average income.
The first community, while technically ‘deprived’ according to the ADI, might exhibit better mental health outcomes and social cohesion than the second, illustrating the inadequacy of a simplistic ADI. Similarly, ADIs often struggle to capture the impact of factors like discrimination, environmental hazards, or access to quality healthcare, which can significantly influence health disparities, even within areas with relatively high socioeconomic status.
The lack of inclusion of these crucial factors results in an incomplete picture of the complex interplay of factors influencing health equity.
Rethinking ADI
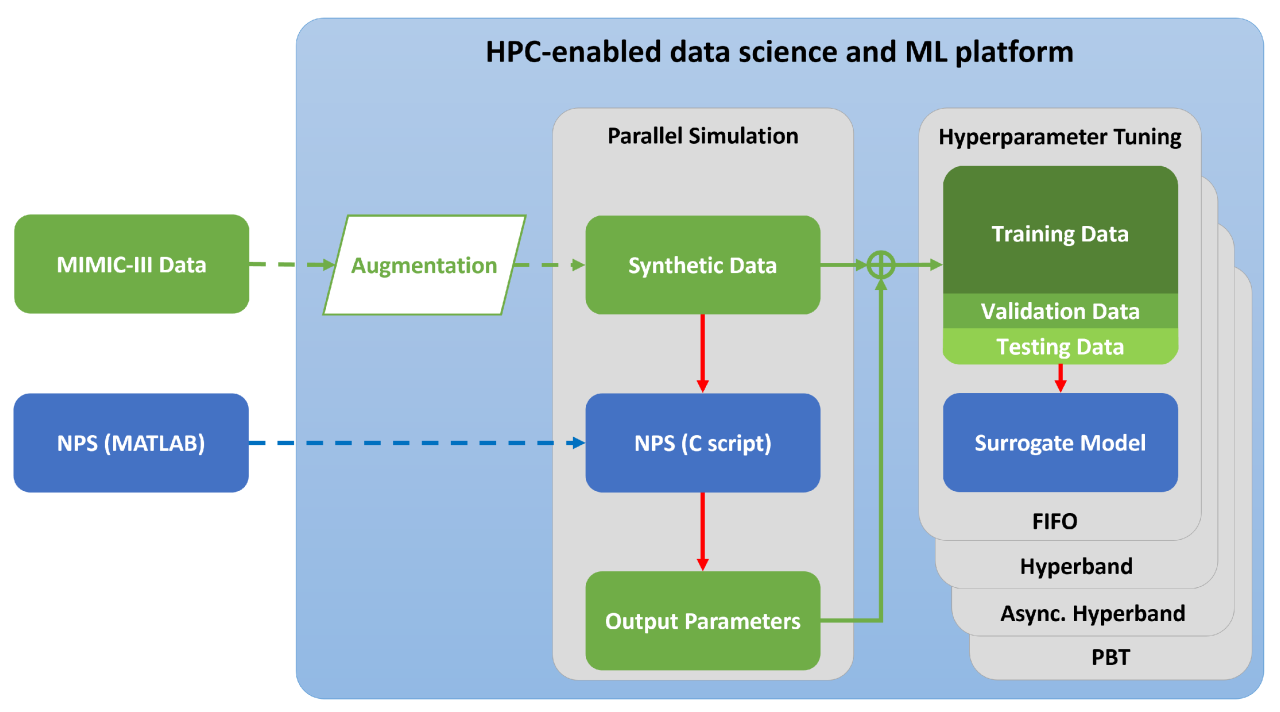
Source: mdpi.com
Area Deprivation Indices (ADIs) are crucial tools for understanding and addressing health inequalities. However, their effectiveness is constantly debated, and improvements are continually sought. Srilekha Palle’s research offers significant contributions to this ongoing conversation, challenging existing methodologies and proposing innovative approaches to better capture the complexities of deprivation and its impact on health.
Srilekha Palle’s Key Contributions to ADI Research, Rethinking area deprivation index effective measures health equity Srilekha Palle
Srilekha Palle’s work significantly advances our understanding of how ADIs can be improved to better reflect the lived experiences of marginalized communities and their relationship to health outcomes. Her research focuses on moving beyond simple aggregations of socioeconomic indicators to incorporate more nuanced and context-specific factors. This includes a critical examination of the data sources used in ADIs, highlighting biases and limitations in existing datasets.
Rethinking the Area Deprivation Index to better measure health equity, as Srilekha Palle’s work suggests, requires a multifaceted approach. Understanding the human element is crucial; improving access to healthcare often hinges on empathetic interactions. This is where technology can play a surprising role, as explored in this fascinating article on enhanced human potential how customer service technology increases empathy.
Ultimately, leveraging technology to enhance empathy could significantly improve health outcomes, especially in underserved communities, and strengthen the effectiveness of indices like the ADI.
Furthermore, her work emphasizes the importance of participatory approaches, involving community members in the design and implementation of ADIs to ensure relevance and accuracy. Her publications and presentations consistently demonstrate a commitment to developing ADIs that are both robust and equitable.
Criticisms of Existing ADIs Addressed by Srilekha Palle
Srilekha Palle’s research likely highlights several key shortcomings of traditional ADIs. These criticisms may include the limitations of relying solely on readily available administrative data, which often fails to capture the lived experiences of individuals and communities. Existing ADIs may overlook the qualitative aspects of deprivation, such as social isolation, discrimination, and lack of access to social support networks, all of which significantly impact health.
The reliance on aggregate measures may mask significant variations within areas, leading to an inaccurate representation of deprivation and its impact on health. Furthermore, the lack of cultural sensitivity in existing ADIs may lead to misclassifications and misinterpretations, particularly in diverse communities. Her work probably argues for a move away from a purely quantitative approach to a more holistic and qualitative understanding of deprivation.
Novel Approaches Proposed by Srilekha Palle for Improving ADI Measurement
Srilekha Palle likely advocates for several innovative approaches to improve ADI measurement. This may include incorporating qualitative data gathered through community engagement and participatory methods. Her research might suggest incorporating measures of social capital, access to resources beyond material wealth, and the presence of environmental hazards, such as pollution or lack of green spaces. She might also propose the development of context-specific ADIs tailored to the unique characteristics of different communities.
This could involve using mixed-methods approaches combining quantitative data with qualitative insights from community members. Finally, she might emphasize the importance of regularly updating and validating ADIs to reflect changes in social and economic conditions.
Comparison of Srilekha Palle’s Proposed Improvements with Traditional ADI Methods
Traditional ADIs typically rely on a relatively small number of readily available indicators, often focusing on income, employment, and housing. These are aggregated at a geographical level, often using census data. In contrast, Srilekha Palle’s proposed improvements emphasize a more holistic and participatory approach. This involves incorporating qualitative data to gain a deeper understanding of the lived experiences of individuals and communities, moving beyond simple quantitative measures.
Traditional ADIs are often static, reflecting a snapshot in time, whereas Srilekha Palle’s approach likely stresses the need for dynamic and regularly updated indices that reflect changing social and economic circumstances. Finally, while traditional ADIs often focus on a broad geographical area, Srilekha Palle’s work may emphasize the need for more granular, localized ADIs that better reflect variations within communities.
Data Sources and Measurement Techniques for Improved ADIs
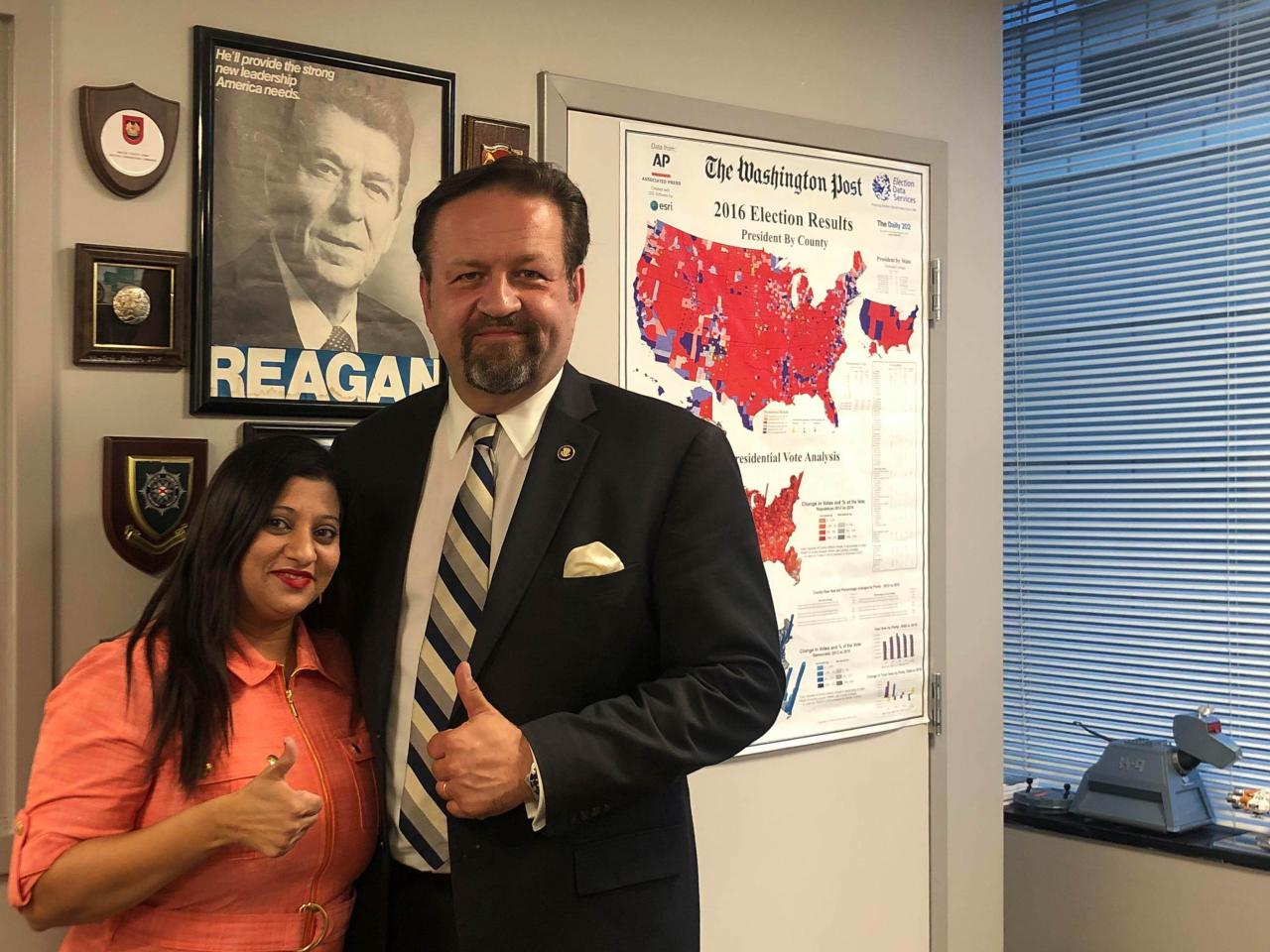
Source: fairfaxgop.org
Improving Area Deprivation Indices (ADIs) requires a multifaceted approach that moves beyond traditional socioeconomic indicators. A more comprehensive ADI should incorporate a wider range of factors influencing health equity, leading to a more nuanced and accurate representation of deprivation. This section details the data sources and methodologies needed to construct such an improved ADI.
A Multi-Dimensional Area Deprivation Index
The design of a new ADI should incorporate multiple dimensions of deprivation, moving beyond the typical focus on income and employment. A robust index needs to account for factors such as access to healthcare, environmental quality, education levels, and social capital. Weighting these factors appropriately is crucial; a simple additive approach might not reflect the relative importance of each dimension in determining health outcomes.
For example, the lack of access to clean water might have a disproportionately large impact on health compared to a slightly lower income. Therefore, a more sophisticated weighting system, perhaps based on factor analysis or other multivariate techniques, is necessary to accurately reflect the complex interplay of these factors.
Data Sources for a Comprehensive ADI
The following table Artikels potential data sources for constructing a more comprehensive ADI. The selection of sources will depend on data availability and the specific geographic area under consideration.
Source | Data Type | Relevance to Health Equity |
---|---|---|
Census Data | Demographic, Socioeconomic | High |
Healthcare Provider Data (e.g., hospital discharge records, claims data) | Health service utilization, disease prevalence | Very High |
Environmental Monitoring Data (e.g., air and water quality measurements) | Environmental risk factors | High |
Education Data (e.g., school enrollment rates, test scores) | Educational attainment, literacy | High |
Transportation Data (e.g., public transit accessibility, road infrastructure) | Access to services | Medium |
Crime Statistics | Safety and security | Medium |
Social Survey Data (e.g., community health surveys, social cohesion surveys) | Social capital, community resources | High |
Retail and Business Data | Access to goods and services | Medium |
Housing Data | Housing quality, affordability | High |
Integrating Qualitative Data into ADI Calculations
While quantitative data forms the backbone of ADI calculations, qualitative data offers valuable insights into the lived experiences of individuals and communities. Qualitative methods, such as focus groups and interviews, can reveal the nuances of deprivation that quantitative data might miss. For example, while a quantitative measure might show low income, qualitative data might reveal the impact of social isolation or lack of community support.
Integrating qualitative data requires careful consideration. One approach is to use qualitative data to inform the weighting of different factors in the quantitative ADI. Qualitative findings could also be used to validate or refine the interpretation of the quantitative results, providing a richer understanding of the complex relationship between deprivation and health.
Statistical Methods for Analyzing the Improved ADI and Health Outcomes
Once the improved ADI is constructed, various statistical methods can be employed to analyze its relationship with health outcomes. Regression analysis, including both linear and generalized linear models, is a common approach. This allows researchers to assess the independent association between the ADI and specific health indicators while controlling for other confounding factors. For example, a multilevel model could be used to account for clustering of individuals within neighborhoods.
Srilekha Palle’s work on rethinking the Area Deprivation Index for better health equity got me thinking about access to care. Improving access is key, and initiatives like the one described in this article, cms launches primary care medicare model aco , are a step in the right direction. Ultimately, effective interventions like these are crucial for addressing the disparities highlighted by Palle’s research and truly achieving health equity.
Spatial analysis techniques, such as geographically weighted regression, can further explore the spatial patterns of deprivation and their association with health disparities. Furthermore, techniques like propensity score matching could be used to compare health outcomes between areas with similar characteristics but differing levels of deprivation. These methods help establish the strength and direction of the relationship between the improved ADI and health outcomes, providing valuable insights for targeted interventions to improve health equity.
Effective Interventions Based on Redefined ADIs
A refined Area Deprivation Index (ADI) offers a powerful tool for designing and implementing targeted health interventions. By moving beyond simplistic measures of deprivation, a redefined ADI can pinpoint specific vulnerabilities within communities, enabling a more effective allocation of resources and a more equitable distribution of healthcare services. This allows for a more nuanced understanding of the complex interplay of factors contributing to health inequities.
Srilekha Palle’s work on rethinking the area deprivation index for better health equity is crucial. It makes me wonder about access to care, especially considering the recent news about Walmart Health’s closures; check out this article on the impact, despite Walmart Health’s closure, the company healthcare destination, Scott Bowman , to see how corporate strategies influence community health.
Ultimately, Palle’s research highlights the need for more equitable distribution of healthcare resources, regardless of corporate decisions.
The key to leveraging a refined ADI lies in its ability to inform a framework for targeted interventions. This framework should consider not only the level of deprivation but also the specific types of deprivation experienced by a community. This allows for a more tailored and effective approach to addressing health disparities.
Framework for Targeted Health Interventions
A framework for targeted health interventions based on a refined ADI should incorporate several key elements. First, a thorough assessment of the refined ADI data is necessary to identify communities experiencing the highest levels of deprivation across various factors (e.g., income, education, employment, housing, access to healthcare). Second, the framework should Artikel specific, measurable, achievable, relevant, and time-bound (SMART) goals for each identified area of deprivation.
Third, the framework must define the appropriate interventions for each area, considering community needs and preferences. Finally, the framework needs to include a robust monitoring and evaluation system to track progress and adapt interventions as needed. This iterative process ensures the framework remains responsive to evolving community needs.
Examples of Tailored Health Interventions
Once specific deprivation factors are identified by the improved ADI, interventions can be tailored to address them directly. This targeted approach maximizes the impact of resource allocation.
- Addressing Food Insecurity: In communities with high rates of food insecurity (identified through a refined ADI incorporating food access metrics), interventions could include establishing community gardens, expanding food bank services, or implementing nutrition education programs. These programs might involve partnerships with local organizations and volunteers to ensure sustainability and community ownership.
- Improving Access to Healthcare: Where the refined ADI highlights limited access to healthcare due to transportation barriers or lack of healthcare providers, interventions could involve mobile health clinics, telehealth services, or subsidized transportation programs. This could involve collaborations with healthcare providers and transportation services to address logistical challenges.
- Addressing Housing Instability: For communities with high rates of housing instability, identified by the ADI’s inclusion of housing quality and affordability data, interventions might include housing assistance programs, home repair initiatives, or programs to prevent homelessness. Such initiatives would likely require partnerships with housing authorities and non-profit organizations.
- Promoting Health Literacy: In communities with low health literacy levels (as reflected in the refined ADI), interventions could focus on developing culturally appropriate health education materials and programs, utilizing community health workers, and providing language access services. These initiatives necessitate collaboration with community leaders and health professionals to ensure effective communication.
Informing Resource Allocation for Health Services
The refined ADI can directly inform resource allocation by providing a data-driven basis for prioritizing communities with the greatest need. For example, funding for new health clinics or the expansion of existing services could be allocated based on the severity and type of deprivation identified in the ADI. This ensures that resources are directed to where they are most needed, improving health equity.
A transparent process for resource allocation, clearly linked to the ADI data, is crucial for building trust and ensuring accountability. This could involve public forums and collaborative decision-making processes with community stakeholders.
Community-Based Participatory Research Approaches
Community-based participatory research (CBPR) offers a powerful approach to leverage the refined ADI. CBPR emphasizes collaboration between researchers and community members throughout the research process, ensuring that interventions are relevant, culturally appropriate, and sustainable.
- Involving community members in the design and implementation of interventions ensures that the solutions are tailored to their specific needs and priorities. This participatory approach increases the likelihood of successful outcomes and fosters a sense of ownership within the community.
- CBPR can help identify local resources and build partnerships with community organizations, strengthening the sustainability of interventions long after the research project concludes. This collaborative model fosters community empowerment and builds capacity for long-term health improvement.
- The refined ADI can be used to identify communities for CBPR projects, ensuring that research efforts focus on areas with the greatest need and the most potential for impact. This targeted approach maximizes the efficiency and effectiveness of research resources.
Visualizing Health Disparities Using the Refined ADI
Our refined Area Deprivation Index (ADI) offers a powerful tool for understanding and addressing health inequities. Visualizing the data derived from this improved index is crucial for effectively communicating these disparities to both policymakers and the public, fostering a shared understanding and driving targeted interventions. By employing clear and compelling visuals, we can translate complex data into actionable insights.Effective visualization of the refined ADI requires a multi-faceted approach.
We need to represent the spatial distribution of deprivation and its correlation with various health outcomes. This allows for a clear identification of areas needing immediate attention and informs the strategic allocation of resources.
Mapping Deprivation Levels and Health Outcomes
A choropleth map would be particularly effective in visualizing the spatial distribution of deprivation levels. This type of map uses color shading to represent the level of deprivation in different geographical areas, such as census tracts or zip codes. A gradient color scheme, progressing from light green (representing low deprivation) to dark red (representing high deprivation), would clearly illustrate the variation across the region.
Overlaying this map with data on specific health outcomes, such as rates of infant mortality, chronic disease prevalence, or life expectancy, would reveal the direct correlation between deprivation and health. For instance, darker red areas signifying high deprivation would likely also show higher rates of infant mortality or lower life expectancy, highlighting the stark health disparities. The map’s legend would clearly define the color scale and the corresponding deprivation and health outcome levels.
Correlation Between Refined ADI and Specific Health Outcomes
A scatter plot provides a powerful visual representation of the correlation between the refined ADI and specific health outcomes. The x-axis would represent the refined ADI score, ranging from low to high deprivation. The y-axis would represent the chosen health outcome, such as the prevalence of diabetes or the rate of hospital admissions. Each point on the scatter plot would represent a specific geographical area, with its position determined by its ADI score and the corresponding health outcome.
A clear positive correlation (a upward-sloping trend) would visually demonstrate how increasing deprivation levels are associated with poorer health outcomes. For example, areas with high ADI scores would cluster towards the top right corner of the plot, indicating high deprivation and high rates of diabetes. A line of best fit could be added to emphasize the trend and quantify the strength of the correlation.
This visualization allows for a straightforward comparison across different geographical areas and health outcomes.
Communicating Health Inequities to Policymakers and the Public
These visualizations – the choropleth map and the scatter plot – serve as powerful communication tools. The choropleth map provides a clear geographical picture of health disparities, immediately identifying areas requiring targeted interventions. Policymakers can use this map to allocate resources strategically, directing funding and programs to the most deprived areas. The scatter plot provides a quantitative measure of the relationship between deprivation and health, strengthening the evidence base for policy decisions.
For the public, these visualizations translate complex data into easily understandable visuals, fostering a shared understanding of health inequities and building support for initiatives aimed at improving health equity. The simplicity and clarity of these visual representations make them accessible and impactful, encouraging community engagement and promoting informed public discourse on health disparities.
Final Conclusion
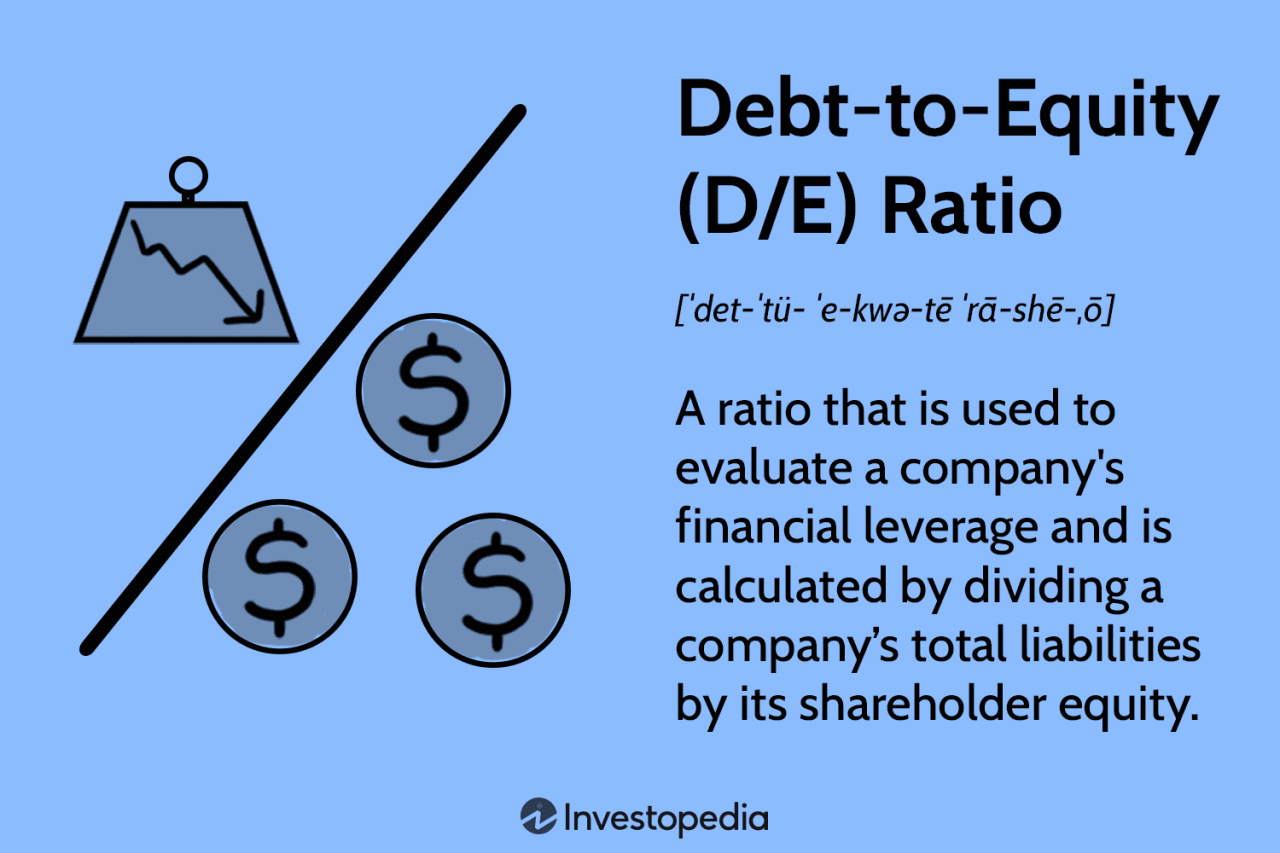
Source: investopedia.com
Ultimately, rethinking the area deprivation index, as advocated by Srilekha Palle, isn’t just about tweaking a formula; it’s about fundamentally shifting our understanding of health equity. By incorporating a more holistic and nuanced approach, we can create more effective interventions, allocate resources more strategically, and empower communities to shape their own health destinies. Palle’s work serves as a powerful reminder that true health equity requires not just better data, but a deeper commitment to understanding and addressing the social determinants of health.
The visualizations and data-driven approaches discussed here can empower us to communicate these disparities effectively, fostering meaningful change and improving the lives of those most in need.
Popular Questions: Rethinking Area Deprivation Index Effective Measures Health Equity Srilekha Palle
What are some examples of qualitative data that could be included in a revised ADI?
Examples include community surveys focusing on access to healthcare, environmental factors (e.g., pollution levels, green spaces), social cohesion, and perceived safety. Interviews with community members can also provide invaluable insights into lived experiences.
How can the improved ADI inform policy decisions?
A refined ADI can help policymakers target resources to areas with the greatest need, prioritize health interventions based on specific deprivation factors, and track the effectiveness of these interventions over time. It provides a data-driven basis for resource allocation and policy development.
What are the ethical considerations in using an improved ADI?
Ensuring data privacy and avoiding stigmatization of communities are crucial ethical considerations. Transparent data collection and community engagement are vital to building trust and ensuring the responsible use of the ADI.