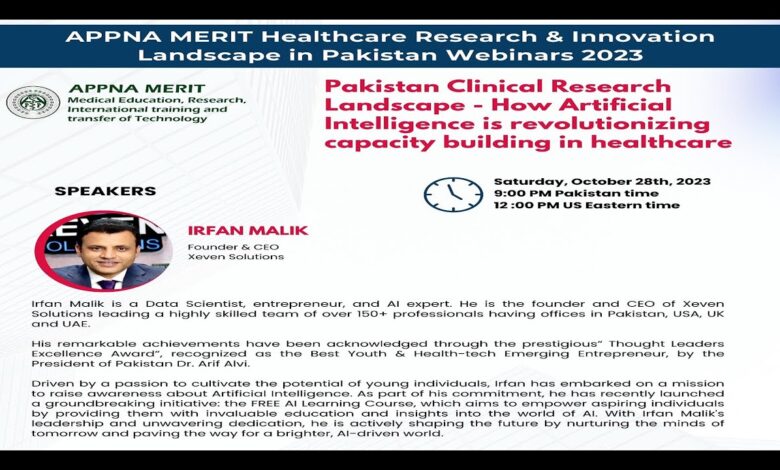
Rural Hospital AI Adoption Bridging the Digital Divide
Rural hospital artificial intelligence adoption digital divide: It’s a phrase that encapsulates a huge challenge facing healthcare today. While AI promises revolutionary improvements in patient care, its implementation in rural areas lags significantly behind urban centers. This disparity isn’t just about technology; it’s about infrastructure, funding, and the very fabric of rural communities. We’ll delve into the reasons behind this gap, explore potential solutions, and examine the ethical considerations that arise when deploying AI in such diverse settings.
This isn’t just a technological problem; it’s a social justice issue.
The lack of reliable internet access, insufficient funding, and a shortage of skilled technicians all contribute to the slow adoption of AI in rural hospitals. This means patients in these areas often miss out on the benefits of cutting-edge diagnostic tools, personalized treatment plans, and improved overall healthcare outcomes. We’ll examine real-world examples of successful AI implementations in rural settings, highlighting strategies that can help overcome these hurdles and pave the way for equitable access to AI-powered healthcare for everyone.
The Current State of AI Adoption in Rural Hospitals
The integration of artificial intelligence (AI) into healthcare is rapidly evolving, but its penetration into rural hospitals lags significantly behind urban centers. This disparity creates a critical challenge, exacerbating existing healthcare inequalities. Understanding the current state of AI adoption in these settings is crucial for developing effective strategies to bridge the digital divide.
Several factors contribute to the uneven distribution of AI technologies across rural hospitals. These include limited resources, a shortage of skilled personnel, unreliable infrastructure, and a lack of awareness regarding the potential benefits of AI. Overcoming these obstacles requires a multi-pronged approach, encompassing technological solutions, educational initiatives, and policy changes.
AI Technology Adoption in Rural Hospitals by Region
The following table provides a snapshot of AI adoption across different regions, acknowledging that data on this topic remains limited and often reported anecdotally. The levels of adoption are subjective estimations based on available information and should be considered as indicative rather than definitive.
Region | AI Technology Used | Level of Adoption | Challenges Faced |
---|---|---|---|
Rural US South | Telemedicine platforms with AI-powered diagnostic support (limited) | Low | Lack of broadband access, shortage of IT specialists, financial constraints. |
Rural Western Europe | AI-powered radiology tools in some larger rural hospitals; limited use of predictive analytics. | Medium | Data privacy concerns, integration challenges with existing systems, resistance to change among staff. |
Rural Canada | Pilot programs exploring AI for remote patient monitoring and chronic disease management. | Low | Funding limitations, regulatory hurdles, concerns about data security. |
Rural Australia | AI-powered image analysis tools in select hospitals; limited use of AI in administrative tasks. | Medium | Geographical isolation, workforce shortages, high implementation costs. |
Specific AI Technologies in Rural Healthcare
Despite the challenges, several AI technologies are showing promise in rural healthcare settings. Their implementation, however, often faces significant hurdles.
- Telemedicine platforms with AI-powered diagnostic support: These platforms enable remote consultations and utilize AI algorithms to assist in diagnosis, potentially reducing the need for patients to travel long distances for specialist care. For example, AI could analyze images from a dermatological exam remotely and flag potential skin cancers.
- AI-powered radiology tools: These tools can assist radiologists in detecting anomalies in medical images (X-rays, CT scans, MRIs), potentially improving diagnostic accuracy and efficiency, even with limited staff. This is particularly valuable in regions with shortages of radiologists.
- Predictive analytics for patient risk stratification: AI algorithms can analyze patient data to identify individuals at high risk of developing certain conditions, enabling proactive interventions and improving patient outcomes. This could lead to better management of chronic diseases like diabetes and heart failure in rural populations.
- Remote patient monitoring (RPM) systems: These systems utilize wearable sensors and AI-powered analytics to monitor patients’ vital signs remotely, allowing for early detection of health deteriorations and timely interventions. This is crucial in rural areas where access to immediate medical attention can be limited.
Factors Contributing to Varying Levels of AI Adoption
The varying levels of AI adoption across rural hospitals stem from a complex interplay of factors. Addressing these disparities requires a comprehensive and nuanced approach.
Financial constraints are a major barrier. The cost of acquiring, implementing, and maintaining AI technologies can be prohibitive for resource-limited rural hospitals. Furthermore, a lack of skilled personnel capable of operating and maintaining these systems poses a significant challenge. Inadequate infrastructure, particularly limited broadband access, hinders the effective deployment of AI-powered applications that rely on reliable connectivity.
Finally, concerns about data privacy and security, coupled with a lack of awareness among healthcare providers regarding the potential benefits of AI, further impede its adoption.
The Digital Divide and its Impact on AI Implementation
The promise of artificial intelligence in healthcare is undeniable, yet its realization faces a significant hurdle: the digital divide. This disparity in access to technology and digital infrastructure profoundly impacts the ability of rural hospitals to adopt and benefit from AI solutions, creating a two-tiered system of healthcare delivery. This section explores the specific challenges rural hospitals face in bridging this gap.
Infrastructure Disparities Between Rural and Urban Areas
The digital infrastructure supporting AI implementation varies dramatically between rural and urban settings. This difference directly impacts a hospital’s ability to leverage AI’s potential. Reliable high-speed internet, robust power grids, and readily available technical expertise are all crucial components for successful AI integration. The following table highlights these differences:
Rural | Urban | |
---|---|---|
Internet Speed | Often significantly slower, with limited broadband availability in many areas, leading to difficulties in transmitting large datasets required for AI applications. | Generally faster and more reliable broadband access, enabling efficient data transfer and AI processing. |
Access to Reliable Power | Power outages are more frequent and prolonged, potentially disrupting AI systems and data storage. Backup power solutions are often less readily available or more costly. | More reliable power grids with redundant systems and readily available backup power options minimize disruptions. |
Availability of Technical Expertise | Shortage of skilled IT professionals and data scientists capable of implementing, maintaining, and troubleshooting complex AI systems. Recruitment and retention of qualified personnel are significant challenges. | Larger pool of qualified IT professionals and data scientists, making it easier to find and retain the necessary expertise for AI implementation and support. |
Financial Barriers to AI Adoption in Rural Hospitals
Rural hospitals often operate on significantly smaller budgets than their urban counterparts. The high initial investment costs associated with AI technologies—including hardware, software licenses, data acquisition, and ongoing maintenance—present a substantial barrier to entry. Furthermore, the need for staff training and ongoing support adds to the financial burden. Many rural hospitals lack the financial resources to invest in cutting-edge AI solutions, hindering their ability to compete with better-resourced urban centers.
For example, implementing a sophisticated AI-powered diagnostic tool might require a capital expenditure far exceeding a rural hospital’s annual IT budget.
Regulatory and Policy Challenges
Navigating the regulatory landscape for AI in healthcare is complex, even more so for rural hospitals with limited resources. Data privacy regulations, such as HIPAA, require stringent compliance, demanding investments in secure data storage and management systems. The regulatory approval process for new AI-based medical devices and software can be lengthy and expensive, further delaying adoption in resource-constrained settings.
Additionally, a lack of clear guidelines and policies specific to AI implementation in rural healthcare settings creates uncertainty and can deter investment. This regulatory complexity can disproportionately impact rural hospitals, which often lack the dedicated legal and compliance staff found in larger urban institutions.
Addressing the Challenges of AI Implementation in Rural Hospitals
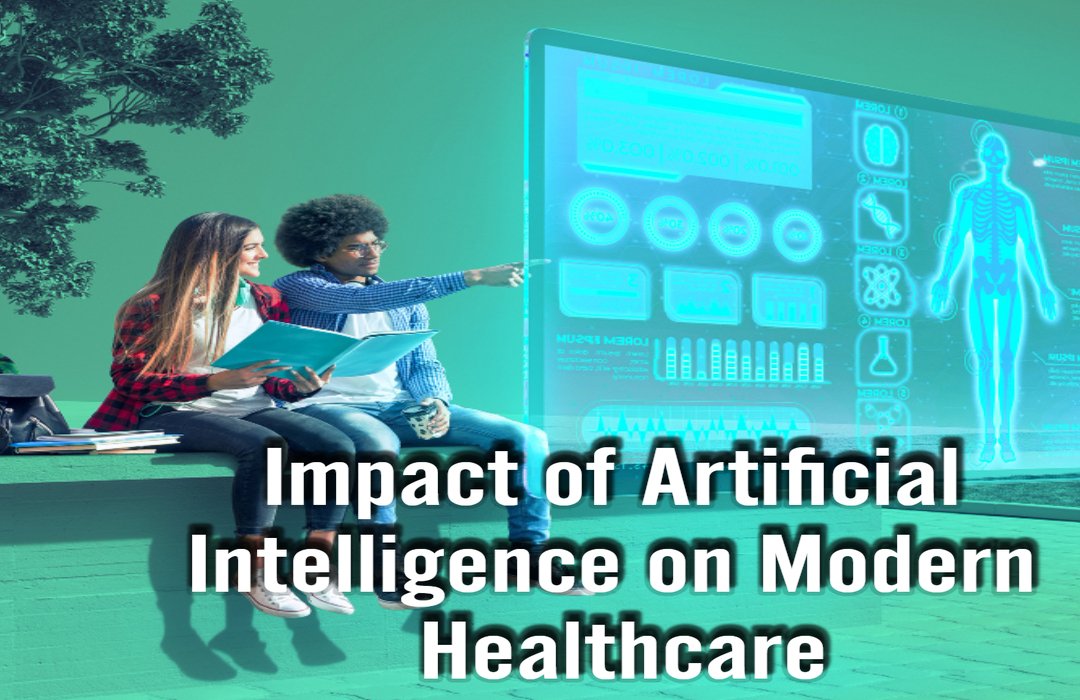
Source: blogwire.in
The successful integration of artificial intelligence (AI) in rural hospitals faces unique hurdles, primarily stemming from the digital divide and resource constraints. Overcoming these challenges requires a multifaceted approach that addresses technological limitations, workforce development, and financial sustainability. This section will explore potential frameworks and strategies to facilitate AI adoption in these underserved settings.
A Framework for Supporting AI Adoption in Rural Hospitals
A successful strategy for AI implementation in rural hospitals must be tailored to the specific needs and resources of each individual institution. However, a general framework can provide a roadmap for progress. This framework should encompass technological support, training and education, financial incentives, and ongoing monitoring and evaluation.
- Technological Infrastructure Development: This includes providing high-speed internet access, robust network infrastructure, and secure data storage solutions. This might involve government subsidies for broadband expansion or partnerships with telecommunications companies to offer discounted services to rural hospitals.
- AI-Specific Training and Education: Healthcare professionals need training on how to effectively use and interpret AI tools. This requires investing in comprehensive training programs tailored to the specific AI technologies being implemented, and ongoing support to address any issues or questions that arise.
- Financial Incentives and Support: Government grants, tax breaks, or loan programs specifically designed to support AI adoption in rural hospitals can incentivize implementation. This could also include funding for the purchase of necessary hardware and software, as well as ongoing maintenance and support.
- Collaborative Networks and Knowledge Sharing: Establishing networks that connect rural hospitals with larger healthcare systems or AI developers can facilitate knowledge sharing and best practice dissemination. This collaborative approach allows for efficient resource allocation and reduces the burden on individual institutions.
- Data Privacy and Security Measures: Robust data privacy and security protocols are crucial for protecting patient information. This requires investment in secure data storage solutions and staff training on data security best practices. Compliance with HIPAA regulations is paramount.
Examples of Successful AI Implementations in Rural Healthcare
Despite the challenges, several rural healthcare settings have successfully implemented AI solutions. These successes often involve strategic partnerships and a focus on addressing specific local needs.
The rural hospital AI adoption digital divide is a serious issue, hindering access to crucial advancements. The recent Supreme Court decision, as reported in this article on the scotus overturns chevron doctrine healthcare , could significantly impact healthcare regulations and funding, potentially exacerbating the problem for already struggling rural facilities trying to implement AI. This legal shift may further limit resources for bridging the digital divide in rural healthcare.
- Telemedicine with AI-powered diagnostics: A rural hospital in Montana partnered with a telemedicine provider using AI-powered image analysis for faster and more accurate diagnosis of skin cancers. This overcame the shortage of dermatologists in the region by providing remote access to expert consultation and diagnostic support.
- AI-driven predictive modeling for patient readmission: A rural hospital in Iowa used AI to predict which patients were at high risk of readmission after discharge. This allowed them to proactively implement interventions, such as increased follow-up calls and home healthcare visits, reducing readmission rates and improving patient outcomes. This required careful data collection and analysis, followed by implementation of new protocols based on the AI’s predictions.
- AI-powered administrative tasks automation: A rural hospital in Nebraska leveraged AI to automate administrative tasks like scheduling and billing. This freed up staff time to focus on patient care, addressing a common staffing shortage issue in rural areas. The implementation involved integrating AI-powered software with existing hospital systems, requiring careful planning and integration support.
Solutions to Bridge the Digital Divide, Rural hospital artificial intelligence adoption digital divide
Addressing the digital divide is crucial for successful AI adoption in rural hospitals. This requires a multi-pronged approach involving governmental, private sector, and community initiatives.
- Government investment in broadband infrastructure: Expanding high-speed internet access to rural areas is paramount. Government funding for infrastructure development and subsidies for internet service providers can help bridge this gap.
- Public-private partnerships for technology access: Collaborations between technology companies, healthcare organizations, and government agencies can facilitate the deployment of affordable and reliable technology solutions in rural hospitals.
- Telehealth infrastructure development: Investing in robust telehealth infrastructure allows for remote access to specialists and AI-powered diagnostic tools, mitigating the limitations of geographical isolation.
- Training and support for rural healthcare workers: Providing training and ongoing support to rural healthcare workers on how to effectively use AI tools is crucial for successful implementation. This might involve online training programs, mentorship opportunities, and technical support.
The Impact of AI on Patient Care in Rural Settings: Rural Hospital Artificial Intelligence Adoption Digital Divide
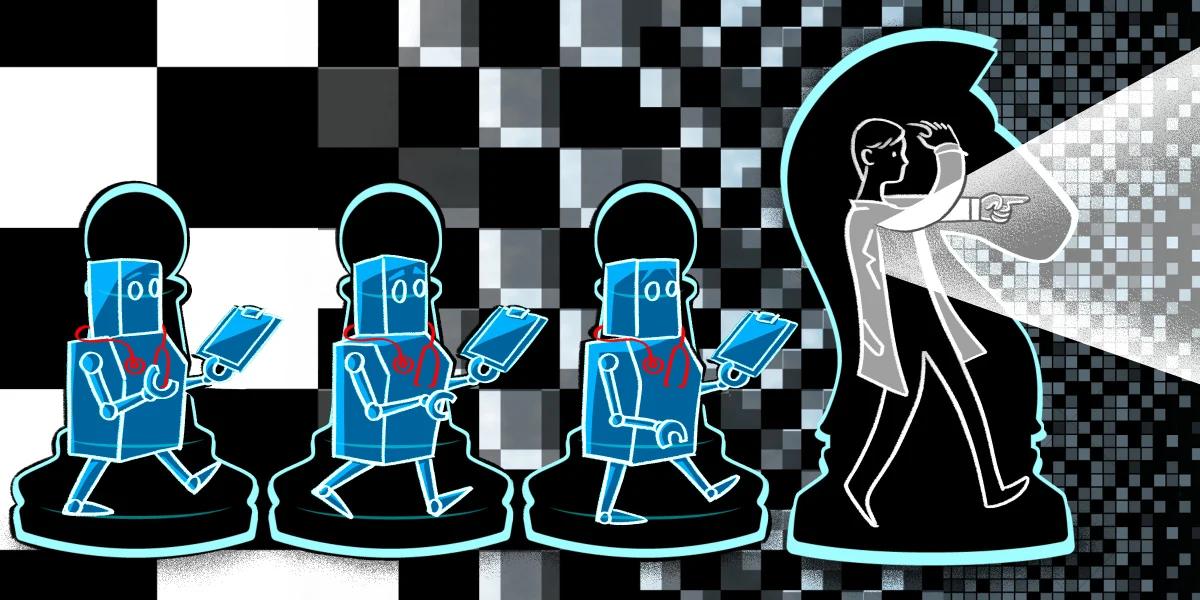
Source: doxcdn.com
The integration of artificial intelligence (AI) into rural healthcare presents a unique opportunity to overcome significant challenges and dramatically improve the quality of patient care. Rural hospitals often grapple with limited resources, specialist shortages, and geographical isolation, all of which impact access to timely and effective medical services. AI offers a powerful tool to mitigate these issues and enhance the overall patient experience.AI technologies can significantly improve the quality of patient care in rural hospitals through various applications.
For instance, AI-powered diagnostic tools can assist clinicians in interpreting medical images (X-rays, CT scans, etc.), leading to faster and more accurate diagnoses, even in the absence of readily available specialists. This is particularly crucial in rural areas where specialists may be scarce or require significant travel time to reach the patient. Furthermore, AI algorithms can analyze patient data to predict potential health risks, allowing for proactive interventions and preventative care, thus reducing hospital readmissions and improving overall patient outcomes.
Improved Diagnostic Accuracy and Efficiency
AI-powered diagnostic tools can analyze medical images with remarkable speed and accuracy, often exceeding human capabilities in certain areas. For example, AI algorithms trained on vast datasets of medical images can detect subtle anomalies in X-rays or CT scans that might be missed by the human eye, leading to earlier detection of diseases like cancer or pneumonia. This improved diagnostic accuracy translates directly into better patient outcomes, especially in rural settings where access to specialists for second opinions might be limited.
The efficiency gains also free up clinicians’ time, allowing them to focus on other critical tasks, such as patient interaction and care coordination.
Predictive Analytics for Proactive Care
AI’s ability to analyze large datasets of patient information enables the development of predictive models for various health conditions. By identifying patients at high risk of developing certain diseases or experiencing adverse events, healthcare providers can implement proactive interventions to prevent or mitigate these risks. This is particularly valuable in rural areas where preventative care might be less accessible.
For example, an AI system could predict which patients are at high risk of developing heart failure based on their medical history, lifestyle factors, and genetic information. This allows for early intervention, such as lifestyle modifications or medication adjustments, to reduce the likelihood of hospitalization.
Enhanced Telehealth Capabilities
The integration of AI with telehealth platforms significantly expands access to specialized care in rural communities. AI-powered remote monitoring systems can track vital signs and other health metrics remotely, allowing healthcare providers to monitor patients’ conditions continuously and intervene promptly if necessary. Furthermore, AI-powered chatbots can provide patients with basic medical information and support, reducing the burden on healthcare professionals and ensuring patients receive timely assistance.
Imagine a scenario where a patient in a remote rural area can use a telehealth app equipped with an AI-powered chatbot to address basic health concerns, receive medication reminders, and connect with a physician when necessary – this drastically improves access to care and reduces the need for lengthy travel.
Addressing Healthcare Disparities
AI has the potential to significantly reduce healthcare disparities experienced by rural populations. By providing access to advanced diagnostic tools, predictive analytics, and telehealth services, AI can help bridge the gap in quality of care between rural and urban areas. This contributes to improved health outcomes and increased equity in access to healthcare, which is crucial for improving the health and well-being of rural communities.
For instance, a rural hospital utilizing AI-powered diagnostic tools might be able to detect and treat certain cancers earlier than previously possible, leading to better survival rates compared to those in areas with less access to such technology.
The digital divide significantly impacts rural hospital AI adoption; access to technology and training is often limited. This disparity highlights the need for equitable resource allocation, especially considering the health implications. Thinking about this, I was reminded of an interesting article I read recently on are women and men receptive of different types of food and game changing superfoods for women , which made me realize how crucial access to information – be it about healthcare or nutrition – is for overall well-being, and how similar these issues are to the digital divide in healthcare.
Ultimately, bridging this gap in rural areas is key to improving health outcomes.
Ethical Considerations and Future Directions
The integration of artificial intelligence (AI) into rural healthcare presents a unique set of ethical challenges alongside its immense potential. Successfully navigating these challenges is crucial to ensuring equitable access to the benefits of AI while mitigating potential harms. A responsible approach requires careful consideration of data privacy, algorithmic bias, and the broader implications for healthcare equity in rural communities.The ethical implications of AI in rural healthcare are multifaceted and demand proactive solutions.
Data privacy, a fundamental right, is particularly vulnerable in the context of limited resources and potentially less robust cybersecurity infrastructure in rural settings. Algorithmic bias, stemming from skewed training data, can perpetuate existing health disparities if not addressed head-on. Equitable access to AI-powered healthcare necessitates careful planning and resource allocation to ensure that the benefits reach all rural populations, regardless of socioeconomic status or geographic location.
Data Privacy and Security in Rural AI Healthcare
Protecting patient data in rural hospitals adopting AI is paramount. The unique challenges include limited IT budgets, potential lack of specialized cybersecurity personnel, and the dispersed nature of rural populations, increasing the risk of data breaches. Robust data encryption, regular security audits, and employee training on data privacy best practices are essential. Furthermore, collaboration with regional cybersecurity hubs or larger healthcare systems could provide valuable support and resources.
Consider a scenario where a small rural hospital partners with a state-level health information exchange (HIE) to leverage their expertise in data security and privacy protocols, ensuring compliance with HIPAA and other relevant regulations. This collaborative approach strengthens data protection while maximizing the benefits of AI implementation.
Mitigating Algorithmic Bias in Rural AI Applications
Algorithmic bias, where AI systems reflect and amplify existing societal biases, poses a significant threat to equitable healthcare. This can manifest in misdiagnosis, inappropriate treatment recommendations, or unequal access to care. To mitigate this risk, careful attention must be paid to the data used to train AI algorithms. This includes ensuring diverse and representative datasets that accurately reflect the demographics and health conditions of the rural population being served.
Regular audits of AI algorithms for bias, along with ongoing retraining using updated and balanced data, are crucial steps to ensure fairness and accuracy. For example, an AI system designed to predict patient risk for heart disease should be trained on data that includes a diverse range of patients from different racial, ethnic, and socioeconomic backgrounds within the rural community, to prevent the system from disproportionately misclassifying certain groups.
Ensuring Equitable Access to AI-Powered Healthcare
Equitable access to AI-powered healthcare in rural areas requires a multi-pronged approach. This includes investing in infrastructure upgrades such as high-speed internet access and robust IT systems, providing training for healthcare professionals on the use of AI tools, and addressing potential digital literacy barriers among patients. Furthermore, financial incentives and support for rural hospitals to adopt AI technologies are essential to bridge the resource gap.
Consider a telehealth program leveraging AI-powered diagnostic tools, where specialists in urban centers remotely assist rural healthcare providers. This ensures access to advanced diagnostic capabilities without requiring the relocation of patients or healthcare professionals. This model exemplifies how targeted investments and innovative collaborations can promote equitable access.
Future Trends and Collaborations in Rural AI Healthcare
The future of AI in rural healthcare is likely to be shaped by several key trends. The rise of edge computing, where AI processing is performed locally on devices within the hospital, could overcome bandwidth limitations in rural areas. The integration of AI with other emerging technologies, such as the Internet of Medical Things (IoMT) and wearable sensors, will enable continuous patient monitoring and proactive healthcare management.
Strengthened collaborations between rural hospitals, academic institutions, technology companies, and government agencies will be crucial to fostering innovation and ensuring successful AI implementation.
A Visual Representation of the Future Landscape of AI in Rural Healthcare
Imagine a vibrant, interconnected network. At its center are rural hospitals, depicted as hubs radiating outwards. Connecting these hubs are strong digital pathways representing high-speed internet and secure data exchange networks. Smaller nodes represent individual patients connected via telehealth platforms and wearable sensors, feeding data into the central hubs. These hubs, in turn, are connected to larger academic medical centers and technology companies, facilitating collaborations and knowledge sharing.
The digital divide drastically impacts rural hospital access to life-saving AI, hindering early diagnosis and treatment of respiratory illnesses. Reading about Monali Thakur’s hospitalization after struggling to breathe, as detailed in this article monali thakur hospitalised after struggling to breathe how to prevent respiratory diseases , highlights the urgent need for better healthcare infrastructure. This underscores how crucial AI adoption in rural areas is for preventing similar tragedies and improving patient outcomes.
AI algorithms are visualized as intelligent threads weaving through this network, facilitating diagnosis, treatment planning, and remote monitoring, all while ensuring data privacy and security through strong encryption and robust cybersecurity protocols. The overall image emphasizes collaboration, connectivity, and the equitable distribution of AI-powered healthcare across rural communities.
Ultimate Conclusion
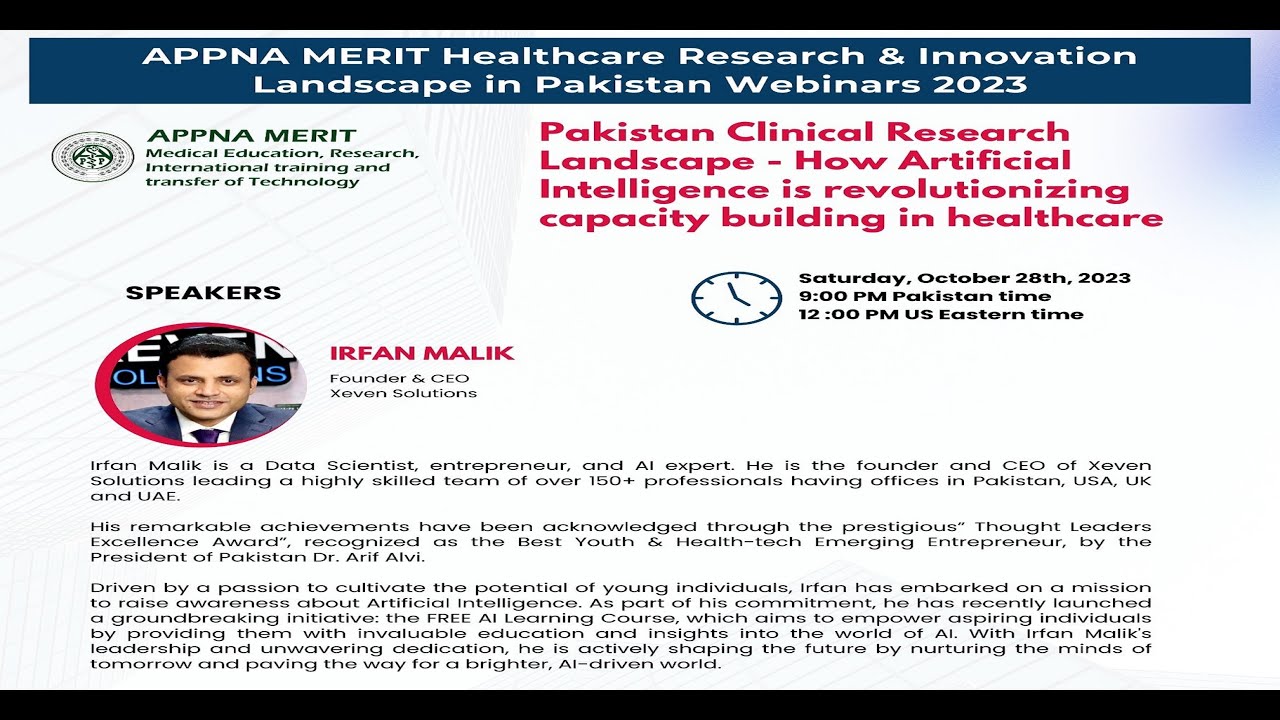
Source: ytimg.com
The digital divide in rural healthcare is a significant obstacle to overcome, but it’s not insurmountable. By addressing the infrastructural limitations, providing adequate funding, and fostering collaboration between healthcare providers, technology developers, and policymakers, we can bridge this gap. The future of rural healthcare hinges on embracing innovative solutions and ensuring that the benefits of AI reach every community, regardless of its location.
The potential for improved patient outcomes and reduced healthcare disparities is immense – let’s work together to make it a reality.
Essential Questionnaire
What specific AI technologies are most relevant to rural hospitals?
Telemedicine platforms, remote patient monitoring systems, AI-powered diagnostic tools (like image analysis for X-rays), and predictive analytics for resource allocation are particularly beneficial.
How can we ensure data privacy and security in rural AI implementations?
Robust cybersecurity measures, adherence to HIPAA regulations, and careful data anonymization techniques are crucial to protect sensitive patient information.
What role can government policies play in promoting AI adoption in rural hospitals?
Incentivizing AI adoption through grants and subsidies, investing in rural digital infrastructure, and creating supportive regulatory frameworks are key governmental roles.