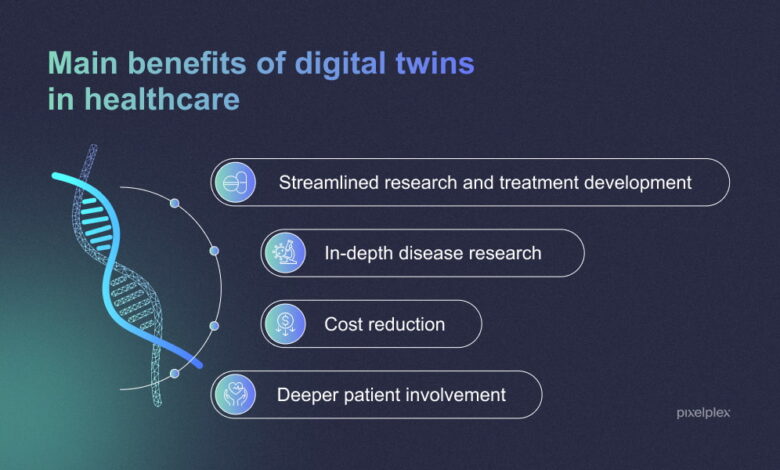
Study Widespread Digital Twins in Healthcare
Study widespread digital twins healthcare – Study widespread digital twins in healthcare: Imagine a world where your doctor has a perfect, constantly updating 3D model of your body, predicting potential health problems before they even arise. That’s the promise of widespread digital twin technology in healthcare. This isn’t just science fiction; it’s a rapidly developing field with the potential to revolutionize how we prevent, diagnose, and treat disease.
This exploration dives into the exciting possibilities and the very real challenges facing the widespread adoption of digital twins in medicine.
From personalized medicine tailored to your unique genetic makeup to more efficient hospital operations and groundbreaking advancements in surgical planning, the applications are vast and transformative. But alongside this incredible potential lies the crucial need to address ethical concerns surrounding data privacy and security. This deep dive examines the current state of digital twin adoption, explores the technological hurdles, and offers a glimpse into the future of healthcare powered by these sophisticated digital representations.
Defining Digital Twins in Healthcare
Digital twins are virtual representations of real-world entities, and in healthcare, this translates to creating detailed, dynamic models of patients, organs, or even entire populations. These models leverage data from various sources to simulate biological processes, predict outcomes, and personalize treatment strategies. This technology holds immense promise for revolutionizing healthcare, offering the potential for more precise diagnoses, proactive interventions, and improved patient outcomes.
Studying the widespread adoption of digital twins in healthcare is fascinating, especially considering the impact of consolidation. News about HSHS and Prevea closing some Wisconsin hospitals and health centers, as reported on this article , highlights the challenges in implementing such technologies across a shifting landscape. Understanding these changes is crucial for developing effective digital twin strategies that account for evolving healthcare structures.
Core Concept of Digital Twins in Healthcare
A healthcare digital twin is a virtual replica of a patient, organ, or population, created using data from various sources. It’s not just a static image; it’s a dynamic model that evolves over time, reflecting changes in the patient’s health status or the population’s health trends. This dynamic nature allows healthcare professionals to simulate interventions, predict future health outcomes, and personalize treatment plans with unprecedented accuracy.
The goal is to use this virtual representation to improve decision-making and ultimately enhance patient care.
Types of Digital Twins in Healthcare
Digital twins in healthcare are categorized based on their scope and focus. Patient-specific digital twins are detailed models of individual patients, created using data from electronic health records (EHRs), wearable sensors, and medical imaging. Organ-specific digital twins focus on a single organ, such as the heart or liver, allowing for detailed simulations of organ function and response to treatment.
Population-level digital twins model the health of an entire population, incorporating demographic data, disease prevalence, and environmental factors to predict and manage public health challenges.
Data Sources for Creating Digital Twins in Healthcare
The creation of accurate and effective digital twins relies on a rich and diverse range of data sources. Electronic Health Records (EHRs) provide a wealth of historical patient data, including medical history, diagnoses, treatments, and lab results. Wearable sensors, such as smartwatches and fitness trackers, continuously monitor vital signs and activity levels, providing real-time data on patient health. Medical imaging, including MRI, CT scans, and X-rays, provides detailed anatomical and physiological information.
Genomic data further enhances the precision of digital twins by incorporating individual genetic predispositions to disease. Other sources include clinical trial data and research publications.
Advantages and Disadvantages of Using Digital Twins in Healthcare
Advantage | Disadvantage |
---|---|
Personalized medicine: Tailored treatments based on individual patient characteristics. | Data privacy and security concerns: Protecting sensitive patient information. |
Improved diagnostic accuracy: Early detection and prevention of diseases. | High computational costs: Developing and maintaining complex digital twin models. |
Enhanced treatment planning: Optimized treatment strategies and reduced adverse events. | Data integration challenges: Combining data from diverse sources. |
Drug discovery and development: Accelerated drug development and reduced costs. | Lack of standardization: Inconsistent data formats and models across different systems. |
Widespread Adoption of Digital Twins in Healthcare
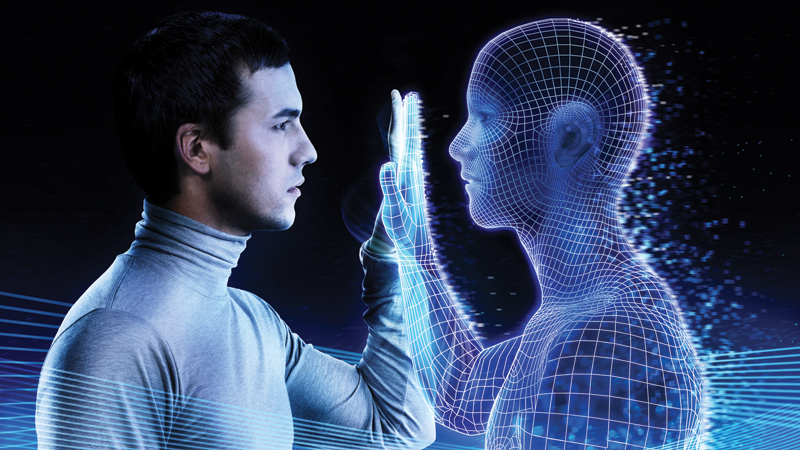
Source: healtharkinsights.com
The potential of digital twins to revolutionize healthcare is undeniable. By creating virtual representations of patients, organs, or even entire healthcare systems, we can unlock unprecedented opportunities for personalized medicine, improved diagnostics, and more efficient resource allocation. However, the journey from potential to widespread adoption is fraught with challenges. This section explores the current state of digital twin implementation, the obstacles hindering broader use, and potential solutions to accelerate their integration into mainstream healthcare.
Current State of Digital Twin Adoption in Healthcare Across Different Regions
The adoption of digital twins in healthcare varies significantly across regions. While pioneering work is occurring in countries with robust technological infrastructure and significant research funding, such as the United States and certain European nations (like Germany and the UK), many developing nations lag behind due to limited resources and technological capabilities. For instance, the US leads in the development and application of digital twins for drug discovery and personalized medicine, while European countries are making strides in using digital twins for population health management and optimizing hospital workflows.
However, even in advanced economies, widespread adoption remains limited, often confined to research settings or specific pilot programs within larger healthcare organizations. The uneven distribution of resources and expertise contributes to this global disparity.
Technological Barriers Hindering Widespread Adoption
Several technological hurdles impede the widespread adoption of digital twins in healthcare. Firstly, the creation of accurate and comprehensive digital twins requires massive amounts of high-quality data from diverse sources, including electronic health records (EHRs), medical imaging, genomics, and wearable sensor data. Integrating and processing this heterogeneous data remains a significant challenge, requiring sophisticated data interoperability solutions and powerful computing resources.
Secondly, the development and validation of sophisticated algorithms and AI models to power these digital twins is a computationally intensive and resource-demanding task. Finally, the lack of standardized protocols and frameworks for building and deploying digital twins limits interoperability and scalability across different healthcare systems. For example, different hospitals might use incompatible imaging systems, making it difficult to combine data for a comprehensive digital twin.
Ethical and Privacy Concerns Associated with Digital Twin Use in Healthcare
The use of digital twins in healthcare raises significant ethical and privacy concerns. The creation and utilization of digital twins involve the collection and processing of highly sensitive patient data, raising concerns about data security, unauthorized access, and potential misuse. Ensuring patient consent and data anonymization are paramount to maintain patient confidentiality and trust. Furthermore, algorithmic bias in the AI models powering digital twins can lead to discriminatory outcomes, particularly for marginalized populations.
The potential for these biases needs careful consideration and mitigation through rigorous testing and validation of algorithms. For example, a poorly trained algorithm used in a digital twin for predicting heart failure risk might inadvertently under-predict risk in certain demographic groups.
Potential Solutions to Overcome Challenges and Accelerate Adoption
Addressing the challenges and accelerating the adoption of digital twins requires a multi-pronged approach. Firstly, investments in robust data infrastructure and interoperability standards are crucial to facilitate seamless data sharing and integration. Secondly, the development of more efficient and robust AI algorithms and machine learning techniques is needed to improve the accuracy and reliability of digital twins. Thirdly, the establishment of clear ethical guidelines and regulatory frameworks is essential to ensure responsible data handling and mitigate privacy risks.
Finally, increased collaboration between researchers, clinicians, technology developers, and policymakers is vital to foster innovation and accelerate the widespread adoption of this transformative technology. Public-private partnerships could play a key role in funding research and development, ensuring accessibility, and addressing ethical concerns. For instance, government funding could support the creation of open-source platforms for building and deploying digital twins, making the technology more accessible to smaller healthcare providers.
Applications of Digital Twins in Specific Healthcare Areas
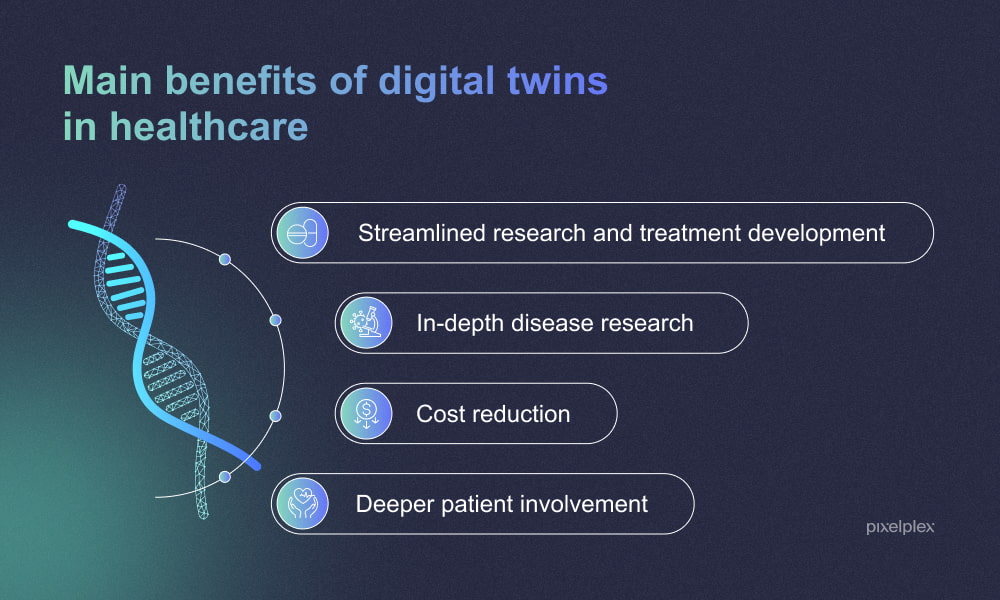
Source: pixelplex.io
Digital twins are rapidly transforming healthcare, offering unprecedented opportunities to personalize treatment, optimize operations, and improve patient outcomes. Their ability to simulate and predict complex biological systems and healthcare processes makes them a powerful tool across various medical disciplines. This section explores some key applications and future possibilities.
Personalized Medicine and Drug Discovery
Digital twins are revolutionizing personalized medicine by creating detailed virtual representations of individual patients. These models integrate genomic data, medical history, lifestyle factors, and real-time physiological data to simulate the effects of different treatments. This allows clinicians to predict individual responses to medications, minimizing adverse reactions and maximizing therapeutic efficacy. For example, a digital twin could simulate the response of a cancer patient to chemotherapy, allowing oncologists to tailor treatment plans to optimize effectiveness and reduce side effects.
In drug discovery, digital twins of organs or even entire bodies can be used to test the efficacy and safety of new drugs in a virtual environment, significantly reducing the time and cost associated with traditional clinical trials. This virtual testing can identify potential drug candidates more efficiently and predict potential side effects before human trials, leading to faster development of safer and more effective treatments.
Studying the widespread use of digital twins in healthcare is fascinating, especially when considering the cost implications. The rising costs associated with treatments like those highlighted in this insightful KFF report on medicare glp1 spending weight loss kff show how crucial predictive modeling, a key benefit of digital twins, could be in managing healthcare budgets. Ultimately, understanding these financial pressures can help us better design and implement effective digital twin strategies for improved patient care.
Surgical Planning and Robotic Surgery
In surgical planning, digital twins of patients’ anatomy are created using medical imaging data (CT scans, MRI scans, etc.). Surgeons can then use these virtual models to plan complex procedures, practice surgical techniques, and assess potential risks. This pre-operative planning reduces surgical time, improves precision, and minimizes complications. For example, a digital twin of a patient’s heart could be used to plan a minimally invasive valve replacement, allowing surgeons to rehearse the procedure virtually and identify potential challenges before entering the operating room.
Furthermore, digital twins are increasingly integrated with robotic surgery systems, providing real-time feedback and guidance during procedures. This enhanced precision and control can lead to improved surgical outcomes and faster patient recovery.
Improving Healthcare Operations and Resource Management
Digital twins can significantly improve the efficiency of healthcare operations and resource management. By simulating hospital workflows, staffing levels, and equipment utilization, healthcare administrators can optimize resource allocation, reduce wait times, and improve overall operational efficiency. For example, a digital twin of a hospital could simulate the impact of different staffing scenarios on patient flow, helping to identify optimal staffing levels to minimize wait times in the emergency room.
Furthermore, digital twins can predict equipment failures and optimize maintenance schedules, reducing downtime and improving the availability of critical medical resources. This proactive approach to resource management minimizes disruptions and improves the overall quality of care.
Future Applications of Digital Twins in Healthcare, Study widespread digital twins healthcare
The potential applications of digital twins in healthcare are vast and continue to expand. Here are some potential future applications:
- Disease Prevention and Public Health: Digital twins can be used to model the spread of infectious diseases, predict outbreaks, and evaluate the effectiveness of public health interventions. This allows for more proactive and targeted public health strategies.
- Personalized Rehabilitation: Digital twins can be used to create personalized rehabilitation plans, adapting exercises and therapies based on individual patient progress and responses.
- Mental Health: Digital twins could model the impact of different therapeutic interventions on mental health conditions, leading to more personalized and effective treatment strategies.
- Drug Repurposing: Digital twins can accelerate the process of identifying existing drugs that could be repurposed to treat new diseases.
- Longitudinal Patient Monitoring: Digital twins can track patient health over time, providing early warnings of potential health problems and allowing for timely interventions.
Data Management and Security in Digital Twin Systems
The burgeoning field of digital twins in healthcare promises revolutionary advancements, but its success hinges critically on robust data management and ironclad security protocols. The sheer volume, sensitivity, and complexity of patient data involved demand a sophisticated approach that prioritizes both privacy and accuracy. Failure to address these crucial aspects could severely undermine the trustworthiness and widespread adoption of this transformative technology.The importance of data security and privacy in the context of digital twins cannot be overstated.
Digital twins inherently contain highly sensitive personal health information (PHI), including medical records, genetic data, and real-time physiological readings. Unauthorized access or breaches could lead to significant harm, including identity theft, medical errors, and reputational damage for both patients and healthcare providers. Moreover, regulatory compliance, such as HIPAA in the United States and GDPR in Europe, mandates stringent security measures to protect patient data.
Data Integrity and Accuracy in Digital Twin Systems
Maintaining the integrity and accuracy of data within a digital twin system is paramount for reliable simulations and informed decision-making. Data inaccuracies can lead to flawed diagnoses, ineffective treatments, and potentially harmful outcomes. Methods for ensuring data integrity include employing rigorous data validation techniques at every stage of the data lifecycle, from data acquisition to storage and analysis.
This includes implementing checksums and data versioning to detect and correct errors, and employing automated data quality checks to identify and flag inconsistencies. Regular audits and reconciliation processes further bolster data accuracy. Real-time data synchronization mechanisms, ensuring consistency between the digital twin and the real-world counterpart, are also crucial.
Framework for Managing Massive Datasets
Digital twin systems require the management of massive and heterogeneous datasets, encompassing various data types such as images, sensor readings, genomic information, and electronic health records. A robust framework should leverage cloud-based storage solutions with scalable capacity and high availability. Data should be organized using a structured approach, such as a data lake or data warehouse, facilitating efficient data retrieval and analysis.
Metadata management is crucial for tracking data provenance, quality, and access permissions. Employing data compression and efficient indexing techniques can significantly improve query performance and reduce storage costs. For example, a large hospital system might utilize a cloud-based data lake to store patient data, structured data from EHRs, and unstructured data like medical images. Data would be organized by patient ID and indexed for efficient retrieval.
This setup allows for scalability and easy access for researchers and clinicians.
Data Governance and Regulatory Compliance
Effective data governance is essential for ensuring compliance with relevant regulations and maintaining patient trust. This involves establishing clear data ownership, access control policies, and data retention guidelines. Data governance should encompass the entire data lifecycle, from data collection to disposal. A comprehensive data governance framework needs to define roles and responsibilities, establish data quality standards, and implement mechanisms for data breach detection and response.
Compliance with regulations such as HIPAA and GDPR requires the implementation of security measures like encryption, access controls, and audit trails. Regular security assessments and penetration testing help identify vulnerabilities and ensure ongoing compliance. For instance, a hospital implementing a digital twin system for heart failure patients must adhere to HIPAA guidelines, encrypting all PHI, implementing strong access controls, and maintaining detailed audit trails of data access.
Regular security audits are vital to ensure continuous compliance.
The Future of Digital Twins in Healthcare
The potential of digital twins in healthcare is vast, extending far beyond current applications. As technology advances, particularly in artificial intelligence and data interoperability, we can expect to see a dramatic transformation in how healthcare is delivered and experienced. This evolution will be marked by more personalized, proactive, and efficient care, ultimately leading to improved patient outcomes.The integration of AI and machine learning will be a cornerstone of this transformation.
Artificial Intelligence and Machine Learning in Digital Twin Development
AI and machine learning will significantly enhance the capabilities of digital twins. AI algorithms can analyze massive datasets from various sources – genomic data, medical imaging, wearable sensor data, electronic health records – to create far more sophisticated and accurate digital representations of patients. This allows for predictive modeling of disease progression, personalized treatment planning, and the identification of individuals at high risk of developing specific conditions.
For instance, an AI-powered digital twin could predict the likelihood of a heart attack based on a patient’s genetic predisposition, lifestyle factors, and real-time physiological data, enabling proactive interventions. Machine learning algorithms can continuously refine the digital twin model, adapting to changes in the patient’s condition and improving the accuracy of predictions over time. This iterative learning process will make digital twins increasingly powerful tools for preventative care.
Interoperability and Data Sharing in Digital Twin Ecosystems
The true power of digital twins will be unlocked through seamless interoperability and data sharing across healthcare systems. Currently, data silos hinder the comprehensive view necessary for effective digital twin creation and utilization. Future digital twin ecosystems will rely on standardized data formats and secure data exchange protocols, enabling the integration of information from diverse sources, including hospitals, clinics, labs, and wearable devices.
My current research focuses on the widespread adoption of digital twins in healthcare; it’s fascinating to see how this technology is revolutionizing patient care. The potential is huge, and initiatives like the one highlighted in this article on the ai most exciting healthcare technology center connected medicine UPMC show just how far we can push the boundaries.
Ultimately, understanding how AI and digital twins interact will be key to improving healthcare outcomes on a global scale.
Imagine a scenario where a patient’s digital twin seamlessly integrates data from their smartwatch, genetic testing results from a specialized lab, and their electronic health record from their primary care physician. This holistic view will allow for a more comprehensive understanding of the patient’s health, leading to better diagnoses and more effective treatments. The establishment of secure and ethical data governance frameworks will be crucial to ensure patient privacy and data security within these interconnected systems.
Transformation of Healthcare Delivery and Improved Patient Outcomes
The widespread adoption of digital twins promises a significant transformation of healthcare delivery, leading to improved patient outcomes across various domains. Digital twins will empower personalized medicine, enabling tailored treatment plans based on individual patient characteristics and predicted responses to therapies. This approach moves away from a “one-size-fits-all” approach to a more precise and effective strategy. Furthermore, digital twins can facilitate proactive healthcare, allowing for early detection and intervention of potential health issues before they become serious.
This can be particularly impactful in managing chronic conditions like diabetes or heart failure, enabling timely adjustments to treatment plans and preventing costly hospitalizations. By simulating the effects of different treatment options within the digital twin environment, healthcare providers can make more informed decisions, optimizing treatment plans and minimizing adverse effects.
A Hypothetical Future Scenario with Widespread Digital Twin Use
Imagine a future where every patient has a personalized digital twin. A patient experiencing unusual symptoms uses a smartphone app to upload data from their wearable device. The app, connected to a secure cloud-based digital twin platform, instantly analyzes the data, comparing it to the patient’s digital twin model and flagging potential concerns. The platform alerts the patient’s physician, who can remotely review the data and initiate appropriate tests or interventions.
If hospitalization is necessary, the physician already possesses a comprehensive understanding of the patient’s condition, allowing for more effective treatment planning and potentially shorter hospital stays. For providers, the system provides real-time insights into patient populations, enabling proactive resource allocation and improved healthcare system efficiency. For patients, it translates to more personalized, proactive, and efficient care, leading to better health outcomes and a higher quality of life.
This seamless integration of technology and healthcare represents a significant leap forward in patient care, making healthcare more accessible, efficient, and effective.
Conclusive Thoughts: Study Widespread Digital Twins Healthcare
The journey into the world of widespread digital twins in healthcare reveals a landscape brimming with both promise and peril. While the potential to revolutionize medicine and improve patient outcomes is undeniable, navigating the complexities of data security, ethical considerations, and technological barriers is paramount. The future of healthcare hinges on responsible innovation, and the successful integration of digital twins will require a collaborative effort from researchers, clinicians, policymakers, and patients alike.
As we move forward, embracing the opportunities while mitigating the risks will be crucial in shaping a future where digital twins truly transform healthcare delivery for the better.
Popular Questions
What are the main privacy concerns surrounding patient digital twins?
The sensitive nature of medical data used to create digital twins raises significant privacy concerns. Robust security measures and strict adherence to data protection regulations are crucial to prevent unauthorized access and misuse of patient information.
How expensive is it to create and maintain a digital twin system?
The cost varies greatly depending on the complexity of the twin, the data sources used, and the level of sophistication of the analytical tools employed. It’s a significant investment, but the potential long-term cost savings and improved outcomes could justify the expense.
What role do patients play in the creation and use of their digital twins?
Patient consent and control over their data are crucial. Transparent communication about data usage and the ability for patients to access and manage their digital twin information are essential for building trust and ethical implementation.