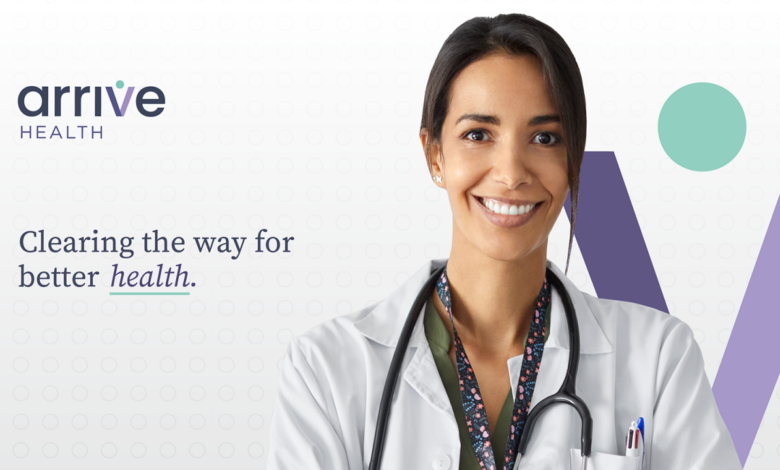
Insurance Lobby Blasts Medicare Advantage Audit Rule
Insurance lobby blasts Medicare Advantage audit rule – that headline alone speaks volumes! This isn’t just another bureaucratic squabble; it’s a clash between powerful interests and the potential for significant changes to how millions of seniors receive healthcare. We’re diving deep into this contentious issue, exploring the arguments from both sides, the potential impacts on Medicare Advantage plans, and what it all means for you and your healthcare future.
Get ready to unravel this complex web of healthcare politics!
The recent announcement of stricter audits for Medicare Advantage plans has ignited a firestorm of debate. The insurance industry, naturally, is pushing back hard, citing concerns about increased costs, reduced services, and potential disruptions to the system. But the government argues these audits are crucial for ensuring taxpayer dollars are being spent efficiently and that seniors are receiving the care they deserve.
This isn’t just a fight over money; it’s a battle over the future of Medicare Advantage itself.
The Insurance Lobby’s Arguments
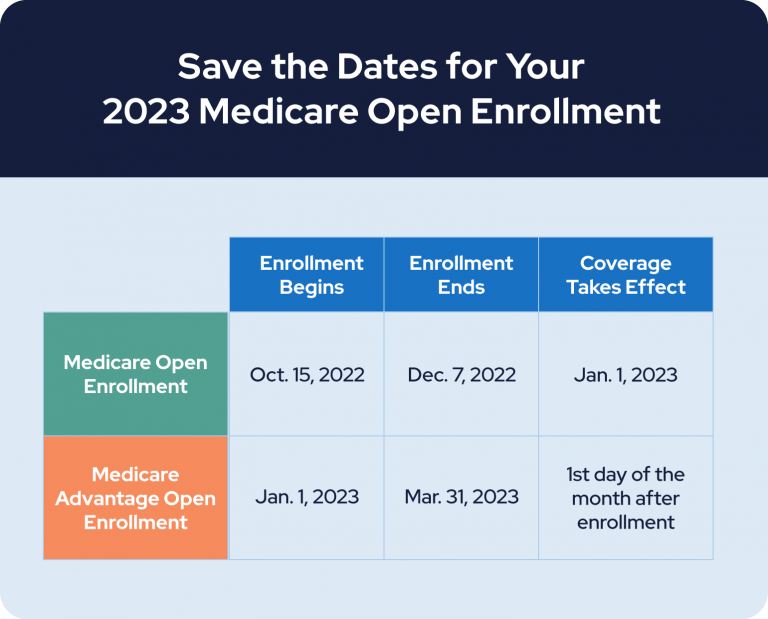
Source: retireguide.com
The recent Medicare Advantage audit rule has ignited a fierce debate between the Centers for Medicare & Medicaid Services (CMS) and the insurance industry. The insurance lobby has mounted a robust opposition, arguing the rule is overly burdensome, costly, and ultimately detrimental to beneficiaries. Their arguments center on the potential disruption to their operations and the perceived negative consequences for Medicare Advantage (MA) plans.
Core Arguments Against the Medicare Advantage Audit Rule
The insurance lobby’s core arguments against the intensified audits focus on several key concerns. They contend that the expanded audits are excessively intrusive, placing an undue financial and administrative burden on MA plans. This burden, they argue, will ultimately lead to reduced plan offerings, higher premiums for beneficiaries, and a decline in the quality of care. They further claim the audits are based on flawed methodologies and will lead to inaccurate and unfair financial adjustments.
Specific Concerns Regarding Operational Impact
The increased scrutiny from the audits, according to the insurance lobby, will necessitate significant investments in compliance and auditing infrastructure. This includes hiring additional staff, developing new internal controls, and investing in advanced data analytics systems. They foresee a diversion of resources away from core operational functions, such as improving member services and expanding plan benefits. The time and resources spent on responding to audits, they argue, could detract from efforts to enhance the quality of care provided to Medicare beneficiaries.
For example, a small MA plan might struggle to afford the necessary compliance staff, potentially leading to errors and penalties.
Predicted Negative Consequences for Medicare Advantage Plans
The insurance lobby predicts several negative consequences resulting from the intensified audits. They argue that the increased cost of compliance will inevitably lead to higher premiums for beneficiaries. They also suggest that the uncertainty surrounding the audits may discourage new MA plans from entering the market, reducing competition and limiting choices for seniors. Furthermore, they fear that the threat of significant financial penalties could lead to a reduction in the benefits offered by MA plans, impacting the quality of care provided.
For example, a plan might cut back on preventive services or reduce access to specialized care to offset the increased auditing costs.
Comparison of Arguments: Proponents vs. Opponents, Insurance lobby blasts medicare advantage audit rule
Argument | Supporting Evidence | Counterargument | Rebuttal |
---|---|---|---|
Increased audit burden will raise administrative costs and premiums. | Estimates of increased staffing and compliance costs; projections of premium increases based on compliance expenses. | Audits are necessary to ensure accurate payments and prevent fraud, waste, and abuse. | The current audit methodology is flawed and leads to inaccurate findings, resulting in unfair financial adjustments and disproportionate burden on plans. A more targeted and less burdensome approach is needed. |
Audits will stifle innovation and reduce competition in the MA market. | Anecdotal evidence of plans reducing benefits or delaying expansion due to audit concerns; predictions of reduced market entry by new plans. | Increased scrutiny promotes accountability and transparency, leading to better plans and improved beneficiary outcomes. | The current level of scrutiny is excessive and disproportionate, outweighing any potential benefits. A more balanced approach would incentivize innovation while maintaining appropriate oversight. |
Audits divert resources from improving beneficiary care. | Examples of plans reallocating resources from member services and benefit enhancement to audit compliance. | Audits ensure taxpayer dollars are spent efficiently and effectively. | While efficiency is important, the current approach prioritizes audit compliance over direct beneficiary care, potentially harming beneficiaries. A more streamlined process could improve both efficiency and quality of care. |
The Medicare Advantage Audit Rule’s Objectives
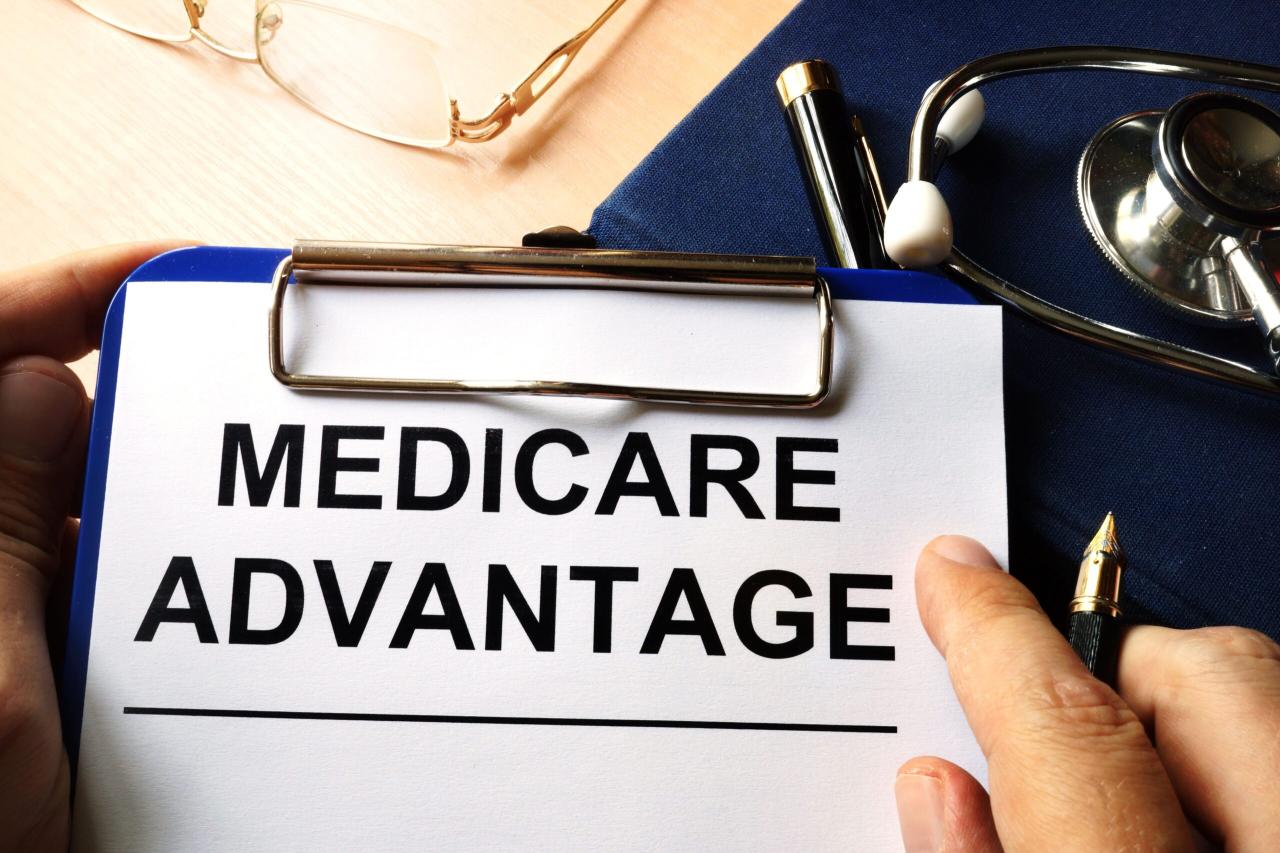
Source: buffalohealthyliving.com
The Medicare Advantage (MA) audit rule, officially known as the Centers for Medicare & Medicaid Services (CMS) audit program, aims to ensure the accuracy and integrity of payments made to MA plans. This is crucial because MA plans, which serve a significant portion of Medicare beneficiaries, receive substantial federal funding. The rule seeks to address concerns about potential overpayments and ensure that taxpayer dollars are used efficiently and effectively.The rule directly addresses several key issues within the Medicare Advantage program.
These issues include potential inaccuracies in risk adjustment coding, leading to inflated payments; inconsistencies in the reporting of beneficiary diagnoses and care provided; and a lack of sufficient oversight to ensure plans are accurately reflecting the true cost of caring for their enrollees. These concerns, if left unaddressed, could lead to significant financial losses for the Medicare program and ultimately, to higher costs for taxpayers.
Improved Accuracy and Efficiency of Medicare Advantage Payments
The audit rule is designed to improve the accuracy and efficiency of Medicare Advantage payments by implementing a robust audit process. This involves rigorous reviews of MA plans’ billing practices, claims data, and risk adjustment models. By identifying and correcting errors, the rule aims to reduce the incidence of overpayments and ensure that payments accurately reflect the actual costs of providing care.
This, in turn, strengthens the financial stability of the Medicare program. For example, if an audit reveals systematic overcoding of diagnoses, the corrected payments could save millions of dollars annually. The process also serves as a deterrent against fraudulent billing practices.
Benefits for Medicare Beneficiaries and Taxpayers
The primary benefits of the audit rule extend to both Medicare beneficiaries and taxpayers. For beneficiaries, a more accurate and efficient payment system ensures the continued viability of the Medicare Advantage program, protecting access to quality healthcare. For taxpayers, the rule safeguards against wasteful spending and ensures that Medicare dollars are used responsibly. By reducing overpayments, the rule helps to contain the growth of Medicare spending, ultimately contributing to a more sustainable healthcare system.
The savings generated through the audits can be redirected to improve other aspects of the Medicare program or to reduce the overall burden on taxpayers.
Key Steps in the Medicare Advantage Audit Process
The audit process typically involves several key steps:
- Plan Selection: CMS selects a sample of MA plans for audit based on risk factors, such as high payment rates or unusual patterns in claims data.
- Data Collection: Auditors request extensive data from the selected plans, including membership rosters, claims data, and risk adjustment models.
- Data Analysis: Auditors analyze the collected data to identify potential areas of concern, such as discrepancies between reported diagnoses and medical records.
- On-Site Reviews (if necessary): In some cases, auditors may conduct on-site reviews of the plan’s operations to gather further information and verify data.
- Audit Report: The auditors issue a report detailing their findings, including any identified overpayments or underpayments.
- Payment Adjustment: Based on the audit report, CMS may adjust payments to the MA plan to reflect the actual costs of care.
Potential Impacts on Medicare Advantage Plans
The intensified scrutiny brought about by the new Medicare Advantage audit rule will undoubtedly reshape the landscape of the Medicare Advantage market. The financial implications, changes in operational practices, and shifts in market dynamics are likely to be significant and far-reaching, impacting both the plans themselves and the beneficiaries they serve. This section explores these potential impacts in detail.
Financial Implications for Medicare Advantage Plans
The increased audit activity will inevitably lead to higher administrative costs for Medicare Advantage plans. Plans will need to dedicate more resources to compliance, including hiring additional staff, investing in new software and systems, and conducting internal audits to ensure accuracy in billing and reporting. This added expense could potentially reduce profitability, particularly for smaller plans with fewer resources.
For example, a smaller plan might see a 5-10% increase in administrative costs, impacting their bottom line and potentially leading to adjustments in provider contracts or benefit structures. Larger plans, while better equipped to handle increased scrutiny, will also face substantial added expenses, although the percentage increase might be lower. The ultimate financial impact will depend on the plan’s current compliance infrastructure, the thoroughness of the audits, and the extent of any identified overpayments.
Changes in Service Offerings and Administrative Practices
In response to the heightened risk of audits and potential penalties, Medicare Advantage plans are likely to implement several changes. We can expect a greater emphasis on rigorous internal controls and improved data management systems to ensure accurate reporting and billing. This might involve investing in sophisticated data analytics tools to identify and correct potential errors before they are flagged by auditors.
Some plans might also choose to streamline their service offerings, focusing on the most profitable and least complex services to minimize administrative burden and reduce the risk of audit findings. Additionally, there could be a shift towards more standardized processes and documentation to facilitate easier auditing and compliance.
Impact on Enrollment and Competition
The increased scrutiny and potential financial pressures could affect enrollment and competition in the Medicare Advantage market. Plans facing higher administrative costs might need to increase premiums, potentially leading to a decrease in enrollment. This could be particularly challenging for plans already operating on thin margins. Conversely, plans that demonstrate strong compliance and efficient operations might gain a competitive advantage, attracting more enrollees seeking stability and reliability.
The insurance lobby’s furious response to the new Medicare Advantage audit rule is understandable – they’re fighting for their bottom line. But this fight overshadows a crucial development: a recent study, study widespread digital twins healthcare , suggests that better data management through technology could actually improve efficiency and reduce fraud, ultimately benefiting both patients and insurers.
Ironically, the very technologies highlighted in this study could help address the concerns driving the insurance lobby’s opposition to the audits.
The rule could also lead to consolidation in the market, with smaller, less financially resilient plans being absorbed by larger organizations better equipped to handle the increased regulatory burden.
Differential Impact on HMOs and PPOs
The impact of the audit rule might vary depending on the type of Medicare Advantage plan. HMOs, with their more tightly controlled networks and simpler administrative structures, might experience a relatively less severe impact compared to PPOs. PPOs, with their broader networks and more complex billing processes, might face greater challenges in ensuring compliance and managing the increased audit scrutiny.
This could potentially lead to a shift in market share towards HMOs, as they may be perceived as less risky by beneficiaries.
Hypothetical Scenario: Impact on a Specific Medicare Advantage Plan
Let’s consider “Sunshine Health,” a medium-sized Medicare Advantage PPO plan serving a geographically dispersed population. Before the new audit rule, Sunshine Health’s administrative costs were around 12% of its total revenue. Following the implementation of the rule, the plan anticipates a 5% increase in administrative costs due to enhanced compliance measures, including additional staff, software upgrades, and increased internal audit frequency.
This increase translates to an additional $5 million in annual expenses. To offset this, Sunshine Health might need to increase premiums by a small percentage or slightly reduce its provider network to control costs, potentially impacting the choice of providers available to its members.
Public Perception and Political Context: Insurance Lobby Blasts Medicare Advantage Audit Rule
The Medicare Advantage program, while offering supplemental benefits beyond traditional Medicare, has become a focal point of political debate, particularly with the recent announcement of increased audits. Public perception is complex, influenced by factors such as personal experiences, media coverage, and political affiliations. Understanding this nuanced landscape is crucial to analyzing the implications of the audit rule.The political context surrounding the Medicare Advantage audit rule is highly charged, involving a complex interplay of interests from various stakeholders.
The rule’s announcement triggered immediate and strong reactions, highlighting the deep divisions on the issue.
Public Opinion on Medicare Advantage and the Audit Rule
Public opinion regarding Medicare Advantage is divided. Many seniors appreciate the supplemental benefits and choice of plans, leading to positive perceptions. However, concerns exist about potential cost increases, limitations on access to care, and the complexities of navigating the system. The audit rule has further polarized public opinion. Those who trust the government’s need to ensure program integrity view the audits favorably.
Others, often aligned with the insurance industry’s messaging, express concerns about potential disruptions to care and increased premiums. Polling data reveals a lack of widespread public awareness of the specifics of the audit rule, suggesting that public perception is heavily influenced by media framing and political messaging.
Political Actors and Their Positions
Several key political figures and organizations have taken strong stances on the Medicare Advantage audit rule. The Biden administration, while supporting the need for program integrity, has faced criticism from some quarters for the scale and approach of the audits. Republican lawmakers, largely aligned with the insurance industry, have voiced strong opposition, framing the audits as an attack on a successful program and a threat to seniors’ access to healthcare.
The insurance lobby’s furious reaction to the new Medicare Advantage audit rule is understandable, given the potential financial impact. This increased scrutiny comes at a time when rural hospitals are already struggling, as highlighted in this insightful article on the challenges facing Rural Hospitals Labor Delivery & services. Ultimately, these issues – tighter regulations and resource scarcity – both point to a larger crisis in healthcare access and affordability.
Conversely, Democratic lawmakers, while acknowledging potential negative consequences, have generally supported the audits, emphasizing the importance of preventing fraud, waste, and abuse within the Medicare Advantage system. The AARP, a powerful advocacy group for older Americans, has adopted a cautious approach, calling for a transparent and fair audit process while expressing concerns about potential negative impacts on beneficiaries.
The insurance industry, represented by groups such as America’s Health Insurance Plans (AHIP), has mounted a significant lobbying effort against the rule, arguing it will lead to higher costs and reduced access to care.
Timeline of Key Events
A clear timeline helps understand the evolution of the situation. This is a simplified version, and further research is needed for comprehensive detail.
Date | Event | Significance |
---|---|---|
[Insert Date – CMS announcement of increased audits] | CMS announces increased audits of Medicare Advantage plans. | This marks the trigger point for the intense political debate. |
[Insert Date – Insurance lobby launches campaign] | The insurance lobby launches a major public relations campaign against the audit rule. | This signals the start of a concerted effort to influence public opinion and policy. |
[Insert Date – Congressional hearings] | Congressional hearings are held to discuss the audit rule. | These hearings provide a platform for various stakeholders to express their views. |
[Insert Date – Further CMS statements/actions] | CMS releases further statements or takes additional actions regarding the audits. | This reflects the ongoing dynamic nature of the situation. |
Alternative Approaches and Solutions
The intense backlash against the new Medicare Advantage audit rule highlights the need for a more nuanced approach to ensuring program integrity. While the goals of reducing fraud and abuse are laudable, the current methodology has sparked significant concerns regarding its impact on plan operations and beneficiary access to care. Exploring alternative strategies is crucial to achieving the desired outcomes without causing undue disruption.The current audit methodology focuses heavily on retrospective reviews, which are costly and time-consuming.
This approach often leads to disputes and delays in payments, impacting both plans and beneficiaries. Alternative strategies should prioritize proactive measures and data-driven analysis to identify potential problems before they escalate.
Data Analytics and Predictive Modeling
Employing sophisticated data analytics and predictive modeling can significantly improve the efficiency and effectiveness of audits. By analyzing large datasets encompassing claims data, provider information, and beneficiary demographics, algorithms can identify patterns indicative of fraud, waste, or abuse. This proactive approach allows for targeted audits, reducing the need for extensive retrospective reviews of all claims. For example, identifying unusually high utilization rates for specific services among a particular group of providers could flag potential issues warranting further investigation.
This method is already successfully employed in other sectors, such as credit card fraud detection, and could be adapted to the Medicare Advantage context.
Risk-Based Auditing
Instead of a blanket approach, a risk-based auditing system would focus resources on plans and providers deemed higher risk. This involves stratifying plans based on factors such as prior audit findings, claims patterns, and provider network characteristics. Plans with a higher risk profile would undergo more rigorous scrutiny, while those with a clean record could benefit from less intensive audits.
This targeted approach would optimize the use of limited audit resources, minimizing disruption to low-risk plans. The Centers for Medicare & Medicaid Services (CMS) could leverage existing data and analytics to create a risk stratification model, prioritizing plans and providers most likely to engage in questionable practices.
Streamlined Audit Processes and Dispute Resolution
The current audit process is often criticized for its complexity and length. Streamlining the process, clarifying audit procedures, and establishing clear timelines for dispute resolution can significantly reduce administrative burdens and costs. This could involve implementing standardized audit protocols, utilizing electronic data exchange, and providing clear guidance to plans on compliance requirements. Furthermore, establishing an independent and impartial dispute resolution mechanism could help address conflicts between plans and CMS more efficiently.
This could potentially involve mediation or arbitration, ensuring a fair and timely resolution of disputes.
The insurance lobby’s furious reaction to the new Medicare Advantage audit rule is understandable, given the potential impact on their profits. It makes you wonder if streamlining administrative tasks, like with the amazing advancements in AI, could help. For example, check out how Nuance is integrating generative AI scribe with Epic EHRs – nuance integrates generative ai scribe epic ehrs – which could potentially reduce administrative burden and, maybe, even lessen the heat on the insurers.
Ultimately, though, the fight over the audit rule is far from over.
Comparative Analysis of Auditing Approaches
Approach | Advantages | Disadvantages | Cost Implications |
---|---|---|---|
Retrospective Audits (Current Method) | Comprehensive review of claims; identifies all instances of fraud, waste, and abuse. | Costly, time-consuming, disruptive to plan operations, prone to disputes. | High |
Data Analytics and Predictive Modeling | Proactive, efficient, targets high-risk areas, reduces unnecessary audits. | Requires significant investment in data infrastructure and expertise; potential for false positives. | Moderate to High (initial investment, then lower ongoing costs) |
Risk-Based Auditing | Efficient allocation of resources, minimizes disruption to low-risk plans. | Requires robust risk stratification model; potential for overlooking low-risk fraud. | Moderate |
Streamlined Audit Processes | Reduces administrative burden, faster dispute resolution, improved transparency. | May require significant upfront investment in process improvement. | Moderate (upfront investment, then lower ongoing costs) |
Final Wrap-Up
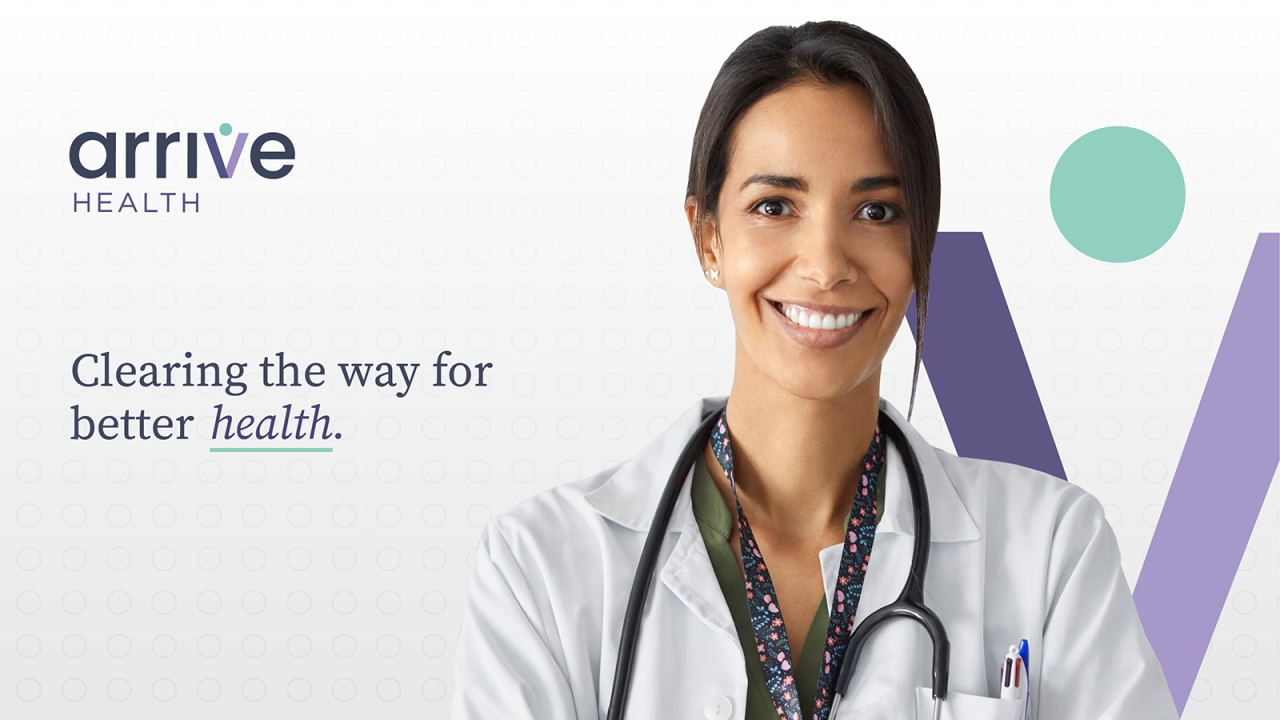
Source: arrivehealth.com
The fight over the Medicare Advantage audit rule is far from over. The insurance lobby’s powerful opposition is creating a significant hurdle, but the need for accountability and transparency within the Medicare Advantage program remains undeniable. The coming months will likely see continued debate, lobbying efforts, and perhaps even legal challenges. Ultimately, the outcome will significantly impact millions of seniors and the future of healthcare financing in the United States.
Staying informed and engaged is crucial – your voice matters in shaping the future of Medicare.
Essential Questionnaire
What exactly is a Medicare Advantage plan?
Medicare Advantage plans are private health insurance plans that offer Medicare benefits. They are an alternative to Original Medicare (Parts A and B).
Why are the audits necessary?
Audits are intended to identify and prevent fraud, waste, and abuse within the Medicare Advantage system, ensuring taxpayer money is used responsibly and beneficiaries receive appropriate care.
How will this affect my Medicare Advantage premiums?
The impact on premiums is uncertain and depends on the outcome of the audit process and how individual plans respond. Some plans might see increased costs, potentially leading to higher premiums for beneficiaries.
What are the potential consequences if the insurance lobby wins?
If the insurance lobby successfully weakens or blocks the audits, it could lead to less accountability, potentially allowing waste and fraud to continue within the Medicare Advantage system.