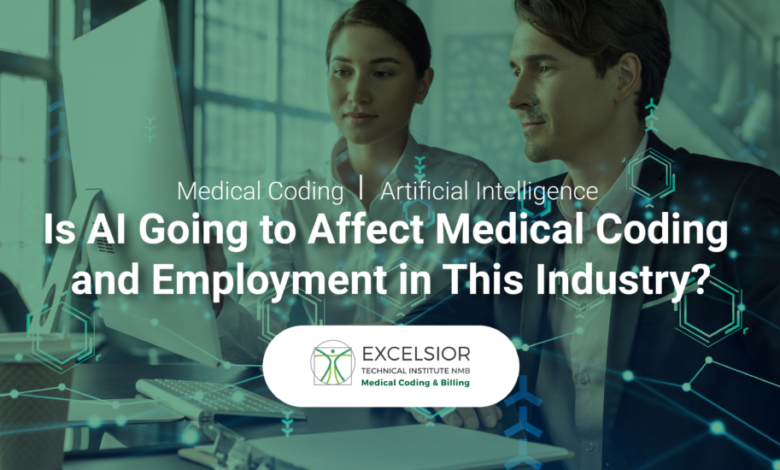
The AI Powered Solution to the Medical Coding Worker Shortage
The AI powered solution to the medical coding worker shortage is no longer a futuristic fantasy; it’s a rapidly evolving reality. Facing a critical shortage of skilled medical coders, the healthcare industry is increasingly turning to artificial intelligence to automate tasks, improve accuracy, and ultimately, ensure timely and efficient patient care. This exploration delves into the current crisis, the innovative AI solutions emerging, and the potential impact on the future of medical coding.
We’ll examine the various AI-powered tools available, their strengths and weaknesses, and the ethical considerations surrounding their implementation. From automating code assignment to enhancing chart review, we’ll explore how AI is reshaping the medical coding landscape and its potential to alleviate the current workforce crisis. We’ll also look at real-world examples of successful (and unsuccessful) AI implementations, providing valuable insights for healthcare providers considering this transformative technology.
The Current Medical Coding Landscape: The Ai Powered Solution To The Medical Coding Worker Shortage
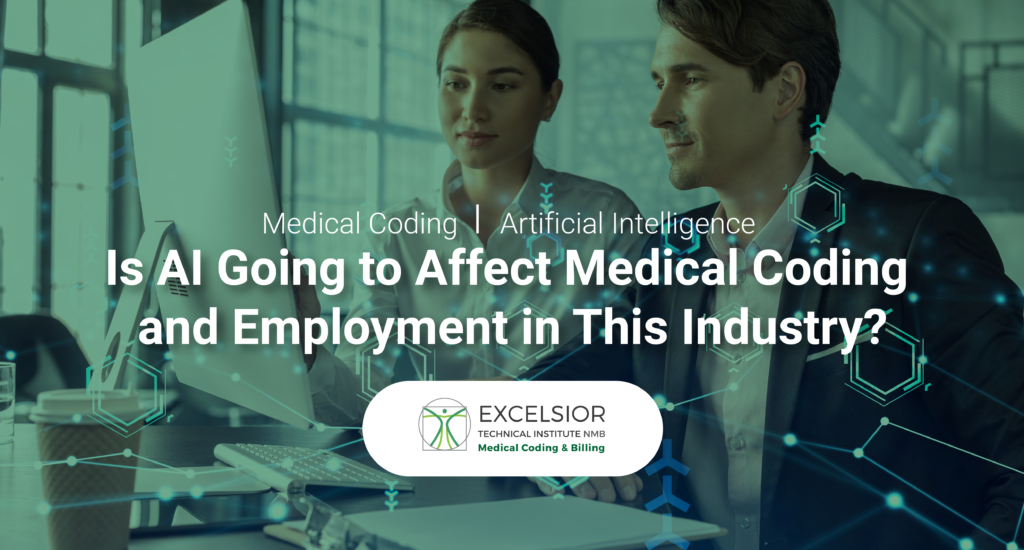
Source: excelsiortechnicalnmb.com
The medical coding profession, crucial for the smooth functioning of healthcare systems, is facing a significant crisis. The combination of increasing complexity in medical procedures and a shrinking workforce is creating a perfect storm, impacting both healthcare providers and patients. This section delves into the challenges, causes, and consequences of this critical shortage.
The current landscape is characterized by a widening gap between the demand for skilled medical coders and the available supply. This isn’t just a matter of inconvenience; it’s a serious threat to the efficiency and financial stability of healthcare organizations, and ultimately, the quality of patient care.
Challenges Faced by Medical Coding Professionals
Medical coding is a demanding profession requiring a high level of accuracy, attention to detail, and a deep understanding of medical terminology, anatomy, physiology, and ever-evolving coding guidelines. The constant updates to coding systems (like ICD-10 and CPT codes) necessitate continuous learning and adaptation, adding to the already considerable workload. Furthermore, the pressure to maintain high accuracy rates while managing large volumes of charts under tight deadlines contributes to high stress levels and burnout.
Contributing Factors to the Medical Coding Worker Shortage, The ai powered solution to the medical coding worker shortage
Several interconnected factors contribute to the current shortage. Firstly, the profession is often perceived as demanding and underpaid relative to its complexity and responsibility. This leads to low recruitment and high turnover rates. Secondly, the aging workforce, with many experienced coders nearing retirement, exacerbates the problem. Finally, the lack of sufficient training programs and educational resources to meet the growing demand further compounds the issue.
The increasing complexity of medical procedures and the rise in electronic health records (EHR) also add to the workload and require more advanced skills.
Impact of the Shortage on Healthcare Systems and Patient Care
The consequences of the medical coding shortage are far-reaching. Delayed or inaccurate coding leads to significant financial losses for healthcare providers due to denied or delayed claims payments from insurance companies. This financial strain can impact a provider’s ability to invest in new technologies, hire additional staff, or provide necessary services. Moreover, delayed processing of medical records can impede timely access to patient care, impacting diagnosis and treatment.
Inaccurate coding can also lead to errors in billing and patient records, potentially affecting the quality of care and creating legal or compliance risks.
Challenges, Causes, Impacts, and Consequences of the Medical Coding Shortage
Challenge | Cause | Impact on Healthcare | Impact on Patients |
---|---|---|---|
High workload and pressure | Increased volume of charts, complex medical procedures, tight deadlines | Delayed claim processing, financial losses, reduced efficiency | Delayed access to care, potential errors in treatment plans |
High turnover rate | Low pay, high stress, lack of career advancement opportunities | Increased training costs, loss of institutional knowledge | Inconsistency in coding accuracy, potential delays in billing and reimbursement |
Lack of qualified coders | Insufficient training programs, aging workforce, lack of career appeal | Backlog of unprocessed charts, financial instability, compromised quality of care | Longer wait times for appointments, potential errors in medical records |
Keeping up with coding updates | Frequent changes in coding guidelines (ICD-10, CPT codes), technological advancements | Increased training costs, need for continuous professional development | Potential errors in coding due to lack of updated knowledge |
AI-Powered Solutions
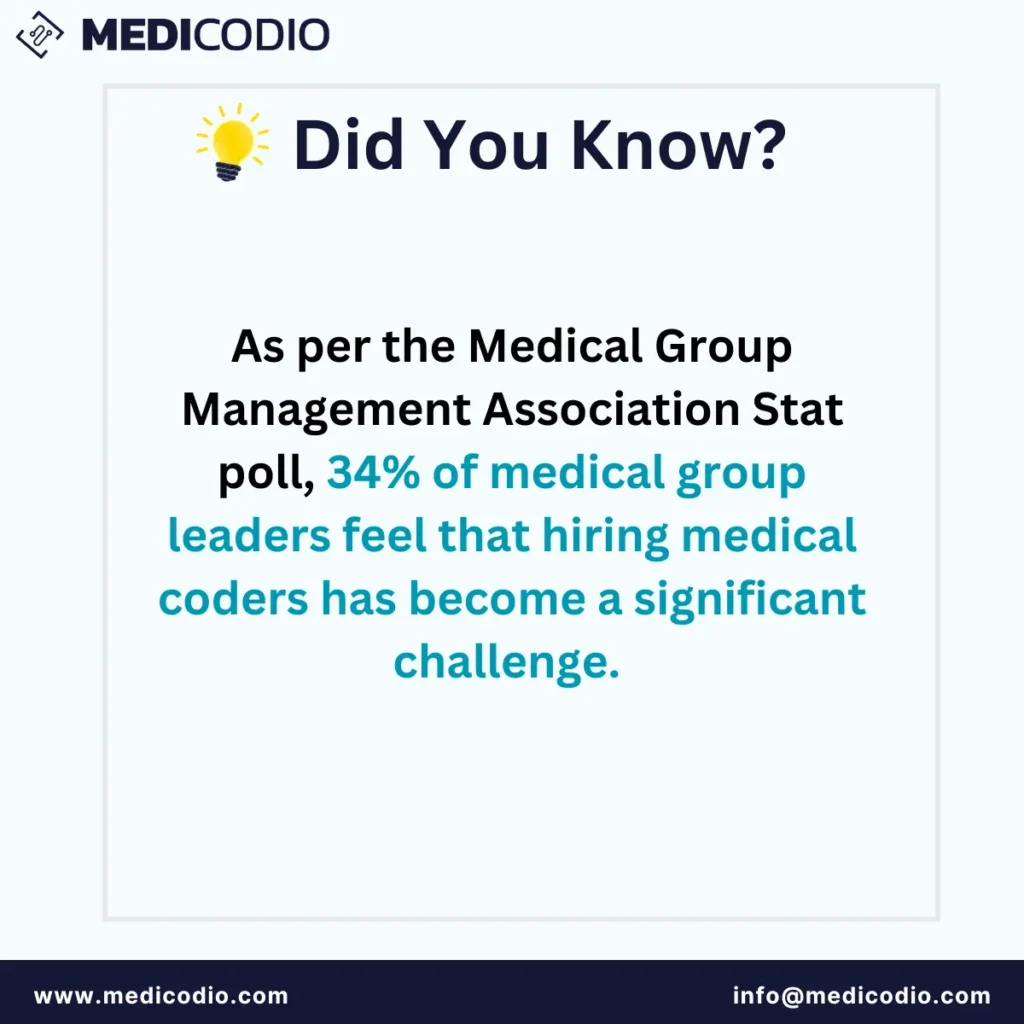
Source: medicodio.com
The medical coding industry is rapidly embracing artificial intelligence (AI) to address the persistent worker shortage. Several innovative solutions are emerging, each leveraging different AI techniques to automate various aspects of the medical coding process. These range from simple rule-based systems to sophisticated deep learning models, each offering a unique set of capabilities and limitations.
Types of AI-Powered Medical Coding Solutions
AI-powered medical coding solutions employ a variety of approaches, each with its strengths and weaknesses. These approaches can be broadly categorized based on the underlying AI techniques utilized. The most common categories include rule-based systems, machine learning (ML) models, and natural language processing (NLP) systems. These often work in conjunction with one another to create a comprehensive solution.
Rule-Based Systems
Rule-based systems utilize pre-defined rules and algorithms to process medical data and assign codes. These systems are relatively simple to implement and maintain, but their accuracy is limited by the comprehensiveness of the ruleset. They are often used for straightforward coding tasks or as a component within a larger, more complex AI system.
- Advantages: Relatively simple to implement and maintain, low computational cost, high explainability.
- Disadvantages: Limited accuracy and flexibility, struggles with complex or ambiguous cases, requires frequent updates to accommodate changes in coding guidelines.
Machine Learning (ML) Models
ML models, particularly supervised learning models, learn to predict medical codes from large datasets of coded medical records. These models can achieve high accuracy levels, especially when trained on high-quality data. However, they require significant amounts of training data and may struggle with unseen or unusual cases. Examples include support vector machines (SVMs), random forests, and neural networks.
- Advantages: High accuracy potential, ability to handle complex relationships in data, adaptability to new data.
- Disadvantages: Requires large amounts of training data, can be computationally expensive, may struggle with unseen or unusual cases, potential for bias if training data is not representative.
Natural Language Processing (NLP) Systems
NLP systems focus on understanding and interpreting unstructured clinical text, such as physician notes and discharge summaries. These systems utilize techniques like named entity recognition (NER) and relationship extraction to identify relevant medical information and map it to appropriate codes. They are crucial for automating the coding of free-text data, which often represents a significant portion of medical records.
- Advantages: Ability to process unstructured clinical text, automation of complex coding tasks, potential for improved efficiency and accuracy.
- Disadvantages: Requires significant computational resources, can be challenging to train and evaluate, susceptible to errors in interpreting ambiguous or poorly written text.
Key Features and Functionalities
Many AI-powered medical coding solutions share common features, such as automated code assignment, quality control checks, and integration with existing Electronic Health Record (EHR) systems. Advanced solutions may also offer features like predictive coding, which anticipates codes based on early clinical information, and real-time feedback to coders to improve their accuracy. Some systems even incorporate features to flag potentially erroneous coding for human review, thereby improving accuracy and reducing the risk of denials.
AI-powered solutions are revolutionizing healthcare, tackling issues like the medical coding worker shortage. This is crucial because timely and accurate coding is essential for patient care, as highlighted by the recent news of Monali Thakur’s hospitalization after struggling to breathe – read more about preventing respiratory diseases like hers here: monali thakur hospitalised after struggling to breathe how to prevent respiratory diseases.
Ultimately, freeing up human coders with AI allows for a more efficient and responsive healthcare system, improving outcomes for everyone.
For example, a system might highlight a potential discrepancy between the diagnosis and the procedure code assigned.
Specific Applications of AI in Medical Coding

Source: medicodio.com
AI is rapidly transforming the medical coding landscape, offering solutions to the persistent workforce shortage and improving both accuracy and efficiency. Its application extends beyond simple automation; AI is enhancing the entire coding process, from initial chart review to final code assignment. This allows coders to focus on more complex cases and improve overall patient care.AI’s ability to analyze large datasets and identify patterns makes it particularly well-suited for the intricacies of medical coding.
This section delves into specific applications, showcasing how AI is revolutionizing this critical field.
AI-Driven Code Assignment
AI algorithms, trained on vast amounts of medical data, can automatically assign codes to medical procedures and diagnoses. This automation significantly reduces the time coders spend on routine tasks, allowing them to focus on more complex cases requiring human judgment and expertise. For example, an AI system might analyze a patient’s chart noting a specific procedure and associated diagnosis, automatically assigning the correct CPT and ICD codes with a high degree of accuracy.
This not only saves time but also reduces the potential for human error in code selection. The AI can even flag potential inconsistencies or missing information, prompting human review where necessary, ensuring a robust and accurate coding process.
AI-Powered Chart Review and Data Extraction
AI can efficiently review medical charts, extracting relevant information needed for coding. This includes identifying key diagnostic statements, procedures performed, and other pertinent details. This task is often time-consuming for human coders, but AI can quickly and accurately process large volumes of unstructured data, such as physician notes and clinical reports. For instance, AI can identify key phrases indicating specific diagnoses or procedures, even within lengthy and complex physician notes, minimizing the need for manual searching and interpretation.
This streamlined process reduces the overall time spent on chart review, contributing to increased coding efficiency.
Improving Coding Accuracy and Efficiency through AI
AI algorithms, through machine learning, continuously improve their accuracy over time. As they process more data, they learn to identify subtle patterns and nuances in medical documentation, leading to increasingly precise code assignment. Studies have shown that AI-powered coding systems can achieve accuracy rates comparable to or exceeding those of human coders, particularly in high-volume, routine coding tasks. This increased accuracy translates to improved billing accuracy, reduced claim denials, and ultimately, better financial performance for healthcare providers.
Furthermore, the efficiency gains allow coding departments to handle a larger volume of charts with the same or fewer staff, addressing the workforce shortage directly.
Real-World Applications of AI in Medical Coding
Several companies have already successfully implemented AI-powered medical coding solutions. For example, some AI systems are used by large hospital systems to pre-code charts, identifying potential codes before human review. This allows coders to verify the AI’s suggestions and make any necessary corrections, significantly reducing the workload and improving turnaround times. Other companies offer AI-powered platforms that assist coders with code selection and compliance, providing real-time feedback and flagging potential errors.
These applications demonstrate the tangible benefits of AI in streamlining medical coding processes and enhancing accuracy.
Flowchart: AI-Assisted Medical Coding Process
Imagine a flowchart with the following steps:
1. Chart Upload
The medical chart (including physician notes, lab results, etc.) is uploaded into the AI system.
AI-powered medical coding is a game-changer, addressing the persistent worker shortage and boosting efficiency. However, the repetitive nature of some coding tasks can lead to repetitive strain injuries like carpal tunnel syndrome, which is why exploring preventative measures is crucial. Luckily, there are effective non-surgical options; check out this helpful resource on ways to treat carpal tunnel syndrome without surgery to keep your coding team healthy and productive.
By prioritizing employee well-being alongside technological advancements, we can build a sustainable and thriving medical coding future.
2. Data Extraction
AI algorithms analyze the chart, extracting relevant information such as diagnoses, procedures, and modifiers.
3. Code Suggestion
The AI suggests appropriate ICD and CPT codes based on the extracted information.
4. Coder Review
A human coder reviews the AI’s suggestions, verifying accuracy and making any necessary corrections.
5. Code Assignment
The final codes are assigned to the chart.
6. Quality Control
The coded chart undergoes a final quality control check to ensure accuracy and compliance.
7. Billing Submission
The coded information is used to generate and submit claims for billing.This streamlined process combines the speed and efficiency of AI with the judgment and expertise of human coders, creating a more robust and accurate medical coding workflow.
Addressing Ethical and Practical Considerations
The integration of AI into medical coding, while promising increased efficiency and accuracy, necessitates a careful examination of the ethical and practical implications. Successfully deploying AI in this field requires addressing potential pitfalls and ensuring responsible implementation to maximize benefits while minimizing risks. This section will delve into the key considerations that must be factored into any AI-powered medical coding strategy.
Data Privacy and Bias in AI Medical Coding
The use of AI in medical coding involves processing vast amounts of sensitive patient data. Maintaining patient privacy is paramount. Robust data anonymization and security protocols are essential to prevent unauthorized access and breaches of confidentiality, adhering to regulations like HIPAA. Furthermore, AI algorithms are trained on data, and if this data reflects existing societal biases (e.g., racial or socioeconomic disparities in healthcare access), the AI system may perpetuate and even amplify these biases in its coding decisions.
This can lead to inaccurate or unfair reimbursement for certain patient populations. Mitigation strategies include carefully curating training datasets to ensure representation of diverse patient groups and implementing ongoing monitoring for bias detection and correction.
Impact of AI on Human Medical Coders’ Roles
The introduction of AI will undoubtedly transform the roles of human medical coders. While AI can automate routine coding tasks, human expertise will remain crucial for complex cases, unusual diagnoses, and situations requiring nuanced clinical judgment. The shift will likely be towards a collaborative model, where coders focus on higher-level tasks like auditing AI-generated codes, handling exceptions, and improving the AI system’s accuracy through feedback.
Reskilling and upskilling initiatives will be vital to prepare medical coders for these evolving roles, focusing on areas such as AI oversight, data analysis, and clinical documentation improvement. For example, coders may become specialists in identifying and resolving discrepancies between AI-generated codes and clinical documentation.
Practical Challenges in Implementing and Maintaining AI Systems
Implementing and maintaining AI-powered medical coding systems presents several practical challenges. High initial investment costs for software, hardware, and data integration are a significant barrier. The need for ongoing system updates and maintenance to address bugs, adapt to changes in coding guidelines, and incorporate new medical terminology also adds to the cost. Furthermore, ensuring the accuracy and reliability of the AI system requires rigorous testing and validation, and continuous monitoring of its performance.
Data quality is crucial; inaccurate or incomplete data will lead to erroneous coding and potentially significant financial losses. Finally, integrating the AI system into existing workflows and training staff to use it effectively requires careful planning and execution.
AI-powered medical coding solutions are becoming increasingly crucial, offering a potential lifeline to healthcare systems struggling with staffing shortages. The recent news that Steward Health Care secured financing to emerge from bankruptcy, as reported by this article , highlights the financial pressures facing healthcare providers. This makes the efficiency and cost-effectiveness of AI in medical coding even more appealing, potentially helping systems like Steward navigate future challenges.
Cost-Benefit Analysis of AI in Medical Coding
Aspect | Costs | Benefits |
---|---|---|
Initial Investment | Software licenses, hardware, data integration, staff training | Improved coding accuracy, reduced operational costs, increased efficiency |
Ongoing Maintenance | System updates, maintenance contracts, technical support | Reduced error rates, minimized claim denials, improved revenue cycle management |
Human Resources | Reskilling/upskilling existing staff, potential hiring of AI specialists | Improved coder productivity, ability to handle more complex cases, reduced workload |
Risk Management | Data security measures, bias mitigation strategies, compliance with regulations | Reduced risk of coding errors, minimized financial penalties, enhanced patient safety |
Future Trends and Developments
The integration of AI in medical coding is still in its nascent stages, but the potential for transformative change is immense. Looking ahead, we can anticipate a future where AI not only alleviates the current worker shortage but fundamentally reshapes the medical coding landscape, leading to increased efficiency, accuracy, and ultimately, better patient care. This evolution will be driven by ongoing advancements in AI technology and a proactive approach to addressing the challenges of implementation.AI’s role in medical coding will evolve from a supplementary tool to a central component of the workflow.
Instead of simply automating repetitive tasks, AI will take on more complex responsibilities, such as nuanced code selection, identifying inconsistencies and errors with greater sophistication, and even proactively suggesting coding changes based on evolving medical guidelines and best practices. This shift will free up human coders to focus on higher-level tasks requiring critical thinking and clinical expertise, leading to a more efficient and fulfilling work environment.
Advancements in AI Technology for Medical Coding
Several technological advancements will propel the evolution of AI in medical coding. Natural Language Processing (NLP) will become increasingly sophisticated, enabling AI systems to understand and interpret complex medical documentation with greater accuracy and speed. Machine learning algorithms will continuously improve their ability to identify patterns and anomalies in medical records, leading to more accurate and consistent coding. The integration of AI with Electronic Health Records (EHR) systems will streamline data flow and automate many manual processes, further enhancing efficiency.
For example, imagine an AI system that not only codes the discharge summary but also cross-references it against prior records, flagging potential discrepancies or inconsistencies for human review. This level of automation could significantly reduce the risk of human error and improve the overall quality of coding.
Strategies for Mitigating AI Implementation Challenges
The successful implementation of AI in medical coding requires careful planning and consideration of several key factors. Data security and privacy are paramount. Robust security measures must be in place to protect sensitive patient information. Furthermore, ensuring the accuracy and reliability of AI systems is crucial. Rigorous testing and validation are essential to minimize errors and build trust among healthcare professionals.
Addressing potential job displacement concerns through reskilling and upskilling initiatives for medical coders is also vital for a smooth transition. This could involve training coders to work alongside AI systems, focusing on tasks requiring human judgment and expertise. Finally, transparent and explainable AI models are necessary to ensure accountability and build confidence in the technology. This requires developing AI systems that can clearly articulate their reasoning behind coding decisions.
A Hypothetical Future Scenario of Seamless AI Integration
Imagine a future where a physician dictates a patient’s discharge summary. The AI system, seamlessly integrated with the EHR, instantly transcribes the dictation, identifies relevant medical codes, and generates a complete coding report, all within seconds. The system flags any potential coding ambiguities or inconsistencies for the coder to review, highlighting the specific sections of the documentation requiring attention.
The coder, freed from repetitive tasks, focuses on resolving these ambiguities, leveraging their clinical expertise to ensure accuracy. The entire process is significantly faster, more accurate, and less prone to human error. The AI system also continuously learns and adapts, improving its accuracy over time based on the coder’s feedback and evolving medical guidelines. This collaborative approach maximizes the strengths of both AI and human expertise, creating a highly efficient and accurate medical coding workflow.
This scenario, while hypothetical, represents a realistic and achievable goal given the current trajectory of AI development in healthcare.
Case Studies
Analyzing real-world implementations of AI in medical coding reveals valuable lessons about both the potential successes and pitfalls of this technology. Understanding these experiences is crucial for organizations considering adopting AI-powered solutions. By examining both successful and unsuccessful deployments, we can identify key factors that contribute to positive outcomes and avoid common mistakes.
Successful AI Implementation: Cedars-Sinai Medical Center
Cedars-Sinai Medical Center, a large academic medical center in Los Angeles, reported a successful implementation of an AI-powered medical coding system. Their system, developed in partnership with a leading AI vendor, focused on automating the initial coding process for common procedures. The AI system analyzed patient charts, identifying relevant codes with a high degree of accuracy. This allowed coders to focus on more complex cases, reducing their workload and improving overall coding efficiency.
The system also incorporated a robust quality control mechanism, flagging potential coding errors for human review. The result was a significant reduction in coding turnaround time, a decrease in coding errors, and a measurable improvement in coder job satisfaction due to a shift away from repetitive tasks. The system’s integration with existing Electronic Health Record (EHR) systems was seamless, minimizing disruption to workflows.
Regular training and support for coders were provided to ensure successful adoption and maximize the benefits of the AI tool.
Unsuccessful AI Implementation: Regional Hospital System
A regional hospital system, which we will refer to as “Regional Health,” attempted to implement a similar AI-powered coding solution but encountered significant challenges. Their chosen system lacked the necessary integration capabilities with their legacy EHR system, requiring manual data entry and creating a bottleneck in the workflow. This led to increased frustration among coders, who found the new system cumbersome and less efficient than their previous methods.
Furthermore, the AI system’s accuracy was lower than expected, leading to a higher-than-anticipated error rate. Insufficient training and support for coders exacerbated the problems, resulting in a lack of confidence in the system’s capabilities. The hospital system ultimately abandoned the project after a six-month trial period, incurring significant costs and experiencing a temporary disruption in coding operations.
The failure highlighted the importance of thorough system integration, accurate AI performance, and adequate coder training and support.
Comparative Analysis
The success of Cedars-Sinai’s implementation stands in stark contrast to the failure of Regional Health’s attempt. The key differences lie in the level of system integration, the accuracy of the AI, and the level of support provided to the coding staff. Cedars-Sinai prioritized seamless integration and provided comprehensive training, leading to a smooth transition and increased efficiency. Regional Health’s failure underscores the critical importance of these factors.
Case Study | Outcome | Success Factors | Failure Factors |
---|---|---|---|
Cedars-Sinai Medical Center | Successful Implementation; Improved efficiency, reduced errors, increased coder satisfaction. | Seamless EHR integration, high AI accuracy, comprehensive coder training and support, robust quality control mechanisms. | None identified. |
Regional Hospital System | Unsuccessful Implementation; Project abandoned due to integration issues, low accuracy, inadequate training, and increased workload. | None identified. | Poor EHR integration, low AI accuracy, insufficient coder training and support, lack of robust quality control. |
End of Discussion
The medical coding worker shortage presents a significant challenge to our healthcare system, but the rise of AI-powered solutions offers a beacon of hope. While ethical considerations and practical challenges remain, the potential benefits of increased accuracy, efficiency, and cost savings are undeniable. As AI technology continues to advance, its integration into medical coding workflows will likely become even more seamless, paving the way for a future where technology and human expertise work in harmony to deliver optimal patient care.
The journey toward this future requires careful planning, responsible implementation, and a proactive approach to addressing the ethical and practical considerations that arise.
FAQ
What are the potential downsides of relying solely on AI for medical coding?
Over-reliance on AI could lead to a loss of human expertise and critical thinking skills. The technology may also struggle with complex or ambiguous cases requiring nuanced medical judgment.
How can healthcare providers ensure the data privacy and security of patient information used by AI systems?
Robust data encryption, anonymization techniques, and compliance with relevant data privacy regulations (like HIPAA) are crucial. Choosing reputable AI vendors with strong security protocols is also essential.
What training and support will medical coders need in an AI-integrated environment?
Coders will need training on how to effectively utilize AI tools, interpret AI-generated results, and handle exceptions or situations where AI assistance is insufficient. Ongoing professional development will be key.
What is the cost of implementing an AI-powered medical coding system?
Costs vary depending on the chosen solution, its features, and the size of the healthcare organization. Factors like software licensing, hardware requirements, integration costs, and staff training all contribute to the overall expense.