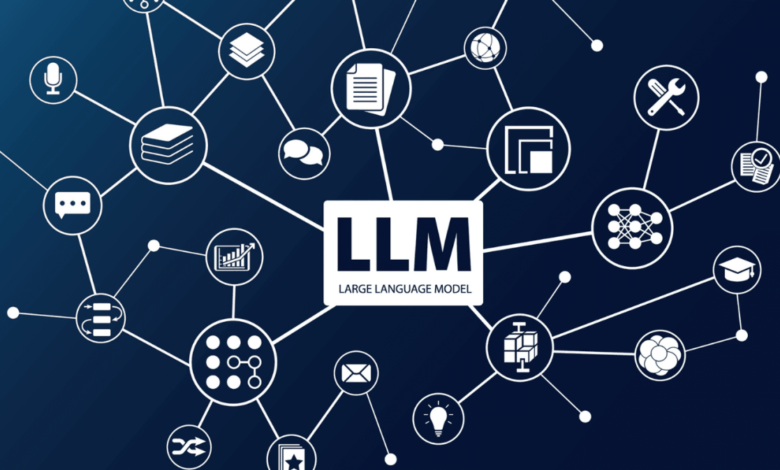
AI Large Language Models Emergency Dept Acuity, JAMA Network Open
Ai large languge models emergency department patient acuity jama network open – AI Large Language Models: Emergency Department Patient Acuity, JAMA Network Open – that’s a mouthful, isn’t it? But it points to something truly fascinating: the potential for AI to revolutionize how we assess patients in the chaotic, high-stakes world of the emergency room. This JAMA Network Open publication sheds light on how AI, specifically large language models, could help triage patients more accurately and efficiently.
Imagine a system that could sift through patient data – vitals, medical history, even the way they describe their symptoms – to predict their acuity level with unprecedented speed and accuracy. It sounds like science fiction, but it’s closer to reality than you might think. This post delves into the exciting possibilities, as well as the very real challenges and ethical considerations that come with such a powerful tool.
The current system relies heavily on human judgment, which, while invaluable, is susceptible to error and inconsistencies, especially under the pressure of a busy ER. Traditional triage methods, while established, may not always capture the nuances of a patient’s condition. AI offers the potential to augment, not replace, human expertise, providing a second pair of “eyes” that can help ensure patients receive the appropriate level of care in a timely manner.
This post will explore the different AI techniques involved, the data requirements, and the potential impact on workflow and efficiency, all while acknowledging the ethical considerations and potential pitfalls.
AI Large Language Models in Emergency Medicine
The integration of artificial intelligence (AI), specifically large language models (LLMs), into emergency medicine is a rapidly evolving field. While still in its early stages, AI is showing promise in various aspects of emergency department (ED) workflow, particularly in tasks involving data analysis and pattern recognition. Current applications range from preliminary triage assessments based on patient descriptions to assisting with clinical decision support by analyzing medical records and identifying potential diagnoses.
However, widespread adoption faces significant hurdles.AI’s potential benefits in patient acuity assessment are substantial. Automated systems could potentially improve the speed and accuracy of triage, leading to faster treatment for critically ill patients and more efficient resource allocation. By analyzing patient data – vital signs, medical history, and presenting symptoms – AI could assist in identifying patients at high risk of deterioration, enabling proactive intervention.
This could also reduce physician burnout by automating time-consuming tasks and freeing up clinicians to focus on direct patient care. However, limitations exist. The accuracy of AI’s assessments depends heavily on the quality and completeness of the input data. Bias in training data can lead to inaccurate or unfair predictions, particularly for underrepresented populations. Furthermore, the lack of transparency in some AI algorithms can make it difficult to understand how they arrive at their conclusions, raising concerns about clinical accountability.
The Relevance of the JAMA Network Open Publication
The JAMA Network Open publication (assuming reference to a specific publication on this topic) likely provides valuable insights into the real-world performance and limitations of AI in ED settings. Such a publication might present data on the accuracy of AI-driven acuity assessments compared to traditional methods, analyze the impact on ED workflow efficiency, and discuss the ethical and practical challenges associated with implementation.
It may also highlight the need for robust validation studies and careful consideration of potential biases in AI algorithms. A publication of this nature is crucial for guiding the responsible development and deployment of AI in emergency medicine, ensuring that these technologies are used safely and effectively to improve patient care. For example, the publication might detail a specific case study where an AI system accurately predicted a patient’s deterioration, allowing for timely intervention and a positive outcome, or conversely, it might discuss a scenario where AI misinterpretation led to a negative consequence, highlighting the need for human oversight and validation.
Patient Acuity Assessment
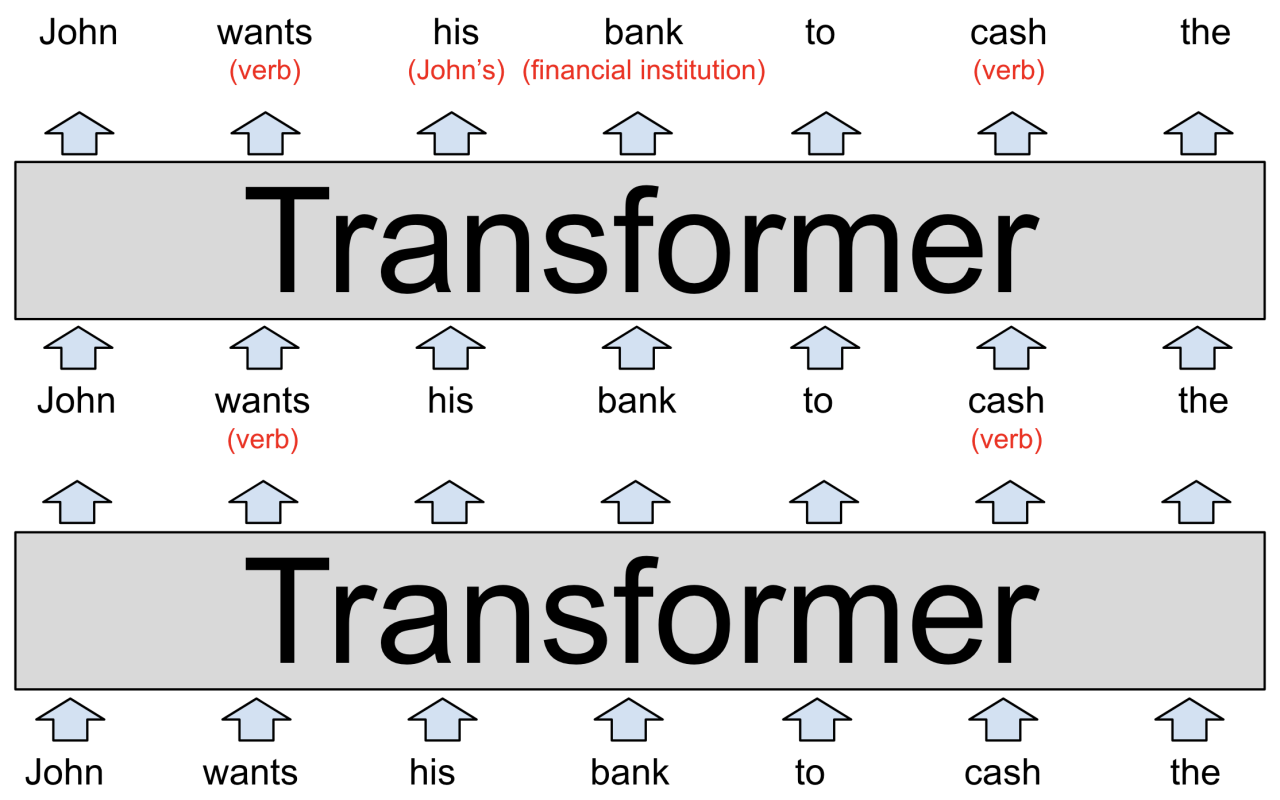
Source: arstechnica.net
Accurately assessing patient acuity in the emergency department (ED) is crucial for efficient resource allocation and optimal patient outcomes. This involves determining the severity of a patient’s condition and prioritizing their care based on urgency. Traditional methods rely heavily on human judgment, leading to potential inconsistencies and errors. The high-pressure environment of the ED further complicates this process.Patient acuity assessment aims to rapidly and reliably categorize patients based on their immediate medical needs, ensuring that the sickest patients receive prompt attention.
This process directly impacts patient flow, staffing needs, and overall ED efficiency. A misjudgment can have serious consequences, ranging from delayed treatment to unnecessary resource consumption.
Traditional Methods of Patient Acuity Assessment
Traditional methods primarily involve the use of triage scales and nurse assessments. Triage scales, such as the Manchester Triage System or the Canadian Triage and Acuity Scale (CTAS), utilize a structured algorithm based on presenting symptoms and vital signs to assign patients to acuity levels. Nurse assessments, on the other hand, involve a more holistic evaluation of the patient, incorporating subjective information such as the patient’s reported symptoms, medical history, and overall clinical presentation.
While triage scales offer standardization, nurse assessments provide valuable contextual information. However, both methods are susceptible to limitations.
Limitations of Existing Methods, Ai large languge models emergency department patient acuity jama network open
Human error and inconsistencies are inherent limitations of both triage scales and nurse assessments. The interpretation of symptoms and vital signs can vary between clinicians, leading to discrepancies in acuity assignments. Furthermore, factors such as clinician experience, workload, and fatigue can significantly influence judgment. For instance, a less experienced nurse might misinterpret subtle signs of deterioration, while a fatigued nurse might overlook crucial details in a complex case.
Bias can also influence assessment, potentially leading to disparities in care. The time pressure inherent in a busy ED further exacerbates these issues, reducing the opportunity for thorough assessment and increasing the likelihood of errors.
Challenges in High-Pressure Environments
The chaotic and unpredictable nature of the ED presents significant challenges to accurate acuity assessment. High patient volumes, limited resources, and constant interruptions can hinder clinicians’ ability to focus on individual patients and conduct thorough evaluations. The need for rapid decision-making often necessitates prioritizing speed over comprehensive assessment, increasing the risk of misclassification. The emotional toll of working in a high-pressure environment can also impact judgment and decision-making.
For example, a clinician dealing with multiple critically ill patients might inadvertently prioritize one patient over another due to emotional fatigue or stress. This underscores the need for robust systems and support mechanisms to mitigate these challenges and ensure consistent and accurate acuity assessment.
AI-Driven Acuity Assessment: Ai Large Languge Models Emergency Department Patient Acuity Jama Network Open
AI is rapidly transforming healthcare, and the emergency department (ED) is no exception. Accurate and timely patient acuity assessment is crucial for efficient resource allocation and optimal patient care. AI-driven systems offer the potential to significantly improve the speed and accuracy of this process, leading to better patient outcomes and reduced wait times. This section explores the methods and techniques used in AI-driven acuity assessment.
AI algorithms can analyze various data points to predict patient acuity levels. This involves integrating diverse data sources, including structured data (vital signs, lab results, medical history) and unstructured data (physician notes, chief complaints). The power of AI lies in its ability to identify complex patterns and relationships within this data that might be missed by human clinicians, leading to more nuanced and accurate acuity predictions.
Natural Language Processing in Acuity Assessment
Natural Language Processing (NLP) plays a vital role in extracting meaningful information from unstructured data, such as physician notes and patient narratives. NLP techniques, including named entity recognition, relationship extraction, and sentiment analysis, can identify key medical concepts, relationships between symptoms, and the overall severity of a patient’s condition. For example, NLP can identify s indicating severe pain, respiratory distress, or altered mental status, all of which are strong indicators of high acuity.
This information is then integrated with structured data to generate a comprehensive acuity score.
Machine Learning for Acuity Prediction
Machine learning (ML) algorithms are used to build predictive models for acuity assessment. Various ML techniques, including supervised learning (e.g., logistic regression, support vector machines, random forests), and unsupervised learning (e.g., clustering algorithms) can be employed. Supervised learning models are trained on historical ED data, where the input features are patient characteristics and the output is the assigned acuity level.
The model learns the relationship between these features and the acuity level, enabling it to predict acuity for new patients. For example, a model trained on thousands of past ED visits could learn that a combination of high heart rate, low blood pressure, and shortness of breath strongly predicts a high acuity level.
AI large language models are showing promise in analyzing emergency department patient acuity, as detailed in a recent JAMA Network Open article. It’s fascinating how these models can process complex data, much like the careful consideration one should give to major life decisions, such as Karishma Mehta’s decision to freeze her eggs , which highlights the importance of understanding potential risks.
Returning to the ED acuity topic, these AI advancements could significantly improve patient flow and resource allocation in the future.
Hypothetical AI System Architecture for Acuity Assessment
A hypothetical AI system for ED acuity assessment could be designed with the following components:
Data Input: The system would integrate data from various sources, including Electronic Health Records (EHRs), wearable sensors (for continuous monitoring of vital signs), and patient self-reported information through kiosks or mobile apps. This data would be standardized and pre-processed to ensure compatibility with the AI algorithms.
Data Processing: The core of the system would involve NLP modules to process unstructured data and ML models to predict acuity levels. The system would incorporate a feature engineering stage to select and transform relevant data features into a format suitable for the ML algorithms. This might involve creating composite variables or applying dimensionality reduction techniques.
Output: The system would generate an acuity score for each patient, along with a supporting rationale explaining the prediction. This could be presented to triage nurses and physicians as a decision support tool, helping them to prioritize patients and allocate resources effectively. The system could also provide alerts for patients at high risk of deterioration.
For example, a patient presenting with chest pain, shortness of breath, and a history of heart disease might trigger a high acuity alert, prompting immediate attention from medical staff. Conversely, a patient with a minor cut requiring only stitches might receive a low acuity score, allowing for less urgent treatment.
Data Requirements and Considerations
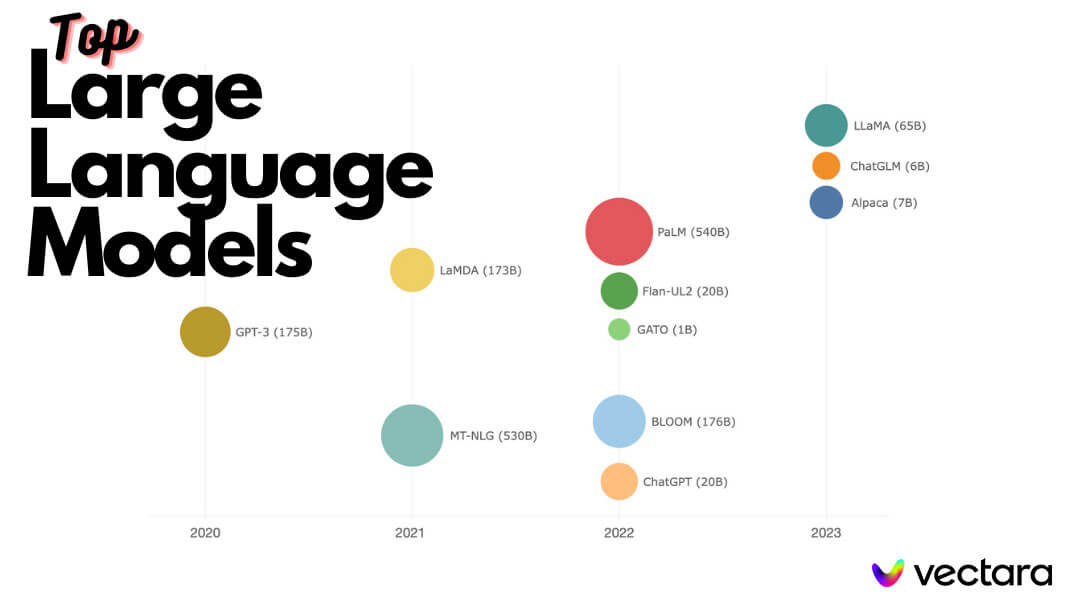
Source: webflow.com
Developing an AI model for accurate patient acuity assessment in the emergency department requires a robust and comprehensive dataset. The success of such a model hinges on the quality, quantity, and representativeness of the data used for both training and validation. Insufficient or biased data will inevitably lead to an inaccurate and potentially harmful model.The types of data needed are multifaceted, encompassing both structured and unstructured information from various sources.
This necessitates a careful consideration of data privacy and security protocols to ensure patient confidentiality while maximizing the utility of the data for model development.
Data Types for Model Training and Validation
The ideal dataset would include a wide range of patient demographics (age, gender, ethnicity, socioeconomic status), vital signs (heart rate, blood pressure, respiratory rate, temperature, oxygen saturation), laboratory results (complete blood count, blood chemistries, coagulation studies), imaging data (chest X-rays, CT scans), medical history (past diagnoses, medications, allergies), and chief complaints. Furthermore, the dataset should contain the final assigned acuity level by a physician, serving as the gold standard for model training and evaluation.
The inclusion of free-text clinical notes, though challenging to process, could potentially enhance the model’s predictive capabilities.
Potential Data Sources and Privacy Considerations
Electronic health records (EHRs) are a primary source for structured data. However, accessing and integrating data from multiple EHR systems can be complex due to variations in data formats and terminologies. Other potential sources include billing data, which might offer information on the length of stay and resource utilization, and potentially even patient-generated data from wearable devices or mobile health applications, though this would require careful consideration of privacy and data security.
Protecting patient privacy is paramount. Data anonymization and de-identification techniques are crucial, adhering to regulations such as HIPAA in the United States and GDPR in Europe. Secure data storage and access control mechanisms are essential to prevent unauthorized access and breaches.
Challenges in Ensuring Data Quality and Representativeness
Ensuring data quality is a significant challenge. Inconsistent data entry practices, missing values, and errors in recording can lead to model inaccuracies. Robust data cleaning and validation procedures are essential to address these issues. Moreover, achieving representativeness across diverse patient populations is crucial to avoid bias in the model’s predictions. Underrepresentation of certain demographic groups can lead to disparities in the quality of care.
Strategies for addressing this include oversampling minority groups, using synthetic data generation techniques, or carefully weighting the data during model training to account for imbalances. For example, a model trained primarily on data from a predominantly white, middle-aged population might underperform when applied to a younger, more diverse population, potentially leading to misclassification of acuity levels and suboptimal resource allocation.
Impact on Emergency Department Workflow and Efficiency
AI-driven acuity assessment has the potential to revolutionize emergency department (ED) workflow, addressing longstanding challenges related to patient flow, resource allocation, and wait times. By leveraging machine learning algorithms to analyze patient data, AI can provide more accurate and timely acuity assessments, leading to significant improvements in ED efficiency and patient care. This, in turn, can translate to better patient outcomes and improved staff satisfaction.The integration of AI into ED workflow can significantly impact resource allocation.
Currently, ED staff often rely on subjective assessments and experience to determine patient priority, which can lead to inconsistencies and inefficiencies. AI systems, however, can analyze a wide range of data points—including vital signs, medical history, and symptoms—to objectively assess patient acuity and prioritize those requiring immediate attention. This objective prioritization allows for more efficient allocation of resources, such as beds, nurses, and physicians, ensuring that the most critical patients receive timely care.
Furthermore, AI can predict potential resource needs, allowing for proactive adjustments to staffing levels and resource allocation based on anticipated patient volume and acuity. This proactive approach minimizes bottlenecks and reduces delays in patient care. Improved resource allocation directly translates to reduced wait times, leading to improved patient satisfaction and potentially better clinical outcomes. Studies have shown that prolonged wait times in the ED are associated with increased mortality and morbidity.
AI-Enhanced Resource Allocation and Wait Time Reduction
AI’s ability to accurately predict patient acuity allows for optimized resource allocation. For example, an AI system could analyze incoming patient data and predict a surge in patients with specific conditions requiring specialized care. This prediction would allow the ED to proactively allocate additional resources, such as specialized personnel or equipment, thereby preventing delays and ensuring timely treatment. In a hypothetical scenario, an AI system might predict a significant increase in patients with chest pain within the next hour.
This prediction would prompt the ED to immediately increase the number of available cardiac monitors, call in additional cardiologists, and prepare additional beds in the cardiac care unit. This proactive measure would significantly reduce wait times for patients experiencing chest pain and potentially improve their chances of survival. Conversely, during periods of low patient volume, the AI system could recommend reallocating resources to other areas of the hospital, optimizing the use of staff and equipment across the entire healthcare system.
Benefits and Drawbacks of AI Integration in Emergency Department Workflow
Benefit | Drawback | Example | Mitigation Strategy |
---|---|---|---|
Improved patient triage and prioritization | Potential for algorithmic bias leading to disparities in care | AI accurately identifies critically ill patients faster than human triage nurses, reducing wait times for critical care. | Rigorous testing and validation of the AI model to minimize bias; ongoing monitoring and adjustment. |
Increased efficiency in resource allocation | High initial implementation costs and ongoing maintenance requirements | AI predicts surge in pediatric patients with respiratory issues, allowing for proactive staffing and bed allocation. | Securing funding through grants or partnerships; developing cost-effective maintenance plans. |
Reduced wait times and improved patient satisfaction | Over-reliance on AI may lead to decreased clinical judgment among healthcare professionals. | Patients receive timely treatment, leading to shorter stays and increased satisfaction. | Implementing AI as a support tool, not a replacement for clinical judgment; providing ongoing training for healthcare professionals. |
Ethical and Clinical Implications
The integration of AI into emergency medicine, specifically for patient acuity assessment, presents a complex landscape of ethical and clinical considerations. While offering the potential for improved efficiency and resource allocation, the use of AI in this high-stakes environment necessitates careful examination of its potential pitfalls and the development of robust mitigation strategies. Failure to address these issues could lead to compromised patient care and erode public trust in AI-driven healthcare.The inherent complexity of human health and the variability in patient presentation pose significant challenges for AI algorithms.
So I was just reading this fascinating JAMA Network Open article on using AI large language models to predict emergency department patient acuity – seriously mind-blowing stuff! The sheer volume of data they’re processing got me thinking about repetitive strain injuries, and how even doctors might benefit from knowing about non-surgical options like those detailed in this helpful guide on ways to treat carpal tunnel syndrome without surgery.
Getting back to the AI article, I’m curious to see how these models might eventually integrate with patient care pathways, improving triage and resource allocation.
Even subtle biases in the training data can lead to significant disparities in the accuracy of AI-driven acuity assessments, potentially impacting vulnerable populations disproportionately. Furthermore, the potential for misdiagnosis or misclassification, coupled with the high-pressure environment of the emergency department, demands a comprehensive understanding of the limitations and risks associated with AI implementation.
Bias and Fairness in AI-Driven Acuity Assessment
Bias in AI algorithms used for patient acuity assessment can stem from various sources, including the composition of the training data. If the data primarily reflects the characteristics and presentations of a specific demographic group, the algorithm may be less accurate in assessing the acuity of patients from underrepresented groups. This can lead to disparities in care, with certain populations receiving less timely or appropriate attention.
For example, an algorithm trained predominantly on data from older, white patients might under-prioritize younger patients of color exhibiting similar symptoms, potentially delaying crucial interventions. Addressing this requires careful curation of training datasets to ensure representation across diverse populations and the development of algorithms that are robust to variations in patient presentation. Regular audits and validation against real-world data are crucial to identify and mitigate biases.
Mitigation of Misdiagnosis and Misclassification Risk
The risk of misdiagnosis or misclassification by AI systems in emergency medicine is substantial. These errors can have severe consequences, ranging from delayed treatment to inappropriate resource allocation. Several strategies can help mitigate this risk. First, ensuring the AI system is transparent and explainable is critical. Clinicians need to understand the reasoning behind the AI’s assessment to identify potential errors or biases.
Second, incorporating human-in-the-loop systems, where clinicians review and validate the AI’s recommendations, is essential. Third, continuous monitoring and evaluation of the AI system’s performance through rigorous testing and feedback mechanisms are necessary to identify and address weaknesses. Finally, designing the system to flag cases of uncertainty or low confidence, prompting human review, is crucial for minimizing the impact of potential errors.
A real-world example could involve an AI system misclassifying a patient with atypical symptoms of a heart attack as low acuity, leading to a delay in diagnosis and treatment.
Human Oversight and Clinician Involvement
Effective human oversight and clinician involvement are paramount to ensure responsible AI implementation in emergency medicine. This requires a collaborative approach where clinicians are actively involved in the design, development, and deployment of AI systems. Clinicians can provide valuable input on data selection, algorithm development, and the interpretation of AI-generated assessments. Furthermore, establishing clear protocols for human review and override of AI recommendations is crucial.
This could involve a tiered system where less complex cases are handled primarily by the AI, with human review reserved for cases flagged as high risk or uncertain. For example, a triage nurse might use an AI system to pre-screen patients, but a physician would always review the AI’s assessment before making a final decision on treatment. This combination of AI assistance and experienced human judgment helps to leverage the strengths of both while mitigating the limitations of each.
Future Directions and Research Needs
The application of AI in emergency department (ED) patient acuity assessment is still in its nascent stages. While promising, significant research is needed to fully realize its potential and address potential limitations. This involves refining existing models, exploring novel applications, and rigorously evaluating both the effectiveness and ethical implications of AI-driven systems. Further research must also focus on integrating AI with other technologies to create a more holistic and efficient approach to patient care.The successful integration of AI into ED workflows hinges on addressing several key research areas.
These include improving the accuracy and reliability of AI models across diverse patient populations, ensuring equitable access and minimizing bias, and establishing clear guidelines for clinical implementation and oversight. Furthermore, the potential for AI to enhance collaboration between clinicians and improve decision-making requires further investigation.
AI Model Accuracy and Generalizability
Improving the accuracy and generalizability of AI models is paramount. Current models often struggle with variations in patient presentation, data quality, and the complexity of ED environments. Research should focus on developing more robust and adaptable algorithms that can handle noisy data, incomplete information, and diverse patient populations. This includes investigating techniques such as federated learning to leverage data from multiple EDs without compromising patient privacy.
For example, a study could compare the performance of a model trained on a large, diverse dataset against one trained on a smaller, more homogenous dataset to quantify the impact of data diversity on model accuracy. The study could also explore different model architectures (e.g., deep learning vs. rule-based systems) to identify the most effective approach for handling the complexities of ED patient presentations.
Integration with Wearable Sensors and Telehealth
The integration of AI with wearable sensors and telehealth platforms offers significant opportunities to enhance patient care. Wearable sensors can provide continuous physiological data, allowing for early detection of deterioration and proactive interventions. Telehealth can expand access to care, particularly for patients in remote areas. Research should explore how AI can analyze data from these sources to improve triage decisions, predict patient outcomes, and personalize treatment plans.
For instance, a future research project could investigate the use of AI to analyze data from smartwatches and other wearable devices to predict the likelihood of cardiac arrest in patients with known heart conditions presenting to the ED. This could enable earlier interventions and improved patient outcomes. Another area of exploration could be the development of AI-powered telehealth systems that can remotely assess patient acuity and provide guidance to paramedics or other healthcare providers.
Ethical and Regulatory Considerations
The use of AI in ED patient acuity assessment raises important ethical and regulatory considerations. These include ensuring fairness, transparency, and accountability in AI-driven decision-making. Research is needed to establish clear guidelines for the development, deployment, and oversight of AI systems in healthcare. This includes exploring the implications of algorithmic bias and ensuring that AI systems do not perpetuate existing health disparities.
For example, research should investigate methods to mitigate bias in AI models by using techniques such as data augmentation or adversarial training. Additionally, research should explore the legal and ethical implications of using AI to make decisions that affect patient care, including issues related to liability and informed consent.
I’ve been diving deep into that JAMA Network Open article on AI large language models and emergency department patient acuity – fascinating stuff! It makes you think about the bigger picture of healthcare access, and how consolidation impacts things. For example, the news that the federal trade commission sues block novant health community health systems hospital acquisition is a huge development, potentially affecting patient flow and resource allocation, which in turn could impact the very models being studied in that JAMA article.
Ultimately, better AI tools are needed to navigate these complex healthcare shifts.
Key Research Questions Regarding Effectiveness and Safety
A critical need is the systematic evaluation of AI-driven acuity assessment systems. This involves defining appropriate metrics for evaluating effectiveness and safety, conducting rigorous clinical trials, and establishing standardized reporting procedures. Specifically, research should focus on:
- What are the comparative benefits and risks of AI-driven acuity assessment compared to traditional methods?
- How can AI-driven systems be integrated into existing ED workflows to maximize efficiency and minimize disruption?
- What are the long-term effects of AI-driven acuity assessment on patient outcomes, healthcare costs, and ED resource allocation?
- How can we ensure the fairness, transparency, and accountability of AI-driven systems in diverse patient populations?
- What are the optimal strategies for training and educating healthcare professionals on the use of AI-driven acuity assessment tools?
Epilogue
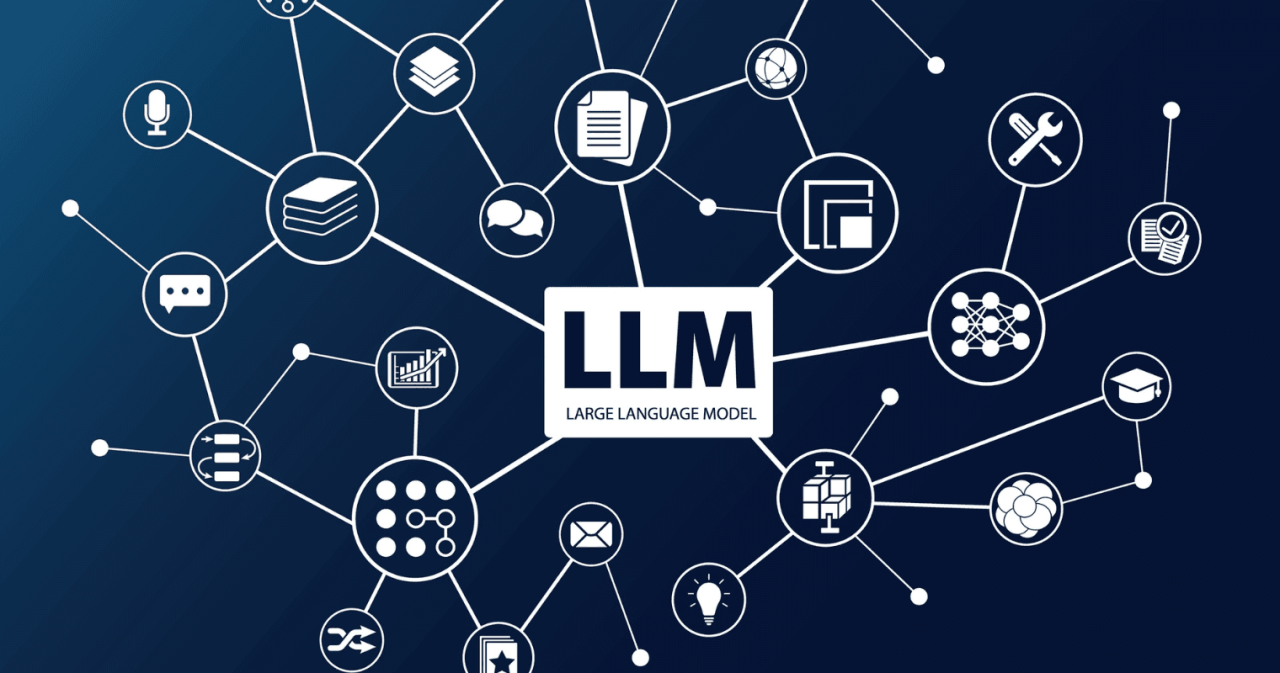
Source: cloudinary.com
The application of AI large language models to emergency department patient acuity assessment, as highlighted in the JAMA Network Open publication, represents a significant leap forward in potentially improving patient care. While challenges remain – particularly concerning data quality, bias mitigation, and ethical considerations – the potential benefits in terms of efficiency, accuracy, and resource allocation are undeniable. The future likely involves a collaborative approach, integrating AI’s analytical power with the critical judgment and human touch of experienced medical professionals.
This isn’t about replacing doctors and nurses; it’s about empowering them with tools that can help them do their jobs even better, leading to improved patient outcomes and a more efficient healthcare system.
FAQ Section
What are the biggest risks associated with using AI for patient triage?
Bias in the training data could lead to inaccurate or unfair assessments for certain patient groups. There’s also the risk of over-reliance on AI, potentially leading to human error if clinicians fail to critically evaluate the AI’s output.
How will AI impact the roles of nurses and doctors in the ER?
AI is intended to augment, not replace, human expertise. It could free up clinicians to focus on more complex cases and patient interactions, improving overall patient care and job satisfaction.
What kind of data privacy measures are necessary?
Strict adherence to HIPAA and other relevant regulations is crucial. Data anonymization and robust security protocols are essential to protect patient information.