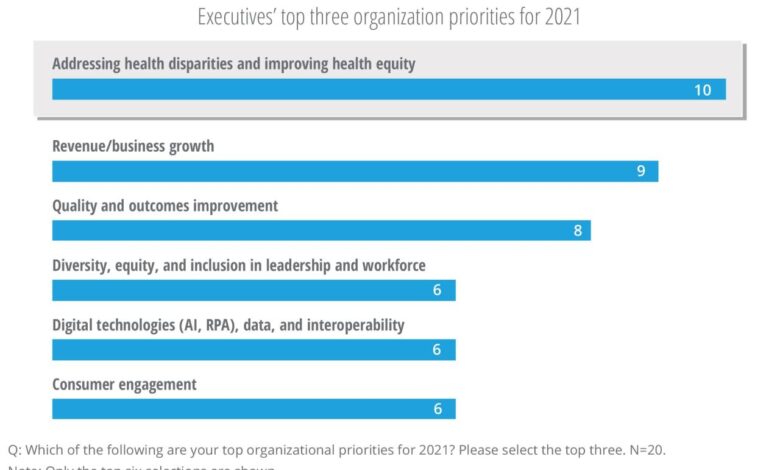
Ready to Drive Health Equity Lets Talk Health Analytics
Ready to drive health equity lets talk about health analytics – Ready to Drive Health Equity: Let’s Talk Health Analytics. We all deserve access to quality healthcare, right? But the reality is, health equity isn’t a given. Disparities in access, outcomes, and even basic preventative care are stark realities for many communities. This isn’t just a healthcare problem; it’s a societal one.
But the good news? Health analytics offer a powerful tool to illuminate these inequalities and drive meaningful change. By analyzing data, we can identify patterns, pinpoint the root causes of disparities, and design targeted interventions to create a healthier future for everyone.
This post dives deep into how health analytics can be the key to unlocking health equity. We’ll explore how data analysis can reveal hidden biases in healthcare systems, how to ethically collect and use sensitive health information, and how to translate complex data into actionable steps for improving community health. We’ll also look at some real-world examples of how health analytics is already making a difference.
Defining Health Equity and its Disparities
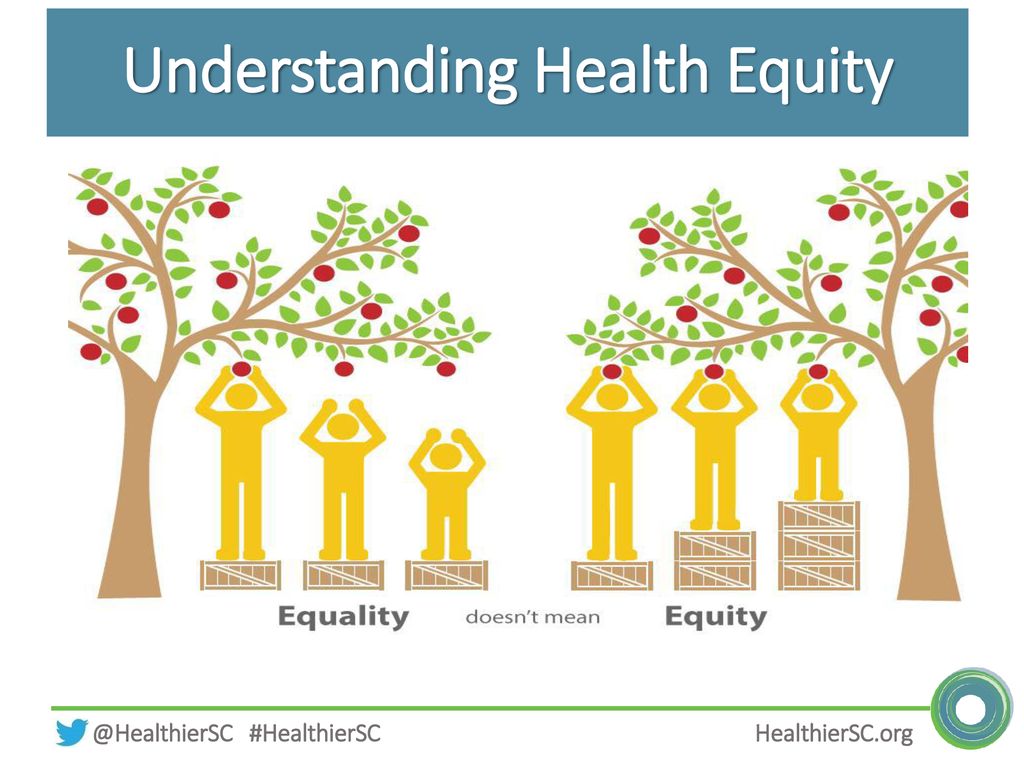
Source: slideplayer.com
Health equity, a fundamental human right, signifies that everyone has a fair and just opportunity to be as healthy as possible. It’s not about treating everyone the same; it’s about addressing the root causes of health disparities that prevent some groups from achieving their full health potential. In modern healthcare, achieving health equity is paramount, as it directly impacts population health outcomes, reduces healthcare costs in the long run, and promotes social justice.
Health Disparities Across Populations
Significant disparities exist in healthcare access and outcomes across various populations, often based on race, ethnicity, socioeconomic status, geographic location, gender, and other social determinants of health. These disparities manifest in unequal access to quality healthcare services, including preventative care, diagnostic testing, and treatment. They also result in differences in health outcomes such as life expectancy, infant mortality rates, and prevalence of chronic diseases.
For example, racial and ethnic minorities frequently experience higher rates of chronic conditions like diabetes and heart disease, and lower life expectancy compared to their white counterparts.
Ready to drive health equity? Let’s talk about health analytics! Improving access to quality care hinges on efficient data analysis, and tools like those mentioned in this article, nuance integrates generative ai scribe epic ehrs , could be game-changers. By streamlining documentation and improving data accessibility, we can better understand and address health disparities, ultimately pushing us closer to achieving true health equity for all.
Social Determinants of Health Contributing to Inequities
Social determinants of health (SDOH) are the conditions in which people are born, grow, live, work, and age. These factors significantly influence health outcomes, often independently of individual behaviors or access to healthcare. Examples include:
- Economic Stability: Poverty, lack of employment opportunities, food insecurity, and housing instability are major contributors to poor health.
- Education Access and Quality: Lower educational attainment is linked to poorer health literacy, limited access to resources, and increased risk of chronic diseases.
- Social and Community Context: Factors like social cohesion, discrimination, crime rates, and access to social support networks all impact health.
- Health Care Access and Quality: This includes access to affordable and quality healthcare services, including preventative care and specialized treatments.
- Neighborhood and Built Environment: Access to healthy food options, safe housing, clean air and water, and recreational facilities are crucial for health.
Comparative Health Outcomes Across Demographic Groups
The following table illustrates disparities in health outcomes for three demographic groups: Non-Hispanic White, Non-Hispanic Black, and Hispanic populations in the United States. Note that these are broad generalizations, and variations exist within each group. Data is drawn from various sources, including the CDC and the National Center for Health Statistics, and represents averages and trends. Precise figures vary depending on the year and specific methodology used.
Demographic Group | Life Expectancy (Years) | Infant Mortality Rate (per 1,000 live births) | Prevalence of Diabetes (%) |
---|---|---|---|
Non-Hispanic White | 79 | 5 | 10 |
Non-Hispanic Black | 75 | 11 | 14 |
Hispanic | 78 | 5 | 13 |
The Role of Health Analytics in Addressing Health Equity
Health analytics offers a powerful toolkit for dismantling health inequities. By systematically analyzing diverse datasets, we can identify disparities, understand their root causes, and design targeted interventions to improve health outcomes for underserved populations. This involves moving beyond simple descriptive statistics to uncover complex relationships and inform policy and program development.
Identifying and Addressing Health Disparities Through Data Analysis
Health analytics allows for the precise identification of health disparities based on various factors such as race, ethnicity, socioeconomic status, geographic location, and access to care. For instance, analyzing claims data might reveal higher rates of diabetes complications in a specific zip code with a predominantly low-income population. This wouldn’t just highlight a disparity; it would pinpoint a geographic area requiring focused intervention.
Further analysis could then explore potential contributing factors like limited access to healthy food options or lack of diabetes management programs in the area. This targeted approach, fueled by data, enables efficient resource allocation and more impactful interventions.
Examples of Data-Driven Improvements in Underserved Communities
Several successful initiatives demonstrate the power of health analytics in improving health equity. One example is the use of predictive modeling to identify individuals at high risk of developing chronic conditions. By analyzing factors like genetics, lifestyle choices, and socioeconomic circumstances, these models can target preventative interventions to those most in need, thus reducing future healthcare costs and improving overall health outcomes.
Ready to drive health equity? Let’s talk health analytics! Understanding disparities requires robust data, and that’s where innovative solutions come in. A fascinating new study, check out this article on study widespread digital twins healthcare , suggests digital twins could revolutionize personalized medicine, offering a powerful tool for identifying and addressing health inequities. Ultimately, leveraging such advancements in analytics is key to achieving true health equity for all.
In some instances, this has led to successful programs providing tailored health coaching or early access to medication, dramatically improving patient adherence and outcomes. Another example is the use of spatial analysis to identify healthcare deserts. By mapping the location of healthcare facilities and the population density of underserved communities, it’s possible to pinpoint areas with limited access to care and advocate for the placement of new clinics or mobile health units.
Data Types for Informing Health Equity Initiatives
Effective health equity initiatives rely on integrating diverse data sources. Clinical data, including diagnoses, treatments, and outcomes, provides a crucial foundation. However, this must be complemented by social determinants of health (SDOH) data. This includes information on factors like income, education, housing, transportation, and access to healthy food. Environmental data, such as air and water quality, also plays a significant role.
Combining these data sources provides a holistic understanding of the complex interplay of factors contributing to health disparities. For example, analyzing clinical data alongside SDOH data might reveal a correlation between low income and higher rates of asthma exacerbations, potentially indicating a need for programs addressing housing conditions or air quality in specific areas.
Hypothetical Health Analytics Project: Improving Preventative Care Access in Rural Communities, Ready to drive health equity lets talk about health analytics
Let’s consider a project focused on improving access to preventative care in a rural community. The project would leverage several data sources:
- Electronic Health Records (EHRs): To identify individuals due for preventative screenings (e.g., mammograms, colonoscopies).
- Census Data: To map population density, income levels, and transportation access within the rural area.
- Geographic Information Systems (GIS): To visualize the location of healthcare facilities and identify healthcare deserts.
- Patient Surveys: To assess transportation barriers and patient preferences for care delivery (e.g., telehealth vs. in-person visits).
The analytical methods would include:
- Predictive Modeling: To identify individuals at high risk of developing preventable conditions based on their EHR data and SDOH factors.
- Spatial Analysis: To map healthcare access and identify areas with the greatest need for intervention.
- Regression Analysis: To determine the relationship between various factors (e.g., distance to healthcare facilities, income, and preventative care utilization).
The expected outcomes include:
- Increased rates of preventative screenings among high-risk individuals in the rural community.
- Improved health outcomes, reduced hospitalizations, and lower healthcare costs.
- Targeted interventions to address transportation barriers and improve access to care.
- Development of evidence-based recommendations for policy changes to improve healthcare access in rural areas.
Data Collection and Privacy Considerations
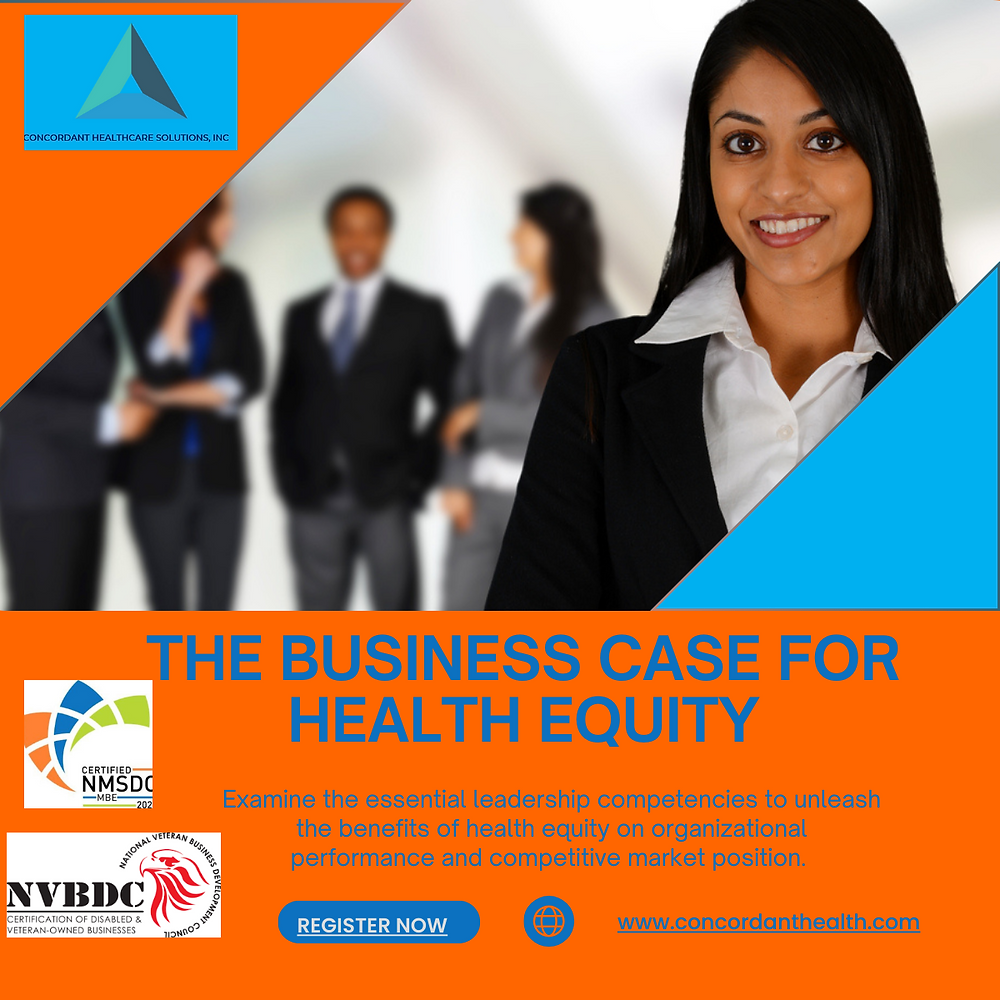
Source: wixstatic.com
Ready to drive health equity? Let’s talk about health analytics! Understanding disparities starts with data, and that includes looking at access to vital services like maternal care. A great example of where this is crucial is highlighted in this article about the challenges faced by rural hospitals regarding labor and delivery services: Rural Hospitals Labor Delivery &.
By analyzing these trends, we can better target resources and improve health equity for all. Ultimately, effective health analytics are key to creating a more just and equitable healthcare system.
The pursuit of health equity through analytics necessitates the careful collection and analysis of health data. However, this process presents significant ethical and privacy challenges. Balancing the need for robust data to identify and address disparities with the fundamental right to privacy is a critical task requiring meticulous planning and implementation. This section explores the key considerations involved in ensuring ethical and responsible data handling.
The sensitive nature of health information demands a high level of vigilance. Data breaches can have devastating consequences for individuals, eroding trust in healthcare systems and hindering progress toward health equity. Furthermore, biases embedded within datasets can perpetuate existing health disparities if not carefully addressed during data collection and analysis. Therefore, robust safeguards are essential to protect individual privacy and ensure the responsible use of health data for equity initiatives.
Data Security and Patient Confidentiality
Protecting patient data requires a multi-layered approach encompassing technical, administrative, and physical safeguards. Technical safeguards include robust encryption protocols to protect data both in transit and at rest, secure access controls to limit access to authorized personnel only, and regular security audits to identify and address vulnerabilities. Administrative safeguards involve developing and implementing comprehensive data governance policies, including clear guidelines on data access, use, and disposal.
Physical safeguards encompass secure storage facilities and controlled access to physical data storage media. For example, a hospital might use a combination of firewalls, intrusion detection systems, and regular penetration testing to enhance data security. They might also implement strict access control policies, limiting access to patient data to only those with a legitimate need to know, based on their role and responsibilities.
Data Anonymization Techniques
Data anonymization aims to remove or modify identifying information from datasets while preserving their utility for analysis. Several techniques exist, each with varying levels of effectiveness and potential risks. De-identification, for instance, involves removing direct identifiers such as names and addresses. However, this is not sufficient to guarantee complete anonymity as quasi-identifiers (e.g., age, gender, zip code) can be combined with other information to re-identify individuals.
Differential privacy adds carefully calibrated noise to the data, making it difficult to infer individual-level information while preserving aggregate statistical properties. k-anonymity ensures that each record in the dataset is indistinguishable from at least k-1 other records based on a set of quasi-identifiers. The choice of anonymization technique depends on the specific data, the intended analysis, and the acceptable level of risk.
For example, a study investigating the prevalence of a specific disease in a particular demographic group might use k-anonymity to protect the identities of individuals while still allowing researchers to draw meaningful conclusions about the disease’s distribution.
Community Engagement and Informed Consent
Meaningful community engagement is crucial for building trust and ensuring that health equity initiatives are relevant and acceptable to the communities they aim to serve. This involves actively involving community members in the design, implementation, and evaluation of data collection processes. Informed consent, a cornerstone of ethical research, requires that individuals are fully informed about the purpose of data collection, how their data will be used, and the potential risks and benefits involved.
This information must be presented in a clear, accessible manner, taking into account the literacy levels and cultural backgrounds of the community. For instance, community meetings and focus groups can be used to solicit feedback on data collection methods and ensure that the process is culturally sensitive and respectful. Obtaining truly informed consent often necessitates using plain language and culturally appropriate materials, and ensuring that participants understand the implications of their participation before they provide consent.
Developing and Implementing Equitable Health Interventions
Successfully addressing health equity requires more than just identifying disparities; it demands the development and implementation of targeted interventions. These interventions must be carefully designed, culturally sensitive, and rigorously monitored to ensure they effectively reach and benefit the populations most in need. This requires a deep understanding of the social determinants of health and a commitment to equitable resource allocation.
Effective interventions are not one-size-fits-all. They must consider the unique needs and contexts of different communities. Successful strategies often involve multi-sectoral collaborations, engaging community members in the design and implementation process, and using data-driven approaches to track progress and adapt strategies as needed.
Examples of Successful Health Interventions Improving Health Equity
Several successful interventions have demonstrably improved health equity. For example, community-based participatory research (CBPR) projects, which actively involve community members in all stages of research, from design to dissemination, have proven effective in addressing health disparities. These projects often lead to interventions tailored to the specific needs and preferences of the communities they serve. Another example is the expansion of telehealth services, which can overcome geographical barriers to access care, particularly beneficial for underserved rural or remote populations.
Finally, targeted public health campaigns addressing specific health issues within minority communities, such as culturally appropriate diabetes prevention programs, have shown promising results in improving health outcomes.
Culturally Competent Healthcare Delivery
Culturally competent healthcare delivery is paramount for achieving health equity. It involves understanding and respecting the cultural beliefs, practices, and values of diverse patient populations. This includes adapting communication styles, providing culturally appropriate health information, and ensuring healthcare settings are welcoming and inclusive. For instance, offering interpreter services, using culturally sensitive educational materials, and having a diverse healthcare workforce are key components of culturally competent care.
Failing to account for cultural differences can lead to mistrust, poor adherence to treatment plans, and ultimately, poorer health outcomes. The ultimate goal is to create a healthcare system that feels safe, welcoming, and effective for everyone, regardless of their background.
Utilizing Health Analytics to Monitor Intervention Effectiveness
Health analytics plays a crucial role in monitoring the effectiveness of health interventions and making necessary adjustments. By tracking key metrics such as participation rates, health outcomes, and cost-effectiveness, we can assess whether interventions are achieving their intended goals and identify areas for improvement. For example, if a diabetes prevention program shows low participation among a specific ethnic group, health analytics can help identify the barriers to participation and inform strategies to address them.
Real-time data analysis allows for timely interventions and adjustments, maximizing the impact of resources and ensuring that interventions remain relevant and effective. This iterative approach, guided by data, is crucial for maximizing the positive impact on health equity.
Actionable Steps for Healthcare Organizations to Improve Health Equity Using Health Analytics
Healthcare organizations can proactively improve health equity by strategically leveraging health analytics. This requires a commitment to data-driven decision-making and a willingness to adapt strategies based on evidence.
- Invest in robust data collection and infrastructure: This includes implementing standardized data collection methods and ensuring data quality and completeness.
- Conduct regular health equity assessments: Identify disparities in access to care, utilization of services, and health outcomes.
- Develop targeted interventions based on data analysis: Tailor interventions to address specific needs of underserved populations.
- Monitor and evaluate intervention effectiveness using health analytics: Track key metrics and make data-driven adjustments.
- Promote data transparency and community engagement: Share data findings with communities and involve them in the design and implementation of interventions.
- Train healthcare professionals on health equity and data analysis: Equip staff with the skills and knowledge to address health disparities effectively.
- Partner with community organizations and stakeholders: Leverage the expertise and resources of community partners to ensure interventions are culturally relevant and effective.
Visualizing Health Equity Data for Effective Communication
Effective communication of health equity data is crucial for driving meaningful change. Complex datasets highlighting disparities need to be translated into easily understandable visuals that resonate with diverse audiences, including policymakers, healthcare providers, community leaders, and the public. Visualizations can effectively bridge the gap between raw data and actionable insights, fostering a shared understanding of the challenges and potential solutions.
Data visualization techniques should be carefully selected to match the type of data and the intended audience. Simple, clear visuals are generally more effective than overly complex ones. The goal is not to overwhelm the viewer with detail, but to highlight key disparities and the impact of interventions in a memorable way.
Examples of Compelling Data Visualizations
Several visualization methods can powerfully illustrate health disparities. For instance, choropleth maps can effectively display geographic variations in health outcomes, such as rates of chronic disease or infant mortality, across different regions or neighborhoods. Color-coding allows for immediate identification of areas with high disparities. Another powerful approach involves using bar charts or column charts to compare health outcomes across different demographic groups (e.g., race, ethnicity, socioeconomic status).
These charts can readily show significant differences in life expectancy, access to care, or prevalence of specific diseases. Line graphs are useful for tracking changes in health outcomes over time, demonstrating the impact of interventions or policy changes.
Utilizing Different Visualization Techniques
The choice of visualization technique depends on the specific data and the message to be conveyed. For instance, a map showing variations in access to healthcare facilities across a state would clearly highlight geographic disparities. A bar chart comparing disease prevalence rates between different racial groups could reveal significant health inequities. Similarly, a line graph tracking the effectiveness of a specific intervention over time would demonstrate its impact on reducing health disparities.
Interactive dashboards, combining various visualization types, allow for exploration of the data from multiple perspectives.
A Visual Representation of Health Equity Data
Consider a combined bar chart and map visualization to illustrate disparities in childhood asthma rates across different neighborhoods in a city. The bar chart would compare asthma rates across neighborhoods, categorized by socioeconomic status (low, medium, high). Each bar would be color-coded according to the neighborhood’s socioeconomic status. Next to the bar chart, a map of the city would display the same data geographically, using a color scale mirroring the bar chart’s color-coding to show the spatial distribution of asthma rates.
This combined visualization provides both a comparative and a geographic perspective, clearly illustrating the correlation between socioeconomic status and childhood asthma rates. The effectiveness stems from its dual representation, allowing for both aggregate comparison and spatial understanding of the issue. This visualization makes it easy to identify high-risk areas and to understand the socioeconomic factors contributing to asthma disparities.
Final Review
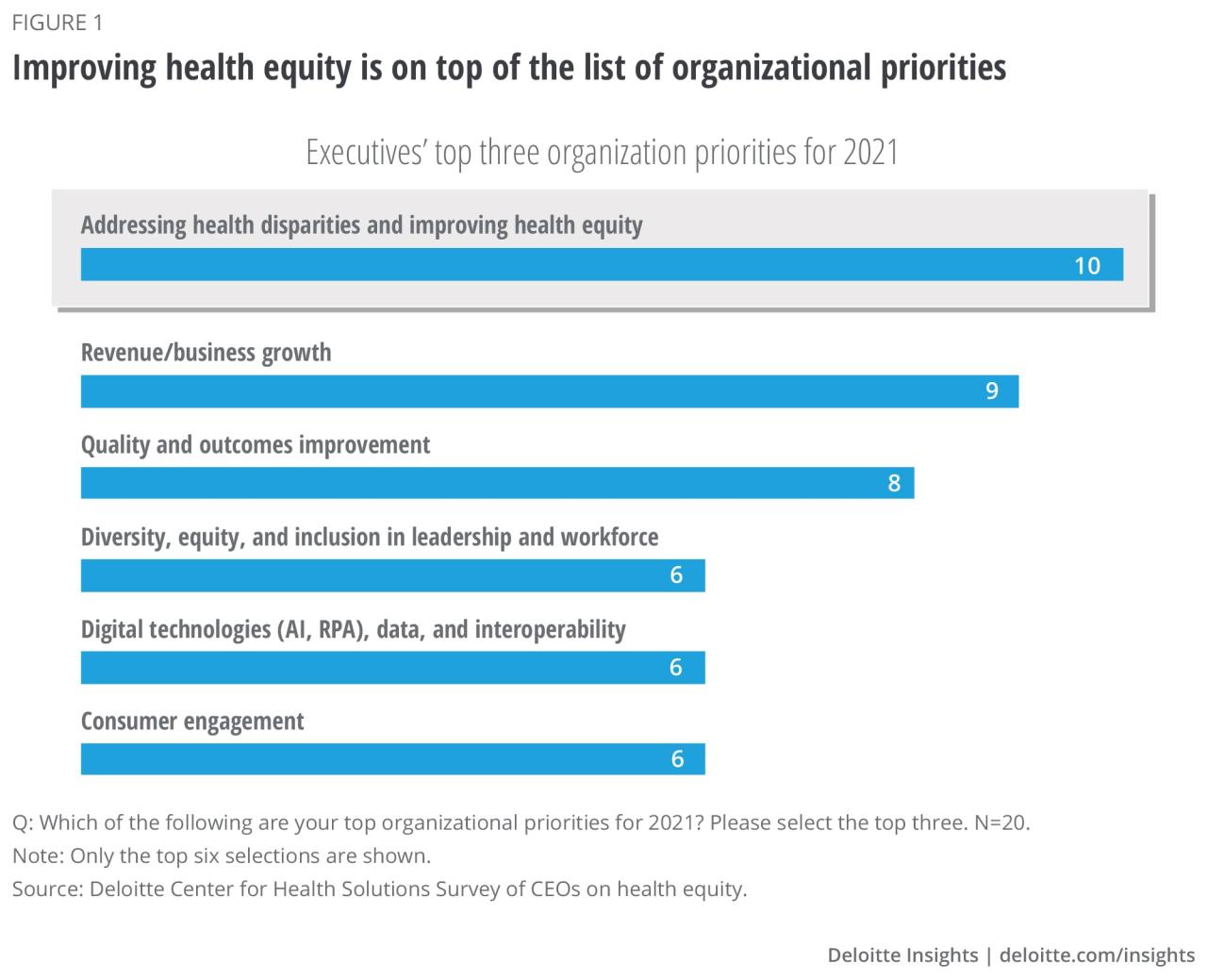
Source: deloitte.com
Ultimately, driving health equity isn’t just about crunching numbers; it’s about building a more just and equitable society. Health analytics provides the roadmap, but it takes a collaborative effort from healthcare providers, policymakers, community leaders, and individuals to pave the way. By understanding the power of data, and working together, we can dismantle systemic barriers and create a future where everyone has the opportunity to live a long and healthy life, regardless of their background or circumstances.
Let’s use health analytics to make a real impact – one data point at a time.
Answers to Common Questions: Ready To Drive Health Equity Lets Talk About Health Analytics
What are some limitations of using health analytics to address health equity?
Data bias, lack of comprehensive data, and the challenge of translating data insights into effective interventions are key limitations. Access to technology and resources to analyze the data also play a significant role.
How can we ensure community trust when collecting health data for equity initiatives?
Transparency, community engagement, and obtaining informed consent are crucial. Working with community leaders and ensuring data privacy are also vital to building trust.
What role do social determinants of health play in health equity?
Social determinants like housing, education, employment, and access to healthy food significantly impact health outcomes. Addressing these factors is crucial for achieving health equity.
What are some examples of successful health equity interventions informed by data analysis?
Targeted interventions based on data analysis have shown success in areas like improving access to preventative care in underserved communities, reducing maternal mortality rates, and addressing chronic disease disparities.