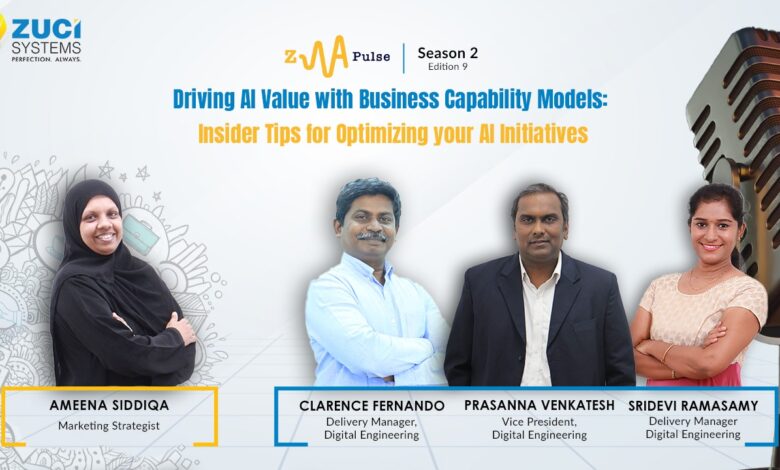
Adaptive AI Driving Value Beyond the Hype
Adaptive ai driving value beyond the hype – Adaptive AI driving: value beyond the hype – that’s the exciting frontier we’re exploring today. Forget the flashy promises; let’s dive into the real-world impact of adaptive AI on autonomous vehicles. We’ll uncover how this technology is revolutionizing safety, efficiency, and the very future of driving, addressing both the incredible potential and the very real challenges along the way.
From understanding the core principles of adaptive AI and its differences from traditional AI, to examining real-world applications and addressing ethical concerns, this post aims to provide a comprehensive look at this rapidly evolving field. We’ll explore how adaptive AI systems, using sophisticated techniques like reinforcement learning and deep learning, are transforming the automotive industry and paving the way for a safer, smarter, and more sustainable transportation future.
Defining Adaptive AI in Driving
Adaptive AI in autonomous driving represents a significant leap forward from traditional AI approaches. While traditional AI relies on pre-programmed rules and fixed decision-making processes, adaptive AI systems learn and improve their performance over time, dynamically adjusting to unpredictable situations and environments. This adaptability is crucial for navigating the complex and ever-changing dynamics of real-world driving.Adaptive AI systems achieve this adaptability through a combination of sophisticated algorithms and continuous learning from data.
Unlike traditional AI, which struggles to handle unforeseen events, adaptive AI can handle unexpected situations, making it much safer and more reliable for autonomous driving applications. This learning process allows the system to refine its decision-making capabilities, leading to improved driving performance and increased safety.
Core Principles of Adaptive AI in Autonomous Driving
Adaptive AI in autonomous driving relies on several core principles. Firstly, it emphasizes continuous learning from real-world data. This means the system constantly updates its models and algorithms based on the experiences it gathers while driving. Secondly, it incorporates robust uncertainty handling mechanisms. Autonomous vehicles must deal with noisy sensor data and unexpected events, and adaptive AI is designed to handle this inherent uncertainty effectively.
Finally, it prioritizes safe and reliable decision-making, even under highly ambiguous conditions. This often involves integrating safety constraints directly into the learning process itself. The system doesn’t just learn to drive; it learns to drive – safely*.
Components of an Adaptive AI Driving System
An adaptive AI driving system comprises several interconnected components working in harmony. Sensor fusion is crucial; it combines data from various sensors, such as cameras, lidar, radar, and ultrasonic sensors, to create a comprehensive and accurate understanding of the vehicle’s surroundings. This integrated perception is then fed into sophisticated decision-making algorithms, often based on reinforcement learning or deep learning, which determine the optimal driving actions (acceleration, braking, steering).
Adaptive AI in driving is more than just a buzzword; it’s about genuinely improving safety and efficiency. Long hours spent testing and developing these systems can lead to repetitive strain injuries, though, like carpal tunnel syndrome. Thankfully, there are effective non-surgical treatments available, such as those outlined on this helpful site: ways to treat carpal tunnel syndrome without surgery.
By addressing these potential downsides, we can ensure the long-term success and positive impact of adaptive AI driving technology.
Finally, control mechanisms translate these decisions into precise commands for the vehicle’s actuators, ensuring the car executes the desired maneuvers smoothly and safely. The interplay between these components is what allows the system to react intelligently and adaptively to dynamic driving situations.
Adaptive AI Approaches in Autonomous Vehicles
Several adaptive AI approaches are employed in autonomous vehicles. Reinforcement learning (RL) is a powerful technique where an AI agent learns to make optimal decisions through trial and error, receiving rewards for good actions and penalties for bad ones. This allows the system to learn complex driving strategies without explicit programming. Deep learning (DL), on the other hand, leverages artificial neural networks with multiple layers to extract intricate patterns and features from large datasets.
DL excels at tasks like object detection and scene understanding, providing the foundation for robust perception capabilities. Often, these approaches are combined; for instance, a deep learning model might provide perception data, which is then used by a reinforcement learning algorithm to learn optimal driving policies. This hybrid approach harnesses the strengths of both methods to achieve superior performance.
For example, Waymo uses a combination of deep learning for perception and reinforcement learning for decision making, continuously refining its algorithms based on real-world driving data gathered from its autonomous vehicle fleet. Tesla’s Autopilot, while less explicitly described as using RL, also relies on vast datasets and deep learning for continuous improvement, though its reliance on solely supervised learning is a significant difference compared to RL-based approaches.
Value Proposition: Adaptive Ai Driving Value Beyond The Hype
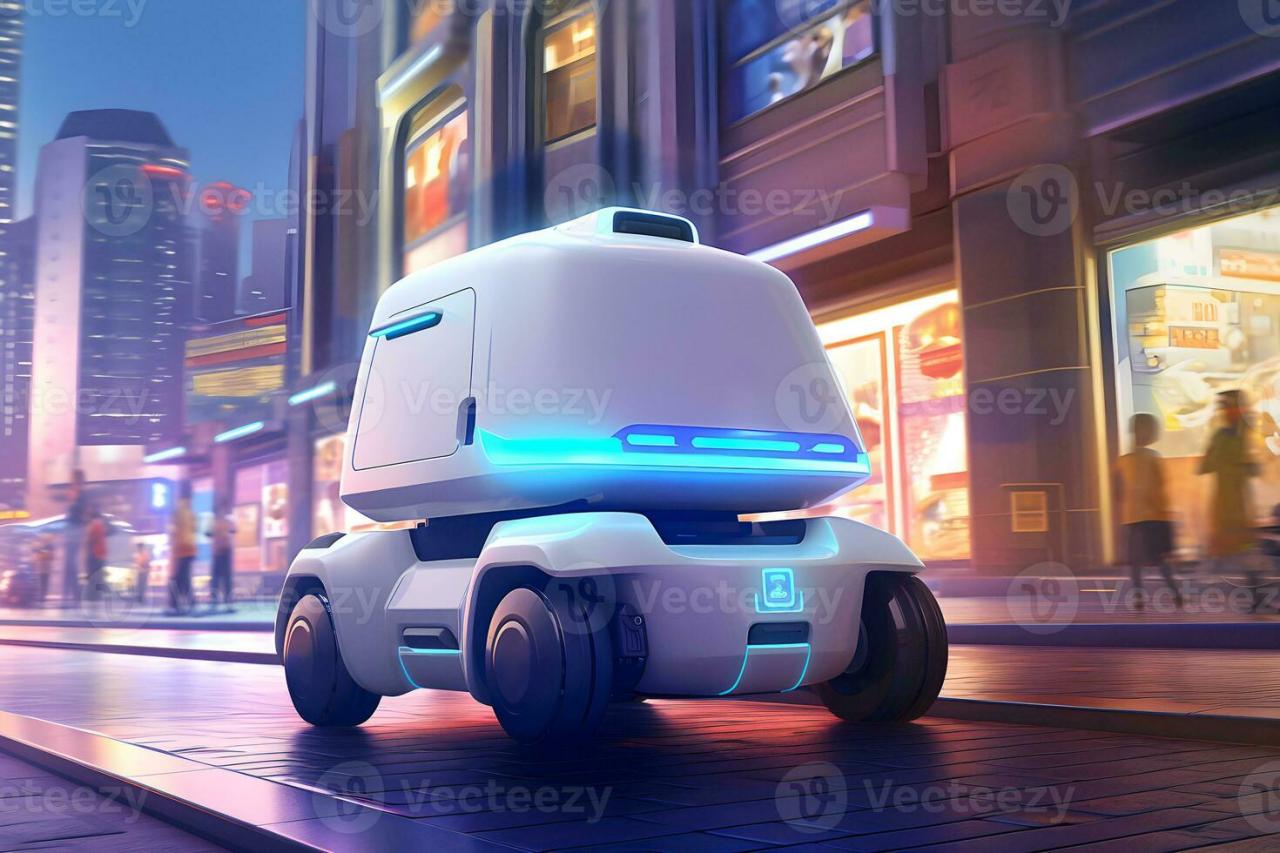
Source: vecteezy.com
Adaptive AI in driving offers far more than just the flashy features often highlighted in marketing campaigns. It represents a fundamental shift in how vehicles operate, leading to tangible improvements in safety, efficiency, and the overall driving experience. Beyond the initial excitement, the real value lies in the demonstrable, measurable benefits it brings to both drivers and the automotive industry as a whole.The core value proposition of adaptive AI hinges on its ability to learn, adapt, and predict.
Unlike traditional driver-assistance systems that rely on pre-programmed rules, adaptive AI systems constantly evolve their understanding of driving conditions and driver behavior. This allows them to respond more effectively to unexpected situations, ultimately enhancing safety and efficiency in a way that static systems simply cannot.
Enhanced Safety Through Predictive Capabilities
Adaptive AI’s predictive capabilities are transforming road safety. By analyzing vast amounts of data – including sensor inputs, GPS data, and even weather forecasts – these systems can anticipate potential hazards long before a human driver might. For example, an adaptive AI system might detect a pedestrian stepping into the road, even if they are initially obscured from the driver’s view, and automatically initiate braking to avoid a collision.
Similarly, these systems can predict potential skidding or loss of control on slippery surfaces, proactively adjusting the vehicle’s stability control to prevent accidents. This proactive approach to safety significantly reduces the likelihood of accidents, saving lives and minimizing injuries.
Improved Fuel Efficiency and Reduced Emissions
Adaptive AI significantly improves fuel efficiency. By analyzing driving style, traffic conditions, and terrain, these systems can optimize acceleration, braking, and speed to minimize fuel consumption. Imagine a system that anticipates upcoming traffic congestion and smoothly adjusts speed to avoid unnecessary braking and acceleration, resulting in a considerable reduction in fuel usage. This translates directly to lower running costs for drivers and contributes to a reduction in overall carbon emissions from the transportation sector.
Real-world studies have shown fuel efficiency improvements of up to 15% in vehicles equipped with advanced adaptive AI systems.
Increased Driver Comfort and Convenience
Beyond safety and efficiency, adaptive AI enhances the driving experience. Features like adaptive cruise control with lane keeping assist not only improve safety but also reduce driver fatigue on long journeys. The system automatically maintains a safe following distance and keeps the vehicle centered in its lane, minimizing driver workload and stress. Furthermore, advanced AI systems can learn individual driver preferences and adjust settings accordingly, providing a personalized and comfortable driving experience.
For instance, the system might learn a driver’s preferred climate control settings or adjust the suspension based on their typical driving style.
Cost Savings and Revenue Opportunities for the Automotive Industry
The automotive industry stands to benefit significantly from adaptive AI. Reduced accident rates translate to lower insurance premiums and fewer warranty claims. Improved fuel efficiency leads to lower manufacturing costs and increased consumer appeal. Moreover, the ability of adaptive AI systems to collect and analyze vast amounts of data creates new revenue opportunities. This data can be used to develop improved vehicle designs, enhance predictive maintenance programs, and even create new personalized services for drivers.
For example, data on driver behavior can be used to offer customized insurance plans or targeted advertising. The potential for revenue generation in this space is vast and still largely untapped.
Addressing Challenges and Limitations
Adaptive AI in driving, while promising a safer and more efficient future, faces significant hurdles in its development and deployment. These challenges span technical complexities, ethical dilemmas, and the inherent risks associated with entrusting critical safety functions to an algorithm. Overcoming these obstacles requires a multi-faceted approach encompassing rigorous testing, robust safety protocols, and a careful consideration of societal implications.The successful implementation of adaptive AI driving systems hinges on addressing a complex interplay of factors.
Simply put, building truly reliable and safe autonomous vehicles is a monumental task.
Technical Challenges in Adaptive AI Driving Systems
Developing robust adaptive AI driving systems presents numerous technical challenges. One major hurdle is the sheer volume and complexity of data required for training. Algorithms need to be trained on massive datasets encompassing diverse driving scenarios, weather conditions, and road types to ensure reliable performance. Furthermore, real-world driving conditions are unpredictable and often involve unexpected events, requiring the AI to handle situations not explicitly covered in its training data.
This necessitates the development of sophisticated algorithms capable of generalizing from limited data and adapting to novel situations in real-time. Another key challenge lies in ensuring the safety and reliability of the system’s decision-making process. The consequences of errors can be catastrophic, demanding extremely high levels of accuracy and fault tolerance. Finally, integrating various sensor technologies, such as cameras, lidar, and radar, into a cohesive and reliable system requires careful calibration and synchronization.
Ethical Concerns Related to Adaptive AI in Autonomous Vehicles
The deployment of adaptive AI in autonomous vehicles raises several significant ethical concerns. One key issue is the potential for algorithmic bias. If the training data reflects existing societal biases, the resulting AI system may perpetuate and even amplify these biases in its decision-making. For example, an AI trained primarily on data from urban areas might perform poorly in rural environments, potentially leading to disproportionate safety risks in underserved communities.
Data privacy is another major concern. Autonomous vehicles collect vast amounts of data about their surroundings, including potentially sensitive information about individuals and their movements. Ensuring the responsible collection, storage, and use of this data is crucial to protecting individual privacy and preventing misuse. The allocation of responsibility in the event of accidents involving autonomous vehicles also poses a significant ethical challenge.
Adaptive AI is proving its worth beyond the initial hype, showing real-world impact in various sectors. It’s fascinating to see how these advancements are applied even in unexpected areas, like the healthcare industry; for instance, the recent news about despite Walmart Health’s closure, the company healthcare destination Scott Bowman highlights the ongoing evolution of healthcare delivery models.
This constant adaptation mirrors the dynamic nature of adaptive AI itself, driving innovation and efficiency across multiple fields.
Determining liability when an AI system makes an error can be complex and requires careful consideration of legal and regulatory frameworks.
Strategies for Mitigating Risks and Limitations
Mitigating the risks and limitations of adaptive AI in driving requires a comprehensive strategy focusing on safety and reliability. Rigorous testing and validation are crucial to identify and address potential vulnerabilities in the system. This involves simulating a wide range of driving scenarios, including edge cases and unexpected events, to assess the AI’s performance under diverse conditions. Redundancy and fail-safe mechanisms are also essential to ensure the system’s continued operation even in the event of component failures.
Implementing robust cybersecurity measures is crucial to prevent malicious attacks that could compromise the system’s integrity and safety. Finally, continuous monitoring and updates are necessary to address emerging issues and improve the system’s performance over time. This requires a robust feedback loop that allows for the collection and analysis of real-world data to identify areas for improvement.
Furthermore, transparent and explainable AI is crucial for building public trust and ensuring accountability. Understanding how an AI system arrives at its decisions is essential for identifying potential biases and improving its reliability.
Future Trends and Developments
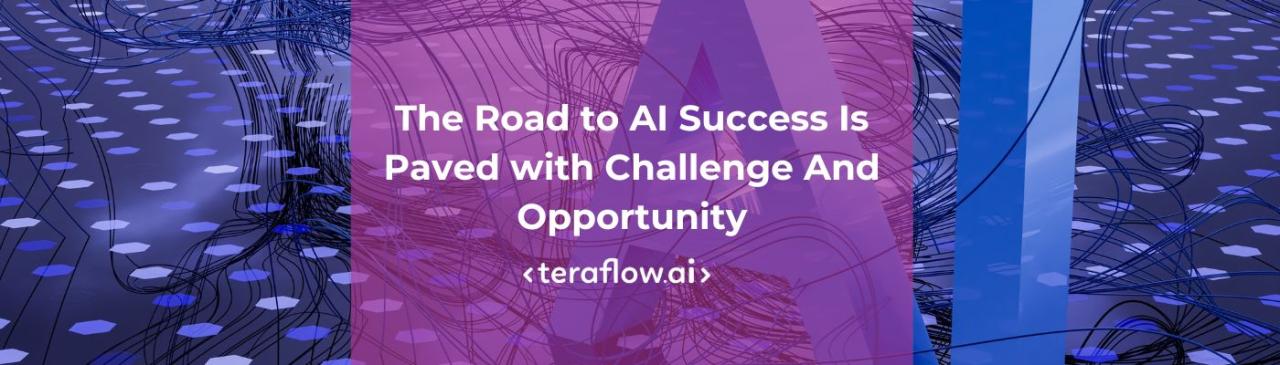
Source: teraflow.ai
The field of adaptive AI in autonomous driving is poised for explosive growth over the next decade. We’re moving beyond the initial stages of self-driving technology, transitioning from systems primarily reliant on pre-programmed rules to truly intelligent systems capable of adapting to unforeseen circumstances and learning from experience. This shift will be driven by advancements in several key areas, leading to safer, more efficient, and more widely accessible autonomous vehicles.The following sections detail anticipated advancements and the timeline for their integration into autonomous driving systems.
These projections are based on current research trends and industry projections, acknowledging the inherent uncertainties in predicting technological progress.
Adaptive AI Evolution Timeline (2024-2034)
The next 5-10 years will witness a significant evolution in adaptive AI for autonomous driving. We can anticipate a phased approach, with incremental improvements leading to increasingly sophisticated systems.
2024-2026: Enhanced perception and sensor fusion. Improved object recognition in challenging weather conditions. Wider deployment of Level 3 autonomous systems in controlled environments (e.g., highways).
2027-2029: Widespread adoption of Level 4 autonomy in specific geographic areas with detailed mapping. Increased reliance on machine learning for decision-making, resulting in more robust and adaptable driving behavior. Initial deployment of systems capable of handling complex urban environments.
2030-2034: Significant advancements in AI explainability and safety verification. Emergence of Level 5 autonomy (fully driverless) in select regions. Increased integration of AI with vehicle infrastructure (V2X communication). Greater focus on personalized driving experiences tailored to individual preferences and driving styles.
Key Technological Advancements in Adaptive AI for Autonomous Driving
Advancement | Expected Impact | Timeline | Potential Challenges |
---|---|---|---|
Improved Sensor Fusion (LiDAR, Radar, Cameras) | More robust and accurate perception in diverse environments, improving safety and reliability. | 2024-2027 | Data processing demands, sensor calibration complexities, cost reduction. |
Advanced Deep Learning Algorithms | Enhanced object recognition, prediction of vehicle and pedestrian behavior, improved decision-making in complex scenarios. | 2025-2030 | Computational requirements, data bias, explainability and trustworthiness of AI decisions. |
Edge Computing and On-board Processing | Reduced reliance on cloud connectivity, enabling faster response times and enhanced privacy. | 2026-2030 | Power consumption, thermal management, development of efficient on-board AI hardware. |
5G and V2X Communication | Enhanced situational awareness through real-time communication with other vehicles and infrastructure, enabling safer and more efficient traffic flow. | 2028-2034 | Network coverage, cybersecurity vulnerabilities, standardization challenges. |
Explainable AI (XAI) | Increased transparency and trust in AI decision-making, facilitating regulatory approval and public acceptance. | 2030-2034 | Development of robust XAI methods, balancing explainability with performance. |
The Impact of Edge Computing and 5G Connectivity
The convergence of edge computing and 5G connectivity will be transformative for adaptive AI in driving. Edge computing allows for processing data closer to the source (the vehicle), reducing latency and dependence on cloud connectivity. This is crucial for real-time decision-making in autonomous driving, especially in situations requiring immediate responses. 5G, with its high bandwidth and low latency, enables seamless communication between vehicles and infrastructure (V2X).
This facilitates cooperative driving, where vehicles can share information about traffic conditions, hazards, and available routes, leading to improved safety and efficiency. For example, a vehicle approaching an intersection could receive real-time information from other vehicles and traffic lights, allowing it to make more informed decisions about speed and trajectory, thus preventing accidents and optimizing traffic flow. This symbiotic relationship between edge computing and 5G will significantly accelerate the development and deployment of safe and reliable autonomous driving systems.
Case Studies
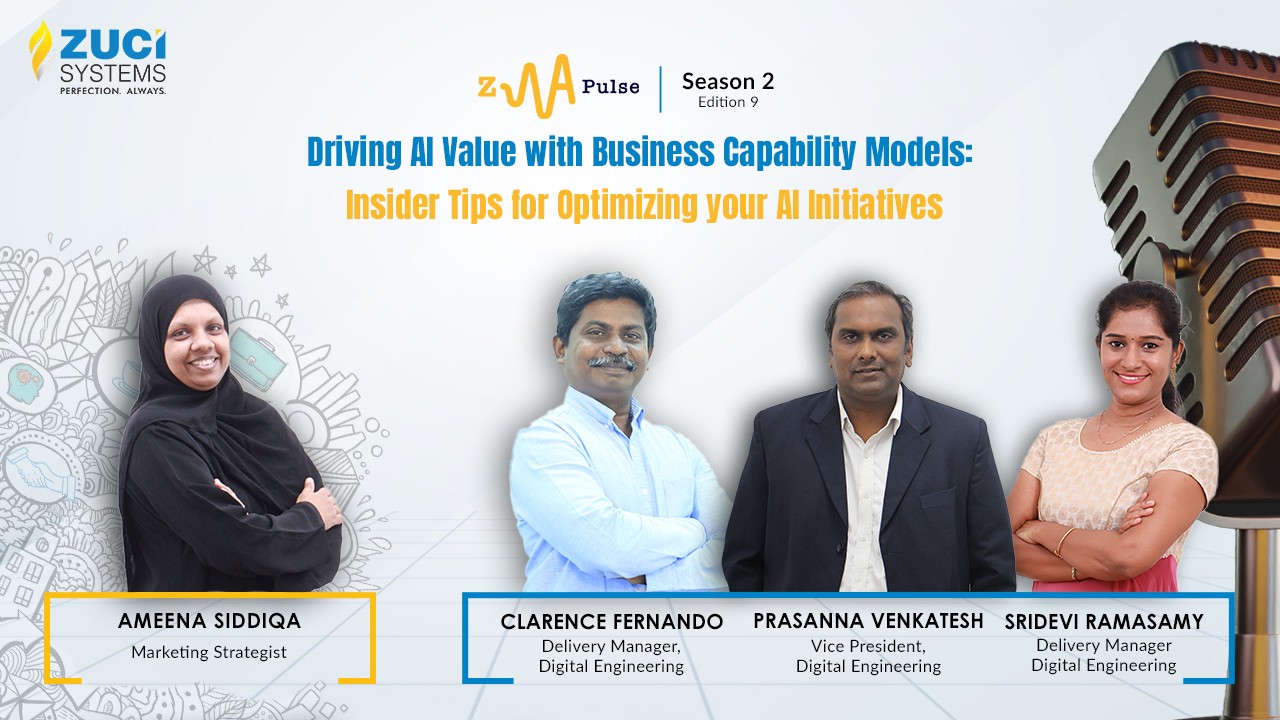
Source: zucisystems.com
Adaptive AI is rapidly transforming the autonomous driving landscape, but its true potential is best understood through real-world applications. The following case studies highlight successful implementations, showcasing the power and challenges of this technology. These examples illustrate not only the achieved results but also the crucial lessons learned during development and deployment.
Tesla’s Autopilot System, Adaptive ai driving value beyond the hype
Tesla’s Autopilot system, while not fully autonomous, represents a significant advancement in adaptive AI for driving. It leverages a combination of computer vision, deep learning, and sensor fusion to achieve features like adaptive cruise control, lane keeping assist, and automatic lane changes. The system uses convolutional neural networks (CNNs) for image recognition, processing data from cameras and radar to identify objects like cars, pedestrians, and traffic signs.
Recurrent neural networks (RNNs) are employed to predict the future trajectory of these objects. Results show improved driver safety and convenience, with data suggesting a reduction in accident rates compared to traditional driver-assistance systems. However, limitations remain, including challenges in handling complex scenarios and the need for continuous software updates to improve accuracy and reliability. A key lesson learned is the importance of robust data collection and annotation for training the AI models, as well as the need for careful consideration of ethical implications and safety protocols.
Waymo’s Self-Driving Vehicles
Waymo, a subsidiary of Alphabet Inc., has been at the forefront of developing fully autonomous vehicles. Their system uses a sophisticated sensor suite including lidar, radar, and cameras, combined with deep learning algorithms to navigate complex urban environments. Their approach relies heavily on reinforcement learning, where AI agents learn optimal driving strategies through simulated and real-world experiences. This approach allows the system to adapt to various traffic conditions and unexpected events.
Waymo’s success lies in its extensive testing and data collection efforts, coupled with a focus on safety and redundancy in their system design. While achieving impressive results in controlled environments, challenges remain in handling unpredictable human behavior and edge cases in diverse geographical locations. A valuable lesson learned is the necessity of rigorous testing and validation in a wide range of scenarios before deploying fully autonomous systems to the public.
Mobileye’s SuperVision System
Mobileye’s SuperVision system offers a driver-assistance system with advanced capabilities, falling somewhere between Tesla’s Autopilot and Waymo’s fully autonomous approach. It employs a combination of cameras and AI algorithms, focusing on accurate object detection and prediction. The system utilizes deep learning models trained on massive datasets to identify and classify objects, enabling features like lane centering, adaptive cruise control, and automated emergency braking.
Adaptive AI in driving is finally delivering on its promises, exceeding the initial hype. The implications of rapid technological advancement are huge, impacting everything from regulations to societal norms, much like the seismic shift caused by the Supreme Court’s decision; check out this article on how the scotus overturns chevron doctrine healthcare and its potential ripple effects.
Ultimately, both these developments highlight the need for adaptable systems and proactive responses to navigate unforeseen consequences. The value of adaptable AI, therefore, isn’t just in the technology itself, but in its ability to respond to such unexpected changes.
Results demonstrate a significant improvement in driver safety and comfort, particularly in highway driving. A key lesson learned from Mobileye’s experience is the importance of designing systems that are both robust and explainable. This means not only achieving high accuracy but also providing drivers with clear and understandable information about the system’s actions. Furthermore, focusing on a more gradual approach to autonomy, rather than aiming for fully autonomous capabilities immediately, allows for a more controlled rollout and minimizes potential risks.
Hypothetical Scenario: Navigating a Crowded Intersection
Imagine a busy city intersection with pedestrians crossing, cyclists weaving through traffic, and vehicles approaching from multiple directions. An adaptive AI system, using sensor fusion from cameras, lidar, and radar, would first identify all objects within its perception range, estimating their speed and trajectory. It would then predict potential conflicts, such as a pedestrian stepping into the vehicle’s path or a cyclist suddenly changing lanes.
Based on these predictions, the adaptive AI system would adjust the vehicle’s speed and trajectory to avoid collisions, using smooth and predictable maneuvers. For instance, it might slow down, stop completely, or gently steer around obstacles, ensuring safe and efficient navigation through the complex intersection. The system’s adaptive nature allows it to respond dynamically to changing conditions, preventing accidents and maintaining a smooth driving experience.
Adaptive AI System Processing Sensor Data
Imagine a visual representation: a central processing unit (CPU) receives data streams from multiple sensors—cameras showing a bird’s-eye view of the intersection, lidar providing distance and depth information, and radar detecting moving objects. This data is fed into a deep learning model, visualized as a complex network of interconnected nodes. The model processes this data in parallel, identifying objects, predicting their trajectories, and evaluating potential risks.
This processing is represented as a flow chart, showing the data moving through different layers of the network. The output of the model—driving decisions such as speed, steering angle, and braking—is then sent to the vehicle’s control system, visualized as signals flowing to the vehicle’s actuators. The entire process happens in real-time, allowing the system to react instantly to changes in the environment.
Feedback loops, visualized as arrows looping back into the system, continuously refine the model’s predictions and improve its performance over time.
Closing Notes
The journey into the world of adaptive AI in driving has revealed a technology brimming with potential, yet not without its hurdles. While the hype surrounding self-driving cars is undeniable, the true value lies in the tangible improvements in safety, efficiency, and cost-effectiveness that adaptive AI delivers. As we move forward, addressing ethical considerations and overcoming technical challenges will be paramount to realizing the full transformative power of this groundbreaking technology.
The future of driving is adaptive, and it’s more exciting than ever before.
Common Queries
What are the biggest safety concerns with adaptive AI driving systems?
The biggest safety concerns revolve around unexpected situations, algorithm biases leading to incorrect decisions, and the potential for system failures due to sensor malfunctions or cyberattacks. Robust testing and fail-safes are crucial.
How does adaptive AI differ from traditional AI in autonomous driving?
Traditional AI often relies on pre-programmed rules, struggling with unpredictable situations. Adaptive AI, however, learns and adapts to new scenarios in real-time through machine learning, making it more robust and flexible.
What is the role of sensor fusion in adaptive AI driving?
Sensor fusion combines data from multiple sensors (cameras, lidar, radar) to create a more complete and accurate picture of the driving environment, improving decision-making in complex situations.
What is the timeline for widespread adoption of adaptive AI in autonomous vehicles?
Widespread adoption is still some years away, with incremental improvements and phased rollouts expected over the next decade. Full autonomy faces significant regulatory and technological hurdles.