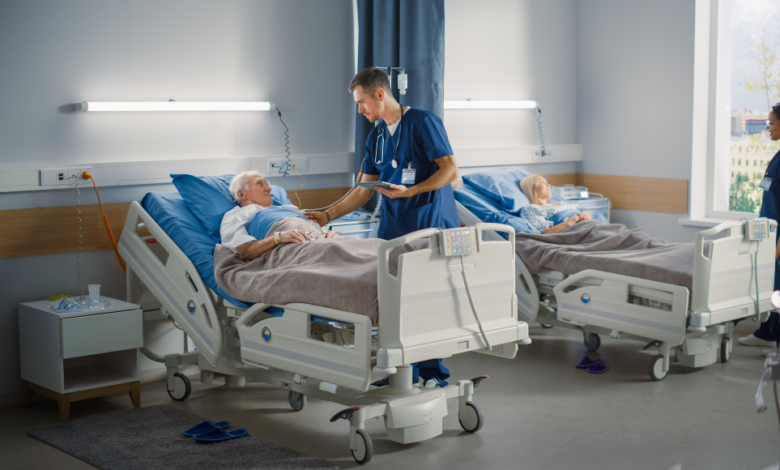
Patients Accept Healthcare AI But Dont Prefer It
Patients accept healthcare ai but dont prefer it – Patients Accept Healthcare AI But Don’t Prefer It – that’s the surprising truth we’re diving into today. While many patients readily accept the use of AI in their healthcare, a closer look reveals a significant gap between acceptance and genuine preference. This isn’t simply about tech-savvy versus tech-averse; it’s a nuanced story involving trust, convenience, and the irreplaceable human element in healthcare.
We’ll explore the reasons behind this disconnect, examining the factors that influence patient acceptance and preference, and ultimately, how we can bridge this gap.
From the convenience of AI-powered appointment scheduling to the potential for more accurate diagnoses through AI-driven image analysis, the benefits are undeniable. Yet, many patients express reservations, highlighting concerns about data privacy, the depersonalization of care, and a lack of transparency in how these systems operate. This post will unpack these concerns, examining different age groups and demographics to understand the varying perspectives on AI’s role in healthcare.
We’ll also look at specific examples of AI applications that patients tolerate but don’t actively seek out, illustrating the complexity of this issue.
Patient Acceptance of AI in Healthcare
Many patients find themselves in a curious position regarding AI in healthcare: they accept its use, even if they don’t actively prefer it. This acceptance, often passive, stems from a complex interplay of factors, not solely driven by a desire for technological advancement. Understanding this nuanced acceptance is crucial for developers and healthcare providers aiming to integrate AI effectively.
Reasons for Patient Acceptance Despite Lack of Preference, Patients accept healthcare ai but dont prefer it
Patients may tolerate AI applications due to a combination of perceived benefits and lack of viable alternatives. For instance, the convenience of automated appointment scheduling or online symptom checkers might outweigh any reservations about interacting with a machine. Furthermore, the perceived authority of a system processing vast amounts of medical data can lead to a passive acceptance, even if the patient doesn’t fully understand or trust the underlying algorithms.
In essence, acceptance in this context is often a matter of pragmatic resignation rather than enthusiastic endorsement.
Factors Influencing Patient Acceptance: Convenience versus Trust
Convenience plays a significant role. Features like telehealth platforms, automated prescription refills, and AI-powered diagnostic tools offer undeniable ease and efficiency. However, trust is a separate and equally crucial factor. Trust involves belief in the accuracy, safety, and ethical considerations of the AI system. While convenience might lead to initial adoption, sustained acceptance depends heavily on establishing and maintaining trust.
This requires transparency in the AI’s decision-making processes, clear communication about limitations, and robust data security measures. A lack of trust can quickly undermine the benefits of convenience, leading to rejection or apprehension.
Patient Acceptance Across Age Groups and Demographics
Acceptance levels of AI in healthcare vary across demographics. Generally, younger generations, more familiar with technology, exhibit higher levels of acceptance, even if preference remains moderate. Older generations, often less comfortable with technology, might demonstrate lower levels of acceptance, sometimes due to concerns about data privacy and potential errors. Socioeconomic factors also play a role; individuals with limited digital literacy might find AI tools more intimidating, regardless of their potential benefits.
Cultural factors can also influence perceptions, with certain communities exhibiting greater skepticism towards technology-driven healthcare solutions.
Examples of Tolerated but Not Actively Sought AI Applications
Many AI applications fall into this category. For example, patients might passively accept an AI-powered triage system that routes them to the appropriate medical professional, even if they would prefer a human interaction. Similarly, the use of AI in administrative tasks, such as appointment reminders or billing processes, is often tolerated without active preference. The use of AI in medical imaging analysis, while potentially improving diagnostic accuracy, might not be something patients actively seek out; they are more likely to accept the results if presented by a physician.
Hypothetical Scenario: Acceptance Without Preference
Imagine Sarah, a 60-year-old patient with diabetes. She receives automated reminders for her medication refills via a text message from her clinic’s AI system. While she finds this convenient, she doesn’t actively seek out this technology; she would be equally happy with a phone call from a nurse. However, the convenience of the text messages prevents her from missing her refills, leading to better health management.
It’s fascinating how patients often accept AI in healthcare, acknowledging its potential, but rarely actively prefer it. This hesitancy might be amplified by factors like the recent closures announced by HSHS Prevea, as reported in this article: hshs prevea close wisconsin hospitals health centers. Such closures could increase anxieties about healthcare access, making people even more wary of relying on potentially impersonal AI solutions.
Ultimately, trust and a human touch seem crucial in winning over patient preference.
This scenario exemplifies acceptance driven by practicality rather than preference. Sarah accepts the AI system’s functionality because it works effectively and improves her health outcomes, but she wouldn’t actively choose it over other methods if given a choice.
Understanding Patient Preferences Regarding AI
Patient acceptance of AI in healthcare is a complex issue, extending beyond simple adoption rates. A deeper understanding of patient preferences, including their concerns, experiences, and desires, is crucial for successful AI integration. This requires acknowledging the human element within the healthcare equation and addressing the anxieties that arise when technology interacts with personal health and well-being.
Top Patient Concerns Regarding AI in Healthcare
Three primary concerns consistently emerge regarding AI’s role in healthcare. First, data privacy and security remain paramount. Patients worry about the potential misuse or unauthorized access to their sensitive medical information. Second, algorithmic bias is a significant concern, with fears that AI systems might perpetuate existing health disparities based on factors like race, gender, or socioeconomic status. Third, the lack of transparency in how AI systems arrive at their diagnoses or treatment recommendations breeds distrust and reduces patient confidence.
Negative Patient Experiences with AI-Driven Healthcare Systems
While AI offers potential benefits, negative experiences can significantly impact patient preferences. Imagine a scenario where an AI-powered chatbot fails to accurately assess a patient’s symptoms, leading to delayed or inappropriate treatment. Or consider a situation where an AI system incorrectly flags a patient’s medical record, resulting in unnecessary anxiety and further investigation. These instances, however infrequent, can severely erode trust in AI-driven healthcare.
Another example could involve a patient feeling ignored or rushed due to the system prioritizing efficiency over personalized care, which leads to dissatisfaction.
Perceived Loss of the Human Touch in AI-Integrated Healthcare
Many patients value the personal connection and empathy provided by human healthcare professionals. The integration of AI, particularly in areas like initial assessments or appointment scheduling, can be perceived as depersonalizing the experience. The absence of nonverbal cues, empathetic responses, and the ability to build rapport can lead to feelings of isolation and dissatisfaction. For example, a patient needing emotional support might find an AI-driven system inadequate in providing the necessary comfort and reassurance.
This loss of human connection can significantly impact a patient’s overall healthcare experience and willingness to embrace AI technologies.
Physician Communication and Patient Preference for AI
Physicians play a crucial role in shaping patient preferences regarding AI. Open and honest communication about the capabilities and limitations of AI systems is essential. Physicians should explain how AI is used to enhance, not replace, human care. Emphasizing the collaborative nature of AI and human expertise can build trust and alleviate anxieties. For instance, a physician explaining that AI assists in early disease detection, allowing for quicker intervention and better outcomes, can positively influence patient perception.
Conversely, a lack of clear communication or a dismissive attitude towards patient concerns can lead to resistance and skepticism.
Patient Preferences: Human Interaction vs. AI Interaction
Healthcare Setting | Human Interaction Preference | AI Interaction Preference | Comments |
---|---|---|---|
Diagnosis/Treatment Planning | High | Low | Patients prefer physician expertise and personalized care. |
Appointment Scheduling | Medium | High | Convenience and efficiency are valued, but personal touch is still appreciated. |
Medication Reminders | Low | High | AI-driven reminders offer convenience and improve adherence. |
Post-operative Care Monitoring | Medium | Medium | AI can provide remote monitoring, but human follow-up is still needed. |
The Role of Trust and Transparency
The success of AI in healthcare hinges significantly on patient trust and transparency. Patients are more likely to accept and utilize AI-powered healthcare solutions when they understand how these systems work and can trust the accuracy and ethical implications of their recommendations. A lack of transparency, on the other hand, can lead to mistrust, reluctance to use the technology, and ultimately, hinder the potential benefits of AI in improving healthcare outcomes.Transparency in AI algorithms directly influences patient preference.
When patients understand the factors contributing to an AI’s diagnosis or treatment plan, they are more likely to accept the recommendations. This understanding fosters a sense of collaboration between patient and technology, reducing anxieties about potential biases or inaccuracies. For example, if an AI system recommends a specific medication, explaining the factors considered (patient history, current symptoms, drug interactions) increases the patient’s confidence in the recommendation and their willingness to follow it.
Strategies to Build Patient Trust in AI-Powered Healthcare Solutions
Building trust requires a multifaceted approach. It’s not enough to simply state that the AI is accurate; patients need concrete evidence and explanations.
- Clear and Accessible Explanations: AI systems should be designed to provide understandable explanations of their recommendations, using plain language and avoiding technical jargon. This could involve providing a summary of the key data points considered and the reasoning behind the decision.
- Data Privacy and Security: Patients must be assured that their data is handled responsibly and securely. This requires clear communication about data usage policies, encryption protocols, and measures to prevent unauthorized access.
- Human Oversight and Accountability: While AI can automate many tasks, human oversight is crucial. Patients need to know that a qualified healthcare professional is ultimately responsible for reviewing and validating AI recommendations.
- Demonstrable Accuracy and Validation: Transparency should include information on the AI’s accuracy rates, validation processes, and limitations. Openly acknowledging the system’s potential for errors builds trust by demonstrating honesty and realism.
- Patient Education and Engagement: Providing educational materials and opportunities for patients to ask questions and voice concerns is essential. Interactive tutorials or workshops can help patients understand how AI works and how it can benefit their care.
Impact of Different Levels of AI System Explainability on Patient Acceptance
The level of explainability directly correlates with patient acceptance. “Black box” AI systems, where the decision-making process is opaque, tend to generate distrust and resistance. Conversely, systems that provide clear and understandable explanations, even if simplified, are more readily accepted. Studies have shown a marked increase in patient satisfaction and willingness to use AI when the reasoning behind the system’s recommendations is made transparent.
For instance, a study comparing a black-box AI diagnostic tool with a more explainable version found significantly higher acceptance rates for the explainable system, even if its diagnostic accuracy was slightly lower.
Ethical Considerations Surrounding the Use of AI in Healthcare Decision-Making and its Effect on Patient Preference
Ethical considerations are paramount. Bias in AI algorithms, for example, can lead to unfair or discriminatory outcomes, severely impacting patient trust. Ensuring fairness, equity, and accountability in AI development and deployment is crucial. Transparency in addressing potential biases and ensuring the equitable application of AI across different patient populations is essential for maintaining patient trust and preference.
Furthermore, the potential for AI to exacerbate existing healthcare disparities needs careful consideration and mitigation strategies. For example, if an AI system is primarily trained on data from one demographic group, it may perform poorly for other groups, leading to biased outcomes and eroding patient trust.
Effectively Communicating the Benefits and Limitations of AI to Patients
Effective communication involves presenting information in a clear, concise, and accessible manner. Avoid technical jargon and focus on the practical benefits of AI for patients. It is equally important to acknowledge the limitations of AI. This honesty fosters trust and avoids unrealistic expectations. For example, instead of saying “This AI will cure your disease,” a more appropriate statement would be “This AI can help your doctor make a more informed diagnosis by analyzing your medical data, which can lead to a more effective treatment plan.” Using real-life examples of successful AI applications in healthcare can also be very effective in building patient confidence.
For example, highlighting AI’s role in early cancer detection or personalized medicine can showcase the positive impact of the technology.
Improving Patient Experience with AI: Patients Accept Healthcare Ai But Dont Prefer It
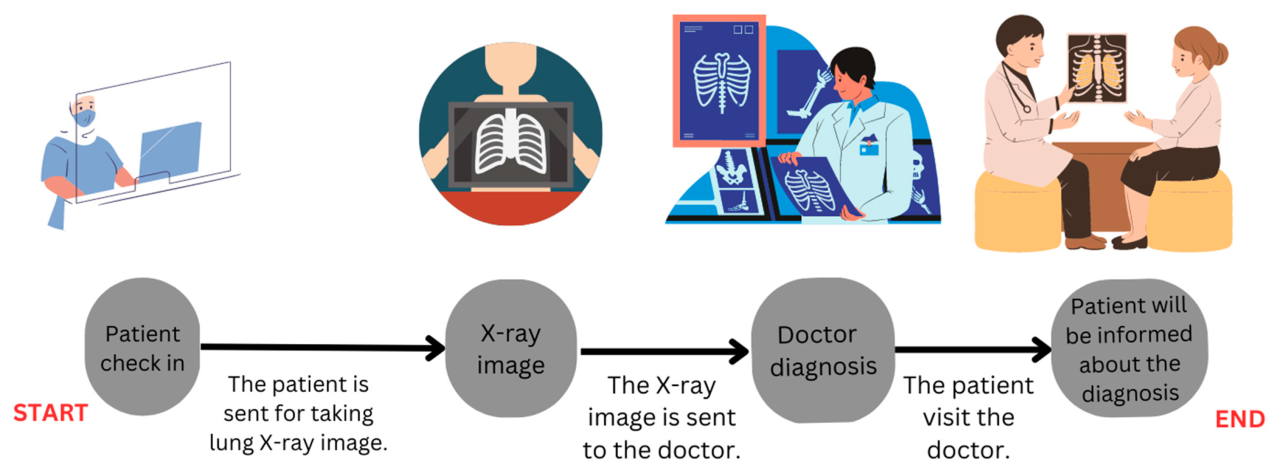
Source: mdpi.com
AI’s potential in healthcare is vast, but its successful implementation hinges on patient acceptance. Simply building sophisticated algorithms isn’t enough; we must prioritize user experience to ensure patients find AI-driven tools helpful and intuitive. A positive patient experience translates to increased trust, better adherence to treatment plans, and ultimately, improved health outcomes.Designing AI-driven healthcare tools requires a deep understanding of patient needs and preferences.
This goes beyond simply making the technology functional; it involves creating a seamless and empathetic interaction that respects patient autonomy and privacy. By focusing on usability and accessibility, we can overcome potential barriers to adoption and foster a more positive relationship between patients and AI in healthcare.
Design Methods to Enhance the User Experience of AI-Driven Healthcare Tools
Effective design methods for AI healthcare tools focus on simplicity, clarity, and personalization. Intuitive interfaces, clear instructions, and minimal technical jargon are crucial. Consider the cognitive load on patients – especially those with health conditions that may impact their ability to understand complex information. Design should account for diverse literacy levels and technological proficiency. For example, a diabetes management app should prioritize clear visual representations of blood sugar levels and medication schedules over complex data graphs.
Similarly, a mental health chatbot should employ natural language processing that feels conversational and supportive rather than clinical and impersonal. Usability testing with target patient populations is essential to identify and address potential usability issues early in the design process.
The Potential of Personalized AI Interventions to Improve Patient Satisfaction
Personalized AI interventions hold immense promise for improving patient satisfaction. By tailoring interventions to individual needs, preferences, and health profiles, AI can deliver more effective and engaging healthcare experiences. For example, a personalized medication reminder system might account for a patient’s daily routine and preferred communication channels (SMS, email, app notification). Similarly, an AI-powered mental health platform could adapt its interventions based on a patient’s progress, providing customized support and resources.
This level of personalization fosters a sense of control and empowers patients to actively participate in their own care, leading to higher satisfaction rates. A real-world example is the use of AI to personalize cancer treatment plans, taking into account individual genetic profiles and tumor characteristics to optimize treatment efficacy and minimize side effects, thereby increasing patient satisfaction with the overall treatment process.
Features That Could Make AI-Powered Healthcare More Appealing to Patients
A number of features can significantly enhance the appeal of AI-powered healthcare tools. Prioritizing these features during the design phase is crucial for increasing patient preference.
- User-friendly interfaces: Simple, intuitive designs that are easy to navigate and understand.
- Personalized experiences: Tailored recommendations and interventions based on individual needs and preferences.
- Clear and concise communication: Avoiding technical jargon and using plain language.
- Data privacy and security: Assuring patients that their data is protected and handled responsibly.
- Accessibility features: Designing tools that are usable by individuals with diverse abilities and technological proficiency.
- Integration with existing healthcare systems: Seamlessly connecting AI tools with electronic health records and other relevant systems.
- Human oversight and support: Providing access to human healthcare professionals when needed.
These features work synergistically; for instance, a user-friendly interface is enhanced by personalization, ensuring the patient’s experience feels relevant and efficient.
The Role of User Feedback in Refining AI Systems and Improving Patient Preference
User feedback is paramount in refining AI systems and improving patient preference. Continuous feedback loops allow developers to identify areas for improvement, address usability issues, and ensure that the AI tools meet the needs of the users. This can be achieved through various methods, such as surveys, interviews, focus groups, and in-app feedback mechanisms. Analyzing user feedback can reveal insights into user behavior, preferences, and pain points, informing design iterations and ensuring the AI tools remain relevant and effective.
For example, if user feedback consistently points to a lack of clarity in a particular feature, developers can redesign that feature to improve its usability and understanding. This iterative process ensures that the AI tools are continuously optimized to meet patient needs and preferences.
Illustrative Examples of User-Centered Design Principles Applied to AI Healthcare Tools
User-centered design principles are essential in developing AI healthcare tools that address patient concerns. Consider a virtual assistant for medication management: instead of simply providing reminders, a user-centered approach would incorporate personalized prompts tailored to the patient’s daily routine, preferred communication method, and any cognitive impairments they may have. Another example is an AI-powered chatbot for mental health support: instead of using clinical language, the chatbot should engage in empathetic and conversational interactions, allowing users to feel comfortable sharing their feelings and concerns.
By prioritizing patient needs and preferences throughout the design process, we can create AI healthcare tools that are not only functional but also empathetic, trustworthy, and genuinely beneficial.
It’s fascinating how patients often grudgingly accept AI in healthcare, even if they don’t actively prefer it. This makes me wonder about the human element; consider the approach of places like the humana centerwell primary care centers walmart , which prioritize in-person care. Perhaps the success of such models hints that even with AI advancements, the personal touch remains crucial, explaining why patient acceptance doesn’t always translate to preference.
Future Directions and Implications
The acceptance of AI in healthcare, while growing, is not necessarily translating into widespread preference. This gap presents both challenges and opportunities for the future of healthcare delivery. Understanding the underlying reasons for this disconnect is crucial for maximizing the benefits of AI while mitigating potential risks. Further research is needed to bridge this divide and ensure AI is not just accepted, but actively embraced by patients.
The long-term implications of patients accepting AI without preferring it are significant. A passive acceptance, driven perhaps by a lack of alternatives or a perceived lack of choice, could lead to lower patient satisfaction, reduced adherence to treatment plans facilitated by AI, and ultimately, poorer health outcomes. This could also impact the industry’s ability to fully realize the cost-saving and efficiency gains promised by AI-driven healthcare.
Furthermore, a lack of genuine preference could hinder the development and implementation of AI tools that are truly patient-centered and address specific needs.
Areas for Future Research
Further research should focus on several key areas. Firstly, qualitative studies exploring the nuanced reasons behind patient acceptance without preference are needed. This involves in-depth interviews and focus groups to uncover underlying anxieties, misconceptions, and unmet needs. Secondly, comparative studies examining the effectiveness of different AI implementation strategies in fostering genuine patient preference are crucial. Finally, longitudinal studies tracking patient experiences and outcomes over time, comparing AI-assisted care with traditional methods, will provide invaluable insights into the long-term impact of AI on patient preference and overall healthcare quality.
For example, research could compare patient satisfaction and health outcomes in hospitals using AI-powered diagnostic tools versus those relying solely on traditional methods. This would provide empirical data to inform future AI implementation strategies.
It’s fascinating how patients generally accept AI in healthcare, even if they don’t actively prefer it. This acceptance, however, highlights the crucial need for robust security measures, especially considering the hhs healthcare cybersecurity framework hospital requirements cms which are vital to protecting sensitive patient data. Ultimately, building trust in AI hinges not just on functionality, but also on the unwavering security protecting patient information.
Advancements in AI Technology to Bridge the Gap
Advancements in explainable AI (XAI) are crucial. Patients need to understand how AI-driven decisions are made, building trust and transparency. The development of AI systems that are more intuitive and user-friendly, incorporating personalized interactions and adaptive learning, will also enhance patient preference. For instance, an AI-powered chatbot that can adapt its communication style based on individual patient preferences, offering clear explanations of its recommendations, is likely to increase patient trust and satisfaction.
Similarly, the development of AI systems that integrate seamlessly with existing healthcare workflows, minimizing disruption to the patient experience, will also be crucial.
Recommendations for Healthcare Providers
Healthcare providers need to prioritize patient education and engagement. This involves transparent communication about how AI is used in their care, actively soliciting patient feedback, and offering choices whenever possible. Providers should also focus on building trust by demonstrating the benefits of AI in a clear and accessible manner, highlighting improved accuracy, efficiency, and personalized care. Furthermore, actively involving patients in the design and implementation of AI systems, ensuring their needs and preferences are considered, will be critical in fostering acceptance and preference.
A hospital system, for example, could establish a patient advisory board to provide input on the design and implementation of new AI-powered tools.
A Hypothetical Future Scenario with High Patient Preference for AI
Imagine a future where AI is seamlessly integrated into healthcare. Patients use AI-powered personal health assistants to manage their conditions, receive personalized recommendations, and communicate with their healthcare providers. AI-driven diagnostic tools provide accurate and timely diagnoses, leading to faster and more effective treatments. AI systems personalize treatment plans, tailoring them to individual patient needs and preferences, improving adherence and outcomes.
Patient data is securely managed and used to improve healthcare services, with strong privacy protections in place. In this scenario, AI is not just accepted, but actively preferred because it has demonstrably improved patient care, convenience, and overall well-being. This scenario, while hypothetical, is achievable through a patient-centric approach to AI implementation, prioritizing transparency, trust, and personalized experiences.
Ultimate Conclusion
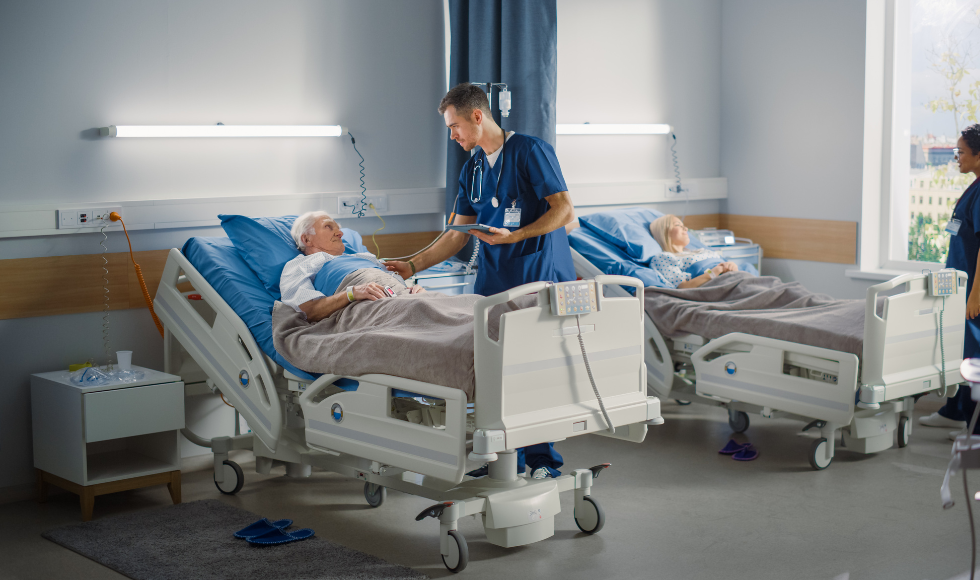
Source: mcmaster.ca
So, while patients may grudgingly accept AI in healthcare due to its increasing prevalence and perceived benefits like efficiency, a true preference remains elusive. Building trust and transparency is paramount; clear communication about AI’s capabilities and limitations is crucial. By focusing on user-centered design, personalized interventions, and fostering open communication between patients and healthcare providers, we can move beyond mere acceptance to genuine enthusiasm for AI’s potential to improve healthcare experiences.
The future of AI in healthcare hinges on addressing these concerns and building a system that prioritizes both efficiency and the human connection.
User Queries
What are the biggest privacy concerns patients have about AI in healthcare?
Patients worry about data security breaches, unauthorized access to their medical information, and the potential for misuse of their personal data by AI systems.
How can doctors better explain AI’s role to patients?
Doctors should use clear, concise language, focusing on the benefits and limitations of AI, and emphasizing the continued importance of the human doctor-patient relationship.
Are there any examples of AI successfully improving patient experience?
AI-powered chatbots offering 24/7 support, personalized medication reminders, and virtual assistants scheduling appointments have shown promise in improving patient convenience and satisfaction.
What is the role of regulation in building patient trust in AI?
Strict regulations regarding data privacy, algorithmic transparency, and system accountability are essential to building patient trust and ensuring responsible AI implementation.