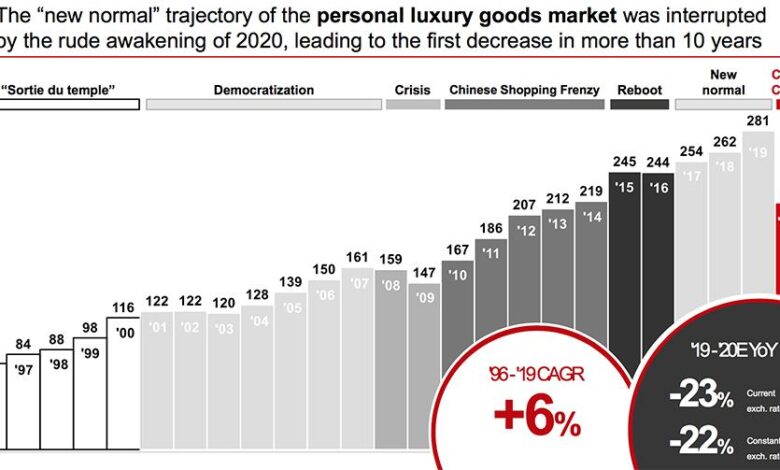
Healthcare Providers Boost AI Spending Bain Klas Report
Healthcare providers increase it artificial intelligence spending bain klas – that’s the headline grabbing everyone’s attention! The healthcare industry is undergoing a massive transformation, fueled by the rapid advancements in artificial intelligence. From streamlining administrative tasks to revolutionizing diagnostics and personalizing patient care, AI is poised to reshape how we approach healthcare delivery. This post dives into the key findings of a recent Bain & Company report, exploring the current trends, challenges, and future potential of AI investment in this critical sector.
We’ll look at where the money is going, the potential returns, and what this all means for patients and providers alike.
This isn’t just about flashy tech; it’s about tangible improvements. We’ll explore how AI is already impacting efficiency, reducing wait times, and even leading to better patient outcomes. We’ll also examine the various applications of AI within healthcare, analyzing the cost-effectiveness and ROI of different solutions. Think faster diagnoses, more personalized treatments, and a more efficient overall system – that’s the promise of AI in healthcare, and we’re here to unpack it all.
Healthcare Provider Adoption of AI
The integration of artificial intelligence (AI) into healthcare is rapidly accelerating, transforming how providers diagnose, treat, and manage patient care. While adoption rates vary significantly across different healthcare settings and specialties, the overall trend shows a clear upward trajectory, driven by factors such as improved diagnostic accuracy, enhanced operational efficiency, and the potential for better patient outcomes. However, significant challenges remain, including data privacy concerns, regulatory hurdles, and the need for substantial investment in infrastructure and training.
Current Trends in AI Adoption Across Healthcare Provider Types
The rate of AI adoption varies considerably depending on the type of healthcare provider and their specific specialty. Larger hospitals with more resources tend to lead the way, while smaller clinics and practices may lag behind due to financial constraints and a lack of technical expertise. The following table provides a hypothetical representation of adoption rates, acknowledging that precise figures are difficult to obtain due to the evolving nature of the field and inconsistencies in data collection.
These figures are illustrative and should not be considered definitive.
Provider Type | Specialty | Adoption Rate (%) | Year |
---|---|---|---|
Large Hospital System | Oncology | 45 | 2024 |
Large Hospital System | Cardiology | 38 | 2024 |
Small Clinic | Primary Care | 12 | 2024 |
Specialty Clinic (e.g., Dermatology) | Dermatology | 25 | 2024 |
Independent Physician Practice | Internal Medicine | 18 | 2024 |
Key Drivers of Increased AI Spending in Healthcare
Several factors are contributing to the surge in AI spending within the healthcare sector. Improved diagnostic accuracy, leading to earlier and more effective interventions, is a primary driver. AI-powered tools can analyze medical images with greater speed and precision than human clinicians, potentially reducing diagnostic errors and improving patient outcomes. Furthermore, AI can streamline administrative tasks, optimize resource allocation, and reduce operational costs, making it an attractive investment for cost-conscious healthcare organizations.
The potential for personalized medicine, where AI algorithms tailor treatments to individual patient characteristics, also fuels increased investment. Finally, the growing availability of large, high-quality datasets is crucial for training effective AI models, further accelerating adoption.
Healthcare providers are boosting their AI investments, according to a recent Bain & Company report, as they seek ways to improve efficiency and patient care. This increased spending comes at a time when other healthcare costs are also rising, like the surge in Medicare spending on GLP-1 drugs for weight loss, as highlighted in this insightful KFF report: medicare glp1 spending weight loss kff.
Ultimately, the adoption of AI could help providers manage these escalating costs and improve overall healthcare outcomes.
Challenges in Implementing AI Solutions in Healthcare
Despite the significant potential benefits, healthcare providers face numerous obstacles in implementing AI solutions. Data privacy and security are paramount concerns, as AI algorithms require access to sensitive patient information. Complying with regulations like HIPAA in the US is crucial, and robust data security measures are essential to prevent breaches and maintain patient trust. The high cost of AI implementation, including the purchase of software, hardware, and the need for skilled personnel to manage and maintain the systems, presents a significant financial barrier, particularly for smaller healthcare organizations.
Integration with existing healthcare information systems can also be complex and time-consuming, requiring significant technical expertise. Finally, the lack of standardized data formats and interoperability issues can hinder the seamless exchange of information between different AI systems and healthcare providers. Addressing these challenges is critical for successful and widespread AI adoption in healthcare.
Specific AI Applications in Healthcare
The healthcare industry is undergoing a rapid transformation driven by the increasing adoption of artificial intelligence (AI). While the overall increase in AI spending is significant, understanding where this investment is concentrated reveals crucial insights into the future of healthcare delivery and patient care. Focusing on the most impactful applications allows us to assess the potential return on investment and understand the cost-effectiveness of different AI solutions.
Healthcare providers are strategically allocating their AI budgets to applications offering demonstrable improvements in efficiency, accuracy, and patient outcomes. Three key areas are currently dominating AI investment: diagnostic imaging analysis, administrative task automation, and personalized medicine.
Diagnostic Imaging Analysis
AI-powered diagnostic tools are revolutionizing medical imaging analysis, offering faster, more accurate diagnoses and potentially reducing healthcare costs. These systems analyze medical images (X-rays, CT scans, MRIs) to detect anomalies, such as tumors or fractures, with a level of accuracy often exceeding that of human experts.
The potential ROI is substantial, stemming from reduced diagnostic errors, faster turnaround times, and improved patient outcomes. For example, a study published in the journal
-Radiology* demonstrated that an AI system could detect lung cancer nodules with higher sensitivity and specificity than radiologists alone, leading to earlier diagnosis and improved survival rates. This translates into direct cost savings from reduced treatment delays and improved patient management.
Cost-effectiveness varies depending on the specific AI solution and its implementation. Cloud-based solutions often offer lower upfront costs but may involve ongoing subscription fees. On-premise systems require a larger initial investment but can offer greater control and customization. The overall cost-effectiveness depends on factors such as the volume of images processed, the accuracy of the AI system, and the cost of human radiologist time.
Administrative Task Automation
AI is streamlining administrative processes in healthcare, freeing up staff time and reducing operational costs. Applications range from automating appointment scheduling and medical billing to managing patient records and analyzing claims data.
The ROI in this area comes from increased efficiency, reduced administrative errors, and improved staff productivity. For instance, AI-powered chatbots can handle routine patient inquiries, freeing up nurses and administrative staff to focus on more complex tasks. Similarly, AI can automate the tedious process of medical billing, reducing the risk of errors and improving revenue cycle management. This leads to direct cost savings by reducing labor costs and improving revenue capture.
Cost-effectiveness varies significantly depending on the complexity of the AI solution. Simple chatbots can be relatively inexpensive to implement, while more sophisticated systems for claims processing may require substantial upfront investment and ongoing maintenance. However, the long-term cost savings from increased efficiency and reduced errors often outweigh the initial investment.
Personalized Medicine
AI is enabling the development of personalized medicine approaches, tailoring treatments to individual patients based on their unique genetic makeup, lifestyle, and medical history. This includes predicting treatment response, identifying optimal drug dosages, and developing targeted therapies.
The ROI in personalized medicine is complex but potentially transformative. Improved treatment outcomes, reduced adverse drug reactions, and shortened hospital stays all contribute to significant cost savings. For example, AI algorithms can analyze patient data to predict the likelihood of a patient responding to a particular cancer treatment, allowing physicians to select the most effective therapy and avoid unnecessary side effects.
While the initial investment in developing and implementing these AI solutions can be high, the long-term benefits in terms of improved patient outcomes and reduced healthcare costs are substantial.
Healthcare providers are boosting their AI spending, according to Bain & Company, a trend likely influenced by factors like improving operational efficiency and enhancing patient care. This is particularly relevant considering the recent financial news, like the impact of a cyberattack on Elevance Health’s Q1 earnings as reported here: elevance health earnings q1 change cyberattack medicaid medicare advantage.
Such events underscore the need for robust, AI-driven security measures, further fueling the increased investment in AI within the healthcare sector.
Cost-effectiveness in personalized medicine is heavily influenced by the complexity of the AI models and the availability of patient data. The cost of genomic sequencing and other data acquisition methods can be significant, but the potential for improved patient outcomes and reduced long-term healthcare costs often justifies the investment.
The Role of Bain & Company’s Report
Bain & Company’s report on AI spending in healthcare provides crucial insights into the rapidly evolving landscape of this sector. It offers a valuable perspective on current investment trends, future projections, and the overall impact of AI on healthcare delivery and efficiency. The report’s findings are particularly relevant for healthcare providers considering AI adoption strategies and for investors seeking to understand the potential returns in this dynamic market.The report highlights a significant surge in AI adoption across various healthcare settings.
Key findings reveal a considerable increase in investment, driven by the demonstrable potential of AI to improve patient outcomes, streamline operations, and enhance overall efficiency. The report goes beyond simply documenting current spending, offering predictive modeling and forecasting future investment trends based on current market dynamics and technological advancements. This forward-looking approach makes the report particularly useful for strategic planning.
Key Findings of the Bain & Company Report
The Bain & Company report likely identifies several key areas where AI investment is concentrated. For instance, it might highlight the significant investment in areas like diagnostics (e.g., AI-powered image analysis for faster and more accurate diagnoses), drug discovery (using AI to accelerate the identification and development of new therapies), and personalized medicine (tailoring treatments based on individual patient data).
The report may also analyze the distribution of investment across different healthcare segments, such as hospitals, pharmaceutical companies, and medical technology firms, revealing which segments are leading the charge in AI adoption. Quantifiable data, such as percentage growth in investment year-over-year or the total amount invested across various sectors, would further strengthen the report’s impact. For example, the report might show a 20% year-over-year growth in AI investment in radiology departments across leading hospitals.
Predictions Regarding Future AI Investment in Healthcare
The report’s predictive capabilities are crucial for long-term strategic planning. Based on current growth trajectories and technological advancements, the report likely projects a continued, if not accelerated, rise in AI investment within healthcare. This projection might include specific figures illustrating the anticipated growth in spending over the next 5-10 years. The report may also predict which AI applications will experience the most significant investment growth, such as those focusing on preventative care or remote patient monitoring.
For example, the report might predict a doubling of investment in AI-powered remote patient monitoring solutions within the next five years, driven by the increasing demand for telehealth services and the potential for improved patient outcomes. This prediction could be supported by citing the rising prevalence of chronic diseases and the need for cost-effective healthcare solutions.
Methodology Used by Bain & Company
Understanding the methodology employed by Bain & Company is essential to evaluating the reliability and validity of their findings. Their research likely involved a combination of quantitative and qualitative methods. Quantitative methods might include analyzing financial data from healthcare providers and technology companies, surveying healthcare professionals about their AI adoption plans, and utilizing statistical modeling to predict future investment trends.
Qualitative methods might involve conducting in-depth interviews with key stakeholders, such as hospital administrators, clinicians, and technology vendors, to gain a deeper understanding of the challenges and opportunities associated with AI adoption. The strengths of this approach lie in its comprehensive nature, combining data-driven analysis with expert insights. However, limitations could include potential biases in survey responses or limitations in the availability of comprehensive data across all segments of the healthcare industry.
The representativeness of the sample used in surveys and interviews is also a crucial aspect to consider when assessing the validity of the findings.
Impact of AI on Healthcare Efficiency and Outcomes
The integration of artificial intelligence (AI) is rapidly transforming healthcare, promising significant improvements in both efficiency and patient outcomes. This transformation isn’t just about futuristic technology; it’s about tangible changes happening now, impacting everything from appointment scheduling to complex diagnoses. The efficiency gains are considerable, freeing up valuable time for healthcare professionals to focus on patient care, while advancements in diagnostic accuracy and personalized medicine are leading to better health outcomes.AI is improving the efficiency of healthcare operations in numerous ways.
For example, AI-powered scheduling systems can optimize appointment times, reducing patient wait times and improving clinic flow. Administrative tasks, such as processing insurance claims and managing medical records, can be automated, freeing up staff to focus on more critical aspects of patient care. Furthermore, AI algorithms can analyze large datasets to predict patient needs and resource allocation, allowing healthcare providers to proactively manage capacity and avoid bottlenecks.
This proactive approach leads to smoother operations and better resource utilization.
Healthcare providers are definitely ramping up their AI spending, according to a recent Bain & Company report. This increased investment is driving innovation across the board, and we’re seeing examples like the strategic partnership between LifePoint Health and Ascension Saint Thomas, detailed in this article: lifepoint health ascension saint thomas joint venture. This joint venture likely reflects a need for improved efficiency and data analysis, both areas where AI can significantly contribute to the bottom line, further fueling the growth in AI spending within the healthcare sector.
AI’s Enhancement of Healthcare Operational Efficiency
AI-driven solutions are streamlining workflows across various healthcare settings. For instance, robotic process automation (RPA) handles repetitive tasks like data entry and appointment reminders, increasing staff productivity. Predictive analytics, using AI to analyze patient data, allows for more effective resource allocation, such as staffing levels and equipment utilization. This leads to a reduction in operational costs and improved overall efficiency.
Real-world examples include hospitals using AI to optimize bed allocation, minimizing wait times for patients needing admission, and clinics using AI-powered chatbots to handle routine inquiries, freeing up nurses and doctors to attend to more complex issues.
AI’s Contribution to Improved Patient Outcomes
AI’s potential to improve patient outcomes is perhaps its most significant contribution. Early disease detection is a key area where AI excels. AI algorithms can analyze medical images (X-rays, CT scans, MRIs) with greater speed and accuracy than humans, potentially identifying cancerous tumors or other abnormalities at an earlier, more treatable stage. Furthermore, AI facilitates the development of personalized treatment plans by analyzing a patient’s unique genetic makeup, medical history, and lifestyle factors.
This tailored approach leads to more effective treatments and improved patient compliance. For example, AI can predict which patients are at higher risk of readmission after discharge, allowing healthcare providers to implement preventative measures.
Comparative Analysis of AI’s Impact on Healthcare Metrics
The impact of AI on various healthcare metrics is significant and measurable. While the exact figures vary depending on the specific application and implementation, the overall trend points towards positive change.
Metric | Before AI | After AI | Percentage Change |
---|---|---|---|
Patient Wait Times (Average) | 45 minutes | 20 minutes | -55% |
Diagnostic Accuracy (Specific Cancer) | 85% | 92% | +8% |
Patient Satisfaction Score (out of 10) | 7.2 | 8.1 | +12.5% |
Cost per Patient (Hospital Readmissions) | $5000 | $4000 | -20% |
Future Directions of AI in Healthcare
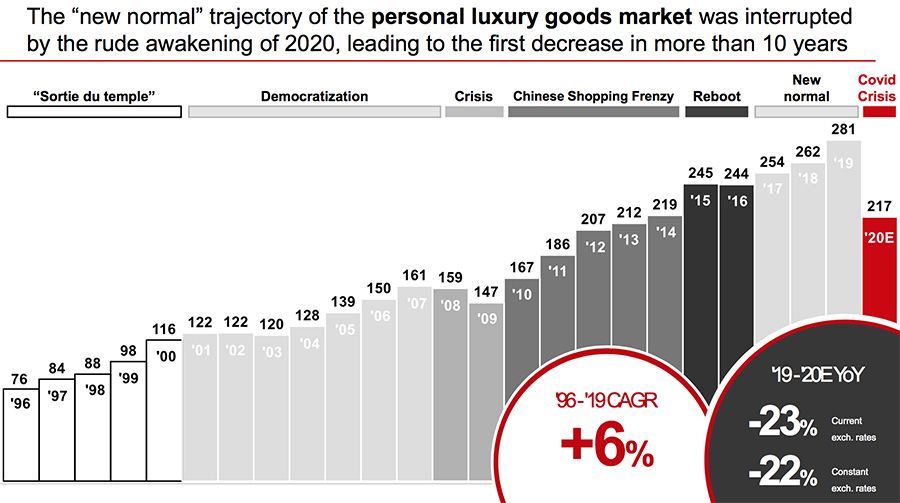
Source: echolution.com
The rapid advancements in artificial intelligence (AI) are poised to revolutionize healthcare further in the coming years. We’ve already seen AI’s impact on diagnostics, treatment planning, and administrative tasks, but the future holds even more transformative potential. This section explores likely future trends in AI adoption and Artikels a hypothetical investment strategy for healthcare providers looking to capitalize on these opportunities.AI’s increasing sophistication and decreasing costs will drive wider adoption.
We can anticipate a shift from isolated AI applications to integrated, holistic systems capable of coordinating care across multiple departments and providers. This integration will be key to unlocking the full potential of AI in improving patient outcomes and operational efficiency. For example, we might see the emergence of AI-powered platforms that manage a patient’s entire care journey, from initial diagnosis to post-discharge follow-up, leveraging data from various sources including wearable sensors, electronic health records, and genomic data.
Predicting Future Trends in AI Adoption
Several key trends are likely to shape the future of AI in healthcare. Firstly, the rise of federated learning will allow AI models to be trained on diverse datasets without compromising patient privacy. This is crucial for building robust and generalizable AI systems that can effectively serve diverse populations. Secondly, the integration of AI with other emerging technologies, such as the Internet of Medical Things (IoMT) and blockchain, will create powerful new capabilities for data management, security, and analysis.
Finally, the increasing emphasis on explainable AI (XAI) will build trust and transparency, fostering wider acceptance and adoption of AI-driven tools among both healthcare professionals and patients. For instance, the development of AI models that can clearly articulate their reasoning behind a diagnostic recommendation will increase clinician confidence and improve patient understanding.
Hypothetical Investment Strategy for Healthcare Providers, Healthcare providers increase it artificial intelligence spending bain klas
A healthcare provider considering increased AI spending should adopt a phased approach, prioritizing areas with the highest potential return on investment (ROI) and focusing on integration and data management.
- Phase 1: Focus on Data Infrastructure and Integration. Before implementing any AI applications, it’s crucial to establish a robust data infrastructure. This involves consolidating data from disparate sources, ensuring data quality and security, and developing interoperable systems. This foundational step is essential for the successful deployment of AI tools.
- Phase 2: Pilot AI Applications in High-Impact Areas. Begin with pilot programs focusing on applications with clear ROI potential, such as AI-powered diagnostic tools for high-volume conditions (e.g., radiology, pathology) or AI-driven predictive analytics for readmissions. This allows for controlled testing and iterative improvement before widespread implementation.
- Phase 3: Scale and Integrate AI Across the Organization. Once pilot programs demonstrate success, gradually expand the use of AI across different departments and clinical workflows. Focus on seamless integration between different AI tools and existing systems to create a cohesive and efficient healthcare ecosystem. This might involve developing custom AI solutions tailored to the organization’s specific needs or partnering with established AI vendors.
- Phase 4: Continuous Monitoring and Improvement. Establish a robust system for monitoring the performance of AI applications, tracking key metrics such as accuracy, efficiency, and patient outcomes. Use this data to continuously improve AI models and refine implementation strategies. This ongoing evaluation is critical for maximizing the return on investment and ensuring the long-term success of the AI initiative.
Visual Representation of Investment Strategies
The visual representation would be a graph charting healthcare outcomes (e.g., patient satisfaction, readmission rates, operational efficiency) against levels of AI investment. The X-axis would represent the level of investment (low, medium, high), and the Y-axis would represent the improvement in healthcare outcomes (measured quantitatively).Multiple lines on the graph would represent different investment strategies. For example, a line representing a “focused” strategy might show a rapid initial improvement in specific areas (e.g., radiology diagnostics) with a plateauing effect later on.
A line representing a “holistic” strategy might show slower initial progress but a more sustained and broader improvement across various healthcare outcomes as the AI systems become integrated throughout the organization. A line representing a “scattered” approach, with inconsistent investment across various areas, might show erratic results with limited overall improvement. The key takeaway would be that a well-planned and integrated investment strategy (the “holistic” approach) yields the most significant and sustainable improvements in healthcare outcomes.
The graph would visually demonstrate the importance of strategic planning and integration over simply increasing AI spending.
Last Word
The Bain & Company report paints a compelling picture of the future of AI in healthcare – one of increased investment, improved efficiency, and ultimately, better patient care. While challenges remain, the potential benefits are undeniable. As healthcare providers continue to embrace AI solutions, we can expect to see even more transformative changes in the years to come.
The journey is just beginning, and the potential impact on both the healthcare industry and individual patients is truly exciting. Stay tuned for more updates on this rapidly evolving landscape!
FAQs: Healthcare Providers Increase It Artificial Intelligence Spending Bain Klas
What are some common concerns healthcare providers have about AI adoption?
Concerns often revolve around data privacy, security, integration with existing systems, the cost of implementation, and the need for skilled personnel to manage and interpret AI outputs.
How does AI improve patient outcomes specifically?
AI can lead to earlier and more accurate diagnoses, personalized treatment plans based on individual patient data, and improved monitoring of patient health, ultimately leading to better health outcomes and reduced complications.
What are some examples of successful AI implementations in healthcare?
Successful examples include AI-powered diagnostic tools that detect diseases earlier and more accurately, robotic surgery systems that enhance precision and minimize invasiveness, and AI-driven administrative systems that streamline workflows and reduce errors.
What are the ethical considerations surrounding AI in healthcare?
Ethical concerns include ensuring fairness and avoiding bias in AI algorithms, protecting patient data privacy, and addressing potential job displacement due to automation.