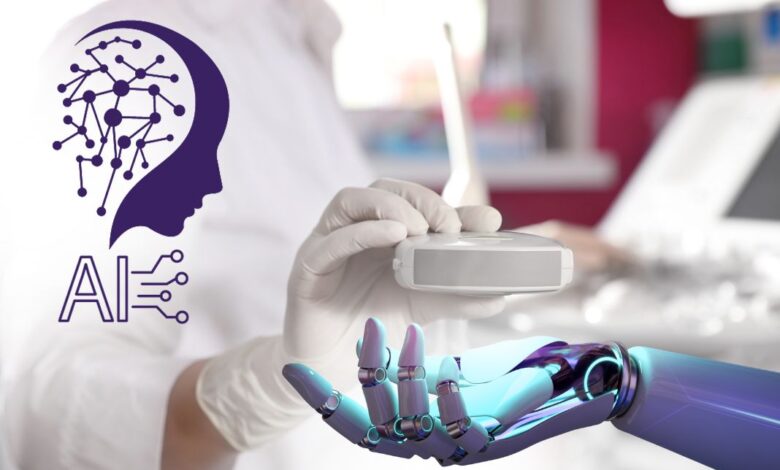
AI Use Medical Imaging Klas Research
AI use medical imaging Klas research is revolutionizing healthcare! This fascinating field blends cutting-edge artificial intelligence with the power of medical imaging to diagnose diseases earlier and more accurately. We’re diving deep into the world of AI algorithms, exploring how they analyze medical images (think X-rays, MRIs, CT scans), and discussing the incredible potential – and challenges – this technology presents.
From deep learning techniques to ethical considerations, we’ll unpack it all.
Imagine a future where AI assists doctors in detecting subtle signs of cancer, heart disease, or neurological disorders long before they become visible through traditional methods. That’s the promise of AI in medical imaging, and Klas research is leading the charge. This isn’t just science fiction; it’s happening now, and the implications are profound. We’ll explore the specific AI techniques employed, the datasets used to train these powerful models, and the rigorous evaluation methods ensuring accuracy and reliability.
Introduction to AI in Medical Imaging (Klas Research Focus)
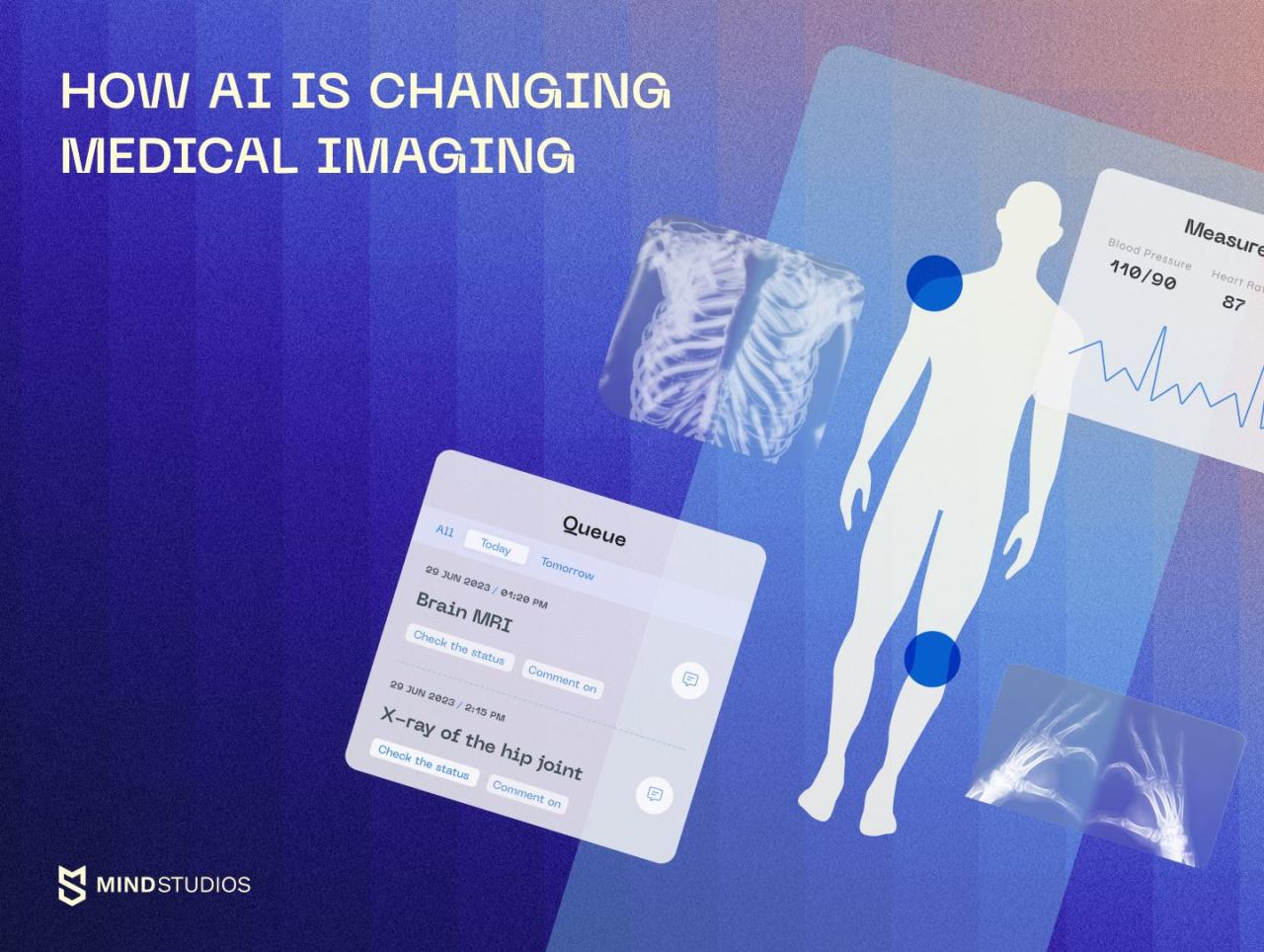
Source: themindstudios.com
Artificial intelligence (AI) is rapidly transforming medical imaging analysis, offering the potential to revolutionize diagnosis, treatment planning, and patient care. Klas research, a significant area within this field, focuses on leveraging AI algorithms to extract meaningful information from medical images, improving accuracy and efficiency compared to traditional methods. This involves the development and application of sophisticated machine learning models trained on vast datasets of medical images, enabling computers to identify patterns and anomalies that might be missed by the human eye.AI’s application in medical imaging analysis, particularly within Klas research, presents both significant opportunities and considerable challenges.
The sheer volume of data involved, the need for robust algorithms capable of handling variations in image quality and patient characteristics, and the ethical considerations surrounding AI-driven decision-making are all key aspects that need careful consideration. However, the potential rewards are immense, promising more accurate diagnoses, earlier detection of diseases, and personalized treatment strategies.
AI Algorithms in Medical Image Analysis
Klas research utilizes a variety of AI algorithms, including convolutional neural networks (CNNs), recurrent neural networks (RNNs), and other deep learning architectures. CNNs, for example, are particularly well-suited for analyzing image data due to their ability to learn hierarchical representations of features. These algorithms are trained on large datasets of annotated medical images, learning to identify subtle patterns indicative of specific diseases or conditions.
For instance, a CNN might be trained to detect subtle variations in tissue density that are indicative of early-stage lung cancer, allowing for earlier intervention and improved patient outcomes. RNNs, on the other hand, are useful for analyzing sequential data, such as time-series of medical images, which can be crucial for monitoring disease progression or treatment response.
AI’s use in medical imaging, particularly KLAS research on its effectiveness, is a fascinating area. The implications are huge, especially considering leadership changes like the recent retirement of AdventHealth CEO Terry Shaw, as reported here: adventhealth ceo retire terry shaw. This transition might influence how quickly new AI technologies are adopted, impacting the future of AI-driven medical imaging analysis and its impact on patient care.
Challenges in Implementing AI in Medical Imaging
One significant challenge lies in the availability of high-quality, annotated data. Training effective AI models requires large, well-labeled datasets, which can be expensive and time-consuming to create. Furthermore, ensuring data privacy and security is paramount, given the sensitive nature of medical information. Another challenge involves the validation and regulatory approval of AI-based medical imaging tools. Rigorous testing and clinical trials are essential to ensure the safety and efficacy of these technologies before they can be widely adopted.
Finally, integrating AI tools into existing clinical workflows can be complex and require significant changes to established practices.
Potential Benefits of AI-Driven Medical Imaging
The potential benefits of AI in medical imaging are substantial. AI-powered systems can improve diagnostic accuracy, leading to earlier and more effective treatment. For example, AI algorithms have shown promise in detecting subtle signs of diabetic retinopathy, a leading cause of blindness, allowing for timely intervention to prevent vision loss. Furthermore, AI can assist radiologists and other healthcare professionals by automating tasks such as image segmentation and annotation, freeing up their time to focus on more complex cases.
This increased efficiency can improve patient throughput and reduce waiting times. Ultimately, AI-driven medical imaging has the potential to enhance the quality of patient care and make healthcare more efficient and accessible. A real-world example is the use of AI in analyzing mammograms, leading to a reduction in false positives and improved detection rates of breast cancer. This results in fewer unnecessary biopsies and more timely treatment for patients with cancer.
Specific AI Techniques Used in Medical Imaging (Klas Research)
The application of Artificial Intelligence (AI) in medical imaging, particularly within the framework of Klas research, has revolutionized diagnostic capabilities and treatment planning. Several powerful AI techniques are driving this progress, each offering unique strengths and weaknesses depending on the specific application. This section delves into three prominent AI techniques commonly employed in Klas research, comparing their functionalities and suitability for various medical imaging tasks.
Deep Learning in Medical Image Analysis
Deep learning, a subset of machine learning, utilizes artificial neural networks with multiple layers to extract complex features from medical images. These networks learn directly from the data, identifying patterns and relationships that might be missed by human observers. In Klas research, deep learning excels at tasks such as image classification (e.g., identifying cancerous tissue), object detection (e.g., locating tumors), and image segmentation (e.g., delineating the boundaries of organs).
The hierarchical structure of deep learning models allows for the progressive extraction of increasingly abstract features, leading to highly accurate predictions. However, deep learning models typically require large amounts of labeled data for training, which can be a significant bottleneck. Furthermore, their “black box” nature can make it difficult to interpret their decisions, raising concerns about transparency and trust.
Convolutional Neural Networks (CNNs) for Image Processing, Ai use medical imaging klas research
Convolutional Neural Networks are a specialized type of deep learning architecture particularly well-suited for processing grid-like data, such as images. CNNs employ convolutional layers that scan the image using filters, detecting local patterns like edges and textures. These patterns are then combined in subsequent layers to recognize more complex features. In Klas research, CNNs are extensively used for tasks such as image classification, segmentation, and registration.
Their ability to efficiently process high-dimensional image data and their inherent capacity for feature extraction make them a powerful tool. However, similar to other deep learning models, CNNs require substantial computational resources for training and can be sensitive to the quality and quantity of training data. Overfitting, where the model performs well on training data but poorly on unseen data, is also a potential challenge.
Recurrent Neural Networks (RNNs) for Temporal Data Analysis
While CNNs excel at analyzing static images, Recurrent Neural Networks are designed to handle sequential data, such as time-series data derived from medical imaging. RNNs maintain an internal state that is updated as they process each element in a sequence. This allows them to capture temporal dependencies and relationships within the data. In Klas research, RNNs find applications in analyzing dynamic medical imaging data, such as cardiac MRI sequences or time-lapse microscopy images.
They can be used to track changes in organ structure over time, predict disease progression, or monitor treatment response. However, RNNs can be challenging to train due to the vanishing or exploding gradient problem, which can hinder the learning process. Their computational demands can also be high, particularly for long sequences.
Comparison of AI Techniques
Technique Name | Description | Advantages | Disadvantages |
---|---|---|---|
Deep Learning | Uses artificial neural networks with multiple layers to extract complex features from data. | High accuracy, can learn complex patterns, suitable for various tasks. | Requires large labeled datasets, “black box” nature, computationally intensive. |
Convolutional Neural Networks (CNNs) | Specialized deep learning architecture for processing grid-like data like images, using convolutional layers to detect local patterns. | Efficient for image processing, good at feature extraction, relatively high accuracy. | Requires substantial computational resources, sensitive to data quality, prone to overfitting. |
Recurrent Neural Networks (RNNs) | Designed to handle sequential data, capturing temporal dependencies and relationships. | Suitable for analyzing time-series medical imaging data, can track changes over time. | Challenging to train, computationally expensive, prone to vanishing/exploding gradients. |
Klas Research Datasets and Data Preprocessing
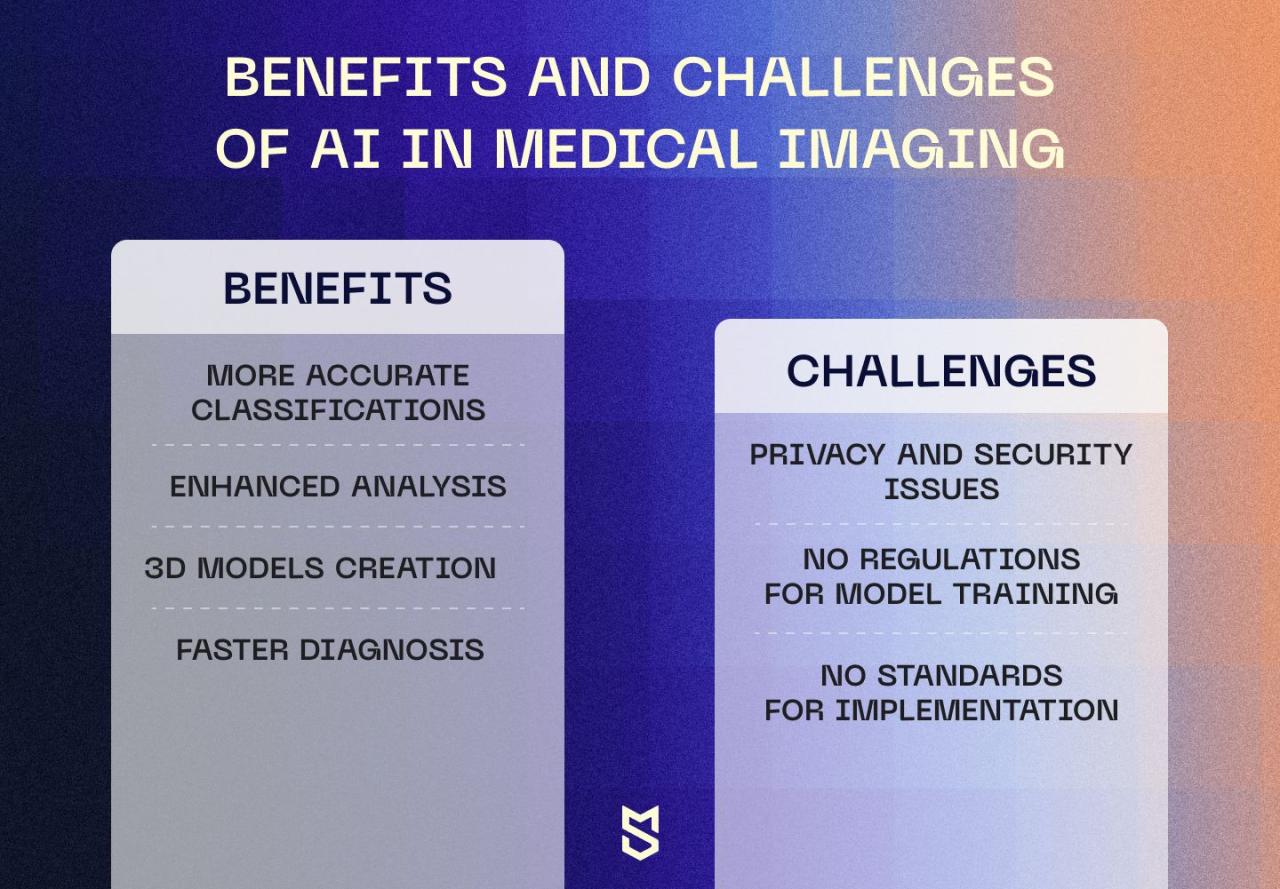
Source: themindstudios.com
The success of AI in medical imaging hinges critically on the quality and quantity of the data used to train and validate the models. Klas research, like any serious endeavor in this field, relies on robust datasets and rigorous preprocessing techniques to ensure the reliability and accuracy of its AI algorithms. The datasets employed are diverse, reflecting the range of imaging modalities and clinical applications under investigation.
Furthermore, careful preprocessing is essential to mitigate the impact of noise and inconsistencies inherent in medical images, thereby improving model performance and generalizability.The types of datasets used in Klas research encompass a variety of medical imaging modalities, including X-rays, CT scans, MRI scans, and ultrasound images. Data size varies considerably depending on the specific research project, ranging from hundreds to thousands of images.
The diversity of the datasets is crucial for training robust models capable of handling variations in image acquisition techniques, patient demographics, and disease presentations. Data quality is paramount, requiring careful curation and annotation by experienced medical professionals to ensure accuracy and consistency in labeling. For instance, a study on lung cancer detection might utilize a dataset of chest X-rays with detailed annotations indicating the presence and location of cancerous nodules, while a study on brain tumor segmentation might leverage MRI scans with meticulous segmentations of tumor regions.
Maintaining high data quality involves rigorous quality control checks to eliminate corrupted or poorly acquired images.
Dataset Characteristics in Klas Research
Klas research emphasizes the importance of diverse and representative datasets. This means including images from a wide range of patients with varying demographics (age, gender, ethnicity), disease severities, and imaging acquisition parameters. For example, in a study involving cardiovascular imaging, the dataset might include images from patients with different stages of coronary artery disease, acquired using various scanner types and protocols.
This diversity helps to mitigate bias and improve the generalizability of the AI models to unseen data in real-world clinical settings. The datasets are typically anonymized to protect patient privacy, complying with all relevant ethical guidelines and regulations. The size of the datasets used depends on the complexity of the task and the desired performance level. Larger datasets generally lead to better model performance, but data acquisition and annotation can be time-consuming and resource-intensive.
Common Preprocessing Steps in Klas Research
Proper preprocessing is vital for optimizing the performance of AI algorithms in medical imaging. Raw medical images often contain noise, artifacts, and inconsistencies that can negatively impact model training and accuracy. Therefore, a series of preprocessing steps are applied to enhance image quality and prepare the data for AI algorithms.
- Image Normalization: This involves adjusting the intensity values of the images to a standard range, ensuring that variations in scanner settings or acquisition protocols do not unduly influence the model’s learning process. For instance, histogram equalization is a common technique used to improve the contrast and uniformity of image intensity.
- Noise Reduction: Medical images are often corrupted by various types of noise, such as Gaussian noise or salt-and-pepper noise. Filtering techniques, such as median filtering or Gaussian smoothing, are applied to reduce the noise while preserving important image features.
- Data Augmentation: To increase the size and diversity of the training dataset, data augmentation techniques are employed. These include random rotations, flips, crops, and scaling of the images. This helps to improve the robustness and generalizability of the AI models to variations in image appearance.
- Image Registration: For multi-modal imaging or longitudinal studies, image registration is crucial to align images from different sources or time points. This ensures that corresponding anatomical structures are consistently located across different images.
- Bias Field Correction: In MRI, for example, intensity inhomogeneities (bias fields) can significantly affect image quality and model performance. Techniques like N4 bias field correction are used to mitigate this issue.
AI Model Development and Evaluation in Klas Research
Developing and training effective AI models for medical image analysis within the Klas research framework involves a rigorous process, encompassing data preparation, model selection, training, and comprehensive evaluation. This process is iterative, with results from each stage informing improvements in subsequent steps. The ultimate goal is to develop models that are accurate, reliable, and clinically useful.The development process typically begins with careful selection of an appropriate AI architecture.
This choice depends heavily on the specific medical imaging task (e.g., classification, segmentation, detection) and the characteristics of the available data. Common architectures include Convolutional Neural Networks (CNNs) for image classification and segmentation, and Recurrent Neural Networks (RNNs) for analyzing temporal sequences of images. Once the architecture is chosen, the model is trained using the pre-processed dataset, optimizing its parameters to minimize a chosen loss function.
This training process often involves techniques like data augmentation to increase the robustness and generalizability of the model. Regularization methods are also employed to prevent overfitting, ensuring the model performs well on unseen data.
Model Training and Hyperparameter Tuning
Training an AI model involves iteratively feeding the model with the prepared data, adjusting its internal parameters to minimize the difference between its predictions and the ground truth. This process is guided by a chosen loss function, which quantifies the error in the model’s predictions. Hyperparameter tuning, the process of adjusting settings that control the training process (e.g., learning rate, batch size, number of epochs), is crucial for achieving optimal model performance.
Techniques like grid search, random search, and Bayesian optimization are often used to efficiently explore the hyperparameter space and find the optimal settings. For example, in a Klas research project focused on detecting lung nodules in CT scans, researchers might use a CNN architecture and tune hyperparameters such as the learning rate and the number of convolutional layers to optimize the model’s sensitivity and specificity in detecting nodules of varying sizes.
Evaluation Metrics
Assessing the performance of AI models in medical imaging requires a comprehensive evaluation using multiple metrics. These metrics provide a nuanced understanding of the model’s strengths and weaknesses, crucial for clinical translation. Commonly used metrics include accuracy, sensitivity, specificity, precision, F1-score, and the area under the receiver operating characteristic curve (AUC-ROC).
Comparison of Evaluation Metrics
Metric | Definition | Interpretation | Example |
---|---|---|---|
Accuracy | (True Positives + True Negatives) / Total | Overall correctness of the model. Can be misleading with imbalanced datasets. | A model with 90% accuracy correctly classifies 90 out of 100 images. |
Sensitivity (Recall) | True Positives / (True Positives + False Negatives) | Proportion of actual positives correctly identified. Important for minimizing false negatives. | A model with 85% sensitivity correctly identifies 85% of all cancerous lesions. |
Specificity | True Negatives / (True Negatives + False Positives) | Proportion of actual negatives correctly identified. Important for minimizing false positives. | A model with 95% specificity correctly identifies 95% of all non-cancerous lesions. |
AUC-ROC | Area under the Receiver Operating Characteristic curve | Measures the model’s ability to distinguish between classes across different thresholds. Higher AUC indicates better performance. | An AUC-ROC of 0.9 indicates excellent discrimination between positive and negative cases. |
Ethical and Practical Considerations
The application of AI in medical imaging, while promising, necessitates a careful consideration of ethical and practical implications. Successfully integrating AI into clinical workflows requires addressing potential biases, ensuring patient privacy, and navigating the complexities of cost, regulatory hurdles, and workflow integration. Failure to do so risks undermining the benefits of this technology and potentially harming patients.The ethical landscape surrounding AI in medical imaging is multifaceted.
My latest research focuses on AI’s application in medical imaging, specifically using KLAS research to understand its impact. The efficiency gains are incredible, and it got me thinking about the broader healthcare workforce – especially considering the challenges highlighted in this article about the ai powered solution to the medical coding worker shortage. This shortage directly impacts the speed of processing medical images, making AI advancements in image analysis even more critical for improving patient care.
Ultimately, both AI-powered image analysis and streamlined coding are key to a more efficient and effective healthcare system.
A key concern revolves around algorithmic bias. AI models are trained on data, and if this data reflects existing societal biases (e.g., underrepresentation of certain demographics), the resulting AI system may perpetuate or even amplify these biases in diagnosis and treatment recommendations. This could lead to disparities in healthcare access and quality, disproportionately affecting vulnerable populations. Furthermore, the use of patient data in AI model training raises significant privacy concerns.
My latest research focuses on applying AI to medical imaging, specifically using KLAS research methodologies. Understanding how AI can detect subtle changes indicative of stroke is crucial, especially when considering the risk factors that make stroke more dangerous , like high blood pressure and diabetes. Early detection, aided by AI, could significantly improve patient outcomes and that’s what drives my work in this fascinating field.
Strict adherence to data anonymization and security protocols is crucial to protect sensitive patient information.
Algorithmic Bias and Fairness
Addressing algorithmic bias requires a multi-pronged approach. Firstly, ensuring diverse and representative datasets for training AI models is paramount. This involves actively collecting data from a wide range of demographics to mitigate biases present in existing datasets. Secondly, rigorous testing and validation of AI models are essential to identify and quantify any biases present. Techniques such as fairness-aware machine learning can be employed to develop algorithms that explicitly account for and minimize biases.
Finally, ongoing monitoring and auditing of deployed AI systems are necessary to detect and address emerging biases over time. For example, if an AI model consistently misdiagnoses skin cancer in patients with darker skin tones, immediate action is required to rectify the underlying issue, perhaps through retraining with a more representative dataset or adjusting the algorithm itself.
Patient Privacy and Data Security
Protecting patient privacy in the context of AI in medical imaging requires robust data governance frameworks. This includes implementing strict data anonymization techniques to remove or mask personally identifiable information from medical images and associated metadata. Secure data storage and access control mechanisms are crucial to prevent unauthorized access or breaches. Furthermore, transparent data usage policies should be established, clearly outlining how patient data will be used for AI development and deployment.
Compliance with relevant regulations, such as HIPAA in the United States and GDPR in Europe, is essential. The use of federated learning, where AI models are trained on decentralized datasets without directly sharing patient data, offers a promising approach to enhancing privacy while still enabling AI development.
Cost and Regulatory Approval
The implementation of AI-based medical imaging systems in clinical settings presents significant practical challenges. The high cost of developing, validating, and deploying these systems can be a barrier to adoption, particularly for smaller healthcare providers. Regulatory approval processes can also be lengthy and complex, requiring substantial time and resources. Furthermore, integrating AI systems into existing clinical workflows requires careful planning and consideration of potential disruptions to established practices.
Strategies to address these challenges include exploring cost-effective AI solutions, streamlining regulatory pathways, and developing user-friendly interfaces that facilitate seamless integration into existing workflows. Government funding and public-private partnerships can also play a vital role in accelerating the adoption of AI in medical imaging. For instance, a hospital system might partner with an AI company to pilot a new AI-based diagnostic tool, sharing the costs and risks while gaining valuable experience and data.
Workflow Integration and Training
Successful integration of AI into clinical workflows requires careful consideration of the human element. Clinicians need adequate training and support to effectively use and interpret the outputs of AI systems. This involves developing comprehensive training programs and providing ongoing support to address any challenges or concerns that arise. Furthermore, the design of AI systems should be user-centric, ensuring that they are intuitive and easy to use within the existing clinical workflow.
The integration process should also involve collaboration between clinicians, IT specialists, and AI developers to ensure that the system meets the specific needs of the healthcare setting. A phased rollout, starting with a pilot program in a limited setting before broader implementation, can help minimize disruptions and allow for iterative improvements.
Future Directions of AI in Medical Imaging (Klas Research): Ai Use Medical Imaging Klas Research
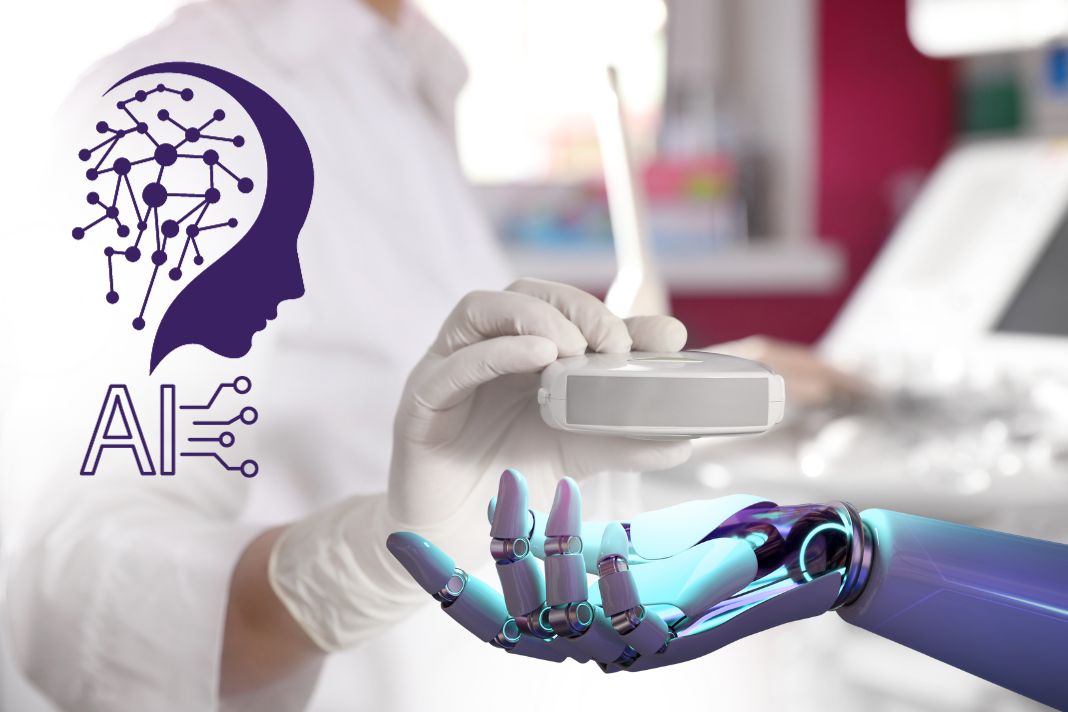
Source: medicalimagingindia.com
The application of AI in medical imaging, while showing remarkable progress within the Klas research framework, remains a field ripe for exploration and advancement. Future research should focus on refining existing techniques, addressing limitations, and leveraging emerging technologies to unlock the full potential of AI for improved diagnostics and patient care. This involves not only enhancing accuracy and efficiency but also ensuring ethical and responsible deployment.The integration of emerging technologies promises to significantly impact Klas research.
Explainable AI (XAI), for instance, is crucial for building trust and facilitating clinical adoption. Federated learning offers a pathway to overcome data privacy concerns while still enabling collaborative model training across multiple institutions. These advancements will pave the way for more robust, reliable, and ethically sound AI solutions in medical imaging.
Explainable AI (XAI) in Klas Research
Improving the transparency and interpretability of AI models is paramount. Current deep learning models, while highly accurate, often function as “black boxes,” making it difficult to understand their decision-making processes. XAI techniques, such as attention mechanisms, SHAP values, and LIME, can provide insights into the factors influencing AI’s diagnoses. Future Klas research will focus on incorporating XAI methods to enhance model explainability, thereby fostering trust among clinicians and enabling better clinical decision-making.
For example, visualizing which specific regions of an MRI scan contributed most to a cancer diagnosis could significantly aid radiologists in confirming or refuting the AI’s assessment.
Federated Learning for Enhanced Data Privacy in Klas Research
Data privacy and security are significant challenges in medical imaging AI. Federated learning offers a solution by allowing multiple institutions to collaboratively train a shared AI model without directly sharing their sensitive patient data. Each institution trains a local model on its own data, and only model updates (parameters) are shared with a central server to aggregate and improve the global model.
This approach protects patient confidentiality while still leveraging the benefits of large datasets for improved model performance. A future Klas research project could focus on implementing and evaluating a federated learning framework for the detection of pulmonary nodules in CT scans, comparing its performance and privacy preservation capabilities to traditional centralized training methods. This would involve collaborating with multiple hospitals to collect data, develop and train the federated model, and then assess its accuracy and efficiency.
Expected outcomes would include a robust model capable of accurate nodule detection while maintaining strict adherence to patient data privacy regulations.
Multimodal AI for Comprehensive Diagnostics in Klas Research
Integrating information from multiple imaging modalities (e.g., combining CT and MRI data) holds significant potential for improving diagnostic accuracy. Future research within the Klas framework will explore the development of multimodal AI models capable of integrating diverse data sources for a more holistic assessment. This could involve developing sophisticated fusion techniques to combine information from different imaging modalities and clinical data, leading to more accurate and comprehensive diagnoses.
For example, combining MRI images showing brain structure with PET scans highlighting metabolic activity could significantly improve the diagnosis and prognosis of brain tumors. A future research project could focus on developing a multimodal AI model for Alzheimer’s disease diagnosis, combining MRI, PET, and cognitive test data to improve early detection and risk stratification. The expected outcome is a more accurate and reliable diagnostic tool that helps clinicians provide better care to patients with Alzheimer’s disease.
Last Recap
The integration of AI into medical imaging, particularly within the framework of Klas research, holds immense promise for transforming healthcare. While challenges remain in areas like data privacy, algorithm bias, and regulatory hurdles, the potential benefits for improved patient outcomes and healthcare efficiency are undeniable. The journey ahead is exciting, with emerging technologies like explainable AI and federated learning poised to further enhance the capabilities and accessibility of AI-driven medical imaging.
The future of diagnosis may well be shaped by the innovative work happening in this field today.
Question Bank
What is Klas research?
Klas research, in this context, refers to a specific area of research focusing on the application of AI to medical imaging. The exact meaning of “Klas” would need further clarification based on the original source material.
What are the limitations of AI in medical imaging?
Current limitations include the need for large, high-quality datasets, potential biases in algorithms, the “black box” nature of some AI models making interpretation difficult, and the cost and complexity of implementation.
How is patient privacy protected in AI medical imaging?
Strict data anonymization techniques, secure data storage, and adherence to regulations like HIPAA (in the US) are crucial for protecting patient privacy in AI medical imaging research and applications.